The Future of AI in Business: 5 Critical Predictions for 2030
- Introduction: Why the Next Decade is Crucial for AI in Business
- Introduction: Why the Next Decade is Crucial for AI in Business
- AI’s Transformative Power on Business and Society by 2030
- The Convergence Driving AI’s Business Applications: Frontier Models, Data, and Ethics
- Critical Questions on AI’s Promise and Peril for Business
- From Automation to Augmentation: The Evolution of AI Capabilities in Business Operations
- From Automation to Augmentation: The Evolution of AI Capabilities in Business Operations
- The Technical Shift: From Rule-Based Automation to Generative and Multimodal Intelligence
- From Repetitive Task Automation to Decision and Creativity Augmentation
- Real-World Examples: Retail and Supply Chain as AI Frontiers
- Challenges in Integration: Legacy Systems and Human Workflows
- Navigating the Path Forward
- Data as the New Currency: Navigating the Explosion of Unstructured Data and Its Impact
- Data as the New Currency: Navigating the Explosion of Unstructured Data and Its Impact
- The Challenge of Managing Unstructured Data’s Deluge
- Unlocking Insights with Advanced AI and Analytics
- The Imperative of Data Quality and Governance
- Real-World Successes and Lessons Learned
- Looking Ahead: Navigating the Data Explosion
- Ethics, Transparency, and Regulation: Preparing for Mandatory AI Accountability
- Ethics, Transparency, and Regulation: Preparing for Mandatory AI Accountability
- Navigating the Emerging Regulatory Landscape
- Technical Pillars for Ethical AI Deployment
- Balancing Innovation with Responsible AI Use
- Lessons from Successes and Failures
- Key Takeaways for Business Leaders
- Hyper-Personalization and Customer Experience: AI’s Role in the Next-Generation Consumer Interface
- Hyper-Personalization and Customer Experience: AI’s Role in the Next-Generation Consumer Interface
- Technical Capabilities Powering Hyper-Personalization
- From Personalization to Hyper-Personalized Customer Journeys
- Societal Implications: Privacy, Ethics, and the Human-AI Boundary
- Key Takeaways
- Competitive Advantage through AI Integration: Benchmarking Industry Leaders and Lagging Sectors
- Competitive Advantage through AI Integration: Benchmarking Industry Leaders and Lagging Sectors
- The Divide Between AI Leaders and Stragglers
- Technical and Organizational Differentiators of AI Frontrunners
- Measuring Impact: Productivity Gains, Market Shifts, and Economic Outcomes
- Bridging the AI Capability Gap
- Final Thoughts
- Conclusion: Navigating Uncertainties and Embracing a Balanced AI Future in Business
- Conclusion: Navigating Uncertainties and Embracing a Balanced AI Future in Business
- AI as Both Catalyst and Challenge
- The Fog of Uncertainty: Technological, Regulatory, and Societal Factors
- Towards a Balanced, Adaptive AI Strategy
- Moving Forward: Embrace Complexity, Avoid Hype
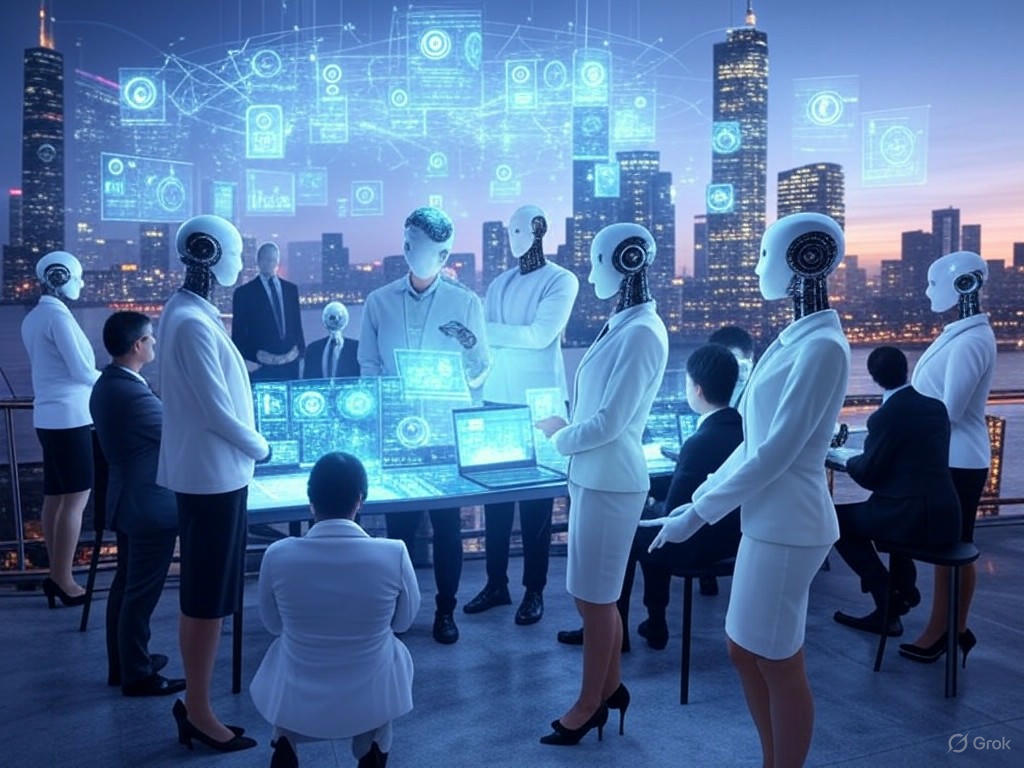
Introduction: Why the Next Decade is Crucial for AI in Business
Introduction: Why the Next Decade is Crucial for AI in Business
What if the next ten years determine not only which companies thrive but also how societies adapt to AI’s sweeping transformations? The coming decade is more than a simple timeline—it represents a pivotal juncture where artificial intelligence will redefine business operations, reshape competitive landscapes, and prompt profound ethical considerations.
AI’s Transformative Power on Business and Society by 2030
Today, nearly every company invests in AI tools, yet only about 1% consider themselves mature in their AI journey, according to McKinsey’s global survey. The long-term economic opportunity from AI adoption is estimated at a staggering $4.4 trillion. However, this value will not be realized simply by deploying technology; it demands a fundamental shift in how businesses operate and how people work.
By 2030, the World Economic Forum projects that roughly 70% of the skills required for most jobs will change. This is not merely an incremental upgrade but a near-complete overhaul of workforce capabilities. C-suite executives overwhelmingly agree that AI will spark a cultural shift, fostering more innovative and agile teams. Yet, this transformation requires leadership that goes beyond superficial AI adoption—embedding AI deeply into processes while making substantial investments in upskilling and reskilling employees.
The global AI market is poised for explosive growth, projected to surge from $189 billion in 2023 to nearly $5 trillion by 2033, per UNCTAD. This expansion reflects AI’s evolution from a niche advantage to a business imperative across industries—from healthcare and manufacturing to finance and retail. For instance, Microsoft’s AI-powered Copilot tools are already utilized by over 85% of Fortune 500 companies to automate workflows and reduce costs, illustrating AI’s shift from experimental to essential.
Yet, AI’s impact extends far beyond economic metrics. It will reshape societal roles and expectations. While AI promises productivity gains—boosting labor productivity growth by an estimated 1.5 percentage points over the next decade—it also raises concerns about job displacement and inequality. The International Monetary Fund projects that up to 40% of jobs worldwide could be affected. Companies face the delicate task of balancing automation benefits against social responsibility.
The Convergence Driving AI’s Business Applications: Frontier Models, Data, and Ethics
What fuels this unprecedented AI revolution? It is the convergence of three dynamic forces: cutting-edge AI models, an explosion of accessible data, and an accelerating focus on ethical AI deployment.
Frontier AI models such as large language models (LLMs) and generative AI have moved from futuristic concepts to central components of today’s business strategies. In 2024 alone, private investment in U.S.-based AI reached $109 billion, with generative AI attracting nearly $34 billion globally, according to the 2025 Stanford AI Index. These investments are driving not just larger models but smarter ones capable of reasoning, personalization, and complex decision support.
However, most AI input data remains unstructured—encompassing emails, documents, images, and more—presenting both challenges and opportunities. Organizations are increasingly leveraging generative AI to organize and interpret their own data pools, enhancing decision intelligence and operational agility. MIT Sloan emphasizes that leaders are learning to respect data stewardship as much as the data itself, recognizing that clean, diverse datasets are foundational to reliable AI outcomes.
Ethical considerations are integral, not auxiliary. Issues such as algorithmic bias, data privacy, and energy consumption are front and center. For example, AI’s growing computational demands threaten to increase data center energy use by 35% by 2026, raising sustainability concerns. Additionally, AI risks exacerbating existing inequalities—a challenge that leaders must address through inclusive workforce development and transparent governance. Bhaskar Chakravorti highlights that companies which build trust through ethical AI practices can not only mitigate harm but also unlock greater demand and market opportunities.
Critical Questions on AI’s Promise and Peril for Business
As AI’s footprint expands, executives and stakeholders face pressing questions:
- How can true productivity gains from AI be measured beyond hype and anecdote?
- Will AI-driven automation ultimately create more jobs than it displaces?
- What governance structures ensure AI systems are trustworthy, unbiased, and secure?
- How can companies prepare their workforce for an AI-integrated future without leaving anyone behind?
- How can rapid innovation be balanced with ethical imperatives and societal impact?
Current expert analyses offer cautious optimism. PwC stresses that “leading with trust” is essential to realizing AI’s potential in transforming business outcomes. McKinsey’s surveys reveal that while 78% of organizations use AI in at least one business function, only a small fraction report mature, enterprise-wide impact. This gap underscores the critical need for deliberate scaling strategies, robust experimentation, and meaningful outcome measurement.
Concurrently, AI is revolutionizing enterprise risk management by anticipating threats and streamlining compliance, as noted by Workday’s insights on document intelligence. Yet, concerns about AI’s ethical dilemmas persist across academia and industry, emphasizing the necessity of a moral compass in AI development and deployment.
The next decade will not merely be about technological advances in AI but about how businesses harness these advances responsibly to redefine work, competitiveness, and societal roles. Navigating this complex landscape requires clear-eyed understanding—cutting through hype, embracing evidence, and committing to ethical stewardship. Only through such deliberate efforts can AI’s transformative potential be fully realized as a force for inclusive growth and innovation.
Topic | Details |
---|---|
AI Maturity in Companies | ~1% consider themselves mature in AI journey (McKinsey) |
Economic Opportunity from AI | Estimated $4.4 trillion long-term value |
Skill Changes by 2030 | ~70% of skills required for most jobs will change (World Economic Forum) |
AI Market Growth | From $189 billion in 2023 to nearly $5 trillion by 2033 (UNCTAD) |
Microsoft AI Adoption | Over 85% of Fortune 500 use AI-powered Copilot tools |
Labor Productivity Growth | Boost by estimated 1.5 percentage points over next decade |
Jobs Affected by AI | Up to 40% of jobs worldwide could be affected (IMF) |
Private AI Investment (2024) | $109 billion in U.S., $34 billion in generative AI globally (Stanford AI Index) |
Data Center Energy Use Increase | Projected 35% increase by 2026 due to AI computational demands |
AI Use in Organizations | 78% use AI in at least one business function; few report mature enterprise-wide impact (McKinsey) |
From Automation to Augmentation: The Evolution of AI Capabilities in Business Operations
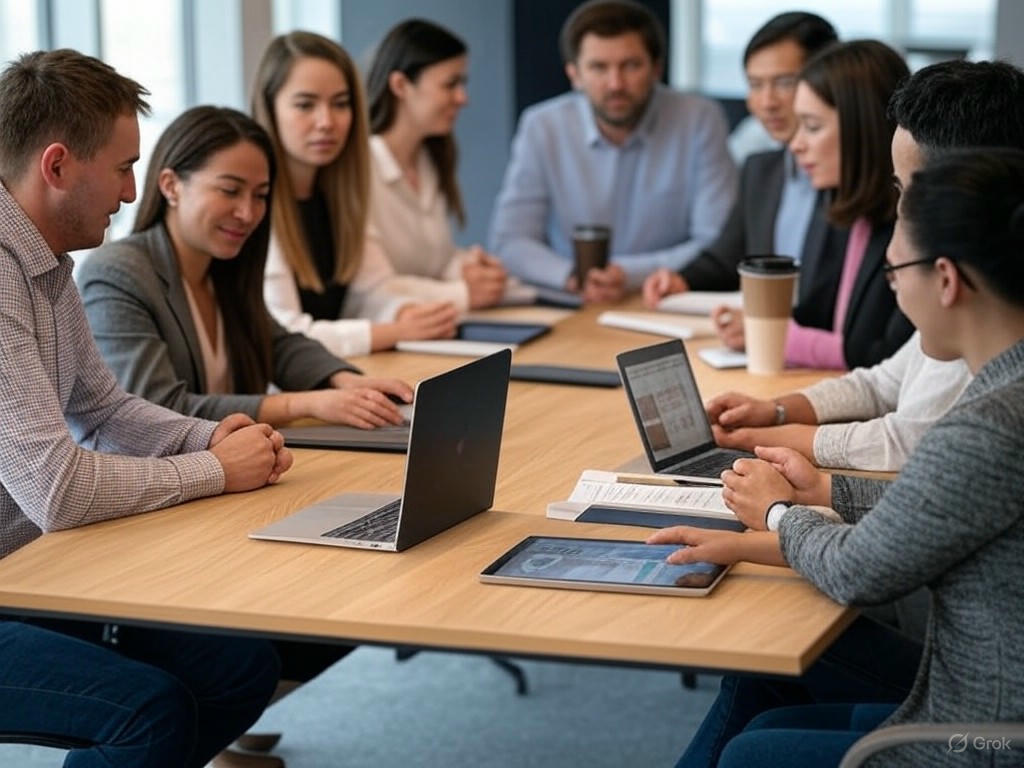
From Automation to Augmentation: The Evolution of AI Capabilities in Business Operations
What if AI in business was not about replacing humans but about becoming their most capable partner? Over the past decade, AI’s technical trajectory reveals a profound shift—from rigid rule-based automation to dynamic augmentation powered by generative and multimodal AI models. This evolution transcends technology; it redefines how enterprises operate, innovate, and compete in an increasingly complex landscape.
The Technical Shift: From Rule-Based Automation to Generative and Multimodal Intelligence
Traditional automation resembled a well-scripted factory line: predefined rules executed repetitive tasks with high precision but zero adaptability. Consider a basic chatbot that answers a fixed set of FAQs—functional yet limited in scope and flexibility. Today, AI systems are evolving into what McKinsey terms a “superagency” within enterprises, where AI not only executes but actively collaborates with human workers.
At the forefront of this evolution are generative AI (GenAI) and multimodal AI models. GenAI systems, trained to create new content—including text, images, designs, and code—transcend fixed instructions and enable real-time business innovation. For example, conversational “copilots” assist maintenance technicians by generating troubleshooting steps on the fly rather than relying solely on static manuals (LinkedIn, 2025).
Multimodal AI integrates and processes diverse data types simultaneously—text, images, audio—bringing a natural fluidity to human-computer interactions. Instead of navigating separate apps or isolated data silos, employees can query complex datasets conversationally, supported by AI that “sees” and “hears” contextually (SuperAnnotate, 2025). This multi-sensory approach is already transforming retail and e-commerce, where AI aligns product images, customer reviews, and inventory data into cohesive, actionable insights.
From Repetitive Task Automation to Decision and Creativity Augmentation
The most striking impact of AI’s evolution is its empowerment of human decision-making and creativity rather than merely replacing laborious tasks. In retail automation, AI-driven systems are approaching near-full task augmentation—handling inventory restocking, personalized customer engagement, and dynamic marketing campaigns. This shift is about more than efficiency; it enables scaling of human judgment and creative capacity (Microsoft Blog, 2025).
In supply chain management, AI’s role extends to predictive analytics and real-time optimization, enabling companies to navigate volatility from climate disruptions or geopolitical risks. AI-powered risk management tools offer end-to-end visibility and resilience, facilitating proactive decision-making that would be impossible at scale without AI (World Economic Forum, 2025; International Journal of CSIT Research, 2025).
Creativity is also experiencing a renaissance with AI assistance. Studies show that teams using AI content tools produce 68% more material while reducing production time by nearly half (Medium, 2025). AI generates initial drafts or design concepts, which human experts refine and contextualize. This collaboration breaks through traditional creative blocks and opens new avenues for innovation.
Real-World Examples: Retail and Supply Chain as AI Frontiers
-
Retail Automation: Leading retailers employ AI agents to automate inventory management, customer service, and personalized marketing. These AI tools analyze customer behavior, predict trends, and tailor promotions dynamically, creating more responsive and efficient shopping experiences (Microsoft Blog, 2025).
-
Supply Chain Optimization: AI-powered platforms integrate multimodal data sources—including weather reports, transportation logs, and geopolitical news—to anticipate disruptions and optimize logistics routes. Companies leveraging these technologies report significant cost reductions and improved sustainability through smarter resource allocation (SupplyChainBrain, 2025; World Economic Forum, 2025).
Challenges in Integration: Legacy Systems and Human Workflows
Despite these advances, integrating AI augmentation into existing business ecosystems is far from straightforward. Approximately 70% of enterprises still depend on legacy systems built before AI’s rise, posing significant integration barriers (ItSoli, 2025). These systems often lack the flexibility or infrastructure necessary for modern AI workloads, exposing organizations to security vulnerabilities and operational disruptions (Integrass, 2025).
Moreover, AI augmentation requires deliberate redesign of human workflows. Poorly designed AI-human interfaces risk alienating employees, diminishing productivity, or causing operational friction. The optimal balance between automation and human oversight varies by industry and task complexity. For instance, retail environments may favor higher automation levels, whereas healthcare demands cautious augmentation to preserve critical decision-making oversight (LinkedIn Workflow Integration, 2025).
Workforce readiness is another crucial factor. Skills such as “prompt engineering” and AI ethics literacy are becoming essential to ensure employees can effectively command, interpret, and audit AI outputs (UNLEASH, 2025). Without these competencies, the “superagency” potential of AI remains untapped or, worse, leads to mistrust and misuse.
Navigating the Path Forward
The ongoing challenge for businesses is to treat AI not as a one-off project but as a living system requiring continuous adaptation. This includes modernizing data infrastructures, investing in skills development, and embracing iterative deployment models (LinkedIn Enterprise AI Adoption, 2025). The promise of AI augmentation is immense but demands clear-eyed understanding of technical complexity, organizational change, and ethical responsibility.
In sum, the evolution from automation to augmentation marks a pivotal moment in business operations. AI is no longer just a tireless worker executing tasks; it is becoming a creative and analytic partner that extends human potential. The next decade will belong to organizations that master this partnership—harnessing AI’s capabilities while thoughtfully integrating it with human expertise and legacy realities.
Aspect | Traditional Automation | Modern AI Augmentation |
---|---|---|
Technology | Rule-based, predefined tasks | Generative AI, multimodal AI models |
Functionality | Repetitive task execution | Decision-making and creativity support |
Examples | Basic chatbots answering FAQs | Conversational copilots, multimodal data integration |
Business Impact | Efficiency in repetitive tasks | Scaling human judgment and innovation |
Industry Applications | Limited to automation of basic tasks | Retail automation, supply chain optimization |
Challenges | Minimal integration complexity | Legacy system integration, workflow redesign, workforce readiness |
Workforce Skills | Basic operational skills | Prompt engineering, AI ethics literacy |
Data as the New Currency: Navigating the Explosion of Unstructured Data and Its Impact
Data as the New Currency: Navigating the Explosion of Unstructured Data and Its Impact
By 2030, the volume of unstructured data is poised to grow tenfold, fundamentally reshaping how businesses operate. This surge is not speculative; by 2025, unstructured data—including emails, images, videos, social media posts, and sensor outputs—is expected to constitute 80% of all data collected globally. This vast and varied data landscape presents a paradox: an immense reservoir of actionable insights locked behind significant technical and organizational challenges.
The Challenge of Managing Unstructured Data’s Deluge
Unstructured data resists traditional storage and analysis methods. Unlike structured data organized in rows and columns, unstructured data arrives in diverse and unpredictable formats, making it difficult to locate, organize, and interpret efficiently.
The risks of unmanaged data are stark. Currently, the average time to identify a data breach is 207 days, followed by an additional 70 days to contain it—highlighting vulnerabilities in sprawling data ecosystems. Moreover, poor data quality alone costs the U.S. economy approximately $3.1 trillion annually, underscoring the critical importance of data integrity.
As data scales exponentially, the complexity and risks increase dramatically. Businesses relying on timely, accurate insights must adopt sophisticated data management strategies that emphasize not only volume but also quality, governance, and accessibility.
Unlocking Insights with Advanced AI and Analytics
How can businesses transform this chaotic data environment into a strategic asset? The answer lies in leveraging advances in natural language processing (NLP), machine learning (ML), and real-time analytics.
Transformer-based models, such as GPT-4, have revolutionized machine understanding of human language, enabling nuanced interpretation of complex text and multilingual processing. In healthcare, NLP accelerates drug discovery by extracting insights from clinical notes and medical imaging. Retailers analyze customer feedback across social media platforms like TikTok and Instagram, detecting emergent trends and personalizing experiences at scale.
AI-driven data extraction tools automate parsing diverse data types—including emails, images, videos, and sensor data—simplifying the retrieval of actionable insights. Real-time analytics platforms like Apache Kafka and Apache Flink process streaming data with minimal latency, allowing rapid responses to market fluctuations and operational issues.
This integration of cutting-edge technologies is turning unstructured data from a liability into a potent competitive advantage.
The Imperative of Data Quality and Governance
With greater data comes increased responsibility. The unrelenting growth of unstructured data demands rigorous attention to data quality and governance. Trustworthy data must be accurate, complete, consistent, and timely; without these pillars, AI models falter and organizational decisions suffer.
Data governance is evolving from rigid, top-down control toward collaborative, community-led frameworks. Platforms such as Atlan and Ataccama streamline workflows, automate compliance, and maintain comprehensive data catalogs, embedding stewardship into daily operations. This ensures that data owners, analysts, and business leaders share a unified understanding and accountability.
Modern data leaders must adopt an “everything, everywhere, all at once” mindset—recognizing the ubiquity and velocity of data while balancing agility with control. This entails investing in metadata maturity, automating repetitive tasks, and fostering data literacy throughout the organization. While AI can accelerate governance processes, it requires vigilant oversight to mitigate ethical risks and maintain transparency.
Real-World Successes and Lessons Learned
The impact of data-driven AI is tangible and measurable. For instance, Microsoft 365 Copilot automates workflows across enterprises, yielding an average return of $3.70 for every dollar invested. Netflix’s AI-powered recommendation engine leverages unstructured viewing data to personalize content at scale, significantly enhancing user engagement and retention.
However, many AI initiatives do not advance beyond proof-of-concept stages. Research indicates that 80% of AI and machine learning projects stall, often due to poor data quality, unclear strategy, or misaligned success metrics. Zillow’s iBuying model serves as a cautionary tale, having faltered by neglecting nuanced market signals embedded in unstructured data.
Key pitfalls to avoid include:
- Rushing AI deployment without ensuring data quality and robust governance.
- Neglecting cross-functional collaboration among data engineers, analysts, and business leaders.
- Failing to integrate AI solutions seamlessly with legacy systems, causing costly disruptions.
- Underestimating the importance of continuous measurement and experimentation to validate AI’s business impact.
Organizations like JPMorgan have responded by establishing dedicated AI centers of excellence to ensure security, transparency, and strategic alignment in their AI initiatives.
Looking Ahead: Navigating the Data Explosion
As 2030 approaches, mastering the management and utilization of unstructured data will distinguish business leaders from laggards. The data explosion is not merely a technical challenge but a strategic inflection point requiring a holistic approach that includes:
- Robust data governance frameworks.
- Deployment of advanced AI and real-time analytics tools.
- Cultural shifts toward widespread data literacy.
- Unwavering focus on data quality and ethical stewardship.
In essence, data is the new currency—but its value depends on an organization’s infrastructure, capabilities, and mindset to convert it into meaningful insights. Those who succeed in this endeavor will unlock unprecedented competitive advantages in the decade ahead.
Aspect | Details |
---|---|
Unstructured Data Growth by 2030 | 10x increase in volume |
Unstructured Data Percentage by 2025 | 80% of all data collected globally |
Average Time to Identify Data Breach | 207 days |
Average Time to Contain Data Breach | 70 days after identification |
Annual Cost of Poor Data Quality to U.S. Economy | $3.1 trillion |
AI Project Failure Rate | 80% stall before production |
Microsoft 365 Copilot ROI | $3.70 returned per $1 invested |
Ethics, Transparency, and Regulation: Preparing for Mandatory AI Accountability
Ethics, Transparency, and Regulation: Preparing for Mandatory AI Accountability
What does accountability look like when AI systems become decision-makers in business? The implementation of the EU AI Act, set to take full effect by August 2027, marks a pivotal shift: AI will no longer operate in a regulatory vacuum. As the world’s first comprehensive legal framework specifically targeting AI, the Act categorizes AI systems by their risk—from unacceptable to minimal—and imposes stringent controls on those deemed high-risk. This development mandates that businesses, especially in Europe and increasingly worldwide, demonstrate transparency and ethical stewardship in how they develop and deploy AI technologies.
Navigating the Emerging Regulatory Landscape
The EU AI Act exemplifies a rigorous, risk-based regulatory approach that other regions are beginning to adopt. In contrast, the United States currently maintains a fragmented landscape, relying on a patchwork of state laws and sector-specific guidelines. For instance, California’s AI Transparency Act enforces substantial penalties—up to $5,000 per violation per day—while Colorado’s AI Act incorporates elements from the EU framework to regulate high-risk AI applications.
This regulatory divergence presents complex compliance challenges for multinational organizations. Yet, it also signals a future where AI governance is non-negotiable. Businesses must prepare for a landscape where third-party audits, mandatory risk disclosures, and continuous AI system monitoring become standard practices rather than optional measures.
Technical Pillars for Ethical AI Deployment
Regulatory expectations translate into clear technical imperatives. Pre-deployment risk assessments form the foundation of responsible AI use: organizations need systematic evaluations of AI models to detect bias, privacy vulnerabilities, and unintended consequences before deployment. Frameworks like the AI Risk Profiles categorize risks into nine distinct domains, creating a common language that bridges technical teams and compliance officers.
Continuous monitoring is equally vital. AI models are dynamic—they evolve due to data drift, concept drift, and emergent behaviors. AI observability tools such as Fiddler AI, Superwise, and Dynatrace provide advanced capabilities for tracking model performance, detecting bias, and evaluating explainability metrics in real time. These tools empower organizations to identify warning signs early, ensuring AI decisions remain fair, accurate, and aligned with both ethical standards and regulatory requirements.
Third-party audits have emerged as a crucial control mechanism. The Institute of Internal Auditors’ Third-Party Topical Requirement mandates standardized auditing processes for vendor risk management. Increasingly, companies require AI suppliers to disclose training data provenance, algorithmic logic, and security practices. Such transparency not only mitigates operational and legal risks but also fosters trust among stakeholders.
Balancing Innovation with Responsible AI Use
How can businesses continue to innovate with AI while adhering to ethics and regulatory demands? The key is embedding governance structures and ethical guidelines throughout the AI lifecycle from inception. AI governance experts like Oliver Patel advocate “BYOAI” or “Train Your Own AI” policies, which empower organizations to maintain control over data provenance and model behavior instead of relying blindly on external tools.
Establishing AI ethics boards or cross-functional committees—including technical experts, legal counsel, and ethicists—helps maintain principled development and deployment practices. Clear guidelines focusing on explainability, auditability, and human oversight are no longer optional; they have become competitive advantages that reduce risk and enhance user confidence.
Lessons from Successes and Failures
The stakes for ethical AI deployment could not be higher. Real-world case studies illustrate both the perils and promises of AI ethics in business.
-
IBM Watson for Oncology: Despite significant investment, Watson’s AI-driven treatment recommendations faltered due to inaccuracies and unsafe guidance. This failure underlines the necessity of rigorous validation and continuous oversight before deploying AI in high-risk domains.
-
Amazon’s Hiring Algorithm: The system was found to produce discriminatory outcomes against female candidates, highlighting how unchecked biases embedded in training data can cause real-world harm and legal liability.
-
The Wrongful Conviction of Michael Williams: Algorithmic errors in predictive policing algorithms contributed to a miscarriage of justice, starkly reminding us that AI’s societal impacts extend beyond business metrics to fundamental human rights.
Conversely, companies that proactively implement transparency measures, conduct third-party audits, and maintain continuous AI observability tend to avoid such pitfalls. Integrating ethics into AI development is not just about compliance; it is about safeguarding brand reputation, customer trust, and long-term viability.
Key Takeaways for Business Leaders
-
The EU AI Act is a trailblazer in AI regulation; global frameworks are expected to follow its risk-based model, making AI accountability mandatory rather than voluntary.
-
Robust pre-deployment risk assessments, third-party audits, and continuous monitoring are essential technical controls to meet evolving legal and ethical standards.
-
AI governance must be ingrained in organizational culture, balancing innovation with responsibility through clear policies, ethical oversight, and fostering AI literacy.
-
Learning from past AI failures—and successes—equips businesses to navigate the complexities of AI ethics and regulation confidently.
Preparing for mandatory AI accountability is not a bureaucratic hurdle—it is a strategic imperative. Organizations embracing transparency and ethical rigor today will be the leaders shaping a trustworthy AI-powered tomorrow.
Aspect | EU AI Act | United States | Examples of US State Laws |
---|---|---|---|
Implementation Date | August 2027 (full effect) | Ongoing, fragmented | California AI Transparency Act, Colorado AI Act |
Regulatory Approach | Comprehensive, risk-based framework categorizing AI systems by risk level | Patchwork of state laws and sector-specific guidelines | California: penalties up to $5,000/violation/day; Colorado: adopts EU elements for high-risk AI |
Focus Areas | Transparency, ethical stewardship, mandatory accountability | Varies by state, includes transparency and risk regulation | Transparency, risk disclosure, vendor management |
Compliance Requirements | Third-party audits, mandatory risk disclosures, continuous AI monitoring | Varies; no unified federal mandate | State penalties and compliance mandates |
Technical Control | Description | Examples/Tools |
---|---|---|
Pre-deployment Risk Assessment | Systematic evaluation to detect bias, privacy vulnerabilities, unintended consequences before AI deployment | AI Risk Profiles (9 risk domains) |
Continuous Monitoring | Ongoing tracking of AI models to detect data drift, concept drift, emergent behaviors | Fiddler AI, Superwise, Dynatrace |
Third-Party Audits | Standardized auditing processes for vendor risk management, including transparency on training data and algorithms | Institute of Internal Auditors’ Third-Party Topical Requirement |
Case Study | Outcome/Issue | Lesson Learned |
---|---|---|
IBM Watson for Oncology | Inaccurate and unsafe treatment recommendations | Need for rigorous validation and continuous oversight in high-risk AI applications |
Amazon’s Hiring Algorithm | Discriminatory against female candidates | Unchecked biases in training data cause harm and legal liability |
Wrongful Conviction of Michael Williams | Algorithmic errors in predictive policing | AI impacts extend to fundamental human rights beyond business metrics |
Key Takeaway | Description |
---|---|
EU AI Act as Trailblazer | Sets global precedent for mandatory AI accountability through risk-based regulation |
Essential Technical Controls | Pre-deployment risk assessments, third-party audits, and continuous monitoring are critical |
Embedding AI Governance | Governance and ethics must be part of organizational culture balancing innovation and responsibility |
Learning from Past AI Failures and Successes | Proactive transparency and oversight mitigate risks and build trust |
Hyper-Personalization and Customer Experience: AI’s Role in the Next-Generation Consumer Interface
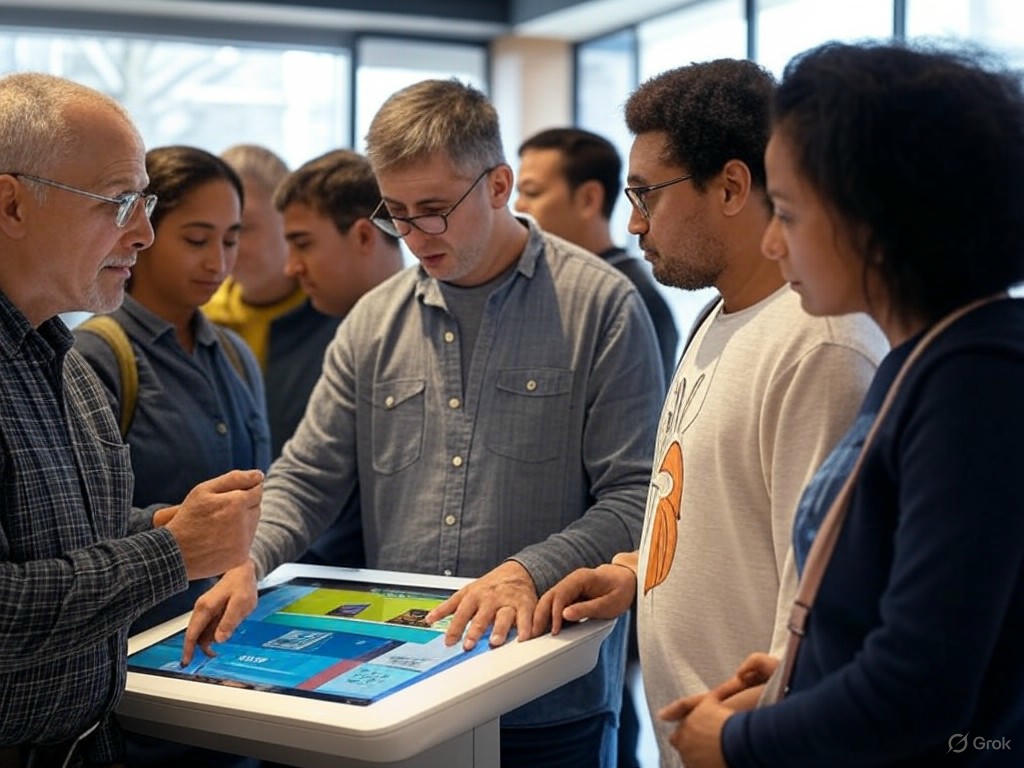
Hyper-Personalization and Customer Experience: AI’s Role in the Next-Generation Consumer Interface
Imagine a world where your favorite brand senses not only what you want but also how you feel in the moment. This is no longer a distant sci-fi scenario but an imminent reality propelled by rapid advances in emotion recognition, behavioral pattern analysis, and hybrid human-AI interactions. By 2030, customer experiences will reach an unprecedented level of hyper-personalization, fundamentally changing how businesses engage consumers across every touchpoint.
Technical Capabilities Powering Hyper-Personalization
At the heart of this transformation lies emotion AI—technologies that analyze facial expressions, voice tone, physiological signals, and behavioral cues to infer emotional states in real time. Platforms like Viso Suite and Luxand.cloud already enable scalable deployment of AI vision applications capable of detecting emotions such as anger, happiness, fear, and sadness with remarkable accuracy.
For instance, algorithms trained on benchmark datasets like the Extended Cohn–Kanade database (CK+) can identify subtle microexpressions that feed into real-time service adjustments or personalized recommendations. Advances in edge computing, exemplified by frameworks such as TensorFlow Lite, facilitate on-device inference with minimal latency, bolstering both privacy and responsiveness.
Beyond facial analysis, multimodal emotion AI integrates voice sentiment analysis and behavioral pattern recognition. Leading AI platforms like IBM Watson, Google Cloud AI, and Microsoft’s AI offerings demonstrate how fusing multiple data modalities yields a richer and more precise understanding of customer intent and mood—crucial for delivering empathetic, context-aware interactions.
On the recommendation side, AI systems are evolving beyond traditional collaborative filtering toward anticipatory personalization. These sophisticated engines incorporate not just purchase history but also real-time emotional and environmental context. For example, a virtual shopping assistant might detect customer frustration during checkout and proactively offer assistance or alternative options before being prompted.
From Personalization to Hyper-Personalized Customer Journeys
Current personalization strategies primarily leverage demographics, purchase histories, and explicit preferences. While effective, these methods remain largely reactive and confined to individual channels. The future lies in hyper-personalization, a dynamic, continuous tailoring of the entire customer journey powered by AI’s ability to integrate diverse data streams—including emotional signals.
Organizations like The New York Times already use AI to schedule content delivery optimized for individual reader behavior. Similarly, Microsoft 365 Copilot adapts workflows in real time based on user context. By 2030, seamless omnichannel experiences will be the norm, where AI intuitively knows not just what customers need but when and how to deliver it, adjusting tone and content according to emotional cues.
Hybrid human-AI interactions will become the standard. AI systems will manage routine, data-intensive tasks and monitor emotional signals, while human agents intervene when empathy, creativity, or complex judgment is necessary. This synergy enhances customer satisfaction and operational efficiency, as seen in emerging AI-powered customer service models.
Establishing hyper-personalization demands more than cutting-edge algorithms; it requires rigorous experimentation, transparency, and robust ethical frameworks. Explainable AI (XAI) will be indispensable, enabling businesses and consumers to understand AI-driven decisions and fostering trust in an era where AI anticipates needs before they are explicitly communicated.
Societal Implications: Privacy, Ethics, and the Human-AI Boundary
With advanced capabilities come significant responsibilities. The widespread use of AI to read and respond to human emotions raises profound privacy and ethical concerns. Regulatory frameworks such as the European Union’s AI Act already restrict AI emotion recognition in workplaces except for medical or safety-critical purposes, reflecting societal unease about pervasive surveillance and informed consent.
While consumers generally appreciate AI-driven personalization, they place a premium on data privacy and transparency. According to the Cisco 2025 Data Privacy Benchmark Study, 80% of customers are more inclined to engage with brands that respect their privacy and clearly communicate data usage. Consequently, companies must implement rigorous data governance, adopting privacy-preserving techniques like federated learning, which processes data locally on devices to minimize exposure.
Another complex challenge is the blurring of lines between human and machine interactions. As AI agents grow increasingly emotionally intelligent, distinguishing genuine human empathy from AI-generated responses becomes more difficult. This raises risks of manipulation and potential erosion of authentic human connection.
The path forward requires balancing innovation with ethical safeguards. This includes designing AI systems that empower users without deception, ensuring informed consent and fairness, and maintaining clear escalation protocols where humans assume control when nuance and empathy are paramount.
Key Takeaways
-
Emotion AI and behavioral analytics are advancing rapidly, enabling real-time, multimodal detection of user emotions.
-
Hyper-personalization will revolutionize customer journeys by integrating emotional context and situational awareness, moving well beyond traditional segmentation and recommendation models.
-
Hybrid human-AI teams will become essential for delivering empathetic, contextually appropriate customer experiences at scale.
-
Privacy, transparency, and ethical AI design are non-negotiable to preserve consumer trust and comply with evolving regulations like the EU AI Act.
-
The next decade will test businesses’ ability to balance technological capabilities with respect for human dignity in customer interactions.
Having architected AI systems for over 15 years, I view hyper-personalization as a double-edged sword: a powerful tool to delight customers and drive business growth, yet one that demands ongoing vigilance, humility, and a steadfast commitment to ethical stewardship. The technology is poised to transform consumer engagement—how we wield it will define the future of customer experience.
Key Takeaway | Description |
---|---|
Advancement in Emotion AI and Behavioral Analytics | Enables real-time, multimodal detection of user emotions using facial expressions, voice tone, physiological signals, and behavioral cues. |
Hyper-Personalization Revolution | Transforms customer journeys by integrating emotional context and situational awareness, surpassing traditional segmentation and recommendation models. |
Hybrid Human-AI Teams | Essential for delivering empathetic, contextually appropriate customer experiences at scale by combining AI capabilities with human creativity and judgment. |
Privacy, Transparency, and Ethical AI Design | Critical to preserving consumer trust and complying with regulations like the EU AI Act, emphasizing data governance and ethical frameworks. |
Balancing Technology and Human Dignity | The next decade will test businesses’ ability to wield AI technology responsibly to enhance customer engagement without compromising ethical standards. |
Competitive Advantage through AI Integration: Benchmarking Industry Leaders and Lagging Sectors
Competitive Advantage through AI Integration: Benchmarking Industry Leaders and Lagging Sectors
The defining trait of AI frontrunners is not merely the adoption of technology but the profound integration of AI within their data strategies, organizational culture, and operational core. Leading businesses embed AI into fundamental processes, transforming it from a peripheral tool into a strategic enabler that creates differentiated value propositions difficult for competitors to replicate.
The Divide Between AI Leaders and Stragglers
PwC’s 2025 AI Business Predictions emphasize that top-performing companies prioritize trust and transparency in their AI initiatives. Trust forms the bedrock for user adoption and ethical deployment, underpinning sustainable AI strategies. These leaders pair AI investments with robust data governance, enabling actionable insights that drive innovation and customer-centric offerings. For example, Southwest Airlines employs generative AI to modernize crew leave management, streamlining complex logistics and enhancing both operational efficiency and employee satisfaction.
Conversely, sectors such as manufacturing, agriculture, and hospitality exhibit slower AI adoption rates. As highlighted by TechTarget’s 2025 analysis, these industries often cite high upfront costs for AI infrastructure and a reliance on human judgment in unpredictable environments as significant barriers. This cautious stance risks ceding market share to AI-enabled competitors who leverage faster innovation cycles and greater operational agility.
McKinsey’s 2025 survey reinforces this disparity: while 72% of organizations deploy AI in at least one business function, only 1% consider their AI adoption mature. Notably, employee readiness is high, but leadership inertia hampers scaling. Without decisive leadership, companies risk relegating themselves to mere commodity users of generic AI tools instead of innovators shaping new market frontiers.
Technical and Organizational Differentiators of AI Frontrunners
Several critical factors distinguish AI leaders from lagging organizations:
-
Talent Acquisition and Development: Reports from Korn Ferry and SHRM reveal that leading companies prioritize AI-specific skills and invest heavily in continuous workforce upskilling. Utilizing AI-powered recruitment tools accelerates talent acquisition, while embedding cultural alignment enhances retention. Top organizations regard AI talent as a strategic asset integral to sustained innovation.
-
Infrastructure Investments: The demand for AI-driven computational capacity is immense. McKinsey projects that by 2030, data centers will require $6.7 trillion in capital expenditures to support AI workloads, with $5.2 trillion allocated explicitly to AI processing. Hyperscalers such as Microsoft, Alibaba, and Iliad are aggressively expanding proprietary AI compute resources, establishing formidable moats through scalable, efficient infrastructure.
-
Innovation Culture and Governance: Successful enterprises adopt a venture-capital approach to AI projects, funding multiple experiments and encouraging agile iteration. Leadership champions AI-driven transformation by instituting clear governance frameworks that foster organizational trust. Insights from HLTH underscore that embedding AI as a collaborative partner amplifies human creativity and decision-making rather than replacing it.
Microsoft’s deployment of AI tools like Microsoft 365 Copilot exemplifies this synergy—automating workflows to boost productivity while enhancing employee capabilities. This approach addresses ethical and trust concerns often associated with AI, fostering a balanced human-AI partnership.
Measuring Impact: Productivity Gains, Market Shifts, and Economic Outcomes
AI’s economic impact is substantial and unevenly distributed. PwC’s latest reports project AI adoption could add up to 15 percentage points to global GDP growth by 2035, contributing an estimated $15.7 trillion to the global economy by 2030. However, productivity gains are predominantly captured by early adopters.
-
Productivity: McKinsey reports that companies with mature AI deployments achieve notable cost reductions and efficiency improvements across functions such as IT, customer service, and R&D. Generative AI alone has the potential to lower R&D costs by 10–15%, accelerating innovation pipelines.
-
Market Share: Firms integrating AI deeply into customer insights and supply chain management—like Nestlé and Ralph Lauren—have enhanced inventory management and marketing precision, securing competitive advantages in volatile markets.
-
Economic Impact: PwC highlights that AI-driven productivity improvements could boost local economies’ GDP by up to 26%. These benefits, however, are contingent on responsible AI deployment and robust governance structures that cultivate trust and mitigate risks.
Bridging the AI Capability Gap
For organizations trailing in AI adoption, the risk is commoditization—merely using off-the-shelf AI tools without strategic integration yields diminishing returns. To close this gap, companies should:
-
Invest in Workforce Upskilling and Reskilling: With 87% of executives reporting skill gaps and fewer than half having active plans to address them, targeted training is essential. Upskilling ensures AI tools augment human expertise rather than replace it, supporting sustainable transformation.
-
Adopt Clear AI Governance and Usage Guidelines: Transparent communication about AI capabilities and limitations builds employee trust and sets realistic expectations. Establishing comprehensive governance empowers users to leverage AI responsibly and creatively.
-
Reevaluate Infrastructure and Data Strategy: Instead of retrofitting legacy systems, firms must optimize data architectures for AI readiness—prioritizing scalability, security, and privacy compliance to support advanced AI workloads.
-
Foster a Culture of Experimentation: Embracing agile, iterative AI development with active leadership sponsorship is critical. This cultural shift enables rapid learning, innovation, and adaptation necessary for AI maturity.
Final Thoughts
The next decade’s AI race transcends technology—it is a strategic contest defining industry leadership. Companies that combine robust data strategies, bold infrastructure investments, and a culture that embraces AI as an augmentative partner will emerge as market leaders.
In contrast, hesitation risks relegating companies to a commoditized middle ground where AI tools become mere cost centers rather than engines of growth. The evidence is clear: AI is reshaping competitive dynamics at an unprecedented pace. The imperative is no longer if businesses will adopt AI but how swiftly and thoughtfully they will integrate it to achieve lasting advantage.
Aspect | AI Leaders | Lagging Sectors |
---|---|---|
AI Integration | Embedded in data strategies, culture, and operations | Peripheral or slow adoption |
Trust & Transparency | Prioritized for sustainable AI initiatives | Often lacking or underdeveloped |
Data Governance | Robust, enabling actionable insights | Limited or insufficient |
Talent Acquisition & Development | Focus on AI skills, continuous upskilling | Less investment, skill gaps prevalent |
Infrastructure Investment | High capital expenditure on AI compute resources | Limited due to high upfront costs |
Innovation Culture | Venture-capital approach, agile iteration, leadership championing AI | Cautious, slower innovation cycles |
Employee Readiness | High readiness with leadership support | High readiness but leadership inertia |
Examples | Southwest Airlines (generative AI for crew management), Microsoft 365 Copilot | Manufacturing, agriculture, hospitality sectors |
Economic Impact | Significant productivity gains and market share growth | Risk of commoditization and losing market share |
Conclusion: Navigating Uncertainties and Embracing a Balanced AI Future in Business
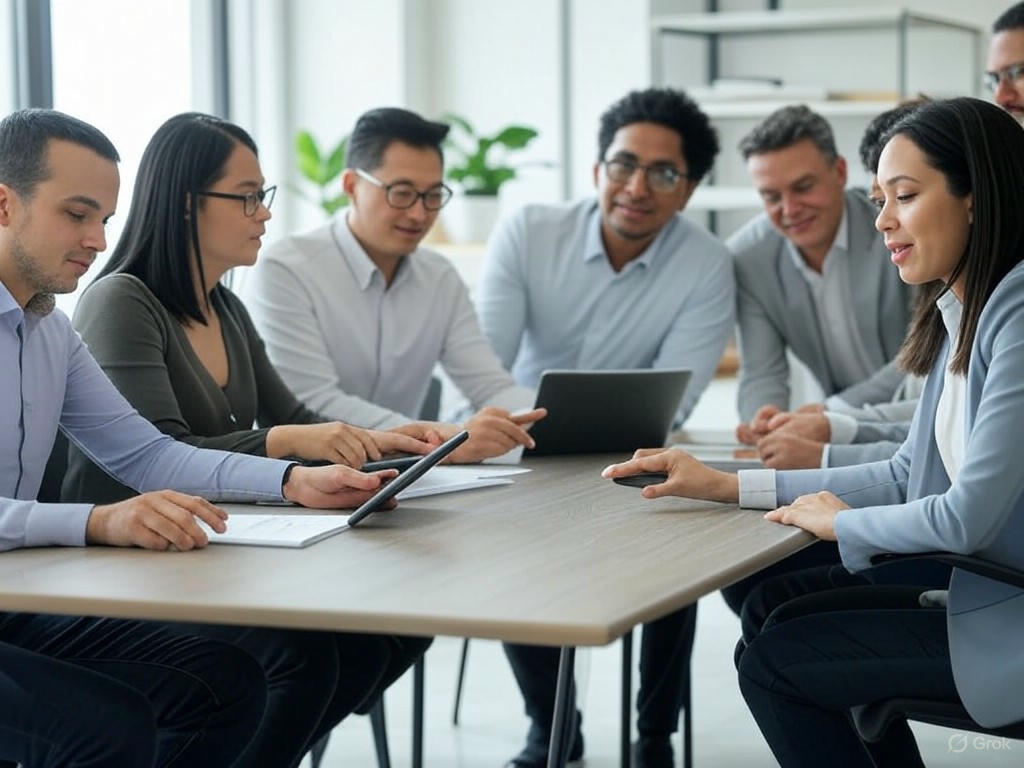
Conclusion: Navigating Uncertainties and Embracing a Balanced AI Future in Business
What if the story of AI in business over the next decade is not a linear march toward an idealized future but a nuanced journey through a landscape of profound opportunities and complex challenges? AI is far from a magic wand that guarantees growth—it is a powerful enabler that simultaneously introduces operational intricacies and ethical dilemmas.
AI as Both Catalyst and Challenge
McKinsey’s research reveals a striking paradox: while 78% of organizations deploy AI in at least one business function, only about 1% feel they have reached maturity in their AI adoption. The estimated $4.4 trillion long-term economic opportunity underscores AI’s vast potential, yet companies face a steep learning curve to realize this value fully.
Interestingly, employees often show greater readiness and enthusiasm for AI integration than their leadership anticipates, indicating a misalignment that could hinder progress if unaddressed. Real-world examples highlight AI’s transformative impact—from Microsoft 365 Copilot streamlining workflows at Volvo Group and Wells Fargo to the NFL Players Association utilizing AI for video reviews—demonstrating AI’s ability to automate routine tasks, boost productivity, and fuel innovation.
However, these successes coexist with significant challenges, including:
- Ethical concerns such as algorithmic bias and data privacy risks
- Talent shortages in data science and AI expertise, with 87% of executives reporting skill gaps
- Infrastructure limitations that complicate AI scaling and integration with legacy systems
- Risks of underperforming or misaligned AI implementations due to insufficient governance and skill development
Navigating these dualities requires business leaders to balance optimism with caution, recognizing that AI’s promise is inseparable from inherent risks.
The Fog of Uncertainty: Technological, Regulatory, and Societal Factors
AI’s trajectory over the next decade is shaped by multiple layers of uncertainty. Technological breakthroughs—such as advances in autonomous AI agents, multimodal models like Google’s Gemini, and edge AI hardware—could dramatically accelerate capabilities. Yet, equally influential are evolving regulatory environments and societal acceptance, which collectively determine the pace and manner of AI’s adoption.
Regulatory frameworks are rapidly developing but remain fragmented. The EU’s AI Act, effective from August 2027, introduces rigorous, risk-based rules demanding transparency, fairness, and accountability. Meanwhile, the U.S. features a decentralized, patchwork approach with varying state laws like California’s AI Transparency Act imposing penalties for non-compliance. This regulatory divergence poses compliance complexity for multinational enterprises but also signals a global shift toward mandatory AI governance.
Societal acceptance remains a wildcard. Studies from Pew Research and the Brookings Institution paint a complex picture of public sentiment—ranging from optimism about AI’s benefits to anxiety over job displacement, privacy, and fairness. Without public trust, AI adoption risks stalling, and missteps may provoke backlash that impedes progress.
Towards a Balanced, Adaptive AI Strategy
Given this multifaceted landscape, the path forward demands a balanced and adaptive AI strategy anchored in three foundational pillars:
-
Technical Rigor
Implement robust data governance frameworks, rigorous bias mitigation techniques, and continuous model performance evaluation. AI systems must be built on high-quality, ethically sourced data and subjected to thorough testing to avoid unintended outcomes and support regulatory compliance. -
Ethical Mindfulness
Treat ethics as a strategic imperative rather than a compliance afterthought. Organizations should embed transparency, fairness, and accountability into AI development and deployment processes. Establishing AI ethics boards and adhering to responsible AI frameworks, as advocated by PwC and McKinsey, are essential to build stakeholder trust and maintain legal compliance. -
Strategic Foresight
Cultivate leadership capable of anticipating technological advances, regulatory shifts, and societal expectations. Proactive adaptation, rather than reactive responses, ensures AI investments align with long-term business goals and societal values.
Practical implementation involves investing in workforce upskilling—leveraging AI-powered learning platforms like 360Learning and Cornerstone OnDemand—fostering cross-functional collaboration among technologists, ethicists, and business leaders, and adopting modular, extensible AI architectures that can evolve with changing conditions. Embracing adaptive AI agents, capable of learning and adjusting post-deployment, will be critical to sustainable growth and resilience.
Moving Forward: Embrace Complexity, Avoid Hype
The coming decade will require business leaders to cut through the AI hype with evidence-based analysis and pragmatic action. AI’s future is neither an unqualified boon nor an insurmountable threat; it is a complex journey demanding humility, vigilance, and a commitment to inclusivity.
By preparing for uncertainties and embracing a balanced approach—combining technical excellence, ethical stewardship, and strategic vision—businesses can unlock AI’s full potential. This will drive innovation and growth while safeguarding ethical standards and societal trust. Success in the AI era is not just good business—it is a defining responsibility of leadership.
Aspect | Details |
---|---|
AI Adoption Statistics | 78% of organizations deploy AI; only ~1% reached AI maturity |
Economic Opportunity | Estimated $4.4 trillion long-term potential |
Employee vs Leadership Readiness | Employees more ready and enthusiastic than leadership anticipates |
Real-World AI Examples | Microsoft 365 Copilot at Volvo & Wells Fargo; NFL Players Association for video review |
Challenges | Ethical concerns, talent shortages (87% report skill gaps), infrastructure limits, governance risks |
Regulatory Landscape | EU AI Act (Aug 2027); U.S. decentralized patchwork laws like California AI Transparency Act |
Societal Acceptance | Mixed public sentiment: optimism and anxiety on jobs, privacy, fairness |
Balanced AI Strategy Pillars | 1. Technical Rigor 2. Ethical Mindfulness 3. Strategic Foresight |
Implementation Approaches | Workforce upskilling, cross-functional collaboration, modular AI architectures, adaptive AI agents |
Leadership Imperative | Cut through hype; combine technical, ethical, strategic efforts to unlock AI potential |