Top 10 Data Labeling Tools Powering Accurate Machine Learning
- Introduction: Why Data Labeling Tools Are Critical for Machine Learning Success
- Introduction: Why Data Labeling Tools Are Critical for Machine Learning Success
- The Foundational Role of Data Labeling in Machine Learning Pipelines
- Ethical Dimensions: Accuracy and Bias Mitigation in Data Labeling
- Criteria for Selecting Top Data Labeling Tools
- The Challenge of Balancing Automation with Human Expertise
- Conclusion: Navigating the Data Labeling Landscape with Informed Choices for Machine Learning Excellence
- Conclusion: Navigating the Data Labeling Landscape with Informed Choices for Machine Learning Excellence
- 1. SuperAnnotate: Enterprise-Grade Annotation with Advanced AI-Assisted Workflows
- SuperAnnotate: Enterprise-Grade Annotation with Advanced AI-Assisted Workflows
- Multi-Modal Support for Diverse Enterprise Needs
- AI-Powered Pre-Labeling and Active Learning to Boost Efficiency
- Collaborative Environment Optimized for Distributed Teams
- Security Compliance and Customization for High-Stakes Applications
- Real-World Impact: Scaling Autonomous Driving AI Annotation
- 2. Appen: Licensed Platform Combining Computer Vision and NLP Annotation
- 2. Appen: Licensed Platform Combining Computer Vision and NLP Annotation
- Hybrid Human-in-the-Loop and AI-Assisted Annotation
- Scalability and Quality Controls for Large-Scale Projects
- Security, Compliance, and Enterprise Suitability
- Pricing Model and Ideal Use Cases
- 3. Labelbox: Data-Centric AI Platform with Robust API and Custom Workflow Support
- Labelbox: Data-Centric AI Platform with Robust API and Custom Workflow Support
- Technical Specifications and Core Features
- Integration with MLOps Pipelines and Cloud Ecosystems
- User Experience and Collaboration Features
- Final Thoughts
- 4. Encord: Specialized Active Learning Platform for Large Datasets and High-Security Needs
- Encord: Specialized Active Learning Platform for Large Datasets and High-Security Needs
- Active Learning at Scale: Cutting Costs Without Cutting Corners
- Robust Technical Architecture: Collaboration, Control, and Traceability
- Designed for High-Security Environments: Meeting Rigorous Compliance Standards
- Bringing It All Together: Efficient, Accurate, and Secure AI Data Labeling
- 5. Dataloop: End-to-End Data Operating System Integrating Annotation, Management, and Automation
- 5. Dataloop: End-to-End Data Operating System Integrating Annotation, Management, and Automation
- A Unified Platform Covering the Full AI Data Lifecycle
- Automation Tools Enhancing Efficiency and Data Quality
- Support for Multimodal Data and Scalable Cloud Infrastructure
- Real-Time Data Stream Processing and Customizable Interfaces
- Industry Use Cases: Enabling Rapid Iteration and Deployment
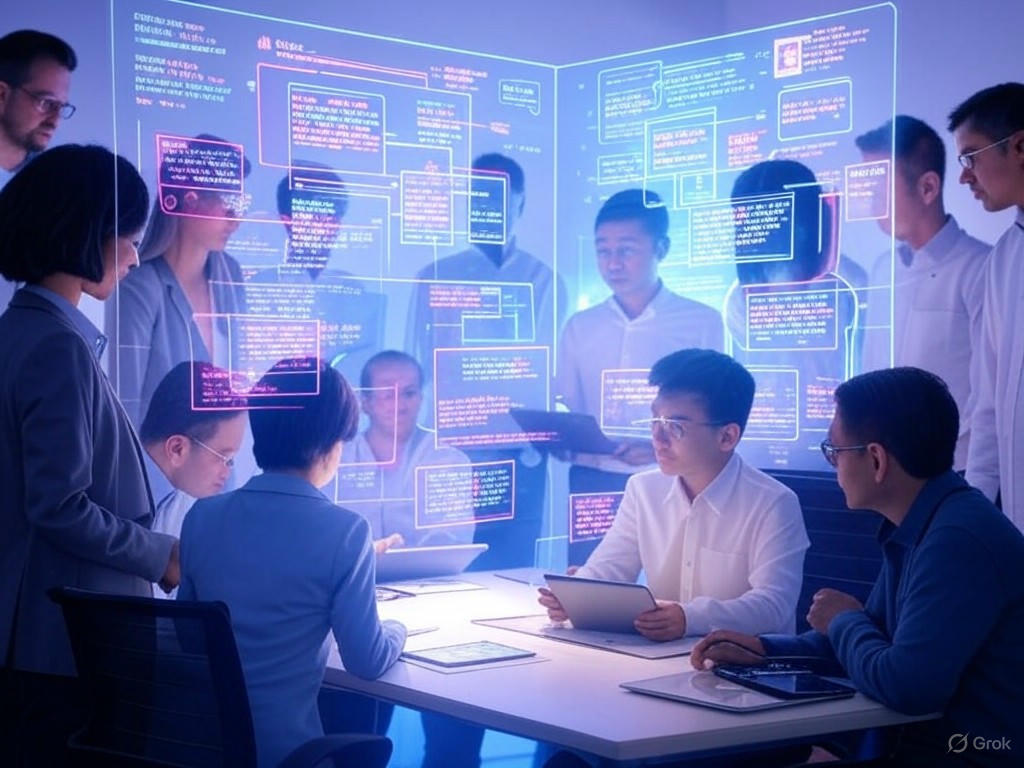
Introduction: Why Data Labeling Tools Are Critical for Machine Learning Success
Introduction: Why Data Labeling Tools Are Critical for Machine Learning Success
Imagine building a sophisticated AI model only to discover it making glaring errors or, worse, perpetuating harmful biases. At the root of such failures often lies a deceptively simple yet critical factor: the quality of the labeled data used for training. Data labeling is far more than a routine preparatory step—it is the very foundation upon which machine learning (ML) systems stand. Without precise, consistent, and ethically curated annotations, even the most advanced algorithms falter in accuracy, fairness, and robustness.
The Foundational Role of Data Labeling in Machine Learning Pipelines
Data labeling converts raw data into meaningful, machine-readable insights. Much like teaching a child to recognize objects by pointing and naming them, labeled data instructs AI on what to identify and how to interpret it. Research from sources such as Granica.ai and DQLabs underscores that the quality, completeness, and relevance of labeled data directly influence model performance.
Poorly labeled or noisy data leads to unreliable predictions—an unacceptable risk in high-stakes domains like healthcare or autonomous vehicles. For instance:
-
Computer Vision: Precise bounding boxes and segmentation labels enable models to differentiate between similar objects, such as distinguishing pedestrians from cyclists in traffic surveillance.
-
Natural Language Processing (NLP): Accurate text annotations enhance sentiment analysis and intent detection, allowing chatbots to respond appropriately.
Beyond accuracy, data labeling is central to ensuring model fairness. Biases introduced during annotation—whether through inconsistent labeling or underrepresentation of certain groups—can perpetuate societal inequities. The well-documented case of Amazon’s AI recruiting tool, which favored male resumes due to historical biases in its training data, highlights the ethical imperative to curate labeled data that reflects diverse and balanced perspectives.
Ethical Dimensions: Accuracy and Bias Mitigation in Data Labeling
Ensuring data labeling contributes to both technical excellence and ethical AI is increasingly urgent as AI systems influence decisions affecting individuals and communities.
Ethical data labeling entails:
-
Precision and Transparency: Annotations must be accurate and consistently documented.
-
Accountability: Clear processes and oversight mechanisms are essential.
-
Proactive Bias Mitigation: Labelers should be trained to recognize and avoid subjective or stereotypical annotations.
While automated tools can flag anomalies or inconsistencies, human oversight remains indispensable to provide contextual understanding and nuance—especially for complex or sensitive content. For example, Stanford HAI notes that annotators often face stressful conditions when labeling toxic or sensitive materials, underscoring the importance of fair labor practices and respectful treatment.
Ethical labeling also aligns with emerging standards such as ISO 42001, which set benchmarks for responsible AI. Organizations embedding ethics into their data labeling workflows not only reduce risks of bias and privacy violations but also build greater trust with users and regulators.
Criteria for Selecting Top Data Labeling Tools
Given the high stakes, selecting the right data labeling tool is a strategic decision. The market offers a diverse range—from open-source platforms like Diffgram to commercial solutions such as SuperAnnotate, Appen, and Amazon SageMaker Ground Truth—each with unique capabilities.
Key selection criteria include:
-
Technical Capabilities: Support for automation and AI-assisted labeling features that accelerate annotation without compromising quality. For example, Labellerr’s AI-assisted pre-labeling reduces manual effort by generating initial annotations for human review.
-
Usability and Collaboration: Intuitive interfaces with drag-and-drop functionality, keyboard shortcuts, and real-time collaboration tools that enable efficient, coordinated annotation across distributed teams.
-
Support for Various Data Types: Capability to handle multiple modalities—including images, video, audio, text, PDFs, and specialized formats such as DICOM and LiDAR—ensuring applicability across industries.
-
Scalability: Ability to handle growing datasets and peak workloads without performance degradation, supporting both small and large annotation teams.
-
Security and Compliance: Robust encryption, access controls, audit trails, and adherence to standards like GDPR and SOC 2 are essential for protecting sensitive data.
-
Cost-Effectiveness: Transparent pricing models balancing upfront investment with long-term ROI, factoring in labor savings from automation and the cost implications of errors from poor labeling.
-
Balancing Automation and Human Oversight: The nuanced challenge is to find the right mix between machine efficiency and human expertise. Automation expedites routine tasks, but complex, ambiguous, or ethically sensitive cases require human judgment to ensure precision and fairness.
The Challenge of Balancing Automation with Human Expertise
With over fifteen years of experience architecting AI systems, I have witnessed the shift from fully manual annotation to over-reliance on automation—and the pitfalls of both extremes. Humans contribute contextual understanding, ethical discernment, and adaptability beyond current AI capabilities. Meanwhile, AI-powered tools provide scale and speed unattainable by humans alone.
This hybrid model is now the industry standard. Platforms like Shaip Cloud and Sama integrate human-in-the-loop workflows, ensuring that automated labels undergo expert review and refinement. Such systems enhance accuracy and facilitate early detection and correction of biases.
Looking ahead, increasingly sophisticated tools will learn iteratively from human corrections to improve over time. Nonetheless, the human element remains vital. Ethical AI demands it.
In summary, data labeling is not merely a preparatory task but the cornerstone of trustworthy, effective, and fair machine learning. Selecting the right tools requires careful evaluation of technical features, ethical safeguards, and operational needs. Only with high-quality labeled data can AI models fulfill their promise without unintended harm or bias. The tools examined in this article embody this balance, empowering teams to build AI systems ready for the complexities of 2025 and beyond.
Selection Criteria | Description | Examples / Notes |
---|---|---|
Technical Capabilities | Support for automation and AI-assisted labeling features to accelerate annotation without compromising quality. | Labellerr’s AI-assisted pre-labeling reduces manual effort by generating initial annotations for human review. |
Usability and Collaboration | Intuitive interfaces with drag-and-drop, keyboard shortcuts, and real-time collaboration for distributed teams. | Enables efficient, coordinated annotation workflows. |
Support for Various Data Types | Capability to handle multiple modalities including images, video, audio, text, PDFs, and specialized formats. | Includes formats such as DICOM and LiDAR for cross-industry applicability. |
Scalability | Ability to handle growing datasets and peak workloads without performance degradation. | Supports both small and large annotation teams effectively. |
Security and Compliance | Robust encryption, access controls, audit trails, and adherence to standards like GDPR and SOC 2. | Essential for protecting sensitive data in regulated environments. |
Cost-Effectiveness | Transparent pricing balancing upfront investment with long-term ROI, including labor savings and error costs. | Considers automation benefits and risks from poor labeling. |
Balancing Automation and Human Oversight | Finding the right mix between machine efficiency and human expertise for precision and fairness. | Platforms like Shaip Cloud and Sama integrate human-in-the-loop workflows for review and bias correction. |
Conclusion: Navigating the Data Labeling Landscape with Informed Choices for Machine Learning Excellence
Conclusion: Navigating the Data Labeling Landscape with Informed Choices for Machine Learning Excellence
Selecting the right data labeling tool is far more than a procedural step—it is a strategic cornerstone that can determine the success or failure of your machine learning project. The vast array of platforms available, from SuperAnnotate’s enterprise-grade solution designed for complex vision tasks to Diffgram’s open-source, collaborative environment, reflects the multifaceted nature of real-world AI applications.
When making your choice, start by assessing your project’s scale and data modalities. Are you tackling millions of images and LiDAR frames for autonomous vehicles, or managing nuanced text annotations for a specialized NLP model? Different tools shine in distinct domains—some excel with images and video, others with audio or multimodal sensor data. For instance, SuperAnnotate’s robust multi-modal support and AI-assisted pre-labeling streamline large-scale annotation, while Appen leverages a vast global workforce for diverse, multilingual NLP and computer vision projects.
Security requirements introduce another essential dimension. Industries such as healthcare and finance mandate stringent compliance features—encryption, access controls, audit trails—that only select platforms like Encord can guarantee with certifications including HIPAA, SOC 2, and GDPR. These safeguards are critical not just for protecting sensitive information but also for meeting evolving regulatory standards like the EU AI Act.
Automation capabilities, including AI-assisted pre-labeling, active learning, and model-in-the-loop workflows, offer tremendous efficiency gains by reducing manual annotation effort and accelerating dataset creation. Encord’s active learning platform, for example, intelligently prioritizes ambiguous or rare samples, improving labeling speed while maintaining accuracy. However, these automation benefits must be balanced with the indispensable role of human expertise to ensure label quality and mitigate bias. Human-in-the-loop (HITL) processes have demonstrated over 30% reductions in false positives for classification tasks, underscoring their importance in handling complex or sensitive data interpretations.
Ethical and practical challenges remain central in data labeling. Automation is powerful but insufficient on its own; without human oversight, models risk perpetuating biases embedded in training data or annotation workflows. Biases related to gender, age, and ability can infiltrate AI systems if datasets lack diversity or if labeling guidelines are inconsistently applied. Addressing these issues requires continuous vigilance through diverse annotation teams, transparent processes, and tooling that supports quality control metrics such as inter-annotator agreement and confusion matrices.
Looking forward, the data labeling landscape is evolving rapidly. Emerging trends include continuous learning annotation platforms that adapt dynamically as AI models evolve, real-time collaborative dashboards enhancing team coordination, and the integration of synthetic data to supplement scarce real-world datasets. The market is projected to grow aggressively, with estimates exceeding a 26% compound annual growth rate over the next five years, driven by demand in generative AI, autonomous systems, and healthcare diagnostics.
Future platforms will blur the lines between automation and human expertise more seamlessly. Adaptive annotation tools will not only expedite labeling but also flag ambiguous or potentially biased examples for human review, fostering a hybrid approach that safeguards AI systems’ contextual understanding and minimizes unintended harms.
Ultimately, choosing a data labeling tool means aligning technology with your project’s unique requirements—scale, data types, security needs, and ethical imperatives. As AI permeates critical sectors, the integrity and quality of labeled data remain foundational to building reliable, fair, and impactful AI models. Maintaining informed awareness of evolving tools, embedding rigorous human oversight, and committing to ethical data management are key to unlocking the full potential of AI while respecting societal values.
The future favors those who master this balance: where human insight and machine efficiency converge to drive machine learning excellence in an increasingly complex and responsible AI ecosystem.
Aspect | Details | Examples/Tools |
---|---|---|
Project Scale & Data Modalities | Assess volume and type of data (images, video, audio, text, LiDAR) | SuperAnnotate (multi-modal, AI-assisted), Appen (multilingual NLP, vision) |
Security & Compliance | Encryption, access controls, audit trails, regulatory certifications | Encord (HIPAA, SOC 2, GDPR) |
Automation Capabilities | AI-assisted pre-labeling, active learning, model-in-the-loop workflows | Encord (active learning prioritizes ambiguous samples) |
Human-in-the-Loop (HITL) | Human oversight to ensure label quality, reduce false positives by 30% | General best practice in labeling workflows |
Ethical Challenges | Bias mitigation (gender, age, ability), diverse teams, quality control metrics | Inter-annotator agreement, confusion matrices, transparent processes |
Emerging Trends | Continuous learning platforms, real-time collaboration, synthetic data integration | Next-gen annotation tools with hybrid human-machine approaches |
Market Outlook | Projected 26% CAGR driven by generative AI, autonomous systems, healthcare | Industry growth forecast |
Strategic Considerations | Align tools with project requirements, embed human oversight, ethical management | Ensures reliable, fair, and impactful AI models |
1. SuperAnnotate: Enterprise-Grade Annotation with Advanced AI-Assisted Workflows
SuperAnnotate: Enterprise-Grade Annotation with Advanced AI-Assisted Workflows
When tackling complex, large-scale AI projects—such as autonomous driving or medical imaging—the choice of a data labeling platform becomes critical. The need for versatility, security, and scalability is paramount, and SuperAnnotate has distinguished itself as a leader in this space. Consistently ranked #1 on G2, it is trusted by enterprises facing the most demanding annotation challenges.
Multi-Modal Support for Diverse Enterprise Needs
SuperAnnotate excels through its unified platform capable of handling multiple data modalities seamlessly. It supports images, video, text, audio, and even LiDAR data, enabling projects that require integrated, rich representations to train sophisticated AI models.
For example, autonomous vehicles rely not just on camera images but also fuse LiDAR point clouds and radar data to achieve robust perception. SuperAnnotate facilitates such workflows by allowing annotators to efficiently cross-reference and label multiple data streams. Similarly, in medical imaging, combining MRI scans with clinical reports demands precise annotation across both visual and textual domains—capabilities that SuperAnnotate fully supports.
AI-Powered Pre-Labeling and Active Learning to Boost Efficiency
Manual labeling at scale is time-consuming and expensive. SuperAnnotate addresses this bottleneck with AI-assisted pre-labeling, where machine learning models generate initial annotations that human experts review and refine. This collaborative approach accelerates labeling throughput without sacrificing accuracy.
Active learning further enhances efficiency by identifying the most informative or uncertain samples for annotation, focusing human effort where it will most improve model performance. SuperAnnotate’s active learning algorithms have demonstrated substantial gains in classification, detection, and segmentation tasks.
Integrated quality assurance tools provide real-time error detection and correction workflows. These tightly coupled feedback loops minimize annotation errors early, crucial because inaccurate labels can degrade downstream model quality.
Collaborative Environment Optimized for Distributed Teams
AI projects frequently involve distributed teams and multiple annotation vendors. Disjointed workflows can cause delays, quality inconsistencies, and operational overhead. SuperAnnotate addresses this by offering a centralized collaboration hub that unifies data operations and vendor management.
Key features include:
- Real-time visibility into annotation progress and quality metrics
- Role-based access control to enforce data security
- Seamless communication channels to resolve ambiguities swiftly
- Performance analytics to assess and optimize annotator productivity
These capabilities are essential for scaling annotation workflows while ensuring quality and security standards remain uncompromised.
Security Compliance and Customization for High-Stakes Applications
Enterprises handling sensitive data—such as patient health records or autonomous driving datasets—demand stringent security. SuperAnnotate meets these needs with enterprise-grade compliance certifications, including GDPR and SOC2.
Robust security features include:
- Single Sign-On (SSO)
- Multi-Factor Authentication (2FA)
- Encrypted data storage and transmission
- Audit trails and governance controls
Highly customizable, the platform allows teams to tailor annotation schemas, workflows, and integrations to domain-specific requirements. This flexibility is vital for projects where off-the-shelf tools fall short.
Real-World Impact: Scaling Autonomous Driving AI Annotation
Consider a large-scale computer vision project undertaken by an autonomous vehicle manufacturer faced with annotating millions of images and LiDAR frames for object detection and semantic segmentation models. By deploying SuperAnnotate, the team harnessed AI-powered pre-labeling to accelerate initial annotation, employed active learning to prioritize challenging edge cases—such as unusual pedestrian movements—and centralized coordination of multiple annotation vendors across continents.
The outcomes were impressive:
- A 10x increase in labeling speed
- Enhanced label consistency and quality
- Faster model development cycles
- Full adherence to stringent automotive data security protocols
This example underscores how SuperAnnotate’s advanced AI-assisted workflows and enterprise features enable high-stakes, complex annotation projects to succeed.
In summary, SuperAnnotate embodies a thoughtfully engineered, AI-enhanced data labeling platform that empowers enterprises to address complex machine learning challenges confidently. Its comprehensive multi-modal support, cutting-edge AI workflows, collaborative environment, and rigorous security compliance make it a top choice for organizations aiming for scalable, reliable, and ethical annotation solutions.
Feature Category | Details |
---|---|
Multi-Modal Support | Images, Video, Text, Audio, LiDAR data |
AI-Powered Capabilities | Pre-labeling, Active Learning, Real-time error detection and correction |
Collaboration Features | Real-time progress visibility, Role-based access control, Communication channels, Performance analytics |
Security Compliance | GDPR, SOC2, Single Sign-On (SSO), Multi-Factor Authentication (2FA), Encrypted data storage and transmission, Audit trails |
Customization | Annotation schemas, workflows, integrations tailored to domain-specific requirements |
Real-World Impact | 10x labeling speed increase, Enhanced label consistency and quality, Faster model development, Adherence to automotive data security protocols |
2. Appen: Licensed Platform Combining Computer Vision and NLP Annotation

2. Appen: Licensed Platform Combining Computer Vision and NLP Annotation
Appen’s licensed platform embodies the critical balance between human expertise and AI-driven automation—a synergy fundamental to delivering high-quality data labeling for complex machine learning projects. It employs a hybrid annotation approach that integrates AI-assisted tools with a vast, vetted global workforce, enabling effective handling of diverse data types such as computer vision and natural language processing (NLP).
Hybrid Human-in-the-Loop and AI-Assisted Annotation
Appen distinguishes itself by orchestrating human-in-the-loop workflows alongside AI automation. This approach goes beyond mere efficiency; it ensures annotation accuracy and contextual awareness that fully automated tools often miss. For instance, in NLP projects, understanding subtleties like sarcasm, idioms, or domain-specific jargon demands human judgment that AI alone cannot reliably provide. Similarly, computer vision tasks—such as discerning objects partially obscured or under challenging lighting—benefit immensely from experienced annotators collaborating with AI pre-labeling.
The platform intelligently assigns annotation tasks based on contributors’ skills using AI, boosting both speed and precision. This dynamic task allocation maximizes workforce productivity while enforcing stringent quality controls. These controls encompass continuous performance monitoring and multi-layered review processes, which effectively identify and eliminate inconsistencies and errors.
Scalability and Quality Controls for Large-Scale Projects
Managing millions of annotations requires more than advanced technology; it demands a scalable, dependable workforce coupled with rigorous quality assurance mechanisms. Appen boasts a global contributor network exceeding one million individuals across more than 170 countries. This extensive reach enables rapid project scaling while supporting multiple languages and diverse cultural contexts.
Appen supplements its workforce with automated quality controls, including its proprietary AI Detector that identifies and removes AI-generated or low-quality human annotations. This capability is crucial in an era where synthetic data could undermine model integrity. The platform’s quality assurance workflows feature ongoing annotator training, continuous task monitoring, and statistical sampling, achieving annotation accuracies often surpassing 99% for complex multimodal datasets.
Real-world use cases underscore this expertise: Appen has supported autonomous vehicle manufacturers requiring meticulous labeling of sensor data, as well as enterprises developing advanced multilingual NLP models. Additionally, its off-the-shelf dataset catalog comprises over 270 datasets featuring audio, video, image, and text data in more than 80 languages—demonstrating the platform’s versatility and breadth.
Security, Compliance, and Enterprise Suitability
Handling sensitive data, especially within regulated industries, demands uncompromising data security and compliance. Appen’s infrastructure supports secure data pipelines compliant with international data protection standards such as GDPR and the forthcoming EU AI Act. This ensures that enterprises can safely outsource annotation tasks without exposing themselves to data breaches or regulatory penalties.
Furthermore, Appen’s licensed platform integrates seamlessly with enterprise workflows, offering comprehensive project management features, performance dashboards, and immutable audit trails. Its vetted workforce model guarantees that annotators undergo continuous screening, training, and monitoring—an essential factor for organizations that must demonstrate data provenance and maintain auditability.
Pricing Model and Ideal Use Cases
Appen’s pricing aligns with enterprise-scale requirements, reflecting the complexity, volume, and quality of its annotation services. While it may not be the most cost-effective choice for small-scale or exploratory projects, its value proposition lies in dependable quality, robust compliance guarantees, and the capacity to manage massive, multifaceted datasets efficiently.
Enterprises that need reliable integration of a vetted global workforce, support for a wide range of data formats, and stringent quality controls will find Appen particularly well suited. Examples include companies developing high-stakes AI applications such as autonomous driving systems, healthcare NLP models, or multilingual voice assistants, which benefit from Appen’s mature processes and scalability.
In summary, Appen’s licensed annotation platform exemplifies how a hybrid human-AI annotation strategy, powered by a global vetted workforce and reinforced by strict quality and security protocols, can meet the demanding needs of modern AI projects. This approach underscores the enduring importance of the human element in training AI systems capable of reliable, real-world performance.
Aspect | Details |
---|---|
Platform Type | Licensed Platform Combining Computer Vision and NLP Annotation |
Annotation Approach | Hybrid Human-in-the-Loop and AI-Assisted Annotation |
Key Features | AI-assisted tools, global vetted workforce, dynamic task allocation, multi-layered quality controls |
Scalability | Global contributor network of over 1 million individuals across 170+ countries; supports multiple languages and cultural contexts |
Quality Controls | Continuous performance monitoring, multi-layered review, AI Detector for low-quality data, ongoing annotator training, statistical sampling; accuracy often surpassing 99% |
Use Cases | Autonomous vehicle sensor data labeling, advanced multilingual NLP models, audio/video/image/text datasets in 80+ languages |
Security & Compliance | Secure data pipelines, GDPR and EU AI Act compliant, vetted workforce with continuous screening and audit trails |
Enterprise Features | Project management, performance dashboards, immutable audit trails, seamless workflow integration |
Pricing Model | Enterprise-scale pricing based on complexity, volume, and quality; not optimal for small-scale projects |
Ideal Use Cases | High-stakes AI applications like autonomous driving, healthcare NLP, multilingual voice assistants |
3. Labelbox: Data-Centric AI Platform with Robust API and Custom Workflow Support
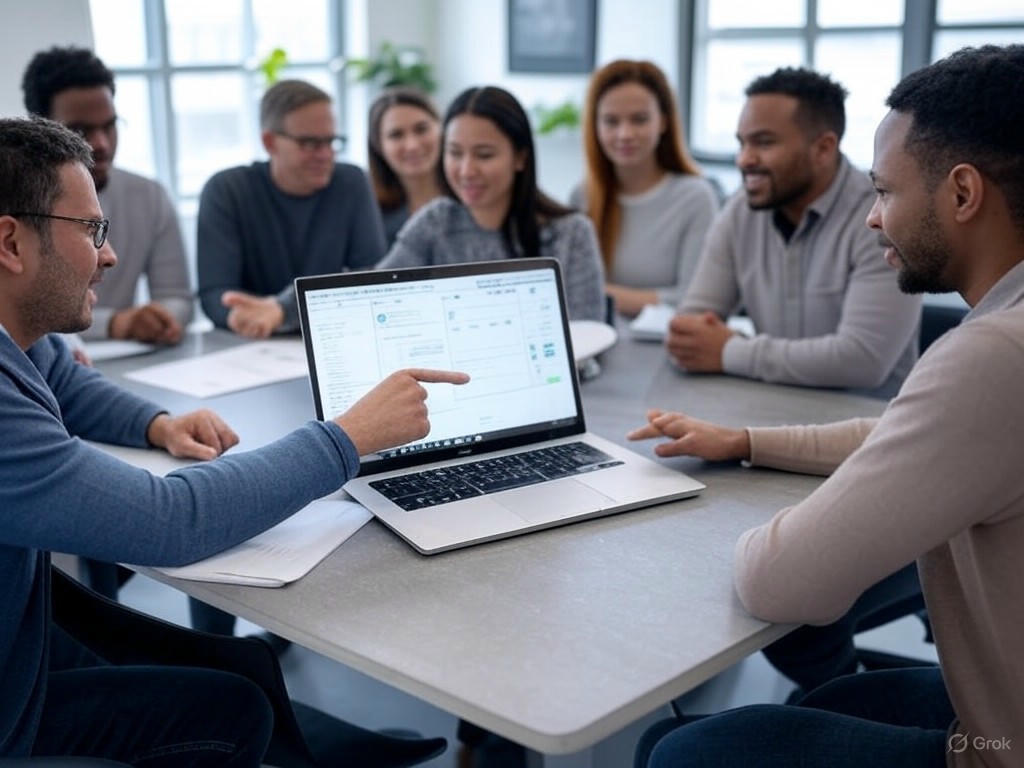
Labelbox: Data-Centric AI Platform with Robust API and Custom Workflow Support
In the competitive landscape of data labeling tools, Labelbox distinguishes itself through a robust, end-to-end approach that emphasizes data quality management, flexible automation, and seamless collaboration. It is more than a conventional labeling tool—Labelbox is a comprehensive data-centric AI platform designed to orchestrate the entire data pipeline, from annotation to model training, prioritizing both usability and precision for machine learning teams handling complex, multimodal datasets.
Technical Specifications and Core Features
Labelbox supports a broad spectrum of annotation types spanning multiple data modalities, including 2D images, 3D point clouds, and video sequences. This versatility is essential for teams working in computer vision fields such as autonomous vehicles, robotics, and augmented reality, where data is diverse and temporally dynamic.
Key strengths of Labelbox include its advanced automation capabilities, notably its integration of pre-labeling models. These AI models generate initial annotations, which human labelers then review and refine. This hybrid workflow balances the meticulousness of manual labeling with the speed of automation, enabling significant efficiency gains. Labelbox quantifies this efficiency through an automation efficiency score, with some projects reporting up to a two-thirds reduction in labeling time and associated costs.
The platform’s interactive, node-based workflow editor stands out by allowing users to create customized, multi-step pipelines tailored to their project’s complexity and quality standards. Its visual flowchart interface offers intuitive tracking of dataset progress and quality status, enhancing transparency and control throughout the annotation lifecycle.
Integration with MLOps Pipelines and Cloud Ecosystems
Labelbox’s true potential manifests when integrated into broader MLOps frameworks. It supports seamless connections with cloud storage solutions such as AWS S3 and enables direct orchestration of model training within its interface. This unified environment reduces context switching for data scientists and ML engineers, streamlining dataset management, annotation, and training workflows in one place.
Designed with an API-first philosophy, Labelbox facilitates extensive automation and extensibility. Teams can programmatically manage projects, upload data, and retrieve labeled datasets, fitting naturally into CI/CD workflows prevalent in sophisticated AI organizations.
Strategic partnerships with technology leaders like Google Cloud and LangSmith further enrich Labelbox’s ecosystem. These collaborations introduce advanced tooling that supports cutting-edge frontier models, including large language models (LLMs) and multimodal AI. For example, its recent multimodal chat editor empowers subject matter experts to collaboratively annotate and evaluate diverse data types—ranging from audio and images to videos and documents—addressing the specific needs of generative AI applications.
User Experience and Collaboration Features
Labelbox’s user interface is thoughtfully designed to democratize data annotation, enabling not only professional labelers but also domain experts without deep machine learning expertise to contribute effectively. Enhancements such as form-based UI components and turn-based audio/video support simplify complex annotation tasks and reduce training overhead.
Collaboration is deeply embedded in the platform through features like role-based access control, leaderboard-driven quality metrics, and real-time project status monitoring. These tools facilitate coordinated efforts among distributed teams, ensuring consistent quality standards and accountability.
On the performance front, Labelbox emphasizes rigorous data quality metrics, implementing over 15 tailored measures adapted to various annotation types. For text-heavy tasks, fine-tuned large language models assess annotation similarity against ground truth, illustrating how AI can both assist and audit human labeling efforts. Such layered quality assurance is crucial in training generative AI systems, where minor annotation errors may propagate into significant model biases or hallucinations.
Real-world applications demonstrate Labelbox’s adaptability: generative AI fine-tuning projects benefit from its multimodal labeling and review workflows, while computer vision initiatives leverage its robust 3D and video annotation capabilities. Its balanced approach between human-in-the-loop accuracy and AI-augmented automation positions Labelbox as an attractive solution for enterprises seeking scalable, high-quality data-centric AI development.
Final Thoughts
Labelbox epitomizes the evolution of data labeling platforms by bridging raw data preparation with model readiness through thoughtful automation and collaborative design. Its flexible workflow editor, broad modality support, and deep integration with MLOps and cloud ecosystems make it a compelling choice for teams tackling advanced AI systems, especially those working with complex, multimodal datasets.
Success with Labelbox, as with any platform, hinges on aligning its capabilities with specific project requirements and organizational scale. While it offers a more user-friendly and cost-effective alternative to some enterprise-grade competitors, careful evaluation of its automation efficiency and integration features within existing AI infrastructures is essential.
Ultimately, Labelbox is not merely a tool for data annotation—it is a data factory that powers the next generation of AI models with precision, scalability, and ethical oversight. This capability is increasingly vital as AI systems expand into generative and multimodal frontiers demanding both speed and rigorous quality control.
Category | Details |
---|---|
Supported Data Modalities | 2D images, 3D point clouds, video sequences, audio, documents |
Annotation Types | Multimodal annotations including image, video, text, and audio |
Automation Features | Pre-labeling AI models for initial annotations, automation efficiency score (up to 66% time/cost reduction) |
Workflow Editor | Interactive, node-based, visual flowchart for custom multi-step pipelines |
Integration | Cloud storage (AWS S3), direct model training orchestration, API-first for CI/CD workflows |
Partnerships | Google Cloud, LangSmith (support for LLMs and multimodal AI) |
User Interface | Form-based UI components, turn-based audio/video annotation support |
Collaboration Features | Role-based access control, leaderboard quality metrics, real-time project monitoring |
Data Quality Metrics | 15+ tailored measures, LLM-based similarity assessment for text annotations |
Use Cases | Generative AI fine-tuning, computer vision (3D and video annotation), multimodal data projects |
Key Benefits | Precision, scalability, ethical oversight, hybrid human-AI labeling workflow |
4. Encord: Specialized Active Learning Platform for Large Datasets and High-Security Needs
Encord: Specialized Active Learning Platform for Large Datasets and High-Security Needs
What if your data labeling platform could not only accelerate annotation but also actively reduce costs while maintaining top-notch accuracy? Encord excels precisely here by harnessing active learning to optimize annotation efficiency on large-scale datasets—especially when stakes are high and security is non-negotiable.
Active Learning at Scale: Cutting Costs Without Cutting Corners
Active learning is not just a buzzword for Encord; it’s the core principle driving its ability to make data labeling smarter, not harder. By intelligently selecting the most informative and diverse samples for annotation, Encord minimizes redundant labeling efforts and focuses human expertise where it counts. This strategic approach drastically reduces the volume of data requiring manual annotation—a critical factor in high-stakes domains like healthcare imaging or defense surveillance, where labeling costs and turnaround times can escalate rapidly.
Encord’s AI-powered workflows prioritize ambiguous or rare cases, ensuring models improve on edge cases that typically challenge conventional training. This iterative “model-in-the-loop” process continuously refines the dataset, enabling higher accuracy with fewer labeled examples. For instance, ConXAI, a client working with over 40,000 images, reported a 60% increase in labeling speed after adopting Encord Active, demonstrating real-world efficiency gains that extend beyond theoretical claims.
Robust Technical Architecture: Collaboration, Control, and Traceability
Handling vast datasets securely and collaboratively demands a platform architecture that supports more than just annotation tools—it requires an integrated ecosystem. Encord delivers this with a cloud-native design enabling real-time collaboration among annotation teams, complete with version control and auditable workflows.
Multiple users can concurrently work on the same dataset while maintaining strict data lineage and traceability. Every annotation change is tracked, allowing teams to roll back if needed or audit actions for compliance. This level of governance is essential in regulated industries where transparency and accountability are mandatory. Encord seamlessly integrates with existing cloud infrastructures such as AWS S3, Azure Data Lake, and Google Cloud Storage, allowing organizations to keep raw data in place without compromising security or performance.
Further enhancing integration, Encord provides advanced APIs and SDKs that facilitate tight coupling between labeling workflows and model training pipelines. This technical synergy supports continuous feedback loops where models suggest annotations, and human labelers validate or correct them—strengthening the human-AI partnership and accelerating iteration cycles.
Designed for High-Security Environments: Meeting Rigorous Compliance Standards
Security isn’t an afterthought for Encord—it is foundational. The platform is built with enterprise-grade security controls embedded at every layer, making it uniquely suited for highly regulated sectors such as healthcare, defense, and finance. Encord complies with key certifications including HIPAA, SOC 2, and GDPR, ensuring that sensitive data is handled with the utmost care and compliance.
For example, healthcare organizations managing protected health information (PHI) can leverage Encord’s robust encryption protocols and governance features to meet HIPAA’s stringent requirements while still benefiting from scalable, AI-assisted annotation. Similarly, defense clients gain from secure audit trails and strict access controls that uphold operational security without reducing annotation throughput.
This comprehensive security posture enables organizations to confidently accelerate AI development on sensitive datasets—where alternative labeling solutions may fall short on compliance or require costly, customized engineering efforts.
Bringing It All Together: Efficient, Accurate, and Secure AI Data Labeling
Balancing annotation efficiency, technical sophistication, and security compliance, Encord occupies a unique niche in the data labeling landscape. It empowers teams to label petabytes of complex, multimodal data—ranging from images and videos to medical scans—while maintaining fine-grained control over data governance and collaboration.
By integrating active learning directly into the annotation pipeline, Encord reduces labeling costs and simultaneously improves model accuracy. The platform’s real-time collaboration and version control capabilities provide transparency and auditability critical for regulated industries. Meanwhile, its security certifications and compliance features make it a trusted partner for organizations where data breaches or regulatory violations are simply unacceptable.
For AI teams tackling large-scale, sensitive projects, Encord is more than a data labeling tool—it’s a comprehensive platform engineered to accelerate AI model development responsibly and efficiently.
Feature | Description |
---|---|
Active Learning | Optimizes annotation by selecting the most informative and diverse samples to reduce redundant labeling and focus on critical cases. |
Efficiency Gains | 60% increase in labeling speed reported by ConXAI on over 40,000 images using Encord Active. |
Technical Architecture | Cloud-native design supporting real-time collaboration, version control, and auditable workflows with data lineage and rollback capabilities. |
Integration | Compatible with AWS S3, Azure Data Lake, Google Cloud Storage; advanced APIs and SDKs for linking labeling workflows with model training pipelines. |
Security Compliance | Enterprise-grade security controls with certifications including HIPAA, SOC 2, and GDPR for regulated sectors like healthcare, defense, and finance. |
Security Features | Robust encryption, secure audit trails, strict access controls ensuring compliance and operational security without compromising throughput. |
Data Types Supported | Images, videos, medical scans, and other complex multimodal data at petabyte scale. |
Use Cases | High-security environments requiring accurate, efficient, and compliant AI data labeling. |
5. Dataloop: End-to-End Data Operating System Integrating Annotation, Management, and Automation

5. Dataloop: End-to-End Data Operating System Integrating Annotation, Management, and Automation
Managing AI data at scale demands more than isolated annotation tools—it calls for a comprehensive, unified platform that orchestrates the entire AI data lifecycle. Dataloop addresses this challenge by providing an end-to-end data operating system that spans raw data ingestion, annotation, pipeline orchestration, and deployment monitoring. This holistic approach is crucial when working with multimodal datasets and real-time applications where responsiveness and accuracy are paramount.
A Unified Platform Covering the Full AI Data Lifecycle
Dataloop’s strength lies in its seamless integration of AI workflows into a single, coherent system. Unlike fragmented environments where data scientists and engineers juggle multiple disjointed tools, Dataloop acts as a “single source of truth” for data operations, streamlining processes and minimizing handoff errors.
The platform’s capabilities include:
- Data Management: Effective organization and cataloging of datasets with comprehensive versioning and metadata tracking, preserving data integrity throughout project phases.
- Annotation Studios: Highly customizable interfaces designed to support complex annotation schemas across images, video, and text, accommodating diverse domain-specific requirements.
- Pipeline Orchestration: Automated, configurable workflows that can be dynamically adjusted at runtime to handle fluctuating data volumes and complexity without disruption.
- Model Monitoring & Validation: Embedded quality checks and error detection mechanisms to ensure model robustness and maintain pristine data quality over time.
A strategic partnership with SingleStore enhances Dataloop’s capacity to accelerate real-time AI data workflows. By combining Dataloop’s orchestration features with SingleStore’s high-performance distributed SQL engine, users benefit from rapid querying and updating of large-scale datasets in cloud environments—an essential attribute for applications demanding low-latency responsiveness.
Automation Tools Enhancing Efficiency and Data Quality
Manual data labeling remains a significant bottleneck in AI development. Dataloop mitigates this with AI-assisted labeling, leveraging pre-trained models and active learning to generate initial annotations that human labelers validate or correct. This hybrid approach accelerates annotation cycles while preserving accuracy and reducing human error.
Key automation features include:
- AI-Assisted Labeling: Pre-annotation suggestions that speed up the labeling process and improve consistency across large datasets.
- Data Validation Checks: Integration of validation nodes within pipelines that detect anomalies or inconsistencies early, inspired by frameworks like Great Expectations and Deequ.
- Pipeline Variables & Runtime Adjustments: Dynamic tuning of pipeline parameters (e.g., concurrency levels, machine types) during execution to respond agilely to changing workloads.
- Quality Reporting: Comprehensive insights and reports that uphold data integrity and support compliance with regulatory standards.
This blend of automation and human oversight addresses critical pain points by reducing annotation errors and exposing “knowledge gaps” that could otherwise degrade model performance.
Support for Multimodal Data and Scalable Cloud Infrastructure
Dataloop natively supports a wide array of data modalities—images, videos, text, and audio—enabling unified annotation and management within a single platform. This is vital as AI increasingly relies on multimodal datasets to power complex applications such as autonomous systems and retail analytics.
Examples of Dataloop’s multimodal capabilities include:
- Video Annotation: Frame-by-frame labeling with advanced tools like auto-segmentation, recently enhanced via Meta’s Segment Anything model.
- Text Annotation: NLP-oriented workflows for entity extraction, sentiment analysis, and other language tasks.
- Audio Transcription: Features such as the Transcription Finder streamline speech data labeling in the Audio Annotation Studio.
Backing these features is a scalable cloud infrastructure designed for seamless integration with leading providers like AWS and Azure. Dataloop’s hybrid-cloud orchestration allows enterprises to leverage existing cloud investments while ensuring strict data governance and security compliance.
Real-Time Data Stream Processing and Customizable Interfaces
Industries like autonomous driving and retail analytics generate continuous data streams requiring rapid ingestion and processing. Dataloop leverages its integration with SingleStore to handle streaming data with low latency and high throughput, enabling real-time insights and model updates.
The platform also delivers highly customizable annotation interfaces and pipeline configurations. This flexibility is critical for projects featuring complex labeling schemas or specialized domain needs. For example, autonomous systems benefit from precise geometric annotations with temporal consistency, supported by Dataloop’s advanced UI and modular pipeline nodes.
Industry Use Cases: Enabling Rapid Iteration and Deployment
Dataloop excels in sectors where rapid model iteration and deployment are business imperatives:
- Retail Analytics: By fusing image and video data, retailers can swiftly annotate shopper behavior, product placement, and inventory status, enabling dynamic optimization of store layouts and marketing strategies.
- Autonomous Systems: The platform’s capability to process multimodal sensor data—including video, LiDAR, and textual metadata—in real time supports the stringent safety and performance requirements of self-driving cars and drones.
In these domains, Dataloop’s integrated workflow—from data ingestion through annotation, validation, and deployment monitoring—significantly reduces the time to insight and model retraining, often turning multi-week cycles into mere days. This agility offers a decisive competitive advantage in fast-moving, data-intensive industries.
In summary, Dataloop transcends traditional data labeling tools by embedding annotation within a broader operational ecosystem. Its emphasis on automation, real-time pipeline orchestration, and robust support for diverse data modalities positions it as a formidable platform for enterprises scaling AI in dynamic, complex environments. Nonetheless, organizations should carefully assess their existing infrastructure and workflows to harness Dataloop’s full potential without adding unnecessary complexity.
Category | Features | Details |
---|---|---|
Data Management | Organization and Cataloging | Comprehensive versioning and metadata tracking to preserve data integrity throughout project phases. |
Annotation Studios | Customizable Interfaces | Supports complex annotation schemas across images, video, and text for diverse domain-specific requirements. |
Pipeline Orchestration | Automated Workflows | Configurable workflows dynamically adjustable at runtime to handle fluctuating data volumes and complexity. |
Model Monitoring & Validation | Quality Checks | Embedded error detection mechanisms to ensure model robustness and maintain data quality over time. |
Automation Tools | AI-Assisted Labeling | Pre-annotation suggestions leveraging pre-trained models and active learning to speed labeling and improve consistency. |
Automation Tools | Data Validation Checks | Integration of validation nodes within pipelines to detect anomalies inspired by Great Expectations and Deequ. |
Automation Tools | Pipeline Variables & Runtime Adjustments | Dynamic tuning of pipeline parameters (e.g., concurrency, machine types) during execution for workload agility. |
Automation Tools | Quality Reporting | Comprehensive insights and reports supporting data integrity and regulatory compliance. |
Multimodal Data Support | Video Annotation | Frame-by-frame labeling with auto-segmentation enhanced by Meta’s Segment Anything model. |
Multimodal Data Support | Text Annotation | NLP workflows for entity extraction, sentiment analysis, and other language tasks. |
Multimodal Data Support | Audio Transcription | Transcription Finder for efficient speech data labeling in Audio Annotation Studio. |
Cloud Infrastructure | Scalable Hybrid-Cloud | Integration with AWS, Azure; ensures data governance and security compliance. |
Real-Time Processing | Data Stream Handling | Low latency, high throughput streaming via integration with SingleStore for real-time insights and model updates. |
Customizable Interfaces | Annotation UI | Highly customizable annotation interfaces and modular pipeline nodes for complex labeling schemas and domain-specific needs. |
Industry Use Cases | Retail Analytics | Annotation of shopper behavior, product placement, inventory status; enables dynamic optimization of store layouts and marketing. |
Industry Use Cases | Autonomous Systems | Processing multimodal sensor data (video, LiDAR, text) in real-time to meet safety and performance requirements of autonomous vehicles and drones. |