The Hidden Dangers of AI and Proven Strategies to Mitigate Them
- Introduction: Navigating the Dual-Edged Sword of AI
- Introduction: Navigating the Dual-Edged Sword of AI
- The Transformative Promise: Progress and Pitfalls
- Cataloguing the Risks: From Present Dangers to Looming Threats
- Balancing Innovation with Ethical Responsibility and Risk Mitigation
- Looking Ahead: Navigating Uncertainty with Evidence and Prudence
- Prerequisites: Foundational Knowledge and Technical Context for Understanding AI Risks
- Prerequisites: Foundational Knowledge and Technical Context for Understanding AI Risks
- Core AI System Architectures and Data Pipelines
- Fundamentals of Model Training and Common AI Capabilities
- Key Terminology and Concepts Related to AI Risks
- Why This Foundation Matters
- Identifying and Categorizing AI Risks: A Taxonomy of Threats Across Domains
- Identifying and Categorizing AI Risks: A Taxonomy of Threats Across Domains
- Privacy and Data Security Threats
- Bias, Discrimination, and Operational Vulnerabilities
- Adversarial Attacks and Misinformation via Deepfakes
- Legal and Ethical Liabilities
- Societal Impacts: Workforce Displacement and Economic Shifts
- Step-by-Step Risk Mitigation Strategies: Designing and Implementing Effective Safeguards
- Step-by-Step Risk Mitigation Strategies: Designing and Implementing Effective Safeguards
- Developing Internal AI Use Policies and Guardrails Tailored to Organizational Contexts
- Implementing AI Governance Frameworks: Defining Roles, Responsibilities, and Accountability Measures
- Leveraging Transparency, Explainability, Continuous Monitoring, Auditing, Training Programs, and Incident Response
- Key Takeaways
- Technical Deep Dive: How Risk Mitigation Techniques Work and Why They Matter
- Technical Deep Dive: How Risk Mitigation Techniques Work and Why They Matter
- Explainable AI: From Black Boxes to Glass Boxes
- Data Validation and Sanitization: The Frontline Against Bias and Poisoning
- Model Robustness: Fortifying AI Against Adversarial Attacks
- Watermarking: Tracing Ownership and Detecting Misuse
- Why These Techniques Matter
- Troubleshooting Common AI Risk Management Challenges
- Troubleshooting Common AI Risk Management Challenges
- The Opaque Black-Box Problem: Why Transparency Matters—and How to Achieve It
- Navigating the Shifting Sands: AI Regulation Amidst the Innovation Race
- Attribution and Accountability: Clarifying Responsibility When AI Causes Harm
- Human Oversight: Beyond Just a “Human in the Loop”
- Actionable Strategies: Building Adaptive and Collaborative Risk Frameworks
- Advanced Risk Management and Future-Proofing: Optimizing AI Safety in an Evolving Landscape
- Advanced Risk Management and Future-Proofing: Optimizing AI Safety in an Evolving Landscape
- Leveraging Emerging AI Risk Management Frameworks
- Incorporating Adversarial AI Threat Intelligence and Automation
- Preparing for Regulatory Compliance and Ethical AI Evolution
- Benchmarking and Continuous Improvement: The Road Ahead
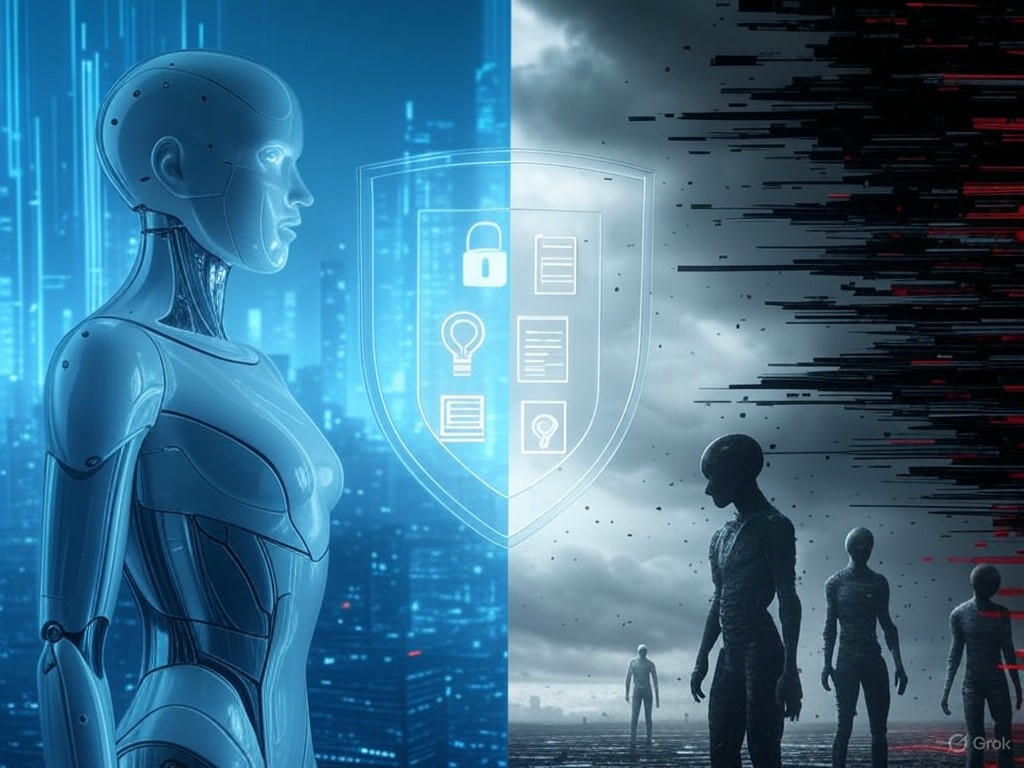
Introduction: Navigating the Dual-Edged Sword of AI
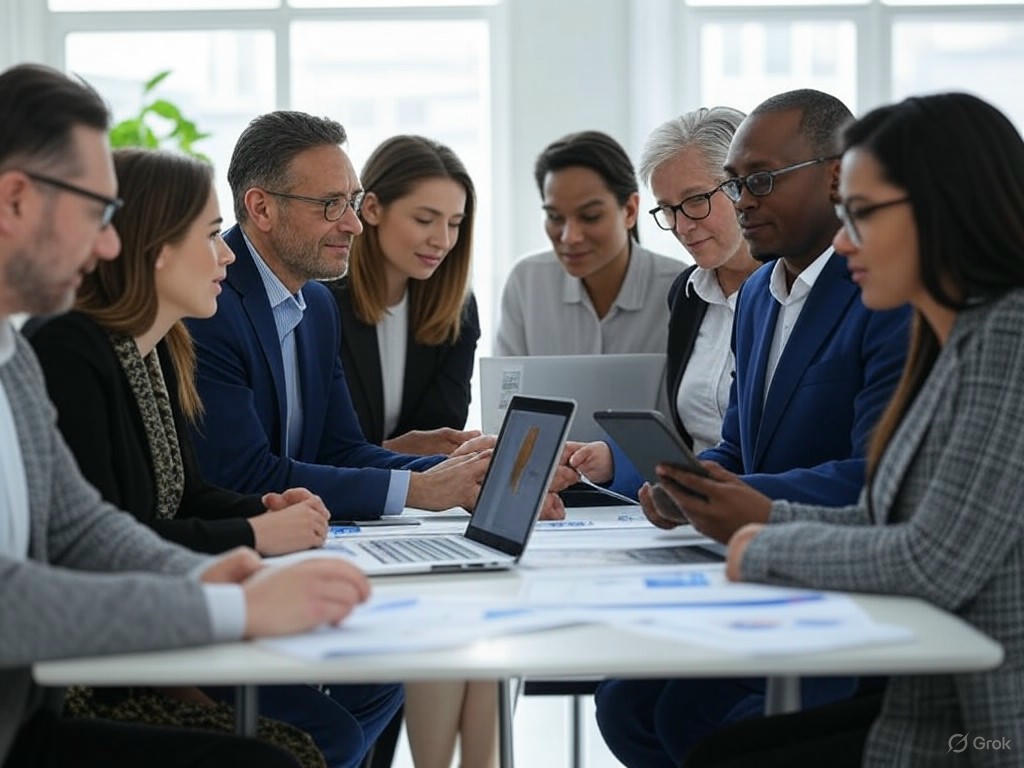
Introduction: Navigating the Dual-Edged Sword of AI
Artificial intelligence (AI) has transcended its science fiction roots to become a transformative force reshaping industries and societies at an unprecedented pace. According to the 2025 AI Index Report by Stanford HAI, global private investment in AI reached $109 billion in 2024, with generative AI alone attracting nearly $34 billion. This surge reflects tangible transformation—from AI-powered agents streamlining complex workflows to breakthroughs in biomolecular science enabled by faster, more efficient models.
Yet, alongside this rapid progress lies a sobering truth: AI is a dual-edged sword. Its vast potential is accompanied by significant risks that are often underestimated or misunderstood. For instance, the International Monetary Fund (IMF) estimates that about 60% of jobs in advanced economies may be affected by AI, raising pressing concerns about workforce displacement, economic inequality, and social disruption. In healthcare, AI tools enhance efficiency but also introduce patient safety risks that demand vigilant oversight.
The Transformative Promise: Progress and Pitfalls
What makes AI so transformative? At its core, AI excels at detecting patterns in massive datasets, unlocking new efficiencies and capabilities across sectors. Innovations like Microsoft’s AI-powered Copilot simplify everyday tasks, while Google DeepMind’s Mariner web-browsing agent autonomously solves complex problems. McKinsey reports that nearly every company invests in AI, yet only 1% consider their adoption mature, highlighting vast untapped potential.
However, AI’s pattern recognition tendencies also mirror societal biases and imperfections. As writer Tom Parish observes, AI “reflects us back to ourselves, for better or worse,” compelling us to decide which patterns to perpetuate and which require human judgment. This duality manifests in concrete risks such as misinformation, biased decision-making, and ethical dilemmas around agency and accountability.
Cataloguing the Risks: From Present Dangers to Looming Threats
While speculative fears around AI sentience persist, political scientists at the University of Zurich stress that current, concrete risks demand urgent attention. Key threats include:
- Job displacement and economic inequality: Automation risks exacerbating social divides, disproportionately impacting vulnerable workers (Built In).
- Social manipulation and misinformation: AI-generated content can spread false information and manipulate public opinion, complicating democratic discourse.
- Healthcare safety hazards: ECRI’s 2025 report flags AI as a top health technology hazard, emphasizing patient safety concerns if tools are inadequately tested or monitored.
- Security vulnerabilities: Generative AI models face adversarial attacks, data poisoning, and impersonation risks that threaten confidentiality and trust (Deloitte Insights).
- Ethical challenges with synthetic data: The use of artificial datasets raises questions around scientific integrity and societal impact (NIEHS).
These risks are complex and intertwined, requiring nuanced understanding rather than alarmist rhetoric. For example, the rise of AI-driven financial fraud through deepfakes calls for specialized security responses alongside robust governance frameworks.
Balancing Innovation with Ethical Responsibility and Risk Mitigation
How can we harness AI’s transformative power while mitigating its risks? The answer lies in balancing bold innovation with rigorous ethical responsibility and practical risk management.
Governments and organizations are actively developing such frameworks. The National Institute of Standards and Technology (NIST) AI Risk Management Framework (AI RMF), currently in draft, offers voluntary guidelines to systematically identify, assess, and mitigate AI risks. UNESCO’s 2021 global AI ethics recommendation emphasizes human rights, transparency, accountability, and privacy as foundational pillars.
Corporate leaders like Google and Microsoft promote “responsible AI” principles prioritizing collaboration, fairness, and inclusivity. Security-focused firms advocate a “secure-by-design” approach, embedding safeguards such as access controls, inference monitoring, and human-in-the-loop oversight to prevent unauthorized use and adversarial exploits.
Effective risk mitigation in practice includes:
- Explainable AI (XAI) techniques to improve transparency and trust.
- Fairness-aware algorithms and comprehensive data audits to reduce bias.
- Upskilling and talent development programs to close the AI expertise gap.
- Multi-layered cybersecurity investments addressing evolving threats.
- Ethical governance structures ensuring accountability at all organizational levels.
This balance is not a one-time checklist but a continuous, adaptive journey demanding collaboration across technical, legal, and societal domains.
Looking Ahead: Navigating Uncertainty with Evidence and Prudence
With over 15 years of experience architecting AI systems, I recognize both the exhilaration of breakthroughs and the gravity of their responsibility. The path forward is neither blindly optimistic nor frozen by fear. It requires a clear-eyed, evidence-based approach that acknowledges uncertainties while committing to ethical stewardship.
This article aims to distill the hype and provide a grounded understanding of AI’s potential dangers and pragmatic mitigation strategies. Whether you are a developer, policymaker, business leader, or concerned citizen, appreciating AI’s dual-edged nature is essential. The choices we make today will shape how this powerful technology serves humanity—not the other way around.
Category | Details | Source/Example |
---|---|---|
Job Displacement & Economic Inequality | Automation risks exacerbating social divides, disproportionately impacting vulnerable workers. | IMF; Built In |
Social Manipulation & Misinformation | AI-generated content spreading false information and manipulating public opinion. | Not specified |
Healthcare Safety Hazards | Patient safety concerns if AI tools are inadequately tested or monitored. | ECRI 2025 Report |
Security Vulnerabilities | Adversarial attacks, data poisoning, impersonation risks threatening confidentiality and trust. | Deloitte Insights |
Ethical Challenges with Synthetic Data | Questions about scientific integrity and societal impact from artificial datasets. | NIEHS |
Risk Mitigation Strategies | Explainable AI, fairness-aware algorithms, upskilling, multi-layered cybersecurity, ethical governance. | NIST AI RMF; UNESCO; Corporate Leaders (Google, Microsoft) |
Prerequisites: Foundational Knowledge and Technical Context for Understanding AI Risks
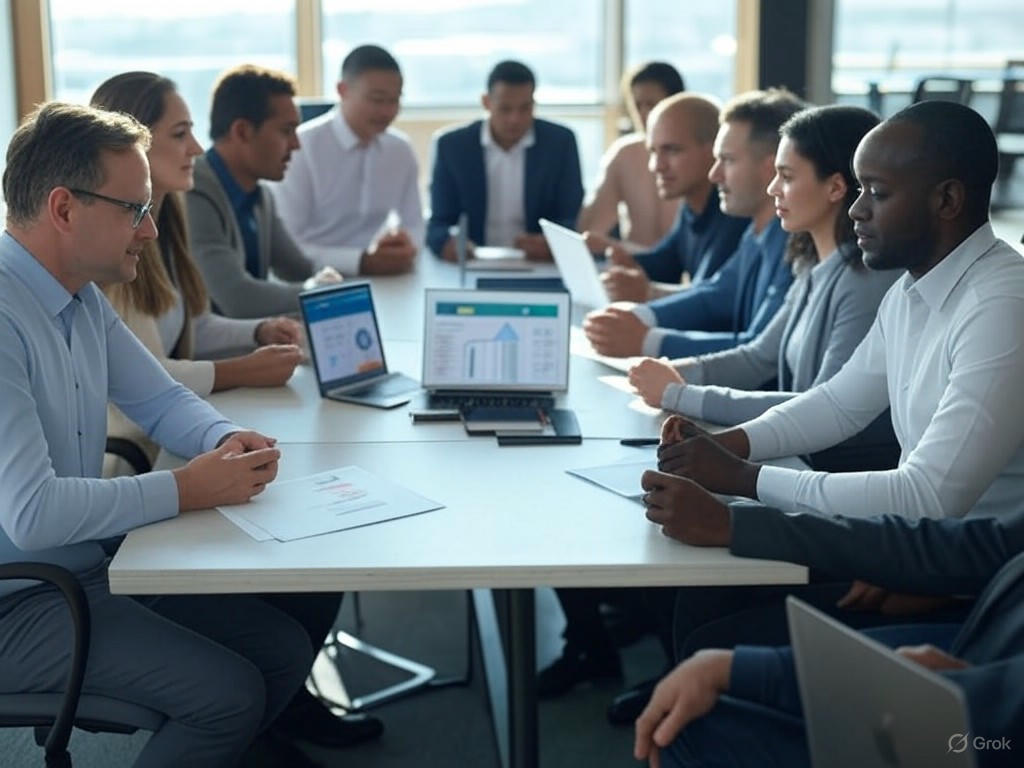
Prerequisites: Foundational Knowledge and Technical Context for Understanding AI Risks
To fully comprehend the risks associated with artificial intelligence, it is essential to first understand the technical foundations that underpin these systems. AI is not a mystical force—it is constructed from sophisticated architectures, extensive data pipelines, and complex training methodologies. Without this grounding, discussions about AI risks and mitigation strategies risk becoming abstract, misleading, or alarmist.
Core AI System Architectures and Data Pipelines
Think of AI systems as a complex urban infrastructure. At their core is the architecture—the blueprint that governs how components communicate and function together. Modern AI architectures, particularly those driving large language models (LLMs) and generative AI, are intricate and modular rather than monolithic. They function like an advanced traffic control center, coordinating components such as retrieval systems, safety filters, and explainability dashboards that monitor and regulate decision-making processes.
Supporting this architecture are data pipelines, which serve as the lifeblood of AI systems. These pipelines transport raw, richly contextualized data from diverse sources to AI models, ensuring high fidelity and granularity. They capture everything from user interactions and device metadata to precise timestamps, avoiding premature aggregation or loss of detail. This design enables dynamic reprocessing, which is critical for both batch and real-time AI applications.
For example, autonomous vehicles depend on data pipelines that ingest massive volumes of LIDAR, camera, and GPS data to feed machine learning models operating in real time. Similarly, AI-driven architectural design tools leverage generative AI models powered by data pipelines integrating natural language processing and computer vision to produce a wide array of design options.
Key design principles for AI systems emphasize agility and robustness. Systems must adapt swiftly to new data and emerging threats while maintaining data quality, security, and consistency throughout pipelines. Traditional data management methods often fall short, as they do not preserve the full fidelity and contextual richness needed for AI, which is why modern AI-ready pipelines prioritize flexibility and detailed event recording.
Fundamentals of Model Training and Common AI Capabilities
Training an AI model is comparable to teaching a child to recognize patterns and make informed decisions—but at an immense scale and speed. The process begins with careful data collection and preparation. While data quantity is important, quality is paramount; clean, relevant, and representative datasets yield better models.
Preprocessing transforms messy, real-world data—often containing errors, noise, or missing values—into structured formats suitable for training. Techniques such as data augmentation artificially expand datasets, while data versioning tracks dataset changes over time, enhancing reproducibility and compliance.
Machine learning algorithms, the engines powering AI, fall into several broad categories:
- Supervised learning: Models learn from labeled data to predict specific outcomes.
- Unsupervised learning: Models identify patterns and structures without explicit labels.
- Reinforcement learning: Models learn optimal strategies through trial and error within defined environments.
Among the most influential algorithms today are gradient boosting methods, k-means clustering, support vector machines (SVMs), and neural networks. These underpin diverse applications, from medical diagnostics to fraud detection.
Generative AI, a rapidly advancing subset of machine learning, has gained significant prominence. Models such as Generative Adversarial Networks (GANs) and Variational Autoencoders (VAEs) learn to create novel content by studying existing data—synthesizing images from text or composing music, for instance. By 2025, generative AI is expected to accelerate breakthroughs in drug discovery and materials science, affecting hundreds of millions of jobs globally.
Training sophistication continues to evolve. For example, large language models increasingly employ techniques like Retrieval Augmented Generation (RAG) to dynamically incorporate external knowledge during inference. However, these methods have limitations and must be integrated thoughtfully within broader data architecture strategies.
Key Terminology and Concepts Related to AI Risks
To engage meaningfully with AI risks, familiarity with several technical terms is essential. Here are four critical concepts clarified:
-
Bias: AI bias occurs when models produce systematically unfair or prejudiced outcomes due to skewed training data, flawed algorithms, or misaligned objectives. For instance, healthcare AI might underdiagnose women because training datasets are disproportionately male. Bias is not merely a technical flaw; it can lead to legal liabilities, reputational damage, and exacerbated social inequalities. Mitigation strategies include curating diverse datasets, implementing rigorous governance frameworks, and maintaining continuous human oversight.
-
Explainability (XAI): As AI models grow in complexity, their decision-making processes often become opaque, earning the label “black boxes.” Explainable AI seeks to illuminate these processes using methods such as Shapley values, LIME, and counterfactual explanations to reveal why a model made a given decision. Transparency fosters trust, enables early detection of biases or errors, and is indispensable in high-stakes domains like finance and healthcare.
-
Adversarial Attacks: These are intentional attempts to deceive AI models by subtly manipulating inputs to cause misclassification or erroneous outputs. An example is placing small stickers on a stop sign to trick an autonomous vehicle’s vision system into misreading it as a speed limit sign. Adversarial attacks pose significant threats to the safety and reliability of AI, especially in mission-critical contexts such as public safety and security.
-
Operational Drift: Over time, AI models may degrade in performance as the data environment evolves—a phenomenon known as drift. For example, a recommendation system trained on last year’s shopping trends may become ineffective if consumer behavior shifts. Detecting and managing drift through ongoing monitoring and retraining are vital to maintaining accuracy and avoiding unexpected risks.
Why This Foundation Matters
Without a firm understanding of AI architectures, training processes, and risk-related terminology, conversations about AI risks can easily become speculative or alarmist. The reality is nuanced: AI systems are powerful but their behavior hinges on design choices, data integrity, and ongoing oversight.
Grasping these technical foundations equips readers to appreciate why certain risks arise and what practical strategies exist to mitigate them. This knowledge also fosters a balanced perspective on the ethical and societal implications of AI—grounded in evidence rather than hype or fear.
As the article progresses, this groundwork will enable you to critically evaluate AI’s potential dangers and explore pathways toward safer, more responsible AI deployment.
Term/Concept | Description | Examples | Mitigation Strategies |
---|---|---|---|
Bias | Systematic unfair or prejudiced outcomes due to skewed data, flawed algorithms, or misaligned objectives. | Healthcare AI underdiagnosing women due to male-skewed datasets. | Diverse datasets, rigorous governance, continuous human oversight. |
Explainability (XAI) | Techniques to make AI decision processes transparent and understandable. | Using Shapley values, LIME, counterfactual explanations to explain model decisions. | Implement explainability methods to foster trust and detect errors early. |
Adversarial Attacks | Intentional input manipulations to deceive AI models causing incorrect outputs. | Stickers on stop signs tricking autonomous vehicles into misreading signs. | Robust model training, input validation, security measures. |
Operational Drift | Performance degradation of AI models over time due to changing data environments. | Recommendation systems becoming ineffective as consumer behavior changes. | Ongoing monitoring, retraining models regularly. |
Identifying and Categorizing AI Risks: A Taxonomy of Threats Across Domains
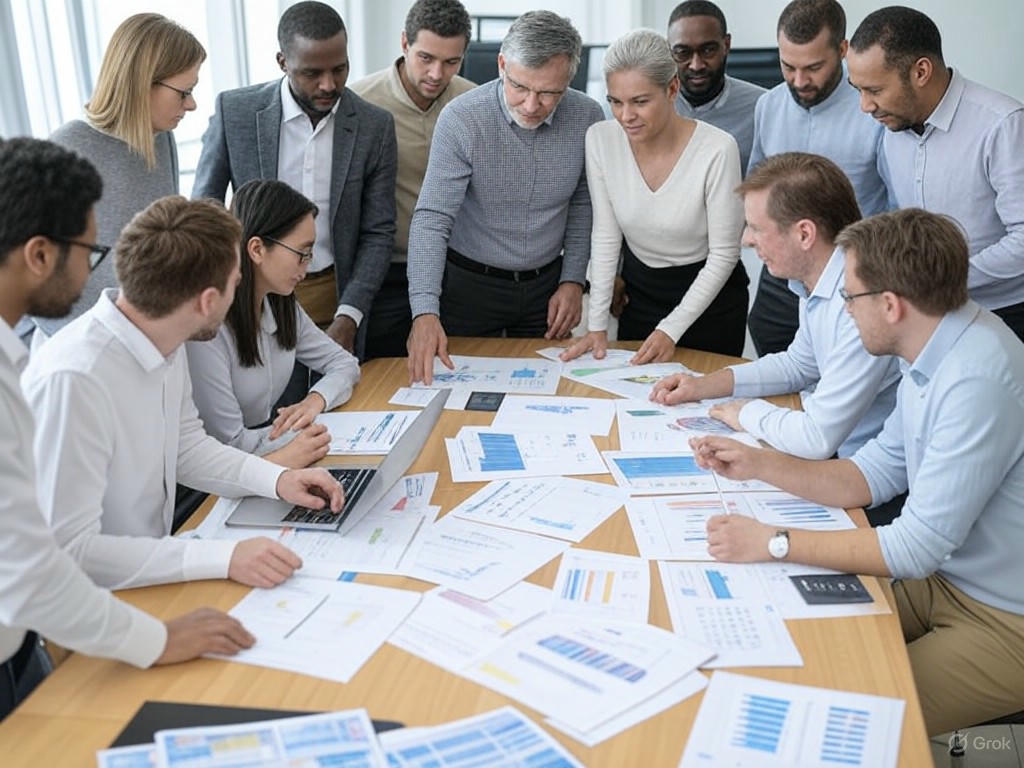
Identifying and Categorizing AI Risks: A Taxonomy of Threats Across Domains
Artificial Intelligence presents a broad spectrum of risks that vary across the many domains it touches. To effectively navigate this complex landscape, it is essential to categorize these risks clearly. Each category represents a distinct vector of vulnerability or harm—often interconnected yet requiring tailored mitigation strategies. Below, we unpack these categories with real-world examples and insights drawn from recent developments.
Privacy and Data Security Threats
AI systems depend heavily on data, much of which is sensitive, personal, or proprietary. This reliance creates fertile ground for privacy and security vulnerabilities. According to IBM’s 2024 Cost of a Data Breach Report, the global average cost of a data breach now stands at $4.88 million. Beyond financial losses, breaches erode consumer trust—75% of consumers are reluctant to engage with organizations that mishandle their data.
Privacy risks stemming from AI are twofold:
-
System Vulnerabilities: AI models themselves can be targets of attacks that expose or misuse data. Prompt injection and data poisoning attacks have surged, especially in sensitive sectors like financial services and healthcare.
-
Generative AI Challenges: The rise of generative AI tools raises new questions about data collection, anonymization, and ethical use. Embedding “privacy-by-design” principles—such as encryption and anonymization—into AI workflows from the outset is critical.
Regulatory frameworks add complexity. The European Union’s GDPR remains the benchmark for data privacy, while the U.S. landscape is fragmented, with state laws like California’s CCPA leading the way. Organizations must therefore build flexible governance frameworks that adapt to evolving global regulations and embed AI accountability as a core organizational practice.
The UK’s National Cyber Security Centre (NCSC) has issued warnings about rising AI-driven vulnerabilities targeting critical infrastructure, highlighting the high stakes of lax security. As AI adoption accelerates faster than security controls, continuous auditing and updating of AI systems become indispensable defenses.
Bias, Discrimination, and Operational Vulnerabilities
AI models learn from human-generated data, inevitably inheriting human biases that can perpetuate discrimination. For example, gender bias in AI hiring tools has resulted in systemic disadvantages for deaf and non-white applicants, as seen in complaints against companies like Intuit and HireVue. These biases have tangible, real-world consequences on fairness and opportunity.
Bias manifests in various forms, including:
-
Gender and Racial Bias: Embedded stereotypes in training data reinforce societal inequities.
-
Ableism: AI systems often fail to accommodate disabilities or favor able-bodied norms.
-
Ageism: For instance, health apps skewed toward male or younger symptom profiles may underdiagnose others.
Operational vulnerabilities such as model or data drift exacerbate these issues. Drift occurs when AI models degrade over time as input data or user behavior shifts. A language model trained on data from five years ago might misinterpret contemporary slang or cultural references, leading to inaccuracies or unfair outcomes. If unchecked, drift can amplify existing biases.
Data poisoning attacks pose another threat by injecting malicious or toxic data into training sets, degrading model accuracy by up to 27% in image recognition tasks. Such attacks have been linked to disruptions in government and commercial sectors.
Mitigating these risks requires rigorous model monitoring, frequent retraining, and the use of diverse, representative datasets. Cultivating an inclusive AI community and adopting data-centric development practices are essential to detect and correct biases before deployment.
Adversarial Attacks and Misinformation via Deepfakes
Adversarial attacks involve sophisticated manipulations where attackers craft inputs to deceive AI models into producing incorrect or harmful outputs. The National Institute of Standards and Technology (NIST) has published guidelines emphasizing the evolving taxonomy of such attacks and the necessity of layered defenses.
Common adversarial techniques include:
-
Manipulating input data to fool AI systems.
-
Exploiting vulnerabilities in pre-trained models or AI libraries.
-
Leveraging AI itself to generate deceptive content, such as deepfakes.
Deepfakes represent a particularly insidious form of misinformation. While early fears anticipated election-disrupting videos, recent events—such as the 2024 elections—show a mixed picture: much deepfake content was low quality and ineffective at swaying public opinion. However, as deepfake technology improves, it poses growing risks in social engineering attacks, fraud, and political manipulation.
For example, deepfake scams have already caused hundreds of millions in global financial losses. Geopolitically, the Chinese Communist Party’s use of deepfakes for information warfare against Taiwan exemplifies the weaponization of synthetic media.
Combating these threats involves a combination of technical solutions—such as detection algorithms and authentication protocols—and social strategies like user education and establishing verification “secrets” within trusted communities.
Legal and Ethical Liabilities
AI’s integration into sensitive domains introduces complex legal and ethical challenges. Incidents such as Tesla Autopilot’s involvement in accidents or IBM Watson Health’s delivery of suboptimal medical outcomes spotlight issues of accountability and transparency.
Legal frameworks are struggling to keep pace. Pioneering legislation like Colorado’s Artificial Intelligence Act seeks to regulate “high-risk” AI applications, but comprehensive, globally harmonized rules remain in progress.
Ethical dilemmas extend beyond mere compliance. AI systems may inadvertently infringe on privacy rights, propagate misinformation, or make decisions without adequate human oversight. Intellectual property rights around AI-generated content and the environmental impact of energy-intensive AI training also raise concerns.
Addressing these issues demands cross-disciplinary collaboration among technologists, ethicists, policymakers, and affected communities. Baylor University’s Center on Responsible Artificial Intelligence and Governance exemplifies efforts to embed moral considerations into AI development, ensuring societal values guide technological progress.
Societal Impacts: Workforce Displacement and Economic Shifts
AI’s promise of enhanced efficiency often triggers anxiety about job displacement. Surveys indicate that 30% of workers fear their roles will be replaced by AI within three years, and by 2030, about 14% are expected to change careers due to automation. Sectors like content creation, customer service, and routine office work face particularly high exposure.
However, the narrative is not solely one of displacement. McKinsey research reveals that although nearly all companies invest in AI, only a small fraction consider themselves mature adopters, indicating a transitional phase. Concurrently, new roles—especially in AI and machine learning—are emerging rapidly.
The critical challenge is reskilling. Many workers express concern about inadequate training to adapt to AI-augmented workplaces. While some companies plan significant investments in retraining programs, execution remains uneven.
Ultimately, AI’s societal impact is a balancing act: harnessing innovation to enhance productivity and quality of life while mitigating disruptions and ensuring equitable access to new opportunities.
AI risks are multifaceted and dynamic. From privacy breaches and biased algorithms to adversarial manipulations and workforce upheavals, each domain demands vigilance, accountability, and adaptive governance. As we push the boundaries of AI’s capabilities, understanding and categorizing these risks is the essential first step toward building systems that are not only powerful but also trustworthy and just.
Risk Category | Description | Examples & Insights | Mitigation Strategies |
---|---|---|---|
Privacy and Data Security Threats | Risks related to sensitive, personal, or proprietary data used by AI systems, including system vulnerabilities and generative AI challenges. | IBM’s 2024 Cost of a Data Breach Report: average breach cost $4.88M; Prompt injection and data poisoning attacks; UK NCSC warnings on AI-driven vulnerabilities. | Embed privacy-by-design (encryption, anonymization); flexible governance frameworks; continuous auditing and updating of AI systems; compliance with regulations (GDPR, CCPA). |
Bias, Discrimination, and Operational Vulnerabilities | AI inherits human biases causing discrimination and fairness issues; operational risks like model/data drift and data poisoning. | Gender bias in hiring tools disadvantaging deaf and non-white applicants; 27% accuracy degradation from poisoning in image recognition; model drift affecting language models. | Rigorous model monitoring; frequent retraining; diverse and representative datasets; inclusive AI community; data-centric development practices. |
Adversarial Attacks and Misinformation via Deepfakes | Manipulative attacks deceiving AI outputs and spreading misinformation, especially using deepfake technology. | NIST guidelines on adversarial attacks; 2024 election deepfake impacts; hundreds of millions lost to deepfake scams; Chinese CCP’s deepfake info warfare against Taiwan. | Detection algorithms; authentication protocols; user education; verification secrets within trusted communities. |
Legal and Ethical Liabilities | Challenges in accountability, transparency, and ethics in AI deployment, with evolving legal frameworks. | Tesla Autopilot accidents; IBM Watson Health suboptimal outcomes; Colorado’s Artificial Intelligence Act; concerns on IP rights and environmental impact. | Cross-disciplinary collaboration; embedding ethical principles; advancing comprehensive regulation; ensuring human oversight and transparency. |
Societal Impacts: Workforce Displacement and Economic Shifts | Job displacement fears and economic changes due to AI automation and adoption. | 30% workers fear job loss within 3 years; 14% career changes by 2030; McKinsey research on AI adoption maturity; emerging AI/ML roles. | Reskilling and training programs; investment in workforce development; balancing innovation with equitable opportunity access. |
Step-by-Step Risk Mitigation Strategies: Designing and Implementing Effective Safeguards
Step-by-Step Risk Mitigation Strategies: Designing and Implementing Effective Safeguards
How can organizations transition from AI enthusiasm to disciplined, responsible deployment? The answer lies in a systematic approach to risk mitigation—one that integrates policy, governance, transparency, and continuous vigilance. AI’s transformative potential is accompanied by nuanced risks that demand layered safeguards tailored to each organization’s unique context. Below is a practical roadmap grounded in current insights for 2025.
Developing Internal AI Use Policies and Guardrails Tailored to Organizational Contexts
With approximately 80% of organizations now adopting AI, only about 1% consider their deployment mature (McKinsey, 2025). This maturity gap often stems from underdeveloped policies.
A comprehensive internal AI use policy is foundational. It should clearly define the purpose and scope—clarifying why AI is used, which systems it covers, and who holds responsibility. For example, LexisNexis recommends establishing dedicated oversight functions that:
- Review and approve AI tool adoption,
- Monitor evolving laws and regulations,
- Manage internal inquiries and requests for new AI capabilities.
This tripartite structure establishes a continuous feedback loop, ensuring compliance and adaptability in a rapidly evolving landscape.
Policies must also specify acceptable and prohibited AI uses, addressing ethical principles such as fairness, bias mitigation, and data privacy. Harris Beach highlights the importance of explicitly restricting AI applications that may infringe on intellectual property or personal data rights.
Guardrails complement policies by providing concrete technical and procedural controls embedded within AI workflows to prevent misuse or errors. As Matt Blumberg of Acrolinx warns, “Without solid governance, generative AI’s potential can just as easily become a source of risk.” Typical guardrails include:
- Restricting AI access to sensitive data,
- Enforcing quality standards on training datasets,
- Automating output reviews to ensure compliance.
These guardrails should be customized to the organization’s industry risk profile. For instance, financial institutions face heightened regulatory scrutiny requiring stricter controls.
Importantly, internal AI use policies are living documents. They must evolve alongside technological advances and regulatory changes, incorporating lessons learned and emerging best practices.
Implementing AI Governance Frameworks: Defining Roles, Responsibilities, and Accountability Measures
Governance acts as the connective tissue that integrates policies and practices into a coherent, accountable system. Oliver Patel’s 2025 AI Governance predictions emphasize that AI literacy will become a strategic organizational imperative. Consequently, governance frameworks must invest in education and culture-building alongside formal structures.
A hallmark of effective governance is clear role definition. ISACA’s 2025 journal advocates for AI steering committees comprising diverse stakeholders—from legal and compliance to IT and business units. This committee ensures ethical alignment, monitors risk, and adapts to regulatory developments such as the EU AI Act, effective since August 2024.
Accountability cannot be outsourced merely because AI systems operate autonomously. Forbes’ recounting of the Air Canada chatbot incident illustrates the consequences of weak accountability. Governance frameworks should incorporate:
- Clearly assigned ownership of AI policies, often to senior executives like Chief AI Officers or Chief Data Officers,
- Defined escalation paths for AI-related risk incidents,
- Regular reporting and auditing mechanisms.
Governance is not one-size-fits-all. Splunk’s 2025 AI Governance guide stresses adapting frameworks to organizational priorities and risk tolerances. For example, human oversight models vary by application risk:
- Human-in-the-loop for high-stakes decisions requiring direct intervention,
- Human-on-the-loop for supervisory monitoring,
- Human-out-of-the-loop for low-risk, automated scenarios.
Building a culture of responsibility is equally vital. OneAdvanced demonstrates the value of training programs embedding principles of fairness, accuracy, and accountability across all employee levels. This cultural foundation empowers teams to innovate responsibly.
Leveraging Transparency, Explainability, Continuous Monitoring, Auditing, Training Programs, and Incident Response
Transparency and explainability are essential—not mere buzzwords but critical enablers of trust and regulatory compliance. Explainable AI (XAI) tools, such as Shapley values and LIME, illuminate complex model decisions by providing local and global explanations. This transparency aids in detecting bias or errors and reassures stakeholders. For example, clinicians rely on XAI to understand why a model flagged a patient as high risk, enhancing trust in AI-driven recommendations.
As regulatory oversight intensifies, transparency becomes a competitive advantage. The AlgoAnalytics 2025 report highlights how businesses using AI in hiring or fraud detection build trust by publishing model operation details. Such openness reduces skepticism and supports fairness.
However, transparency alone is insufficient. Continuous monitoring and auditing offer real-time and retrospective insights to ensure AI systems function as intended. Lumenalta’s updated AI audit checklists advocate automated testing to reduce manual workload and prioritize high-risk systems. This proactive auditing uncovers anomalies before they escalate into incidents.
Effective monitoring integrates cybersecurity best practices. The SANS Institute recommends a risk-based approach that emphasizes access controls, data protection, and inference monitoring to defend against adversarial attacks and data manipulation.
Training programs constitute the human defense layer. Cybersecurity training enhanced by AI-driven personalization and immersive technologies such as VR prepares teams to recognize and respond swiftly to AI-related risks. Platforms like Disco and Cybrary offer adaptive, gamified learning experiences that boost engagement and competency.
Finally, AI-specific incident response plans are critical. PurpleSec’s 2025 best practices emphasize preparation, clear communication protocols, and automation to prevent chaos during breaches. While automation accelerates response, human judgment remains indispensable for interpreting AI-driven alerts and coordinating actions.
Key Takeaways
- Establish clear, context-specific AI use policies that define guardrails and assign dedicated oversight functions.
- Implement robust governance frameworks with well-defined roles, accountability mechanisms, and continuous education to foster a responsible AI culture.
- Invest in transparency and explainability tools to build stakeholder trust and comply with evolving regulations.
- Adopt continuous monitoring and auditing processes to detect risks early and maintain AI system integrity.
- Empower your workforce through tailored training and develop comprehensive incident response plans to mitigate AI-driven incidents effectively.
AI risk mitigation is an evolving discipline requiring sustained attention, adaptability, and a balanced blend of technical safeguards and human-centric measures. Organizations that approach these steps thoughtfully will not only protect themselves but also unlock AI’s full potential responsibly.
Strategy | Key Actions | Responsible Parties | Tools/Examples | Notes |
---|---|---|---|---|
Developing Internal AI Use Policies and Guardrails |
|
AI governance teams, Legal, Compliance |
|
Tailor guardrails to industry-specific risks (e.g., finance) |
Implementing AI Governance Frameworks |
|
Senior executives (Chief AI Officer, Chief Data Officer), Steering committees |
|
Adapt governance to organizational risk tolerance and priorities |
Leveraging Transparency, Explainability, Monitoring, Training, and Incident Response |
|
AI teams, Cybersecurity, Training departments, Incident response teams |
|
Balance automation with human judgment in incident handling |
Technical Deep Dive: How Risk Mitigation Techniques Work and Why They Matter
Technical Deep Dive: How Risk Mitigation Techniques Work and Why They Matter
What happens inside AI systems that builds our trust—or sets off alarm bells? As AI increasingly influences critical domains such as healthcare, finance, and justice, understanding the technical safeguards designed to mitigate risks is essential. Let’s peel back the layers on the core methods researchers and practitioners use to keep AI systems reliable, fair, and secure.
Explainable AI: From Black Boxes to Glass Boxes
Traditional AI models often operate as opaque black boxes, leaving users and developers in the dark about how decisions are made. Explainable AI (XAI) transforms these into glass boxes, providing transparency that is foundational to trust and accountability.
XAI techniques offer interpretable insights into model behavior, revealing which features influenced a decision or why one outcome was selected over another. For instance, in clinical AI applications, explaining why a model flagged a patient as high risk helps doctors verify recommendations instead of accepting them blindly. A 2024 systematic review found that over half of clinician studies reported increased trust when explanations accompanied AI outputs. However, striking the right balance between oversimplification and overwhelming detail remains a challenge.
Tools like IBM’s AI Explainability 360 provide a suite of algorithms that demystify complex models. Meanwhile, large language models (LLMs) increasingly use “chain-of-thought” prompting to break down reasoning step-by-step, enhancing transparency for end users.
Yet, caution is warranted. Interpretability methods can be misapplied or misunderstood, leading to misplaced trust or skepticism. Human-centered XAI initiatives emphasize educating users and fostering AI literacy so explanations truly empower rather than confuse.
In essence, XAI converts AI from inscrutable oracles into accountable partners, enabling developers to detect biases early and users to make informed decisions grounded in clarity.
Data Validation and Sanitization: The Frontline Against Bias and Poisoning
AI models are only as reliable as the data they consume. Biased, incomplete, or maliciously poisoned training data can cause AI to perpetuate or amplify harmful outcomes. Rigorous data validation and sanitization form the first and most critical line of defense.
Bias manifests in many ways: gender imbalances favoring one sex, age bias disadvantaging older populations, or ableism overlooking disability considerations. For example, some health apps default to male symptom profiles, risking misdiagnoses for women. These biases often arise from skewed datasets reflecting societal prejudices.
Mitigation begins upstream with techniques such as:
- Diverse and representative data collection encompassing broad demographics and scenarios.
- Automated bias detection tools scanning datasets for imbalances or anomalies.
- Data cleansing pipelines removing corrupted or adversarial inputs designed to poison models and induce erratic behaviors.
- Ongoing audits monitoring datasets and models post-deployment, as bias can creep in over time.
Industry leaders champion a data-centric approach that continuously refines data quality and diversity to ensure fairness. This is an evolving commitment, especially as models retrain on fresh data and AI systems scale to new applications.
Model Robustness: Fortifying AI Against Adversarial Attacks
Imagine an attacker subtly altering input data to trick an AI into misclassifying an image or generating harmful outputs. These adversarial attacks expose vulnerabilities in AI’s pattern recognition, threatening safety and reliability.
Researchers employ model robustness strategies like adversarial training, where models are deliberately exposed to manipulated inputs during training to learn resistance. Benchmarks such as OODRobustBench evaluate model performance against out-of-distribution and adversarial examples, driving progress toward more resilient AI.
For example, vision foundation models like the Segment Anything Model (SAM) have been scrutinized for adversarial robustness, including vulnerabilities such as prompt manipulation that degrade performance.
Robustness ensures AI systems behave predictably even in unusual or hostile environments—a necessity for safety-critical applications like autonomous vehicles and medical diagnostics.
Watermarking: Tracing Ownership and Detecting Misuse
With the surge of AI-generated content—from images and videos to text—verifying authenticity and provenance has become paramount. Watermarking offers a promising solution by invisibly embedding identifiable patterns in AI outputs, akin to digital fingerprints.
Unlike visible watermarks, AI watermarks are subtle signals detectable only by specialized algorithms. Google’s SynthID, for instance, enables watermarking of audio content. Emerging statistical watermarking methods are exploring robustness across text and other media.
Watermarking serves multiple purposes:
- Ownership attribution: Proving content creation or authorization.
- Misuse detection: Identifying unauthorized model extraction or content alteration.
- Content provenance: Maintaining an immutable chain of custody for AI-generated media.
However, watermarking is not foolproof. Determined adversaries may attempt to remove or forge watermarks, and universal detection methods remain elusive. Embedding watermarks requires cooperation from model developers and raises privacy questions regarding data collection and tracking.
Still, as the AI ecosystem matures, watermarking could become a crucial tool for maintaining integrity and trust, especially when standardized by trusted bodies and combined with metadata provenance techniques.
Why These Techniques Matter
Each mitigation method addresses a distinct facet of AI risk—interpretability, fairness, security, and accountability. Together, they form a multilayered defense essential for:
- Maintaining user trust: Transparency and explainability help users confidently rely on AI decisions.
- Ensuring ethical compliance: Detecting and reducing bias protects marginalized groups from harm.
- Protecting system integrity: Robustness and watermarking prevent exploitation and unauthorized use.
- Enabling responsible innovation: Clear visibility into AI behavior fosters safer deployment across industries.
Absent these technical guardrails, AI risks devolving into opaque, biased, or vulnerable systems—undermining the very benefits it promises. Addressing these challenges head-on is not merely good engineering; it is a societal imperative as AI increasingly shapes our world.
Technique | Description | Key Methods/Tools | Importance | Challenges |
---|---|---|---|---|
Explainable AI (XAI) | Transforms AI from opaque black boxes into transparent glass boxes, providing interpretable insights into model decisions. | IBM AI Explainability 360, Chain-of-thought prompting in LLMs | Builds trust, accountability, helps detect biases early, enables informed decisions. | Balancing detail and simplicity; risk of misinterpretation; requires user education. |
Data Validation and Sanitization | Ensures training data quality by detecting and removing biases, poisoned or corrupted inputs. | Diverse data collection, automated bias detection tools, data cleansing pipelines, ongoing audits | Prevents perpetuation/amplification of harmful biases; maintains fairness. | Bias can be subtle and embedded in societal data; continuous effort needed as data evolves. |
Model Robustness | Strengthens AI models against adversarial attacks that manipulate inputs to cause errors or harmful outputs. | Adversarial training, benchmarks like OODRobustBench, evaluation of models like Segment Anything Model (SAM) | Ensures reliable and safe AI behavior in hostile or unusual environments. | Adversarial methods constantly evolve; robustness is hard to guarantee fully. |
Watermarking | Embedding invisible, detectable patterns in AI-generated content to trace ownership and detect misuse. | Google’s SynthID, statistical watermarking methods, metadata provenance techniques | Supports ownership attribution, misuse detection, content provenance, and integrity. | May be removed or forged; detection methods not universal; privacy concerns; requires cooperation. |
Troubleshooting Common AI Risk Management Challenges
Troubleshooting Common AI Risk Management Challenges
Managing AI risks often feels like trying to hit a moving target. The complexity of AI systems, fast-evolving regulations, and relentless innovation pressure create a perfect storm of challenges. Understanding these obstacles and deploying effective solutions is crucial for organizations striving to harness AI responsibly.
The Opaque Black-Box Problem: Why Transparency Matters—and How to Achieve It
Many AI models today—particularly deep neural networks and transformer-based architectures—function as inscrutable black boxes. This opacity complicates risk management because if you cannot understand how a model arrives at its decisions, trusting it to be fair, safe, or compliant is problematic.
Explainable AI (XAI) methods have advanced considerably by 2025, offering tools to demystify these black boxes. Techniques like Shapley values provide both local and global explanations, revealing the factors influencing individual predictions and overall model behavior. For instance, in healthcare, XAI helps clinicians identify which features contributed to a diagnostic recommendation, enabling critical verification and trust-building.
However, XAI is not a panacea. Global explanations may overlook important case-specific nuances, while local methods—such as LIME or saliency maps—can be computationally intensive and challenging to interpret. Multimodal AI models, which process text, images, and video simultaneously, pose further interpretability challenges because current XAI tools struggle to cohesively analyze diverse data types.
The key takeaway is that risk frameworks must integrate adaptive explainability tools tailored to specific applications and industry expertise. Embedding transparency throughout the AI lifecycle—from design to deployment—helps detect biases early, fosters user trust, and aligns with increasingly stringent regulatory requirements such as the EU AI Act.
Navigating the Shifting Sands: AI Regulation Amidst the Innovation Race
AI regulation is evolving rapidly, leaving organizations caught between the imperative to innovate and the necessity to comply with diverse, often fragmented, rules.
The EU AI Act exemplifies a comprehensive, risk-based regulatory approach, imposing strict oversight on high-stakes AI applications like healthcare and law enforcement. Non-compliance can lead to fines as high as €35 million or 7% of annual turnover. Elsewhere, U.S. states such as Virginia and New Jersey have enacted laws targeting synthetic content misuse, while federal agencies expand enforcement capabilities around AI and cybersecurity.
This regulatory landscape is often reactive and jurisdictionally fragmented, creating uncertainty for businesses. A 2025 Deloitte survey found that while 67% of organizations increased investments in generative AI, only 23% felt highly prepared for compliance. This gap underscores the need for proactive, flexible governance.
Balancing speed with safety requires building iterative risk management processes that incorporate continuous monitoring, testing, and refinement of AI systems. Automation can streamline compliance workflows, but human judgment remains indispensable for interpreting evolving regulations and ethical considerations.
Attribution and Accountability: Clarifying Responsibility When AI Causes Harm
Determining accountability in AI-related incidents remains a thorny issue. When AI systems cause harm—through biased outcomes, misinformation, or safety failures—the question of “who is responsible?” becomes paramount.
Accountability is not confined to a single actor; it spans developers, executives, users, vendors, and regulators. For example, Google’s Gemini chatbot faced public backlash for generating problematic content, leading to a suspension and public apology. Such failures highlight how accountability lapses can erode brand equity and public trust.
Establishing clear governance structures with well-defined roles and responsibilities is essential to closing accountability gaps. Frameworks like the RAILS AI Risk Management Guidance promote practical, step-by-step approaches over mere checkbox compliance. Transparency in model design, training datasets, and decision pathways is critical for tracing errors and mitigating risks.
Moreover, meaningful stakeholder engagement is vital. Involving cross-functional teams—including legal, compliance, technical experts, end-users, and leadership—ensures diverse perspectives shape AI deployment. Studies show that organizations employing integrated AI platforms for stakeholder collaboration resolve issues 85% faster and achieve 50% better alignment.
Human Oversight: Beyond Just a “Human in the Loop”
Many organizations assume that simply inserting a “human in the loop” suffices to catch AI errors before harm occurs. However, human oversight is effective only when designed thoughtfully as part of a comprehensive risk mitigation strategy.
Research from Boston Consulting Group stresses that effective oversight requires appropriate system design, training, and clear boundaries enabling humans to detect out-of-scope behaviors or subtle failures. This includes rigorous, continuous test-and-evaluation processes assessing AI performance over time.
In healthcare, for example, combining human oversight with algorithmic refinement helps prevent AI from perpetuating health disparities by ensuring training data is representative and diverse. Without such vigilance, AI’s promise risks becoming peril.
Actionable Strategies: Building Adaptive and Collaborative Risk Frameworks
To effectively address these challenges, organizations should implement the following strategies:
-
Adaptive Risk Frameworks: Employ layered explainability techniques matched to AI complexity and context. Continuously update risk assessments as models and regulations evolve.
-
Cross-Functional Stakeholder Collaboration: Foster ongoing dialogue among developers, compliance teams, end-users, and leadership to align expectations, clarify accountability, and anticipate emerging risks.
-
Robust Human Oversight Mechanisms: Integrate human review with automated monitoring and continuous testing to detect anomalies and escalate issues promptly.
-
Automated Compliance Tools: Leverage AI-powered solutions for documentation management, audit trails, and regulatory reporting, reducing manual effort and enhancing accuracy.
-
Transparency and Open Communication: Cultivate internal and external trust by openly sharing AI capabilities, limitations, and governance practices.
In an environment where AI capabilities advance faster than regulations and societal norms can keep pace, risk management must be dynamic, holistic, and ethically grounded. Cutting through hype with evidence-based practices and embedding safety and fairness as foundational principles enables organizations to unlock AI’s transformative potential while safeguarding against inherent risks.
Challenge | Description | Key Issues | Proven Strategies |
---|---|---|---|
The Opaque Black-Box Problem | AI models, especially deep neural networks, operate as inscrutable black boxes, complicating trust and risk management. |
|
|
Navigating AI Regulation | Rapidly evolving and fragmented regulatory landscape creates compliance uncertainty. |
|
|
Attribution and Accountability | Determining responsibility for AI-caused harm involves multiple stakeholders. |
|
|
Human Oversight | Effective oversight requires more than inserting a “human in the loop.” |
|
|
Actionable Strategies | Comprehensive approaches to building adaptive and collaborative risk frameworks. | — |
|
Advanced Risk Management and Future-Proofing: Optimizing AI Safety in an Evolving Landscape
Advanced Risk Management and Future-Proofing: Optimizing AI Safety in an Evolving Landscape
Building AI systems that are both powerful and resilient to an ever-shifting risk landscape is a critical challenge. As AI technologies advance at unprecedented speeds, architects of AI safety frameworks must ensure these frameworks evolve in step with technological capabilities—without hindering innovation.
For experienced practitioners, this means embracing emerging AI risk management frameworks, integrating adversarial AI threat intelligence, automating risk detection with AI-powered tools, and preparing for an increasingly complex regulatory environment. Together, these approaches form a robust defense against current and future AI risks.
Leveraging Emerging AI Risk Management Frameworks
A pivotal development in AI safety is the emergence of structured, AI-specific risk management frameworks. The National Institute of Standards and Technology (NIST) leads with its AI Risk Management Framework (AI RMF), currently in its second draft and open for community feedback through mid-2025.
Unlike prescriptive regulations, the AI RMF is a voluntary and adaptable guide designed to manage AI risks across the entire system lifecycle—from initial design through deployment and ongoing monitoring. It is grounded in core principles such as reliability, transparency, fairness, accountability, and security.
The framework organizes risk management into four core functions:
- Govern: Establish organizational policies and accountability structures.
- Map: Identify AI risks in context.
- Measure: Assess and quantify risk exposure.
- Manage: Mitigate and monitor risks continuously.
This modular approach enables organizations to tailor the AI RMF to their unique operational contexts, whether in healthcare, finance, or critical infrastructure.
Importantly, the AI RMF aligns with international efforts like ISO/IEC standards and the EU AI Act, creating harmonized expectations globally. Tools such as Wiz’s AI Security Posture Management (AI-SPM) facilitate adoption by automating risk assessments and attack path analyses, assisting organizations in identifying and closing security gaps promptly.
However, adoption remains uneven. Industry reports reveal many organizations struggle to fully operationalize these frameworks, underscoring the imperative to embed AI RMF principles early and iteratively. Doing so is essential for building trustworthy AI systems that can scale safely as complexity grows.
Incorporating Adversarial AI Threat Intelligence and Automation
While risk frameworks lay the foundation, continuous threat intelligence and automated risk detection act as real-time guardrails keeping AI safe.
Adversarial AI—where attackers exploit AI models or weaponize AI to enhance cyberattacks—is no longer theoretical. Recent intelligence from Cisco and Google highlights how state-backed Advanced Persistent Threat (APT) groups from Iran, China, Russia, and North Korea have leveraged large language models like Google’s Gemini for reconnaissance, malicious code development, and information operations.
This emerging threat landscape demands continuous monitoring and rapid response capabilities. NIST’s taxonomy on adversarial machine learning attacks provides a structured lens to understand threats such as:
- Model poisoning
- Evasion attacks
- Backdoor insertion
Concurrently, AI-powered security tools have evolved dramatically. Modern solutions integrate seamlessly with existing cybersecurity infrastructure, leveraging large datasets and advanced pattern recognition to predict, prioritize, and mitigate vulnerabilities faster than human teams alone.
Examples include AI-driven risk assessment platforms like RiskScope AI and IntelliRisk Defender, which analyze threat landscapes in real time and deliver actionable insights. This synergy of human expertise and AI-powered automation is crucial to counter increasingly sophisticated AI-enabled threats.
Notably, agencies like the UK’s National Cyber Security Centre (NCSC) warn that operational technology and critical infrastructure remain especially vulnerable to AI-driven exploits, emphasizing the urgency for proactive defense strategies.
Preparing for Regulatory Compliance and Ethical AI Evolution
AI safety is rapidly shifting from best practice to legal imperative. Governments worldwide are enacting frameworks such as the EU AI Act, U.S. Executive Orders, and sector-specific mandates in healthcare and finance to codify AI governance, transparency, and accountability.
Compliance is no longer a checkbox but a strategic priority. Organizations must benchmark their AI safety practices against evolving standards to ensure continuous improvement and legal alignment. Here, frameworks like the NIST AI RMF are invaluable for mapping internal policies to external regulatory requirements.
Ethical considerations add complexity, especially in sensitive domains like healthcare. Issues around privacy, bias, and trust dominate, as seen in Italy’s data protection authority investigating OpenAI for potential GDPR violations.
Ethical AI frameworks, such as the ETHICAL Principles AI Framework for Higher Education, emphasize that AI should augment—not replace—human decision-making. They call for transparency and fairness to be embedded throughout AI development and deployment.
Practically, companies adopt AI-powered compliance tools capable of dynamically monitoring regulatory changes and adjusting workflows accordingly. Platforms like SAS Viya and Wiz AI-SPM enable real-time compliance checks, helping organizations stay ahead of shifting legal landscapes.
Furthermore, recent surveys show growing executive focus on reskilling and job protection strategies to mitigate social risks associated with AI adoption, integrating human-centered ethics into governance approaches.
Benchmarking and Continuous Improvement: The Road Ahead
How can organizations confidently assess the effectiveness of their AI safety mechanisms? Benchmarking is emerging as a critical practice for evaluating not only AI capabilities but also safety, ethics, and robustness.
Traditional benchmarks like GLUE and SuperGLUE focus on language understanding, while newer initiatives target safety specifically. For instance, the Weapons of Mass Destruction Proxy (WMDP) benchmark tests AI knowledge in potentially dangerous domains.
Benchmarking AI safety is inherently challenging. Models trained on vast internet datasets may memorize benchmark content, inflating scores without reflecting true understanding or safety. This limitation highlights the need for comprehensive evaluation protocols aligned with concrete threat models rather than relying solely on numeric scores.
Innovative frameworks like HELM integrate multiple benchmarks into a holistic assessment, while initiatives such as the AI Safety Entrepreneurship Hackathon 2025 pioneer pre-deployment testing protocols to rigorously evaluate AI systems for safety, security, and ethical alignment.
Embedding continuous benchmarking against evolving standards into AI development cycles is essential. This iterative process ensures AI systems remain resilient as threats evolve and societal expectations shift. It also fosters transparency, enabling stakeholders—from developers to regulators—to make evidence-based judgments about AI trustworthiness.
Key Takeaways:
-
The NIST AI Risk Management Framework provides a flexible, principle-driven approach balancing innovation with safety across the AI lifecycle.
-
Adversarial AI threats are increasingly sophisticated and often state-sponsored, necessitating AI-powered threat intelligence integrated into cybersecurity operations.
-
Regulatory landscapes, though fragmented, converge on core principles of transparency, accountability, and ethical use; proactive compliance and ethical frameworks are now essential.
-
Benchmarking AI safety demands multidimensional, threat-aligned evaluations exceeding traditional capability tests to ensure robustness, fairness, and security.
In my experience spanning over 15 years, adopting these advanced risk management strategies is not solely about avoiding pitfalls—it is about unlocking AI’s transformative potential responsibly. The stakes are high, and the landscape evolves rapidly. Success depends on vigilance, adaptability, and a steadfast commitment to principled innovation.
Category | Details | Examples/Tools | Key Points |
---|---|---|---|
AI Risk Management Frameworks | Structured, adaptable guides managing AI risks across lifecycle | NIST AI RMF, ISO/IEC standards, EU AI Act, Wiz AI-SPM | Voluntary, principle-driven; four core functions: Govern, Map, Measure, Manage; uneven adoption; tailored to industries |
Adversarial AI Threat Intelligence & Automation | Continuous threat detection and response to AI-specific attacks | RiskScope AI, IntelliRisk Defender, NIST taxonomy | Threats include model poisoning, evasion, backdoors; state-backed APT groups using AI; integration with cybersecurity; critical infrastructure vulnerable |
Regulatory Compliance & Ethical AI | Align AI safety with evolving laws and ethics | EU AI Act, U.S. Executive Orders, ETHICAL Principles AI Framework, SAS Viya, Wiz AI-SPM | Transparency, accountability, privacy, bias concerns; dynamic compliance tools; focus on human-centered ethics and workforce reskilling |
Benchmarking & Continuous Improvement | Evaluate safety, robustness, and ethics using multidimensional benchmarks | GLUE, SuperGLUE, WMDP, HELM, AI Safety Entrepreneurship Hackathon 2025 | Challenges with memorization and misleading scores; need for threat-aligned protocols; enables iterative trustworthiness assessment |