Unlocking AI Power: Smarter, Safer Health and Fitness Plans
- Introduction: Harnessing AI to Transform Your Health and Fitness Regimen
- Introduction: Harnessing AI to Transform Your Health and Fitness Regimen
- AI’s Expanding Role in Personalized Health and Fitness
- What You Can Achieve with AI Integration
- Navigating Innovation with Ethics and Practical Realities
- Prerequisites: Understanding the Foundations of AI in Fitness
- Prerequisites: Understanding the Foundations of AI in Fitness
- Core AI Concepts Behind Fitness Solutions
- Essential Hardware: Wearables, Sensors, and Smartphones
- Data Privacy: A Non-Negotiable Foundation
- Technical Requirements and Managing Expectations
- Preparation: Setting Up Your AI-Enabled Fitness Environment
- Preparation: Setting Up Your AI-Enabled Fitness Environment
- Choosing the Right AI Fitness Apps and Devices
- Calibrating Wearable Sensors and Establishing Baseline Metrics
- Managing Privacy, Ethical Data Usage, and Goal Alignment
- Final Thoughts
- Step-by-Step Integration of AI into Your Health and Fitness Plan
- Step-by-Step Integration of AI into Your Health and Fitness Plan
- 1. Establishing Your Baseline with AI Diagnostics
- 2. Generating Personalized Workout Plans with Continuous Adaptation
- 3. Tracking Nutrition with AI Dietary Analysis
- 4. Monitoring Recovery and Sleep with AI Insights
- 5. Embracing Iterative Optimization: The AI Feedback Loop
- Technical Rationale: How AI Algorithms and Sensors Enhance Fitness Outcomes
- Technical Rationale: How AI Algorithms and Sensors Enhance Fitness Outcomes
- Machine Learning Models: Personalizing Your Workout Through Data
- Self-Learning Motion Sensors: Adapting to Your Unique Movements
- Physiological Data Analytics: Monitoring Recovery and Injury Risk
- Challenges: Data Quality, Bias, and Sensor Limitations
- Bringing It All Together: A Balanced View
- Troubleshooting and Limitations: Navigating Common Challenges with AI Fitness Tools
- Troubleshooting and Limitations: Navigating Common Challenges with AI Fitness Tools
- Sensor Inaccuracies: When Data Doesn’t Tell the Whole Story
- The Human Factor: Why AI Alone Can’t Replace Expertise
- Privacy and Ethical Concerns: Who’s Watching Your Health Data?
- When to Trust AI—and When to Take the Wheel
- Advanced Techniques and Future Directions: Optimizing and Expanding Your AI Fitness Ecosystem
- Advanced Techniques and Future Directions: Optimizing and Expanding Your AI Fitness Ecosystem
- Integrating Genetic Data and AR/VR: The New Frontier of Personalized Fitness
- Multi-Modal AI Platforms: Uniting Exercise, Nutrition, and Mental Wellness
- Critical Evaluation and Ethical Use of Emerging AI Fitness Technologies
- Looking Ahead: The Evolving Role of AI in Democratizing Fitness
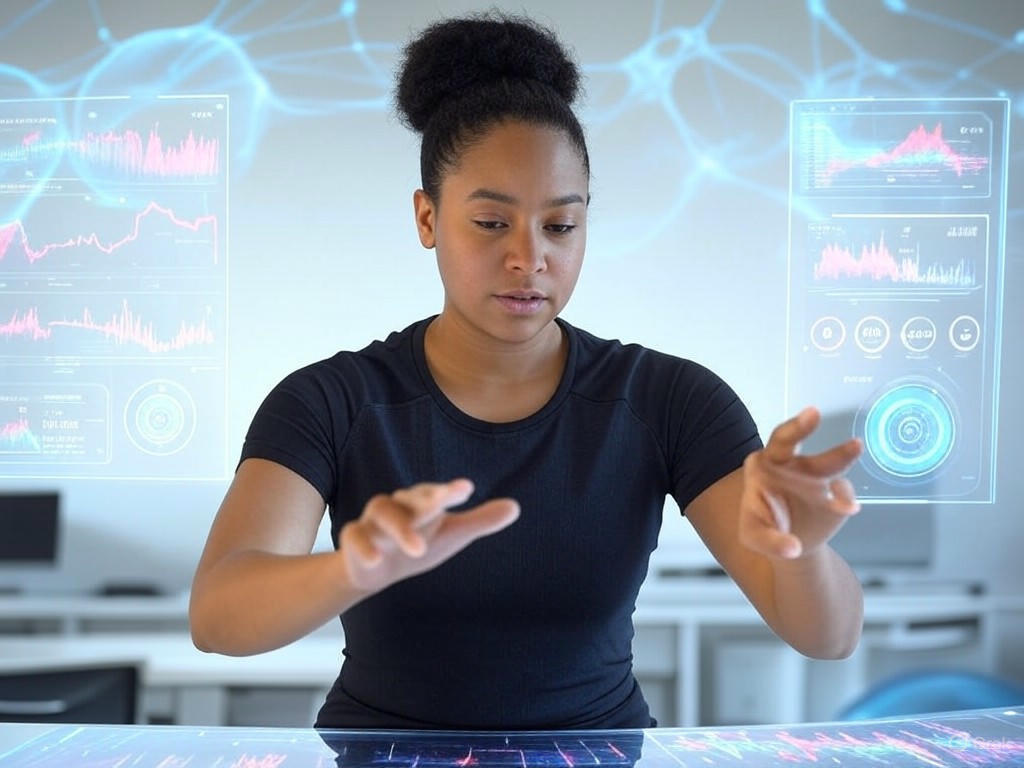
Introduction: Harnessing AI to Transform Your Health and Fitness Regimen
Introduction: Harnessing AI to Transform Your Health and Fitness Regimen
Imagine a workout that adapts dynamically to your body’s daily condition or a nutrition plan that evolves seamlessly with your shifting needs and preferences. This vision is no longer a distant future; it’s becoming reality today, propelled by advances in artificial intelligence (AI) that are revolutionizing personalized health and fitness.
AI’s Expanding Role in Personalized Health and Fitness
By 2025, AI has moved beyond novelty status to become a foundational technology shaping personalized wellness experiences. At CES 2025, new smart fitness devices like the Amp home gym and Gym Monster 2 showcased AI’s capability to monitor your exercise form in real time and provide tailored coaching, effectively replicating personal trainer guidance. These innovations exemplify a broader shift toward adaptive technologies that evolve with your progress, continuously refining your workouts for optimal results.
AI’s impact extends far beyond exercise routines. Wearable platforms such as Whoop analyze physiological signals to offer actionable insights on recovery, strain, and sleep quality, empowering users to make informed decisions about their health. Fitness apps increasingly use machine learning algorithms to generate customized routines accessible to users regardless of gym access or personal trainer availability. It is important, however, to recognize that the effectiveness of AI-driven recommendations depends heavily on the quality, diversity, and comprehensiveness of underlying training data. Some applications may be optimized for younger, healthier populations, potentially limiting their suitability for older adults or individuals with disabilities.
What You Can Achieve with AI Integration
Integrating AI into your health and fitness regimen can deliver tangible, measurable benefits, including:
-
Optimized Workouts: AI-powered platforms like Freeletics and JuggernautAI dynamically tailor training plans based on your goals, performance metrics, and recovery status. They adjust exercises in real time to match evolving strength and endurance levels, enhancing efficiency and effectiveness.
-
Data-Informed Nutrition: Nutrition guidance powered by AI is rapidly advancing. Innovative systems generate personalized meal plans—for instance, smoothie recipes designed to manage obesity and type 2 diabetes—integrating real-time nutrient data alongside environmental sustainability considerations. These solutions help align your diet with health objectives and ecological impact.
-
Injury Prevention: Predictive analytics are emerging as transformative tools, using movement patterns and physiological data to identify injury risks before symptoms arise. This enables proactive interventions that can reduce downtime and improve long-term health outcomes.
-
Sustained Motivation: AI fitness apps incorporate gamification strategies to maintain user engagement. Examples include Sweatcoin’s rewards system and Strava’s adaptive challenges, which foster community support and help sustain motivation crucial for long-term adherence.
Navigating Innovation with Ethics and Practical Realities
Despite the compelling promise of AI-driven health optimization, it is vital to approach this space with a balanced understanding of ethical and practical considerations. Many AI fitness applications collect sensitive personal data, including biometric information and location, which may be shared with third parties such as advertisers or insurers, raising significant privacy concerns.
Moreover, AI outputs are only as unbiased and accurate as their training datasets. Current limitations in dataset inclusivity can lead to less reliable or safe recommendations for underrepresented groups.
Finally, while AI can augment health and fitness practices, it cannot replace essential human elements such as personalized coaching, medical advice, and self-awareness. As AI technologies continue to evolve, maintaining transparency, ensuring user control over data, and embedding robust ethical frameworks will be paramount to realizing AI’s full potential in serving individual and societal well-being.
AI holds transformative potential in health and fitness—enabling smarter workouts, personalized nutrition, injury risk mitigation, and motivational support tailored uniquely to each individual. Yet, realizing this potential requires thoughtful engagement with both opportunities and challenges, moving beyond hype to embrace evidence-based, ethical, and practical applications. This article will explore these dimensions, equipping you to harness AI responsibly and effectively to elevate your health regimen.
Benefit | Description | Examples |
---|---|---|
Optimized Workouts | AI-powered platforms tailor training plans dynamically based on goals, performance, and recovery, adjusting exercises in real time. | Freeletics, JuggernautAI |
Data-Informed Nutrition | AI generates personalized meal plans integrating nutrient data and sustainability considerations to align diet with health and ecological goals. | Smoothie recipes for obesity and type 2 diabetes management |
Injury Prevention | Predictive analytics use movement and physiological data to identify injury risks early for proactive intervention. | Movement pattern analysis tools |
Sustained Motivation | Gamification strategies in AI fitness apps foster engagement and community support to maintain long-term adherence. | Sweatcoin rewards system, Strava adaptive challenges |
Prerequisites: Understanding the Foundations of AI in Fitness
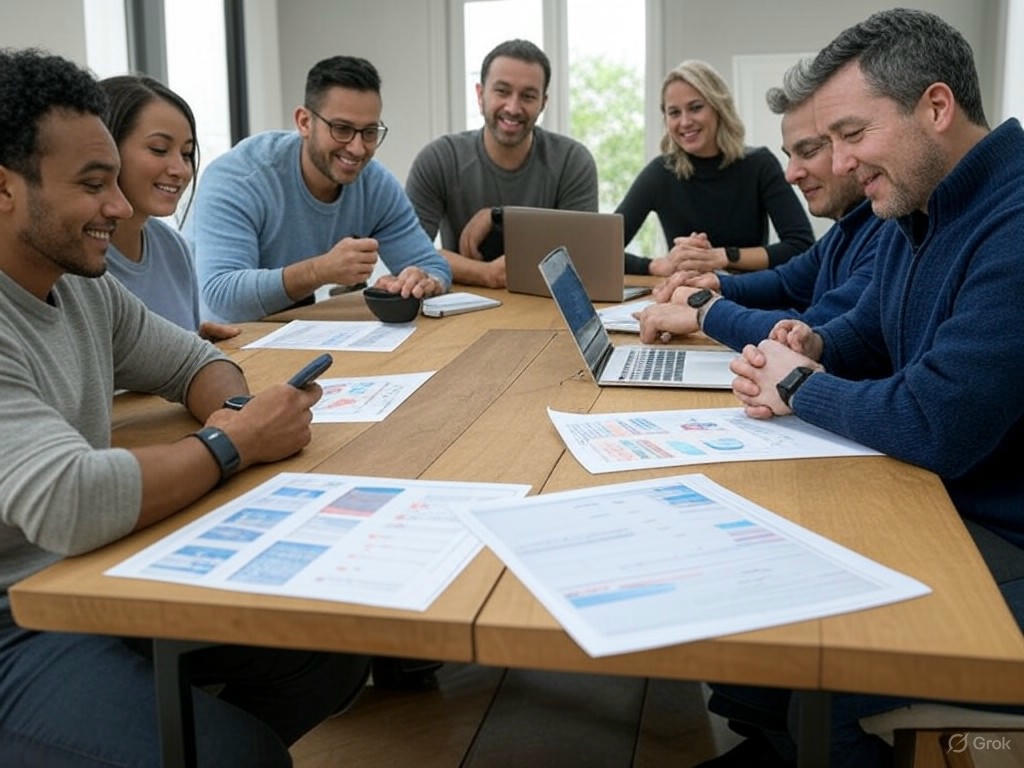
Prerequisites: Understanding the Foundations of AI in Fitness
Before integrating AI-powered fitness tools into your regimen, it is essential to build a solid understanding of the underlying technologies, hardware requirements, and privacy considerations that define their capabilities and limitations. This foundational knowledge helps set realistic expectations and empowers you to make informed decisions, avoiding frustration or disengagement from unrealistic promises.
Core AI Concepts Behind Fitness Solutions
AI fitness applications leverage several key technologies working in concert:
-
Machine Learning (ML): The driving force behind personalized workout plans and predictive insights. ML algorithms analyze extensive user data—ranging from heart rate variability to sleep patterns—to forecast recovery needs and predict adherence to exercise routines. For example, studies using datasets from tens of thousands of users have enabled apps to identify who is most likely to stick to a program, allowing for tailored motivational strategies and guidance.
-
Computer Vision: Many fitness apps utilize computer vision via smartphone cameras or specialized sensors to assess movement and exercise form in real time. This technology provides immediate feedback on posture and technique, closely emulating human trainers by automating visual analysis to enhance safety and effectiveness during workouts.
-
Sensor Fusion: Wearables combine inputs from multiple sensors—such as accelerometers, gyroscopes, and heart rate monitors—using sensor fusion techniques. This integrative approach produces a comprehensive and accurate representation of your physical state. By merging motion data with biometric signals, AI systems better assess strain, fatigue, and injury risk than any single sensor could alone.
It’s important to recognize that these AI components are not infallible. Their accuracy and relevance depend heavily on the quality, diversity, and representativeness of training data. Many AI fitness models are primarily trained on data from younger, healthy adults, which may limit their applicability for older users or those with disabilities. Understanding these nuances is critical for evaluating AI-generated recommendations critically and maintaining realistic expectations.
Essential Hardware: Wearables, Sensors, and Smartphones
AI fitness tools depend on compatible hardware to collect, process, and transmit data. Key considerations in 2025 include:
-
Wearables: The wearable tech market has matured well beyond basic step counters. Devices such as Garmin Venu 3, Fitbit Charge 6, and Oura Ring 4 offer advanced health tracking features—including heart rate variability, sleep staging, oxygen saturation, and recovery metrics. The global shipment of wearable sensors surpassed 1.2 billion units in 2024, underscoring their ubiquity.
-
Sensor Accuracy and Placement: While wrist-based trackers are the most common due to convenience, chest straps still provide superior heart rate accuracy. Smart rings like Oura offer a discreet form factor focused on sleep and recovery monitoring. Selecting the appropriate device depends on your fitness goals, comfort preferences, and required data fidelity.
-
Smartphone Compatibility and Ecosystem Integration: Most fitness wearables sync with apps on iOS or Android, though cross-platform support varies. Apple Watch users benefit from seamless integration with Apple Fitness, while Samsung Galaxy Watch owners enjoy Samsung’s ecosystem connectivity. Understanding your device ecosystem is vital for a smooth and cohesive user experience.
-
Emerging Devices: Innovations like smart glasses and AI-powered home gym equipment (e.g., Amp home gym and Gym Monster 2 showcased at CES 2025) are expanding AI’s reach into fitness, offering new modalities for interactive and adaptive training.
Data Privacy: A Non-Negotiable Foundation
AI fitness platforms collect increasingly sensitive personal data, including biometric signals, location, and behavioral patterns, making privacy a paramount concern.
-
Regulatory Landscape: The U.S. data privacy environment is fragmented, relying on sector-specific laws and state regulations like California’s CCPA and CPRA. HIPAA provides a baseline for health data protection but struggles to keep pace with rapidly evolving digital health technologies.
-
Transparency and Consent: Many users remain unaware of how their data is collected, processed, or shared, jeopardizing trust. Leading AI fitness platforms embed “privacy by design” principles, implementing robust data security measures and clear consent mechanisms from the outset.
-
Ethical Use and Bias: AI systems can inherit biases present in their training data, potentially disadvantaging marginalized groups. Ethical AI governance involves continuous auditing of algorithms to ensure fairness and the avoidance of one-size-fits-all recommendations that fail to account for user diversity.
-
User Control: Practical steps for safeguarding privacy include scrutinizing app permissions, choosing platforms with strong privacy commitments, limiting unnecessary data sharing, and leveraging privacy-enhancing technologies where available.
Technical Requirements and Managing Expectations
Successfully leveraging AI in your fitness journey requires aligning hardware, software, and user engagement while maintaining a grounded perspective on AI’s capabilities.
-
Device Compatibility: Verify that your smartphone and wearable meet the technical requirements of your chosen AI fitness app. Some apps require specific operating system versions or hardware features such as advanced sensors or cameras.
-
User Data Input and Interaction: Many AI fitness applications personalize recommendations based on user-provided information such as age, weight, fitness level, and goals. Accurate and complete data entry is critical, as AI outputs are only as good as their inputs—”garbage in, garbage out.”
-
App Ecosystem and Integration: Top AI fitness platforms often integrate with complementary health and wellness apps (e.g., Gymverse, Hevy for logging workouts; Whoop for recovery tracking; Strava for community challenges), creating a seamless, multi-dimensional experience that encompasses nutrition, sleep, and activity.
-
Realistic Expectations: AI excels at pattern recognition, adaptive suggestions, and data-driven insights but cannot replace human intuition, motivation, or complex medical advice. For instance, Whoop’s AI analyzes physiological metrics to recommend recovery times but does not account for psychological factors influencing adherence.
By understanding these technical and practical boundaries, users can avoid falling into hype traps and instead harness AI as a trustworthy assistant for smarter, safer, and more effective fitness journeys.
In summary, embarking on AI-enhanced fitness requires a firm grasp of the core AI technologies powering personalization, an informed selection of compatible and accurate hardware, vigilant attention to data privacy, and a clear-eyed view of AI’s strengths and limits. This foundation is essential to unlock AI’s full potential as a transformative tool in your health and fitness regimen.
Category | Details | Examples / Notes |
---|---|---|
Core AI Concepts |
|
|
Hardware Requirements |
|
|
Data Privacy |
|
|
Technical Requirements & Expectations |
|
|
Preparation: Setting Up Your AI-Enabled Fitness Environment
Preparation: Setting Up Your AI-Enabled Fitness Environment
Before integrating AI into your fitness routine, setting up the right environment is essential. Success requires more than just downloading an app or wearing a device; it depends on thoughtful selection, precise calibration, and responsible data management. Here’s how to start effectively and set yourself up for meaningful progress.
Choosing the Right AI Fitness Apps and Devices
The market offers over 70 workout apps tested in 2025 alone, catering to a wide range of fitness goals and budgets. The key is to align your technology choices with your personal objectives rather than following trends.
-
Define your fitness goals clearly: Whether you aim to improve strength, endurance, flexibility, or recovery, clarity guides your selection. For instance, strength-focused users may prefer apps like Home Gym Club Training or Caliber Strength Training, which offer structured programs and personalized coaching. Cycling enthusiasts can benefit from Zwift’s immersive virtual worlds and social competition features.
-
Consider coaching style and budget: AI fitness apps differ in personalization and human interaction. Future app connects users to elite trainers at a premium cost of $199/month, while FitnessAI automates routine generation with minimal human input at a lower price point. Choose based on your desired coaching intensity and financial commitment.
-
Complement apps with wearables: Devices such as Whoop and Garmin provide deep physiological insights. Whoop excels in tracking recovery and sleep quality using heart rate variability metrics, making it ideal for cardio athletes. Garmin’s Fenix 8 and Forerunner 965 watches blend advanced health tracking with built-in training programs tailored for runners and multisport athletes.
-
Evaluate user experience and ecosystem compatibility: If you are invested in Apple’s ecosystem, Apple Fitness Plus integrates seamlessly with Apple Watch and iOS devices. Similarly, ensure your chosen apps and devices sync smoothly with your smartphone or health platforms to avoid siloed data that can limit AI effectiveness.
Calibrating Wearable Sensors and Establishing Baseline Metrics
The accuracy and usefulness of AI-driven fitness guidance hinge on the quality of your data. Proper calibration and establishing reliable baseline metrics are non-negotiable for meaningful insights.
-
Follow specific calibration protocols: For example, WHOOP recommends wearing the strap snugly on the wrist or upper arm, ensuring consistent skin contact with sensor LEDs to maximize heart rate and heart rate variability (HRV) accuracy. Correct placement reduces signal artifacts and improves data quality.
-
Gather comprehensive baseline health data: Before allowing AI to tailor your workouts, input accurate information on your current fitness level, weight, resting heart rate, sleep patterns, and any pre-existing health conditions. This baseline serves as the anchor for AI algorithms to track your progress and dynamically adjust plans.
-
Incorporate diverse data sources: Combine wearable sensor data with manual entries like nutrition logs, perceived exertion ratings, and mood tracking. Apps such as Gymverse and Hevy enable logging of equipment used, workout locations, and calorie intake, enriching datasets for more precise coaching recommendations.
-
Regularly monitor and recalibrate: Fitness is an evolving journey. Periodic reassessment of your baseline metrics allows AI to recalibrate your program to accommodate improvements or setbacks. This iterative process is critical for injury prevention and sustainable progress.
Managing Privacy, Ethical Data Usage, and Goal Alignment
As you entrust AI with sensitive health data, privacy and ethics must be front and center in your preparation.
-
Understand privacy settings and data policies: Many fitness apps and wearables collect sensitive personal information, sometimes sharing it with third parties such as advertisers or insurance companies. Privacy experts at IDX recommend opting out of “aggregated data usage” and location-sharing features where possible. Carefully review privacy policies to understand how your data is handled.
-
Demand transparency and control: Ethical AI means you should have access to your data, with the ability to modify or delete it. Seek apps that explain how their algorithms operate and allow customization of data inputs feeding your personalized plans.
-
Set SMART goals aligned with AI capabilities: Specific, Measurable, Achievable, Relevant, and Time-bound (SMART) goals improve your chance of success by 42%, according to fitness psychology research. AI tools perform best when your objectives are clearly defined and realistic. For example, if your app emphasizes progressive overload for strength gains, set incremental weight increase targets rather than vague aims like “get stronger.”
-
Maintain human judgment alongside AI recommendations: AI provides data-driven suggestions but not absolute truths. Balance AI insights with your self-awareness, expert advice, and bodily feedback. Human-in-the-loop approaches safeguard against overreliance on AI outputs and support adaptive decision-making.
Final Thoughts
Laying a strong foundation for AI-enhanced fitness involves deliberate choices about your tools, meticulous attention to data quality, and vigilant privacy management. By selecting apps and wearables that align with your unique goals, calibrating sensors carefully, and safeguarding your personal information, you enable AI to augment your health and performance effectively.
Remember, AI is a powerful assistant—not a magic wand. Ground your expectations in evidence while actively engaging with your fitness journey as a knowledgeable participant. This balanced approach will help you harness AI’s transformative potential responsibly and sustainably.
Aspect | Details |
---|---|
Fitness Goals | Define clearly (strength, endurance, flexibility, recovery); choose apps like Home Gym Club Training, Caliber Strength Training, Zwift based on goals |
Coaching Style & Budget | Future app: elite trainers at $199/month; FitnessAI: automated routines, lower cost |
Wearables | Whoop: recovery & sleep tracking (HRV); Garmin Fenix 8 & Forerunner 965: advanced tracking, training programs |
User Experience & Ecosystem | Apple Fitness Plus integrates with Apple Watch/iOS; ensure app/device compatibility to avoid siloed data |
Calibration Protocols | Wear straps snugly for accurate HR & HRV; consistent sensor contact improves data quality |
Baseline Metrics | Input fitness level, weight, resting HR, sleep patterns, health conditions for AI tailoring |
Data Sources | Combine sensor data with manual logs (nutrition, exertion, mood) via apps like Gymverse, Hevy |
Recalibration | Regularly monitor and update baseline metrics to adapt AI programs |
Privacy & Data Policies | Opt out of aggregated data/location sharing; review app privacy policies carefully |
Transparency & Control | Access, modify, delete data; apps should explain algorithms and allow input customization |
Goal Setting | Use SMART goals (Specific, Measurable, Achievable, Relevant, Time-bound) aligned with AI capabilities |
Human Judgment | Balance AI recommendations with self-awareness, expert advice, and bodily feedback |
Step-by-Step Integration of AI into Your Health and Fitness Plan
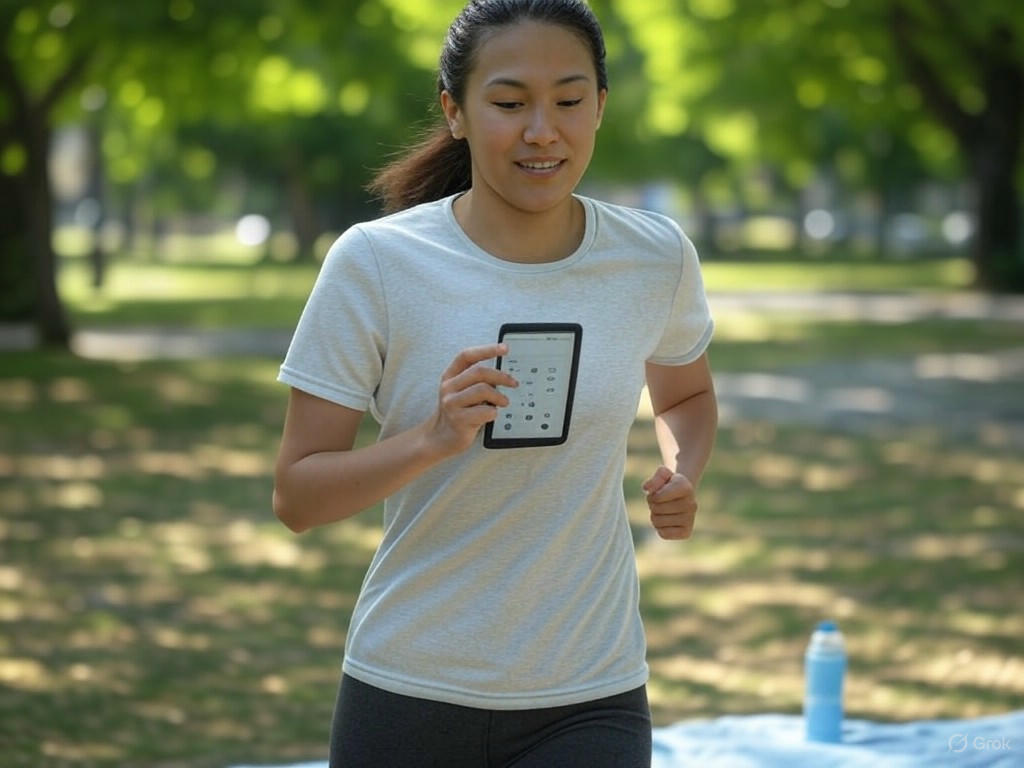
Step-by-Step Integration of AI into Your Health and Fitness Plan
How can you transform AI’s potential into meaningful improvements in your health and fitness routine? The answer lies in a thoughtful, iterative process—starting with an accurate baseline assessment and progressively adding layers of personalization, real-time feedback, nutrition insights, and recovery monitoring. Let’s explore this integration step-by-step, grounded in the latest AI capabilities and realistic expectations.
1. Establishing Your Baseline with AI Diagnostics
Before starting any workout or nutrition plan, obtaining a precise and comprehensive snapshot of your current health is essential. AI diagnostics have become indispensable tools for this purpose.
Platforms like Scispot harness deep learning to analyze extensive medical data, identifying underlying health conditions and monitoring vital signs with diagnostic accuracies exceeding 90% in some cases. The seamless integration of AI with wearable devices enables continuous remote monitoring, turning what once seemed futuristic into a practical reality.
For instance, AI-powered body scanning apps such as FitXpress allow you to perform a detailed 3D scan using just two smartphone photos. This delivers precise body composition metrics and tracks changes over time, providing far more granular insights than traditional scales or tape measures. Establishing such a concrete baseline is crucial for setting realistic and measurable goals.
Keep in mind: AI diagnostics are powerful tools that can save time and reduce healthcare costs, potentially saving billions annually. However, they are not replacements for professional medical oversight. Human interpretation remains vital to contextualize AI outputs within your overall health profile.
2. Generating Personalized Workout Plans with Continuous Adaptation
With your baseline established, AI can design workout plans tailored to your unique profile, goals, and available equipment. Platforms powered by ChatGPT or apps like Fitbod analyze your inputs and dynamically adjust routines as you progress, eliminating the guesswork and plateaus common in generic programs.
Take Juggernaut.AI as an example: this adaptive strength training app modifies your sets, reps, and weights in real time based on performance data. It functions like a personal trainer recalibrating your session after each set, optimizing for both safety and effectiveness.
Real-time feedback technologies built on human pose estimation are revolutionizing exercise supervision. Using just your smartphone camera, AI detects subtle deviations in posture and form, providing immediate corrections to reduce injury risk. This biofeedback loop enhances motivation and engagement by ensuring every rep counts.
Fitness environments are evolving too. The upcoming Fred Fitness gym exemplifies a fully AI-driven facility, blending on-site trainers with AI-powered personalized workouts and monitoring systems, showcasing how AI is reshaping fitness spaces.
3. Tracking Nutrition with AI Dietary Analysis
Exercise is only part of the equation; nutrition represents the other critical pillar. AI is transforming nutritional tracking by making it more precise and personalized.
Imagine snapping a photo of your meal and instantly receiving a detailed nutritional breakdown. The AI food scanner developed by NYU Tandon exemplifies this capability—it identifies foods and quantifies calories, proteins, fats, and carbohydrates with notable accuracy.
Beyond calorie counting, AI systems synthesize scientific research, personal health data, and extensive food databases to generate personalized dietary recommendations. These algorithms optimize meal variety and nutritional balance, adapting plans based on your progress and preferences. This approach aligns with the rising demand for personalized nutrition, a market projected to reach $10.5 billion by 2029.
However, users should remain cautious: the accuracy of AI dietary advice depends heavily on the quality of input data and robustness of nutritional models. AI guidance should complement, not replace, consultation with nutrition professionals when necessary.
4. Monitoring Recovery and Sleep with AI Insights
Recovery is often the overlooked pillar of fitness, yet AI is bringing it into focus like never before.
Advanced sleep tracking tools such as Sleeptracker-AI leverage deep learning to analyze sleep stages and detect disorders with clinical-level accuracy rivaling polysomnography. These systems offer actionable insights, such as identifying disrupted REM cycles or environmental factors hindering rest, empowering users to optimize sleep hygiene.
Wearables like Whoop utilize AI-driven monitoring of strain, recovery, and heart rate variability (HRV), feeding data back into personalized training plans. This creates a closed-loop system where workouts, nutrition, and recovery continuously inform and optimize one another.
This iterative feedback loop significantly compresses the traditional slow trial-and-error of fitness optimization into a dynamic, responsive process. As expert Stephen Malina notes, fast feedback loops extend our sense of self into our tools, increasing engagement and accelerating improvement.
5. Embracing Iterative Optimization: The AI Feedback Loop
The common thread connecting all these phases is AI’s capacity for continuous, iterative optimization. At every stage—from diagnostics and workouts to nutrition and recovery—AI collects data, learns from it, and refines its recommendations.
Think of AI as a GPS recalculating your route in real time, adjusting to traffic, detours, or changing goals. This responsiveness keeps your fitness journey on the most efficient and effective path.
It’s crucial to maintain a balanced perspective: while AI excels at pattern recognition and adaptation, it requires human input to establish meaningful goals, interpret nuanced context, and uphold ethical standards—such as safeguarding data privacy and preventing over-reliance on automation.
In summary, integrating AI into your health and fitness regimen is about amplifying traditional methods with data-driven precision, personalization, and real-time responsiveness. Today’s AI tools enable you to establish a robust health baseline, dynamically tailor workouts, effortlessly track nutrition, and intelligently optimize recovery—all interconnected through iterative feedback loops. Embracing this approach can transform your regimen into a smarter, safer, and more effective journey toward your well-being goals.
Step | Focus Area | Description | Example Tools/Platforms | Key Benefits | Considerations |
---|---|---|---|---|---|
1 | Establishing Baseline with AI Diagnostics | Obtain a precise and comprehensive snapshot of current health using AI diagnostics integrated with wearable devices for continuous monitoring. | Scispot, FitXpress | High diagnostic accuracy (90%+), detailed body composition analysis, time and cost savings | Not a replacement for professional medical oversight; human interpretation needed |
2 | Generating Personalized Workout Plans | Design and dynamically adapt workout routines tailored to individual profiles and progress, including real-time feedback on exercise form. | ChatGPT-powered platforms, Fitbod, Juggernaut.AI, Fred Fitness gym | Eliminates guesswork, prevents plateaus, enhances safety and effectiveness, improves motivation | Requires quality data input; technology still evolving in real-time correction accuracy |
3 | Tracking Nutrition with AI Dietary Analysis | Use AI to analyze meal photos and synthesize personalized dietary recommendations based on scientific research and personal data. | NYU Tandon AI food scanner | Precise nutritional breakdown, personalized diet optimization, adapts to progress and preferences | Accuracy depends on input data quality; advice should complement professional nutrition consultation |
4 | Monitoring Recovery and Sleep with AI Insights | Leverage AI to analyze sleep patterns and recovery metrics, integrating data into personalized training adjustments. | Sleeptracker-AI, Whoop wearables | Clinical-level sleep analysis, actionable insights for recovery, closed-loop optimization of training and rest | Requires consistent wearable usage; needs human interpretation for contextual decisions |
5 | Embracing Iterative Optimization: The AI Feedback Loop | Continuous data collection and learning by AI to refine recommendations across diagnostics, workouts, nutrition, and recovery. | All integrated AI systems | Real-time route recalculation for fitness goals, maximizes efficiency and effectiveness | Human input needed for goal setting, ethics, privacy, and avoiding over-reliance on automation |
Technical Rationale: How AI Algorithms and Sensors Enhance Fitness Outcomes
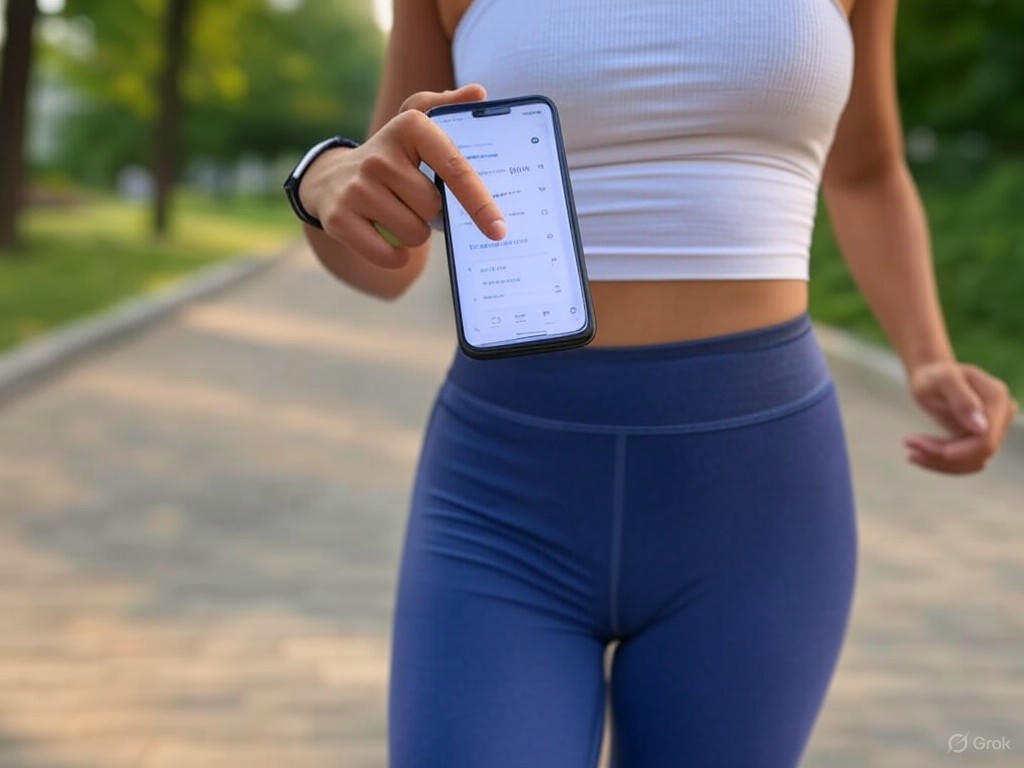
Technical Rationale: How AI Algorithms and Sensors Enhance Fitness Outcomes
What sets AI-driven fitness tools apart from simple step counters? At their core, these systems combine sophisticated machine learning algorithms, self-learning sensors, and physiological data analytics to transform raw data into actionable insights. This synergy enables tailored workouts, recovery monitoring, and even injury risk prediction. Understanding these technical foundations allows us to distinguish genuine innovation from hype and appreciate both the promise and inherent challenges of AI in fitness.
Machine Learning Models: Personalizing Your Workout Through Data
Think of machine learning models as coaches trained by observing millions of diverse workouts across all fitness levels. These AI models analyze extensive datasets—including heart rate variability, motion patterns, workout types, and outcomes—to determine what strategies work best for individuals like you.
For instance, platforms like Whoop analyze physiological data to offer insights on recovery, strain, and sleep quality. Apple’s hybrid approach uniquely blends physiological heart rate kinetics with machine learning to predict heart rate zones during exercise, achieving approximately 67% accuracy compared to laboratory baselines. This hybrid model integrates biological understanding with data-driven adaptation, providing more nuanced and reliable predictions than either method alone.
Many of these models employ reinforcement learning, where the AI continuously tests and refines its workout recommendations based on your feedback and results. Imagine a GPS recalculating your route in real time to avoid traffic jams; similarly, AI adjusts your training load dynamically in response to “traffic” like fatigue signals or injury warnings.
However, the effectiveness of these models hinges on the quality and diversity of their training data. Currently, many AI fitness models are primarily trained on data from young, healthy individuals, which limits their applicability for older adults or people with disabilities. This demographic bias can reduce personalization accuracy and underscores a critical need for more inclusive datasets. Additionally, data sparsity—insufficient examples for certain user profiles—can diminish AI’s predictive power and customization.
Self-Learning Motion Sensors: Adapting to Your Unique Movements
Beyond algorithms, AI fitness tools rely heavily on advanced sensors embedded in wearables. Companies like Bosch Sensortec have developed self-learning motion sensors that do more than just record movement—they interpret and adapt to your unique biomechanics over time.
For example, the BHI260AP smart sensor integrates a 6-axis inertial measurement unit with a programmable microcontroller, enabling real-time processing of complex motions. This allows devices to provide precise feedback on exercise form and technique, critical for injury prevention and maximizing workout effectiveness.
These sensors also monitor physiological markers such as heart rate variability (HRV), a key indicator of recovery and strain. Elite athletes like Cristiano Ronaldo use HRV data to fine-tune training intensity, ensuring adequate recovery before increasing workload. Recent advances combine reinforcement learning with attention-based neural networks to manage the noisy, nonlinear nature of HRV data, enabling more adaptive and personalized recovery monitoring.
Physiological Data Analytics: Monitoring Recovery and Injury Risk
AI’s analysis extends beyond heart rate to a broad spectrum of physiological metrics. Wearables equipped with biosensors can track sweat composition, cortisol levels, muscle activity, and more, offering a holistic view of your fitness state.
Innovative devices like the starfish-shaped heart monitor developed by Mizzou Engineering achieve over 90% accuracy in detecting potential heart problems by integrating AI with sensor data. Similarly, the Withings Omnia Health Scanner combines user-friendly design with advanced analytics to empower users with actionable health insights.
In sports science, AI-driven biomechanical analysis leverages data streams from wearables and video to assess parameters like stride length, ground contact time, and acceleration. This real-time analysis helps identify injury risks, allowing dynamic adjustments to training to prevent overuse or acute injuries.
Challenges: Data Quality, Bias, and Sensor Limitations
Despite rapid technological progress, AI fitness tools face significant challenges. Data quality remains a critical concern; a survey of data professionals found that 81% of companies struggle with AI data quality, which undermines output reliability. In fitness apps, poor data quality can lead to misguided workout recommendations or inaccurate health assessments.
Algorithmic bias is another pressing issue. Many training datasets lack representation from diverse populations, causing AI to underperform for women, older adults, or people with disabilities. This not only limits personalization but also raises ethical concerns about equitable access to effective fitness guidance.
Sensor accuracy is vital but imperfect. While self-learning sensors enhance adaptability, they can still falter with unusual movements, external interference, or improper device fit. Optical heart rate monitors, for example, may struggle with varying skin tones or motion artifacts. Emerging technologies like thin-film ultrasound sensors promise higher resolution and reliability but have yet to reach mainstream adoption.
Additional constraints include battery life limitations and device compatibility issues, which affect continuous data collection and user experience. Data sparsity—when the system lacks sufficient examples for certain user groups or conditions—further restricts AI’s ability to generalize and fine-tune recommendations.
Bringing It All Together: A Balanced View
AI fitness systems function like a finely tuned orchestra: machine learning algorithms interpret diverse data, sensors capture your unique movements and physiology, and analytics translate this into smart training guidance. While these technologies have matured impressively, they are still evolving. Users should remain aware of inherent limitations, particularly regarding inclusivity and data reliability.
In practice, AI fitness tools democratize access to personalized coaching, making expert-level guidance scalable and affordable. They optimize training efficiency, reduce injury risk, and enhance recovery monitoring in ways previously achievable only by elite athletes.
However, just as a GPS relies on up-to-date maps and traffic information, AI fitness tools depend on continuous improvements in data quality, algorithm fairness, and sensor precision. The road ahead requires transparent, ethical AI development and a commitment to inclusivity, ensuring these powerful technologies benefit everyone on their fitness journey.
Component | Description | Examples/Details | Challenges |
---|---|---|---|
Machine Learning Models | Analyze physiological and workout data to personalize training and recovery recommendations using reinforcement learning. | Whoop analyzes recovery, strain, sleep; Apple blends heart rate kinetics with ML for heart rate zone prediction (~67% accuracy). | Bias due to training mostly on young, healthy individuals; data sparsity; limits personalization for older adults and people with disabilities. |
Self-Learning Motion Sensors | Embedded sensors in wearables that adapt to individual biomechanics, interpreting complex motion and physiological signals in real time. | BHI260AP sensor with 6-axis IMU and microcontroller; track HRV for recovery; used by athletes like Cristiano Ronaldo. | Sensor accuracy issues with unusual movements, interference, or poor fit; optical sensors affected by skin tone and motion artifacts; battery life and compatibility constraints. |
Physiological Data Analytics | Analyzes wide range of biosensor data including sweat, cortisol, muscle activity to monitor recovery and predict injury risk. | Starfish-shaped heart monitor (90%+ accuracy for heart issues); Withings Omnia Health Scanner; biomechanical analysis of stride, ground contact, acceleration. | Requires high data quality; complex noisy physiological data; challenges in real-time, accurate injury risk prediction. |
Overall Challenges | Issues affecting AI fitness tools across components. | Data quality problems (81% companies report issues); algorithmic bias; sensor limitations; battery and compatibility issues; data sparsity. | Undermines reliability and personalization; ethical concerns about inclusivity and fairness; technical constraints limit performance and adoption. |
Troubleshooting and Limitations: Navigating Common Challenges with AI Fitness Tools
Troubleshooting and Limitations: Navigating Common Challenges with AI Fitness Tools
How reliable is your AI fitness companion, really? While AI-powered fitness apps and wearables offer personalized guidance and data-driven insights, users often face challenges that can undermine their experience or lead to misguided decisions. Understanding these common pitfalls and adopting practical strategies is essential to keep your health journey on track.
Sensor Inaccuracies: When Data Doesn’t Tell the Whole Story
At the core of AI fitness tools lies sensor data—wearables track heart rate, sleep patterns, respiration, and movement to inform AI recommendations. However, sensor accuracy remains a significant hurdle. For instance, optical heart rate monitors can struggle due to factors like varying skin tones, motion artifacts, or improper device fit, as noted in industry analyses.
Emerging technologies such as thin-film ultrasound sensors promise higher-resolution and more reliable data but have yet to reach mainstream adoption. Until then, users should:
-
Regularly calibrate and maintain devices, keeping firmware and companion apps updated to leverage bug fixes and algorithm improvements.
-
Ensure proper device placement and fit, since mispositioning can skew sensor readings.
-
Cross-verify AI insights with manual measurements or multiple data sources, especially when making critical health decisions.
Sensor noise and variability can also lead AI models to “overfit,” tailoring workout recommendations too narrowly based on flawed or limited data. Research highlights that AI systems trained recursively on generated data without fresh inputs risk accuracy degradation over time. This underscores the importance of continuously feeding AI with diverse, real-world data instead of relying solely on synthetic or self-generated inputs.
The Human Factor: Why AI Alone Can’t Replace Expertise
AI excels at analyzing data and detecting patterns but cannot fully grasp the nuances of individual motivation, emotional state, or contextual factors such as injury history. Many users report that AI-generated workout plans sometimes feel impersonal or fail to address their unique preferences and needs.
Human expertise remains invaluable in complementing AI:
-
Consult trainers, coaches, or healthcare providers to supplement AI recommendations, particularly for complex goals or health conditions.
-
Use AI as a supportive tool, not an inflexible authority. Question advice that doesn’t feel right or causes discomfort.
-
Stay attuned to your body’s signals; personal awareness and judgment are irreplaceable.
Interestingly, approximately 78% of AI fitness app users report increased motivation, demonstrating AI’s potential when applied thoughtfully. However, motivation can decline if AI feedback feels generic or overwhelming. Customizable interfaces and notification settings help tailor the user experience, mitigating “notification fatigue” and fostering trust.
Privacy and Ethical Concerns: Who’s Watching Your Health Data?
AI fitness tools thrive on rich personal data—location, heart rate variability, sleep patterns, and sometimes sensitive attributes like race or sexual orientation. Without robust safeguards, this data can be exposed to unintended parties.
Privacy experts warn that many fitness apps share user data with third parties such as advertisers, insurers, or government agencies, often without explicit consent. This raises concerns about profiling, targeting, or discrimination.
To safeguard your data:
-
Review and adjust privacy settings regularly, opting out of unnecessary data sharing where possible.
-
Choose apps compliant with recognized standards and certifications, such as GDPR and ISO 9001:2015 for sensor manufacturers.
-
Exercise caution when linking multiple apps or platforms, as this can amplify exposure risk.
Ethical AI use requires transparency about how algorithms function and their limitations. While federal healthcare AI regulations now mandate disclosure of testing and bias mitigation efforts, consumer fitness apps often lag. Users should demand transparency and hold developers accountable for responsible data handling.
When to Trust AI—and When to Take the Wheel
AI fitness tools are sophisticated assistants, not infallible authorities. Data inaccuracies, algorithmic bias, and model drift mean AI recommendations can sometimes mislead rather than help.
Best practices for balanced, critical AI use include:
-
Keep AI tools updated and verify data inputs to maximize accuracy.
-
Combine AI insights with professional advice and your own intuition to ensure well-rounded decisions.
-
Monitor your progress closely and be ready to override or adjust AI plans when they conflict with your lived experience.
-
Stay informed about evolving ethical and privacy standards to protect your rights and data.
Successfully navigating AI’s promises and pitfalls in fitness demands technological savvy, critical thinking, and self-awareness. By troubleshooting data issues, respecting privacy, and integrating human judgment, you can harness AI’s power as a valuable ally—one that augments, rather than replaces, the wisdom of your body and mind.
Challenge | Description | Recommended Actions |
---|---|---|
Sensor Inaccuracies | Wearable sensors can provide flawed data due to skin tone variations, motion artifacts, and improper device fit leading to inaccurate AI recommendations. |
|
The Human Factor | AI lacks the ability to understand personal motivation, emotional state, and contextual health factors, sometimes resulting in impersonal or unsuitable workout plans. |
|
Privacy and Ethical Concerns | Personal health data collected by AI tools may be shared with third parties without consent, raising risks of profiling and discrimination. |
|
Trust and User Control | AI tools can mislead due to data inaccuracies, bias, and model drift; users must balance AI advice with personal judgment. |
|
Advanced Techniques and Future Directions: Optimizing and Expanding Your AI Fitness Ecosystem
Advanced Techniques and Future Directions: Optimizing and Expanding Your AI Fitness Ecosystem
What happens when AI moves beyond simple step counters or generic workout plans? The frontier of AI-powered fitness is rapidly evolving into a sophisticated, multi-dimensional ecosystem that tailors every aspect of your health and wellness journey—from your unique genetic makeup to your mental state. Let’s explore how cutting-edge technologies like genetic integration, immersive AR/VR workouts, and multi-modal AI platforms are not only optimizing fitness regimens but also redefining what it means to be healthy.
Integrating Genetic Data and AR/VR: The New Frontier of Personalized Fitness
Imagine a workout program that doesn’t rely on broad factors like age or weight but instead designs every exercise, meal, and recovery plan based on your unique genetic blueprint. Advances in AI-driven genetic analysis are making such personalization a reality. Fitness platforms now harness DNA insights to reveal muscle fiber composition, injury susceptibility, metabolism, and stress responses.
For example, an AI app might recommend specific strength training exercises if your genes favor fast-twitch muscle fibers or suggest low-impact alternatives if you have a higher genetic risk of joint injuries. This level of customization goes far beyond traditional fitness tracking, offering precision-tailored guidance.
But personalization doesn’t stop at genetics. Augmented reality (AR) and virtual reality (VR) technologies are transforming exercise into immersive experiences that boost motivation and technique. Instead of enduring a monotonous treadmill session, you could be running along a virtual mountain trail or cycling through a digitally rendered countryside, with AI coaches providing real-time feedback on your form.
These immersive workouts help overcome common barriers like gym anxiety and boredom, enabling users to exercise comfortably at home and at their own pace. Leading smart fitness devices such as the Amp home gym and Gym Monster 2, showcased at CES 2025, integrate AI-powered form monitoring and tailored coaching to replicate personal trainer guidance.
The future promises even richer sensory environments. Devices like Peloton and Tonal are actively integrating VR and AR, with potential developments including simulations of temperature, wind resistance, or even scents, which could make home workouts more engaging and physiologically effective.
However, the effectiveness of these AI models depends heavily on data quality and inclusivity. Many current fitness AI systems are trained primarily on datasets from young, healthy adults, which may limit their accuracy and safety for older populations or individuals with disabilities. Improving data diversity is essential to avoid perpetuating health disparities and to extend AI benefits equitably.
Multi-Modal AI Platforms: Uniting Exercise, Nutrition, and Mental Wellness
Fitness today is no longer a siloed activity. The future—and increasingly the present—is about holistic health ecosystems where exercise, nutrition, sleep, and mental wellness are seamlessly integrated. Advanced AI-powered platforms unify these dimensions, delivering hyper-personalized coaching that adapts in real time to your physical activity, dietary intake, sleep quality, and stress levels.
For instance, AI nutrition apps now go beyond simple calorie counting. They incorporate health conditions like cardiovascular risk or diabetes to generate meal plans that optimize nutrient intake aligned with your fitness goals and genetic predispositions. When combined with continuous wearable data, these platforms can dynamically adjust recommendations—for example, increasing protein intake after intense strength training or suggesting mindfulness exercises during stressful periods.
This 360-degree approach fosters sustainable habits by addressing not only workout performance but also recovery, mental resilience, and long-term wellness.
Advanced users and fitness professionals can further enhance personalization by customizing AI models or integrating multiple AI tools. Smart clothing embedded with electrochemical sensors, for example, can non-invasively monitor hydration levels or muscle fatigue in real time, feeding data back into AI algorithms for refined adjustments.
Technically skilled developers and enthusiasts can fine-tune AI parameters or leverage open APIs to orchestrate synergistic workflows across platforms, maximizing benefits and user engagement.
Critical Evaluation and Ethical Use of Emerging AI Fitness Technologies
With rapid AI innovation comes the responsibility to critically assess fitness technologies—not only for their effectiveness but also for fairness, privacy, and inclusivity.
Algorithmic bias remains a significant concern. AI systems trained on non-representative data risk reinforcing disparities by delivering suboptimal or even harmful recommendations to marginalized groups. Transparency about data sources and decision-making processes is vital to build trust and accountability.
Frameworks like the FUTURE-AI guidelines emphasize principles such as fairness, explainability, and robustness, providing a valuable roadmap for deploying trustworthy AI in health and fitness. Users and developers should advocate for these standards to ensure AI tools respect privacy, deliver understandable insights, and perform reliably across diverse populations.
Ethical considerations also encompass data security and informed consent. Fitness apps often collect sensitive biometric and genetic information, increasing the stakes for protecting personal health data. Responsible AI architectures must prioritize secure data handling and empower users with control over their information.
On a societal level, AI-powered fitness holds great promise for democratizing access to personalized health guidance. By reducing dependence on costly personal trainers or specialized clinics, AI can bring tailored fitness support to underserved communities and individuals with mobility challenges.
However, caution is warranted. Emerging technologies such as neural implants or advanced AR glasses, while promising, risk widening inequalities if access remains limited to affluent users. Advocating for equitable distribution and affordability will be crucial to ensuring these innovations benefit a broad population.
Looking Ahead: The Evolving Role of AI in Democratizing Fitness
The trajectory of AI in fitness points toward a future where health optimization is highly personalized, immersive, and interconnected. We are advancing toward ecosystems that not only track your movements but also understand your biology, environment, and mental state—offering holistic guidance that evolves with you.
This evolution demands ongoing vigilance to balance technological possibilities with ethical imperatives. Transparency, inclusivity, and user empowerment must serve as foundational pillars as AI becomes deeper embedded in our health routines.
For individuals eager to harness these advances, now is the time to experiment thoughtfully—combining genetic insights, VR/AR experiences, and integrated wellness platforms while maintaining a critical eye toward data privacy and algorithmic fairness.
Ultimately, the future of AI fitness is not about replacing human intuition or expertise but amplifying it—making personalized, effective, and equitable fitness accessible to all. The question isn’t if AI will reshape our health journeys, but how we will ensure it does so responsibly and inclusively.
Advanced Techniques | Description | Examples / Technologies | Benefits | Challenges / Considerations |
---|---|---|---|---|
Integrating Genetic Data | Personalizing workouts, meals, and recovery based on unique genetic makeup | AI-driven genetic analysis revealing muscle fiber composition, injury risk, metabolism | Precision-tailored guidance beyond traditional fitness tracking | Requires high-quality, diverse genetic data to avoid bias |
AR/VR Immersive Workouts | Using augmented and virtual reality to create engaging exercise environments | Virtual mountain trails, AI coaching with real-time feedback, devices like Amp home gym, Gym Monster 2, Peloton, Tonal | Improves motivation, technique, and reduces gym anxiety and boredom | Data inclusivity and safety; future sensory integrations (temperature, wind, scents) |
Multi-Modal AI Platforms | Integrating exercise, nutrition, sleep, and mental wellness into one AI ecosystem | AI nutrition apps, wearable sensors, smart clothing with electrochemical sensors | Hyper-personalized coaching adapting to real-time physical and mental states | Complexity of data integration and model customization |
Ethical Use & Critical Evaluation | Ensuring fairness, privacy, transparency, and inclusivity in AI fitness technologies | FUTURE-AI guidelines, data security frameworks, transparency practices | Builds trust, protects sensitive biometric data, promotes equitable access | Risks of algorithmic bias, data misuse, unequal technology access |
Future Directions | Ongoing development toward holistic, immersive, and interconnected AI fitness ecosystems | Genetic insights, VR/AR experiences, integrated wellness platforms | Amplifies human expertise; makes personalized fitness accessible to all | Balancing innovation with ethical imperatives and inclusivity |