Top 5 AutoML Platforms Reviewed: Real Pros, Cons & Use Cases
- Introduction: Why Comparing AutoML Platforms Matters and Our Selection Criteria
- Introduction: Why Comparing AutoML Platforms Matters and Our Selection Criteria
- Defining the Scope: What AutoML Platforms We Cover
- Our Selection Criteria: What Matters Most
- Setting Reader Expectations
- Conclusion: Key Takeaways and Contextual Recommendations for Choosing an AutoML Platform
- Conclusion: Key Takeaways and Contextual Recommendations for Choosing an AutoML Platform
- Standout Strengths and Weaknesses
- Practical Guidance for Selecting the Right Platform
- The Importance of Trialing Platforms
- Future Trends Shaping AutoML Evolution
- Final Thoughts
- 1. Google Vertex AI AutoML: Technical Specifications and Real-World Performance
- Google Vertex AI AutoML: Technical Specifications and Real-World Performance
- Supported Problem Types and Training Workflow
- Deployment Options and Integration with Google Cloud
- Performance Metrics: Accuracy, Latency, and Scalability
- Practical Use Cases and Real-World Limitations
- Pricing and Cost Considerations
- Summary
- 2. Microsoft Azure Automated ML: Unique Features and Practical Use Cases
- 2. Microsoft Azure Automated ML: Unique Features and Practical Use Cases
- Model Interpretability and Automated Feature Engineering
- AutoML Pipeline Architecture and Hyperparameter Optimization
- Deployment Flexibility and Enterprise-Grade Security
- Comparative Performance Insights
- Real-World Use Cases
- Limitations to Consider
- 3. DataRobot Enterprise AI: Innovation, Usability, and Performance Metrics
- DataRobot Enterprise AI: Innovation, Usability, and Performance Metrics
- Advanced Automation Features: Multi-Model Ensembling and Data Preprocessing
- Technical Architecture: Rapid Iteration and Scalable Monitoring
- Quantitative Performance and Use Case Insights
- User Experience, Collaboration, and Compliance
- Summary
- 4. H2O.ai Driverless AI: Technical Depth and Industry Applications
- H2O.ai Driverless AI: Technical Depth and Industry Applications
- Proprietary Feature Engineering and GPU Acceleration
- Supported Algorithms and Performance Benchmarks
- Scalability with Large Datasets
- Model Explainability Modules
- Integration with Cloud Providers and Open-Source Tools
- Practical Industry Applications
- Pros and Cons
- Final Take
- 5. Amazon SageMaker Autopilot: Specifications, User Experience, and Use Case Suitability
- Amazon SageMaker Autopilot: Specifications, User Experience, and Use Case Suitability
- Technical Specifications and Automated Model Building
- User Experience: Ease of Use, Customization, and Cost Efficiency
- Use Case Suitability and Real-World Deployment Examples
- Competitive Advantages and Drawbacks
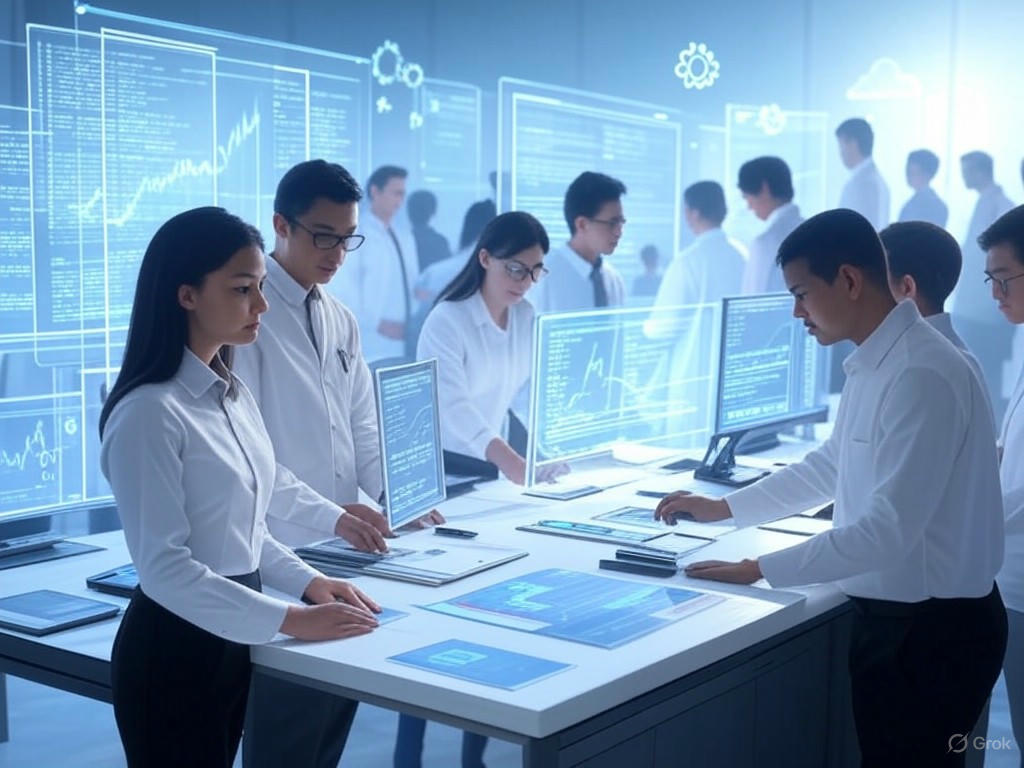
Introduction: Why Comparing AutoML Platforms Matters and Our Selection Criteria

Introduction: Why Comparing AutoML Platforms Matters and Our Selection Criteria
Automated Machine Learning (AutoML) has evolved from a niche convenience into a strategic imperative in 2025. Beyond merely accelerating model development, AutoML fundamentally transforms how enterprises and data scientists approach predictive analytics. Studies indicate that organizations adopting AutoML reduce model development time by 60 to 80 percent, a significant efficiency gain that allows data teams to focus on higher-value activities such as feature engineering and extracting business insights, rather than on repetitive coding and hyperparameter tuning.
Moreover, AutoML democratizes access to advanced machine learning capabilities. Modern platforms enable business analysts and non-experts to develop predictive models without deep programming expertise. This democratization is critical as predictive insights become embedded across industries—from finance and healthcare to retail—powering core business processes. However, AutoML is not a silver bullet. Challenges persist, particularly in domain-specific feature engineering and navigating regulatory compliance, especially under frameworks like the EU’s AI Act. This legislation is driving next-generation AutoML platforms to integrate compliance and explainability directly into their workflows.
Defining the Scope: What AutoML Platforms We Cover
This article focuses on end-to-end AutoML platforms that automate not only model training but the entire machine learning lifecycle. Key stages covered include data preparation, feature engineering, model selection, hyperparameter tuning, deployment, and ongoing model monitoring. Scalability and ease of deployment are paramount, particularly for enterprises aiming to operationalize models at scale without bottlenecks.
We highlight platforms with strong model monitoring and management features—often overlooked yet essential for maintaining production model performance. Solutions such as Google Cloud Vertex AI AutoML, Microsoft Azure AutoML, DataRobot, and Databricks AutoML exemplify this category, offering integrated ecosystems that strike a balance between automation and flexibility.
While open-source alternatives like H2O AutoML and Ludwig exist, our comparison prioritizes platforms that offer seamless integration with enterprise workflows and cloud infrastructure. Deployment and ongoing management capabilities frequently determine real-world success more than raw automation features.
Our Selection Criteria: What Matters Most
Selecting an AutoML platform is a complex decision involving multiple factors. We evaluated contenders across six critical dimensions:
-
Technical Capabilities: Coverage of algorithms, depth of automation (including data preparation, feature engineering, and tuning), support for diverse data types such as tabular, time series, and text, and the ability to handle complex modeling scenarios.
-
Usability: Intuitiveness of the user interface, accessibility for non-ML experts, availability of visual tools versus code-centric options, and the comprehensiveness of documentation.
-
Integration Options: Compatibility with common data sources, cloud platforms, MLOps tools, and deployment environments such as Kubernetes or serverless architectures.
-
Performance Benchmarks: Evaluation based on accuracy, training time, and resource utilization on standardized datasets and real-world case studies.
-
Pricing Transparency: Clarity and predictability of pricing models that scale with usage, with particular attention to total cost of ownership for enterprises.
-
Real-World User Feedback: Insights aggregated from platforms like G2 and Gartner Peer Insights, as well as verified case studies, highlighting strengths and common challenges reported by actual users.
This comprehensive approach ensures our comparison transcends marketing claims, offering practical insights. For instance, while some platforms emphasize extensive automation, user reviews often reveal difficulties in customizing models for domain-specific needs or integrating with legacy systems.
Setting Reader Expectations
This article is designed as a critical, evidence-based evaluation—not a vendor showcase. Our aim is to assist data teams and decision-makers in selecting the AutoML platform that best fits their unique context. We spotlight genuine innovations, such as multi-agent AI workflows and integrated compliance features, while candidly addressing limitations like gaps in unsupervised learning support or the steep learning curves imposed by some platforms.
You can expect comparative metrics and real-world use cases illustrating platform performance under typical enterprise workloads. Our goal is to equip you with the knowledge to weigh trade-offs thoughtfully, enabling accelerated machine learning initiatives without compromising scalability, control, or regulatory compliance.
In the following sections, we provide in-depth comparisons of the top five AutoML platforms, detailing their pros, cons, and best-fit use cases with the technical rigor and practical insights necessary for informed decision-making.
Selection Criteria | Description |
---|---|
Technical Capabilities | Coverage of algorithms, automation depth (data prep, feature engineering, tuning), support for diverse data types, handling complex modeling scenarios |
Usability | User interface intuitiveness, accessibility for non-experts, visual vs code-centric tools, documentation quality |
Integration Options | Compatibility with data sources, cloud platforms, MLOps tools, deployment environments like Kubernetes/serverless |
Performance Benchmarks | Accuracy, training time, resource utilization on standardized datasets and case studies |
Pricing Transparency | Clarity and predictability of pricing models, total cost of ownership considerations |
Real-World User Feedback | Insights from G2, Gartner Peer Insights, verified case studies highlighting strengths and challenges |
Conclusion: Key Takeaways and Contextual Recommendations for Choosing an AutoML Platform
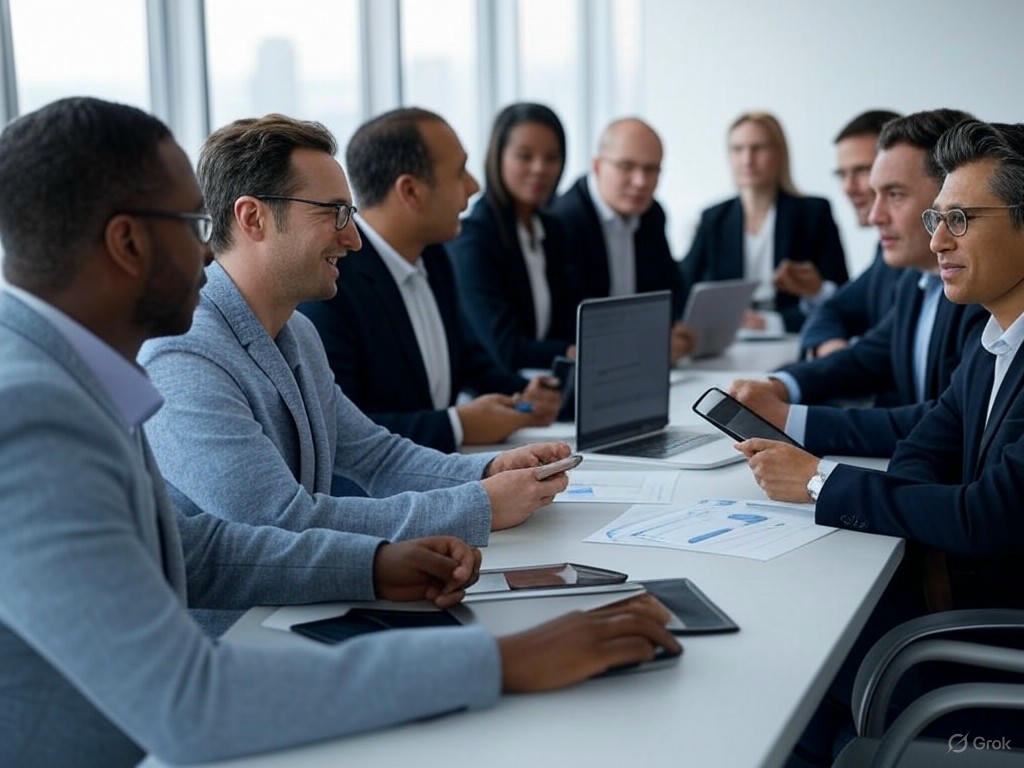
Conclusion: Key Takeaways and Contextual Recommendations for Choosing an AutoML Platform
After a comprehensive evaluation of the leading AutoML platforms from both technical and practical perspectives, distinct patterns emerge to help organizations navigate their selection process. Each platform offers unique strengths tailored to different organizational needs, data complexities, and operational environments, but no single solution fits every scenario perfectly.
Standout Strengths and Weaknesses
-
Google Vertex AI excels through its seamless integration with the Google Cloud ecosystem and sophisticated model evaluation tools. It handles large, complex datasets with advanced hyperparameter tuning and autoscaling infrastructure, making it well-suited for organizations with robust cloud capabilities and experienced ML teams. However, its complexity and pricing model can present barriers for smaller teams or newcomers to AutoML.
-
Amazon SageMaker Autopilot provides a balanced AutoML experience that combines automated model development with transparency and control over the modeling process. It is particularly advantageous for enterprises deeply embedded in the AWS ecosystem, benefiting from tight integration with services like S3, IAM, and Lambda. On the downside, it has a steeper learning curve, limited customization options compared to some competitors, and operational costs that can escalate with scale.
-
DataRobot stands out for democratizing AI with an intuitive interface and enterprise-grade automation. Its advanced multi-model ensembling via Feature Importance Rank Ensembling (FIRE), automated data preprocessing, and integrated MLOps capabilities deliver a powerful platform for scalable AI development. While it supports collaboration and governance well, pricing and resource demands restrict its accessibility primarily to mid-to-large organizations.
-
Microsoft Azure Machine Learning offers a technically sophisticated solution emphasizing model interpretability and versatility. With embedded explainability tools like SHAP and Integrated Gradients, and extensive support for diverse data types and hyperparameter tuning methods, Azure ML caters to enterprises requiring rigorous model governance. Its seamless Azure integration enables scalable deployments but may overwhelm newcomers due to its breadth and requires investment in Azure-specific expertise.
-
Akkio targets businesses seeking rapid AI integration with minimal technical overhead. Its user-friendly design and quick automation capabilities make it accessible for non-experts aiming to deploy AI-driven decision-making without heavy coding. However, Akkio lacks the depth and flexibility of enterprise platforms and is less suited for very large or complex datasets.
Practical Guidance for Selecting the Right Platform
Choosing an AutoML platform requires a nuanced assessment beyond feature checklists or vendor marketing. Key considerations include:
-
Organizational Size and Expertise: Smaller teams or organizations without dedicated data science resources benefit from platforms like Akkio or DataRobot, which simplify workflows and minimize manual coding. Larger enterprises with established cloud infrastructure and specialized personnel might prefer Google Vertex AI or Azure ML for their scalability, customization, and compliance capabilities.
-
Data Complexity and Volume: For organizations dealing with high-dimensional, large-scale datasets or specialized use cases such as computer vision or natural language processing, platforms with advanced technical capabilities and pipeline control (e.g., Vertex AI, SageMaker) are preferable.
-
Budget Constraints: Pricing varies widely across platforms. Enterprise-grade solutions like DataRobot, SageMaker, and Vertex AI often entail several hundred to thousands of dollars per user per month, justified by advanced features and comprehensive support. More affordable, streamlined options such as Akkio provide faster ROI but may lack depth and flexibility.
-
Desired Level of Automation: Consider whether a fully automated workflow or granular control over stages like feature engineering and model tuning is preferable. For example, SageMaker Autopilot offers transparency and manual override options, whereas others emphasize end-to-end automation.
The Importance of Trialing Platforms
Regardless of a platform’s feature list or market reputation, hands-on testing with your own data and workflows is essential. Most leading AutoML providers offer trial periods or sandbox environments. Use these opportunities to:
-
Validate model performance metrics specific to your datasets and business goals.
-
Assess usability, integration with existing tools, and ease of deployment.
-
Test scalability, monitoring features, and operational workflows.
-
Evaluate vendor support responsiveness, documentation quality, and community resources.
This practical evaluation helps prevent costly misalignments and ensures the selected platform accelerates your AI initiatives effectively.
Future Trends Shaping AutoML Evolution
AutoML technology is rapidly evolving, driven by emerging trends that will influence platform capabilities and enterprise adoption:
-
Automated Feature Engineering and Explainability: Platforms increasingly embed explainability tools (e.g., SHAP, LIME) to enhance transparency, build trust, and support regulatory compliance, especially under frameworks like the EU AI Act.
-
Integration of Smaller Language Models (SLMs) and Few-Shot Learning: These approaches reduce computational overhead while maintaining performance, benefiting scalable and resource-efficient AutoML workflows.
-
Edge AI and Federated Learning Support: As IoT and edge devices proliferate, AutoML platforms that enable decentralized training and inference will gain competitive advantages by reducing latency and improving data privacy.
-
AI Agents and Autonomous Workflow Orchestration: The rise of intelligent agents promises further automation beyond model building, impacting business process optimization and accelerating AI adoption across functions.
Leading platforms such as Google Vertex AI and Microsoft Azure ML are proactively incorporating these trends, investing in scalable infrastructure, AI lifecycle management, and enhanced interpretability. Emerging players focus on user experience and vertical-specific solutions, reflecting the market’s diverse demands.
Final Thoughts
Selecting an AutoML platform is a strategic decision balancing technical sophistication, usability, cost, and alignment with organizational context. The platforms compared here represent the leading options available in 2025, each excelling in different dimensions.
Begin with a clear assessment of your team’s expertise, data requirements, budget, and automation preferences. Leverage trial environments extensively to validate fit and performance. Stay informed about evolving AutoML technologies and regulatory landscapes to future-proof your machine learning investments.
By adopting a thoughtful, evidence-based approach, your organization can harness AutoML to accelerate predictive analytics, reduce time-to-insight, and unlock new opportunities in an increasingly AI-driven business environment.
AutoML Platform | Standout Strengths | Weaknesses | Best Use Cases |
---|---|---|---|
Google Vertex AI | Seamless Google Cloud integration; advanced hyperparameter tuning; autoscaling; handles large, complex datasets | Complexity; pricing barriers for smaller teams; requires experienced ML teams | Organizations with robust cloud capabilities and experienced ML teams; large-scale, complex data projects |
Amazon SageMaker Autopilot | Balanced AutoML with transparency and control; integration with AWS services like S3, IAM, Lambda | Steeper learning curve; limited customization vs competitors; operational costs can escalate | Enterprises embedded in AWS ecosystem needing model transparency and control |
DataRobot | Intuitive interface; enterprise-grade automation; advanced multi-model ensembling (FIRE); integrated MLOps; strong collaboration and governance | High pricing; resource intensive; mostly accessible to mid-to-large organizations | Mid-to-large organizations seeking scalable AI development with governance and collaboration |
Microsoft Azure Machine Learning | Model interpretability (SHAP, Integrated Gradients); supports diverse data types; extensive hyperparameter tuning; scalable Azure integration | Broad and complex; may overwhelm newcomers; requires Azure-specific expertise | Enterprises needing rigorous model governance and scalable deployments within Azure ecosystem |
Akkio | User-friendly; rapid AI integration; minimal technical overhead; quick automation | Lacks depth and flexibility of enterprise platforms; less suited for very large or complex datasets | Businesses seeking fast AI deployment without heavy coding; smaller teams or non-experts |
1. Google Vertex AI AutoML: Technical Specifications and Real-World Performance
Google Vertex AI AutoML: Technical Specifications and Real-World Performance
Google’s Vertex AI AutoML is a mature, cloud-native platform designed for organizations seeking to leverage machine learning without deep expertise in coding or algorithm development. Drawing on over eight years of experience reviewing cloud AI products, Vertex AI stands out for its seamless integration within the broader Google Cloud ecosystem and its evolving support for generative AI capabilities. Below, we provide a detailed technical and practical evaluation of its offerings.
Supported Problem Types and Training Workflow
Vertex AI AutoML currently focuses on three primary text-based problem domains:
- Classification: Categorizing text into predefined labels.
- Entity Extraction: Identifying and labeling entities such as names, dates, or locations within text.
- Sentiment Analysis: Detecting sentiment polarity and intensity in textual data.
This specialization is evolving alongside Google’s September 15, 2024 launch of the Vertex Gemini prompting and tuning framework. The Gemini system enables more granular model customization through advanced prompt engineering, supporting hybrid reasoning and multimodal inputs (text, images, audio). Users building or refining models in these areas will increasingly rely on Gemini prompts for enhanced control.
Vertex AI enforces a best-practice supervised learning workflow with a standardized dataset split:
- Training Set: Used to fit model parameters.
- Validation Set: Used for hyperparameter tuning and to prevent overfitting.
- Test Set: A holdout dataset—typically 10% of total data—reserved for final unbiased evaluation.
This three-way split ensures reliable estimates of generalization performance prior to deployment.
Beyond text, Vertex AutoML also offers action recognition capabilities for video data, classifying shots and segments. However, this modality is less mature and less widely adopted compared to text-based features.
Deployment Options and Integration with Google Cloud
Models trained with Vertex AI AutoML can be deployed directly to scalable endpoints within the Vertex AI environment. Both AutoML-generated and custom-trained models are accessible via REST APIs, facilitating seamless integration into production pipelines or applications.
Key deployment features include:
- Autoscaling Infrastructure: Automatically adjusts replica counts every 15 seconds based on recent traffic patterns, ensuring responsiveness and cost efficiency.
- Google Cloud Storage Integration: Simplifies dataset management and versioning.
- Google Kubernetes Engine (GKE) Support: Enables scalable inference workloads for demanding production environments.
- Unified Security and Billing: Leverages Google Cloud’s authentication, authorization, and billing frameworks for consistent policy enforcement.
Dataset preparation tools within Vertex AI streamline data ingestion, supporting diverse formats—images for vision tasks and structured formats for tabular and text data. Managed datasets reduce manual overhead in data versioning, preprocessing, and quality control.
Performance Metrics: Accuracy, Latency, and Scalability
Public benchmarks and user reports indicate that Vertex AI AutoML models deliver competitive accuracy in supported tasks. Notably, the Google Cloud Console provides a visual “score threshold” slider that allows users to balance precision and recall dynamically. Lowering the threshold increases classification coverage but may elevate misclassification risk.
Latency for online predictions depends on model complexity and deployment configuration. Advances in Google infrastructure, such as Ironwood TPUs and GKE inferencing improvements, have demonstrated serving cost reductions of up to 30% and tail latency reductions approaching 60%. In real-world scenarios, users report sub-second latency for typical text classification workloads with autoscaling endpoints.
The platform scales robustly with workload fluctuations. However, some users note increased latency during periods of low query-per-second (QPS), a common trade-off in autoscaling cloud systems.
Practical Use Cases and Real-World Limitations
Vertex AI AutoML excels in enterprise contexts requiring rapid deployment of classification and extraction models with minimal ML engineering overhead. For example, customer support teams leverage it to automatically categorize support tickets by topic and sentiment, enabling faster routing and prioritization.
Nonetheless, limitations exist:
- Category Recognition Challenges: While Vertex AI can handle an extensive number of categories, models may struggle with rare or poorly represented classes—a limitation inherent to supervised learning systems and emphasized in Google’s documentation.
- Transition to Vertex Gemini Prompts: The shift toward Gemini prompting introduces a learning curve. Although Gemini’s hybrid reasoning and multimodal capabilities represent significant innovation, teams must adapt workflows to fully exploit prompt tuning and customization.
Pricing and Cost Considerations
Vertex AI AutoML pricing aligns with legacy Google Cloud AI Platform rates and follows a pay-as-you-go model, covering:
- Training compute time.
- Model deployment resources.
- Prediction request consumption.
Generative AI features, including Gemini models, incur charges based on input/output tokens or processing time for images and video. Google offers new users $300 in cloud credits, aiding initial experimentation.
Enterprises should carefully model anticipated usage to manage costs effectively, especially when deploying large-scale or multimodal AI models.
Summary
Google Vertex AI AutoML delivers a technically robust, scalable solution for building and deploying classification, entity extraction, and sentiment analysis models. Its strengths include deep integration with the Google Cloud ecosystem, automated dataset management, and flexible deployment via REST APIs.
Performance metrics generally meet or exceed industry standards, supported by Google’s infrastructure investments reducing latency and cost. The ongoing transition to Vertex Gemini prompts marks a strategic evolution toward more customizable AI workflows but demands workflow adaptation.
Limitations such as challenges with rare category recognition and pricing complexity suggest Vertex AI AutoML is best suited for organizations with moderate to large datasets and a readiness to engage with evolving Google AI tooling. For these users, it remains a leading choice in the AutoML landscape.
Category | Details |
---|---|
Supported Problem Types | Classification, Entity Extraction, Sentiment Analysis, Action Recognition (video) |
Training Workflow | Supervised learning with Training, Validation, and Test set split (Test set ~10%) |
New Features | Vertex Gemini prompting and tuning framework for hybrid reasoning and multimodal inputs |
Deployment Options | Scalable endpoints in Vertex AI, REST APIs, Autoscaling every 15 seconds, GKE support |
Integration | Google Cloud Storage, Google Kubernetes Engine, Unified security and billing |
Performance | Competitive accuracy, sub-second latency for text tasks, up to 30% cost reduction, 60% latency reduction (infrastructure improvements) |
Scalability | Robust autoscaling with noted latency increase at very low QPS |
Use Cases | Enterprise classification, entity extraction, sentiment analysis (e.g., customer support ticket routing) |
Limitations | Challenges with rare categories, learning curve for Vertex Gemini prompt tuning |
Pricing | Pay-as-you-go for training, deployment, predictions; generative AI charged by tokens/time; $300 free credits for new users |
2. Microsoft Azure Automated ML: Unique Features and Practical Use Cases

2. Microsoft Azure Automated ML: Unique Features and Practical Use Cases
Microsoft Azure Automated ML distinguishes itself in the AutoML market by offering a comprehensive, enterprise-grade solution that emphasizes model interpretability, broad data type support, and deep integration within the Azure ecosystem. While its power and flexibility cater well to complex organizational needs, these advantages come with a notable learning curve and cost implications that decision-makers should carefully consider.
Model Interpretability and Automated Feature Engineering
A defining strength of Azure Automated ML is its rich suite of model interpretability tools. Unlike many AutoML platforms that treat models as opaque black boxes, Azure incorporates advanced explanation techniques such as SHAP (Shapley Additive exPlanations), Integrated Gradients, and XRAI. These methods enable data scientists and business stakeholders to understand precisely why models make specific predictions and identify avenues for improvement.
For instance, SHAP’s hierarchical token-based explanations provide granular insights into feature importance within text models. This level of transparency is especially critical in regulated industries like finance and healthcare, where auditability and compliance hinge on clear model reasoning.
On the feature engineering front, Azure’s AutoML pipeline automates complex transformations across a wide range of data types. It natively supports tabular data, time series forecasting, and even deep neural networks for more sophisticated modeling tasks. The platform’s SDK allows fine-grained customization of preprocessing steps such as scaling, normalization, and encoding, which is essential for handling heterogeneous real-world datasets effectively.
AutoML Pipeline Architecture and Hyperparameter Optimization
From a technical perspective, Azure Automated ML orchestrates multiple parallel pipelines that explore diverse algorithms and hyperparameter configurations simultaneously. It leverages Bayesian optimization, grid search, and bandit policies to efficiently navigate the hyperparameter space, striking a balance between thorough exploration and early stopping of underperforming trials.
Ensembling is enabled by default, combining base learners using meta-models like Logistic Regression for classification and ElasticNet for regression. This approach consistently enhances predictive accuracy by aggregating strengths across models.
The platform’s Responsible AI dashboard adds another layer of robustness by offering comprehensive evaluation metrics, including F1 score, balanced accuracy, calibration curves, lift charts, and fairness and error analysis tools. These capabilities facilitate not only model performance monitoring but also ethical and regulatory compliance.
Deployment Flexibility and Enterprise-Grade Security
Azure Automated ML integrates seamlessly with the broader Azure ecosystem, supporting deployment across Azure Container Instances, Azure Kubernetes Service (AKS), and Azure Functions. This flexibility accommodates various production scenarios, from batch processing to real-time inference pipelines.
Security is a core consideration in Azure’s design. Enterprise-grade features such as Role-Based Access Control (RBAC), managed identities, and secure data storage options (e.g., Azure Data Lake Storage and Cosmos DB) ensure sensitive data and models remain protected. Monitoring dashboards provide visibility into pipeline health and resource consumption, supporting operational stability in production.
Comparative Performance Insights
Independent evaluations position Azure Automated ML as a highly competitive platform in terms of accuracy and scalability, particularly for large-scale enterprise workloads involving tabular and time series data. Its parallel pipeline execution and default ensembling consistently yield near state-of-the-art performance benchmarks.
However, some competitors, notably AWS SageMaker Autopilot, offer faster iteration cycles and a more modular, developer-friendly experience, appealing to teams prioritizing agility and flexibility. Azure’s comprehensive feature set and integration depth can introduce complexity that may slow onboarding, especially for users less familiar with Azure services or ML operations.
Real-World Use Cases
-
Finance: Azure Automated ML is widely adopted for credit risk modeling and fraud detection, where transparency and regulatory compliance are paramount. The platform’s detailed model explanations support audit and compliance teams in validating predictions and mitigating bias.
-
Healthcare: Healthcare providers utilize Azure Automated ML to predict patient outcomes and optimize resource allocation. Its native support for time series data and stringent security features align well with healthcare’s privacy and compliance demands.
-
Retail: Retailers leverage Azure’s automated feature engineering and scalable deployment options for demand forecasting and customer segmentation. This enables rapid responsiveness to market fluctuations and inventory management challenges.
Limitations to Consider
Despite its capabilities, Azure Automated ML can be daunting for beginners. Mastery requires familiarity with Azure’s ecosystem and ML workflows, often necessitating dedicated training and skilled personnel.
Cost is another important factor. Azure’s pay-as-you-go pricing, combined with parallel pipeline execution and compute-intensive hyperparameter tuning, can lead to rapid cost accumulation. Careful budget management and usage monitoring are essential to avoid unexpected expenses.
In summary, Microsoft Azure Automated ML offers a technically sophisticated, enterprise-ready AutoML platform excelling in model interpretability, support for diverse data types, and secure, flexible deployment within Azure’s cloud ecosystem. It is particularly well-suited for large organizations embedded in Azure infrastructure that require rigorous model governance and compliance. However, teams new to AutoML or those seeking more cost-effective, lightweight solutions might find Azure’s complexity and pricing structure challenging.
Category | Details |
---|---|
Model Interpretability | SHAP, Integrated Gradients, XRAI for explanation; granular feature importance insights; critical for regulated industries |
Automated Feature Engineering | Supports tabular, time series, deep neural networks; customizable preprocessing like scaling, normalization, encoding |
Pipeline Architecture | Parallel pipelines exploring algorithms and hyperparameters; uses Bayesian optimization, grid search, bandit policies; default ensembling with Logistic Regression and ElasticNet |
Responsible AI Dashboard | Evaluation metrics: F1 score, balanced accuracy, calibration curves, lift charts; fairness and error analysis tools |
Deployment Options | Azure Container Instances, Azure Kubernetes Service, Azure Functions; supports batch and real-time inference |
Security Features | Role-Based Access Control (RBAC), managed identities, secure storage (Azure Data Lake, Cosmos DB), monitoring dashboards |
Performance Insights | High accuracy and scalability; excels in tabular and time series data; slower iteration versus some competitors like AWS SageMaker Autopilot |
Use Cases |
|
Limitations | Steep learning curve; requires Azure ecosystem knowledge; potentially high costs due to compute-intensive tuning and parallel pipelines |
3. DataRobot Enterprise AI: Innovation, Usability, and Performance Metrics
DataRobot Enterprise AI: Innovation, Usability, and Performance Metrics
DataRobot’s AutoML platform distinguishes itself through sophisticated automation capabilities that extend well beyond basic model building. Features like multi-model ensembling, automatic data preprocessing, and integrated MLOps functionalities combine to create a comprehensive environment tailored for rapid and scalable AI development. But how do these innovations perform in practice, and what does the user experience look like? Below, we unpack the platform’s technical strengths alongside practical considerations.
Advanced Automation Features: Multi-Model Ensembling and Data Preprocessing
At the core of DataRobot’s automation is an advanced feature selection technique known as Feature Importance Rank Ensembling (FIRE). This method aggregates feature impact scores across multiple models to minimize redundancy and improve predictive accuracy. By iteratively constructing a feature selection curve, FIRE balances model simplicity with performance—often outperforming approaches relying on single-model feature importance.
Key aspects include:
- Median rank aggregation of feature importance across several top-performing blueprints, excluding blends and frozen models, to optimize feature subsets.
- Broad applicability across project types such as binary classification, regression, multiclass classification, and time series forecasting.
- DataRobot’s autopilot mode generates diverse model candidates, enabling intelligent ensembling and feature list refinement with up to three “lives” to prevent accuracy loss.
Beyond feature selection, DataRobot automates data cleaning and preprocessing within its NextGen Workbench interface. It efficiently addresses common data quality issues—including missing values, outliers, and inconsistent formats—through automated scans complemented by visual insights. This significantly reduces the time data scientists spend on manual data wrangling.
Additional capabilities include:
- Support for direct ingestion of multiple file formats such as CSV, Excel, and SAS.
- Interactive data wrangling recipes that allow users to prepare data in place, preserving data integrity by avoiding unnecessary data movement.
- Feature discovery across multiple datasets, facilitating interaction-based feature creation.
Technical Architecture: Rapid Iteration and Scalable Monitoring
DataRobot’s underlying architecture supports accelerated model iteration and deployment through flexible deployment options, including cloud-based and self-managed environments. The platform’s Workbench interface streamlines workflows by enabling teams to organize assets, run experiments, and tune hyperparameters with advanced tools like Smart Search optimization and manual override.
Notable technical features include:
- A Training Dashboard offering granular insights into metrics such as model loss, accuracy, learning rate, and momentum over training iterations, empowering informed hyperparameter tuning.
- Advanced tuning capabilities that provide extensive control over preprocessing steps—a rare feature among automated platforms.
DataRobot’s MLOps integration is a significant differentiator. Its unified AI production tools consolidate model deployment, monitoring, and governance within a single platform. The DataRobot AI Production extension notably expands monitoring to generative AI and large language models (LLMs), tracking data drift, performance degradation, and overall service health in real time.
Highlights of the MLOps ecosystem:
- A Model Registry enabling connections to popular LLMs such as GPT-3.5 and LaMDA.
- A monitoring agent, typically deployed outside the core platform, feeds performance metrics back into DataRobot for centralized oversight.
- Support for external model deployment and monitoring, accommodating hybrid infrastructure requirements.
Quantitative Performance and Use Case Insights
DataRobot delivers measurable improvements in accuracy and efficiency across diverse industries. For instance, financial institutions employing DataRobot’s fraud detection models report a 25–40% reduction in false positives, which directly translates into reduced operational costs. In retail, DataRobot’s multiseries time series forecasting enables precise sales predictions across multiple stores, enhancing inventory management and workforce planning.
Performance and use case highlights:
- Multiseries forecasting capabilities that handle complex temporal and hierarchical data structures, supporting “Known in Advance” variables and calendar IDs.
- Case studies indicating significant time savings in model iteration due to automation and prebuilt workflows.
- Training times vary depending on dataset size and complexity; however, DataRobot’s optimized pipelines and ensembling strategies typically achieve faster convergence than manually coded solutions.
- Users benefit from rapid identification of top-performing models, facilitating confident and timely deployment.
User Experience, Collaboration, and Compliance
DataRobot scores highly for user experience, particularly for organizations prioritizing collaboration and governance. Its NextGen Console and Workbench provide a unified environment where data scientists, analysts, and business stakeholders can share projects, track experiments, and manage deployments seamlessly.
Key usability and governance features include:
- Collaboration tools that simplify access control and asset sharing, reducing friction in multi-user workflows.
- Automated compliance documentation and AI governance frameworks addressing regulatory requirements in industries such as healthcare and finance.
- Real-time monitoring and intervention safeguards that support ethical AI use and risk mitigation.
On the downside, DataRobot’s pricing lacks transparency, which may pose a barrier for smaller organizations or AutoML newcomers. The platform’s extensive feature set also introduces a noticeable learning curve, especially for users without prior data science experience. While the user interface is thoughtfully designed, mastering advanced tuning and MLOps integrations generally requires dedicated training and onboarding.
Summary
DataRobot Enterprise AI effectively combines cutting-edge automation with robust MLOps and compliance capabilities, positioning it as a leading choice for enterprises aiming to scale AI responsibly. Its multi-model ensembling via FIRE and automated data preprocessing deliver tangible gains in accuracy and efficiency. The platform’s architecture supports rapid model iteration and comprehensive monitoring—including for emerging generative AI workloads.
Prospective users should carefully weigh the platform’s complexity and pricing opacity against their organizational needs. For teams prepared to invest in onboarding and training, DataRobot offers a powerful, enterprise-grade AutoML solution that balances technical rigor with practical usability, enabling accelerated AI deployment without sacrificing governance or transparency.
Category | Details |
---|---|
Advanced Automation Features |
|
Technical Architecture |
|
Quantitative Performance & Use Cases |
|
User Experience, Collaboration & Compliance |
|
Summary |
|
4. H2O.ai Driverless AI: Technical Depth and Industry Applications
H2O.ai Driverless AI: Technical Depth and Industry Applications
H2O.ai’s Driverless AI platform distinguishes itself through two core technical strengths: proprietary feature engineering and GPU-accelerated training. These capabilities enable rapid development of highly accurate models, positioning Driverless AI as a robust solution for both experimental research and scalable production deployments.
Proprietary Feature Engineering and GPU Acceleration
Unlike standard transformation pipelines, Driverless AI employs evolutionary algorithms that iteratively generate, combine, and evaluate features to maximize predictive performance. This automated process emulates the creativity and trial-and-error approach of expert data scientists but executes at a scale and speed beyond manual capabilities.
A key differentiator is the platform’s use of GPU acceleration during model training. While many AutoML tools rely primarily on CPU resources, Driverless AI harnesses GPUs to expedite training of complex models, including deep learning architectures and convolutional neural networks (CNNs) for image-related tasks. This results in markedly shorter model development cycles, an essential advantage when handling large datasets or tight project timelines.
Supported Algorithms and Performance Benchmarks
Driverless AI offers a wide algorithmic repertoire, covering Gradient Boosting Machines (GBMs), Generalized Linear Models (GLMs), deep neural networks, as well as specialized natural language processing (NLP) and image processing models. Its evolutionary competition approach searches the space of algorithm combinations and hyperparameters to deliver models that frequently surpass manually built counterparts.
Real-world benchmarks validate these claims. For instance, Resolution Life insurance reported significant gains in predictive accuracy for claims triage after adopting Driverless AI. In comparative studies, models generated by the platform often match or exceed expert data scientists’ results while requiring a fraction of the development time.
Scalability with Large Datasets
Built on H2O.ai’s foundation of distributed, in-memory computing, Driverless AI scales efficiently to handle millions of records without degradation in performance. It integrates seamlessly with big data frameworks like Hadoop and Spark, supporting linear scalability as data volumes increase.
The platform’s versatility extends to diverse data types—tabular, text, image, and audio are all natively supported. For example, video inputs are automatically broken down into frames for image classification within the same processing pipeline, simplifying complex multimodal workflows.
Model Explainability Modules
A standout feature of Driverless AI is its comprehensive explainability framework. In contrast to many black-box AutoML solutions, it generates detailed, natural language narratives that elucidate model behavior, feature importance, and the rationale behind predictions. This level of transparency is critical in regulated sectors such as insurance and finance, where auditability and compliance are mandatory.
Interactive dashboards enable both technical and business users to explore model insights intuitively, reducing barriers to adoption across organizational silos and supporting governance requirements.
Integration with Cloud Providers and Open-Source Tools
Driverless AI supports cloud-agnostic deployment across major providers including AWS, Microsoft Azure, and Google Cloud. Its flexible architecture accommodates local, on-premises, or fully cloud-based experimentation. Kubernetes integration facilitates scalable, containerized deployments with granular resource management.
The platform also aligns well with open-source ecosystems. Python and R APIs allow custom preprocessing, postprocessing, and evaluation workflows. Models can be exported in formats compatible with MLOps systems such as H2O’s own MLOps platform and third-party tools like MLflow, enabling smooth incorporation into existing data science pipelines.
Practical Industry Applications
-
Insurance: Driverless AI is extensively used for claims triage automation, fraud detection, and risk scoring. Resolution Life’s public case highlights how the platform accelerates time-to-insight and enhances underwriting accuracy. The explainability features are particularly valuable for meeting stringent regulatory transparency standards.
-
Marketing: The platform supports customer churn prediction, cross-sell and upsell optimization, and personalized campaign targeting. Its ability to blend structured data with unstructured inputs like text and images enables richer customer segmentation and more effective marketing strategies.
-
Manufacturing: Predictive maintenance and process optimization are key applications. Driverless AI analyzes sensor data streams to foresee equipment failures, minimizing downtime and reducing maintenance costs. Its scalability and rapid model iteration support near real-time analytics in industrial IoT settings.
Pros and Cons
Pros:
-
Speed and Efficiency: GPU acceleration combined with automated, evolutionary feature engineering significantly reduces model development time.
-
Interpretability: Embedded explainability modules provide transparent, natural language insights that facilitate trust and regulatory compliance.
-
Scalability: Robust distributed computing supports processing of large, heterogeneous datasets with consistent performance.
-
Integration: Broad compatibility with cloud platforms, Kubernetes, and open-source tools like Python, R, and MLflow ensures flexible deployment and workflow integration.
Cons:
-
Resource Intensity: The platform’s reliance on GPUs and distributed infrastructure often entails higher hardware and operational expenses.
-
Licensing Costs: As commercial software, Driverless AI’s licensing fees may be prohibitive for smaller teams or budget-conscious organizations.
-
Learning Curve: While automation simplifies many tasks, fully leveraging advanced features and orchestrating deployments requires technical expertise, especially in MLOps contexts.
Final Take
H2O.ai Driverless AI stands out as a mature and comprehensive AutoML platform optimized for complex, data-intensive environments where speed, accuracy, and interpretability are paramount. Its evolutionary feature engineering and GPU-accelerated training differentiate it from many competitors, while its explainability framework addresses a critical enterprise need for transparency.
However, its higher resource demands and licensing costs make it best suited for organizations with sufficient scale and budget to support its infrastructure. For insurers, marketers, and manufacturers poised to invest in AI-driven transformation, Driverless AI offers a powerful end-to-end solution that balances cutting-edge technology with practical usability.
Category | Details |
---|---|
Technical Strengths | Proprietary feature engineering, GPU-accelerated training |
Feature Engineering | Evolutionary algorithms that generate, combine, and evaluate features automatically |
GPU Acceleration | Speeds up training of complex models including deep learning and CNNs |
Supported Algorithms | GBMs, GLMs, deep neural networks, NLP, image processing models |
Performance Benchmark | Models often surpass manually built counterparts; Resolution Life insurance case with improved claims triage accuracy |
Scalability | Distributed in-memory computing; integrates with Hadoop and Spark; supports millions of records |
Data Type Support | Tabular, text, image, audio, video (frames extracted automatically) |
Explainability | Natural language narratives on model behavior and feature importance; interactive dashboards |
Cloud & Integration | Supports AWS, Azure, Google Cloud; Kubernetes; Python and R APIs; exports to MLOps platforms like MLflow |
Industry Applications | Insurance (claims triage, fraud detection), Marketing (churn prediction, campaign targeting), Manufacturing (predictive maintenance) |
Pros | Speed & efficiency; interpretability; scalability; broad integration |
Cons | High resource and hardware costs; licensing fees; learning curve for advanced features |
5. Amazon SageMaker Autopilot: Specifications, User Experience, and Use Case Suitability
Amazon SageMaker Autopilot: Specifications, User Experience, and Use Case Suitability
Amazon SageMaker Autopilot is a comprehensive AutoML platform deeply integrated within the AWS ecosystem, designed to automate the entire machine learning (ML) lifecycle—from data ingestion and model building to deployment and monitoring. Unlike many AutoML tools that operate as opaque black boxes, Autopilot emphasizes transparency and control, allowing users to inspect and intervene in the model development process.
Technical Specifications and Automated Model Building
SageMaker Autopilot primarily targets tabular data and supports a variety of problem types, including:
- Regression
- Binary and multiclass classification
- Time-series forecasting (accepting CSV and Parquet formats)
- Fine-tuning of large language models (LLMs) for text generation tasks
Data ingestion is flexible, supporting common enterprise data formats such as CSV and Parquet, as well as protobuf recordIO-like formats that handle n-dimensional tensors. This ensures seamless compatibility with datasets stored in Amazon S3, a standard for many organizations.
The platform’s model building process leverages a blend of built-in algorithms combined through ensembling techniques. Autopilot incorporates the AutoGluon library to ensemble base models like Random Forest, Extra Trees, and linear models, automatically selecting optimal strategies based on dataset size and complexity. This approach strikes a balance between training speed and model performance.
Scalability is a core strength. SageMaker Autopilot integrates tightly with AWS’s distributed training infrastructure, scaling effortlessly from small proof-of-concept experiments to large-scale production workloads. The SageMaker Training Compiler further enhances training efficiency by optimizing model graphs, reducing both training time and resource consumption.
For deployment, Autopilot benefits from native AWS integrations. Models can be deployed directly as real-time endpoints or batch inference jobs within SageMaker, offering flexible serving options. Additionally, it integrates with AWS security features such as IAM, VPC, and KMS, making it well-suited for environments with strict compliance and security requirements.
User Experience: Ease of Use, Customization, and Cost Efficiency
In terms of usability, SageMaker Autopilot offers a balanced experience that caters both to data scientists seeking control and business users desiring simplicity. Its evolution into SageMaker Canvas introduces a more approachable, no-code interface featuring integrated data preparation tools, what-if analysis, and built-in visualizations. This lowers the barrier for non-technical users to create and interpret models without requiring programming skills.
However, user feedback from platforms like G2 and Gartner Peer Insights reveals some constraints. While Autopilot automates much of the workflow, advanced users may find customization options—such as hyperparameter tuning and algorithm selection—more limited compared to competitors like Google Vertex AI, which offers neural architecture search and more granular controls. Autopilot’s opinionated automation can restrict flexibility for domain-specific fine-tuning.
Cost efficiency benefits from AWS’s pay-as-you-go pricing and serverless architecture, which eliminate the need for provisioning dedicated instances during training or inference. This model can reduce overhead for intermittent workloads. However, costs can escalate in sustained or large-scale deployments, as resource consumption scales proportionally with job size and frequency.
Users also appreciate the detailed data exploration and model performance reports Autopilot generates. These include statistical metrics such as Pearson’s correlation and residual histograms, which help surface data quality issues and provide actionable model insights. Such transparency supports iterative refinement and troubleshooting.
Use Case Suitability and Real-World Deployment Examples
Amazon SageMaker Autopilot is particularly well-suited for enterprises with a strong AWS footprint requiring scalable, end-to-end ML automation embedded within their cloud infrastructure. Typical use cases include:
- Ecommerce: Automating demand forecasting, customer segmentation, and dynamic pricing using tabular and time-series data.
- Internet of Things (IoT): Developing predictive maintenance models that analyze sensor data streams to anticipate equipment failures.
- Predictive Maintenance: Optimizing maintenance scheduling and reducing downtime with support for both batch and real-time inference.
A notable example is the BMW Group, which leverages SageMaker Canvas (which integrates Autopilot) to augment AI/ML workflows across manufacturing and supply chain operations. This demonstrates the platform’s capability to handle complex, industrial-scale use cases requiring robust automation and integration.
Competitive Advantages and Drawbacks
Advantages:
- Deep AWS Integration: Offers seamless connectivity with services such as Amazon S3, IAM, VPC, Lambda, and SageMaker Training Compiler, simplifying data pipelines, security, and deployment.
- Scalability: Efficiently handles workloads ranging from small datasets to enterprise-scale ML projects with distributed training and deployment.
- Transparency and Control: Provides full visibility into the AutoML process, model diagnostics, and data quality metrics, which is uncommon among AutoML platforms.
- Cost Efficiency for Sporadic Use: Serverless infrastructure and pay-as-you-go pricing help minimize costs for intermittent workloads.
Drawbacks:
- Limited Customization: Less flexibility in tuning algorithms and hyperparameters compared to platforms like Google Vertex AI, which offers advanced AutoML features including neural architecture search.
- Moderate Learning Curve: Despite improvements with SageMaker Canvas, the broader SageMaker ecosystem requires familiarity with AWS services and ML concepts.
- Pricing Complexity: Costs can escalate quickly for continuous or large-scale training, necessitating careful resource monitoring and cost management.
Compared to competitors such as Google Vertex AI or Microsoft Azure ML, SageMaker Autopilot excels in integration, scalability, and enterprise-grade security but may be less accessible to non-technical users and lacks some cutting-edge AutoML capabilities.
Summary: Amazon SageMaker Autopilot is a powerful, enterprise-grade AutoML platform ideal for organizations heavily invested in AWS. It combines robust automation, transparency, and scalability, making it a strong fit for production-grade ML workflows in ecommerce, IoT, and predictive maintenance. However, prospective users should weigh its customization limits and pricing considerations against their specific requirements and technical expertise.
Category | Details |
---|---|
Supported Problem Types | Regression, Binary & Multiclass Classification, Time-series Forecasting, Fine-tuning LLMs for Text Generation |
Data Formats | CSV, Parquet, Protobuf RecordIO-like n-dimensional tensors |
Model Building | Built-in Algorithms, Ensembling with AutoGluon (Random Forest, Extra Trees, Linear Models) |
Scalability | AWS Distributed Training Infrastructure, SageMaker Training Compiler |
Deployment Options | Real-time Endpoints, Batch Inference Jobs within SageMaker |
Security Integrations | AWS IAM, VPC, KMS |
User Experience | Balanced for Data Scientists & Business Users, SageMaker Canvas No-Code Interface, Integrated Data Prep & Visualizations |
Customization | Limited Hyperparameter Tuning & Algorithm Selection Compared to Competitors |
Cost Model | Pay-as-you-go, Serverless Architecture; Cost scales with usage |
Use Cases | Ecommerce (Demand Forecasting, Customer Segmentation, Dynamic Pricing), IoT (Predictive Maintenance), Predictive Maintenance Scheduling |
Notable Deployment | BMW Group – Manufacturing & Supply Chain AI/ML Workflows |
Advantages | Deep AWS Integration, Scalability, Transparency & Control, Cost Efficiency for Intermittent Use |
Drawbacks | Limited Customization, Moderate Learning Curve, Pricing Complexity for Large-Scale Use |