Open-Source vs Commercial AI: Choosing the Right Tool for You
- Introduction: Why the Open-Source vs Commercial AI Debate Matters for Your Project
- Introduction: Why the Open-Source vs Commercial AI Debate Matters for Your Project
- Why This Debate Is More Than Just a Technical Choice
- Cutting Through the Hype with Evidence-Based Evaluation
- What to Expect Ahead
- Technical Foundations and Specifications of Open-Source and Commercial AI Tools
- Technical Foundations and Specifications of Open-Source and Commercial AI Tools
- Architecture Types, Model Sizes, and Training Data Scope
- Hardware Requirements: Deployment and Fine-Tuning Demands
- Software Capabilities: Customization, API Access, and Ecosystem Maturity
- Model Transparency, Update Cadence, and Vendor Support
- Performance Benchmarks and Evaluation Metrics: Accuracy, Latency, and Scalability
- Performance Benchmarks and Evaluation Metrics: Accuracy, Latency, and Scalability
- Accuracy: How Do Open-Source and Commercial AI Tools Stack Up?
- Latency and Throughput: Meeting Real-World Demand
- Scalability: Fine-Tuning, Robustness, and Customization at Scale
- Quantitative Insights: Benchmark Highlights
- Final Thoughts
- Security, Privacy, and Regulatory Compliance Challenges
- Security, Privacy, and Regulatory Compliance Challenges
- Data Security and Privacy Safeguards: Open-Source vs. Commercial
- Navigating Compliance: GDPR, HIPAA, and Beyond
- Transparency, Auditability, and the Trade-Off Between Control and Convenience
- Industry-Specific Regulatory Impacts on AI Tool Choice
- Key Takeaways
- Practical Use Cases and Industry-Specific Implications
- Practical Use Cases and Industry-Specific Implications
- Organizational Choices: Scale, Specificity, and Resources
- Sector-Specific Nuances: Healthcare, Finance, and Technology
- Startups vs. Enterprises: Strategic Advantages and ROI
- Case Studies: Successes and Challenges in Implementation
- Balancing Act: The Hybrid Approach
- Final Thoughts
- Comparative Analysis: Strengths, Limitations, and Hybrid Approaches
- Comparative Analysis: Strengths, Limitations, and Hybrid Approaches
- The Power and Pitfalls of Open-Source AI
- Commercial AI Solutions: Convenience and Control
- Hybrid AI Models: Blending the Best of Both Worlds
- Decision Checklist: Choosing the Right Path for Your Project
- Final Thoughts
- Conclusion and Recommendations: Making an Evidence-Based Choice for Your AI Project
- Conclusion and Recommendations: Making an Evidence-Based Choice for Your AI Project
- Aligning AI Tool Choice with Your Project’s Unique Needs
- Pragmatic Evaluation: Total Cost of Ownership, Scalability, and Sustainability
- Staying Ahead: Continuous Monitoring and Ethical Stewardship
- Key Takeaways

Introduction: Why the Open-Source vs Commercial AI Debate Matters for Your Project
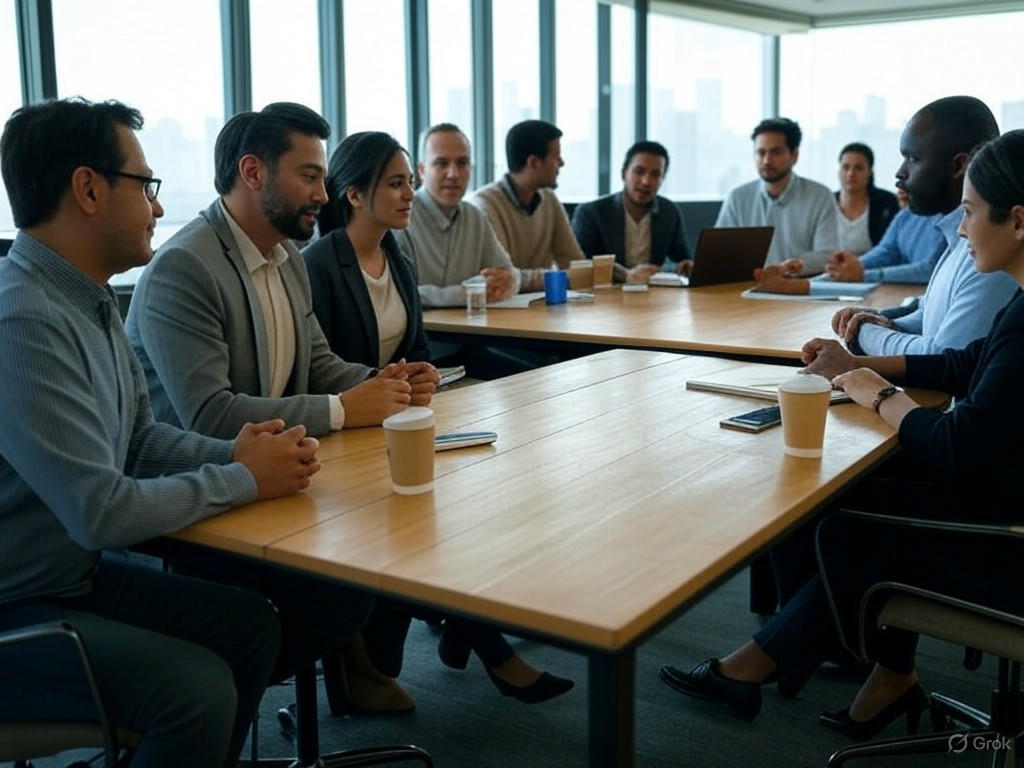
Introduction: Why the Open-Source vs Commercial AI Debate Matters for Your Project
Artificial intelligence has evolved from a niche research topic to a fundamental driver reshaping industries worldwide. By 2025, McKinsey estimates the AI opportunity to reach a staggering $4.4 trillion, with nearly every company investing in AI tools to some extent. Despite this widespread adoption, only about 1% of organizations consider themselves mature in AI deployment—a critical gap revealing challenges in harnessing AI’s full potential without succumbing to hype or misaligned expectations.
A pivotal decision in AI adoption is choosing between open-source and commercial AI solutions. These paths diverge fundamentally in philosophy, accessibility, cost structure, control, and support. Open-source AI offers transparency, customization, and community-driven innovation, enabling teams to tailor AI systems tightly to their unique data and workflows. Commercial solutions promise turnkey usability, vendor support, and often faster time-to-value, bundling advanced capabilities such as multimodal reasoning and integrated cloud services. Selecting the right approach is essential for your project’s success.
Why This Debate Is More Than Just a Technical Choice
The choice isn’t simply “which AI tool is better?” but rather “which approach aligns best with your project’s specific context and constraints?” Key dimensions where open-source and commercial AI diverge include:
-
Innovation and Customization: Open-source models empower organizations to innovate rapidly by fine-tuning AI on proprietary datasets, adapting architectures, or integrating novel features. For example, Meta’s Llama 4 series employs a Mixture-of-Experts (MoE) architecture, allowing scalable, specialized intelligence that can be customized extensively. Commercial platforms often provide bundled advanced features that accelerate deployment but limit deep customization.
-
Cost and Resource Allocation: Open-source AI eliminates licensing fees and supports incremental scaling. However, it shifts infrastructure management, training, compliance, and security responsibilities onto your team. Commercial solutions exchange upfront and ongoing fees for managed services, professional support, and simplified operational overhead.
-
Performance and Reliability: Commercial AI products undergo rigorous testing and optimization, delivering robust performance for standard use cases. Open-source tools may require more engineering effort to achieve comparable reliability but can outperform when fine-tuned for domain-specific needs, as seen in specialized healthcare or finance applications.
-
Security and Compliance: Open-source transparency facilitates thorough audits critical for regulated industries, but demands internal expertise to manage risks like data privacy and adversarial attacks. Commercial vendors embed compliance features and security protocols, yet may obscure proprietary algorithms, limiting auditability and data sovereignty.
-
Ethical Considerations: Ethical AI deployment requires transparency, fairness, and accountability. Open-source communities foster collaborative scrutiny and iterative improvement, while commercial vendors assume responsibility for ethical safeguards but often restrict visibility into inner workings. Frameworks like the EU AI Act and organizations such as the Montreal AI Ethics Institute emphasize these principles across sectors.
Cutting Through the Hype with Evidence-Based Evaluation
The AI ecosystem in 2025 is saturated with dazzling new tools and bold promises. From ChatGPT variants and Meta’s Llama 4 to emerging open-source frameworks like Stability AI’s Stable Diffusion, the choices can overwhelm decision-makers. Yet, as experience in AI architecture shows, enthusiasm must be tempered with critical analysis:
-
Real-World Adoption Is Uneven: While generative AI boasts hundreds of millions of users, only a small fraction of organizations have formally integrated it into core operations, reflecting the complexity of sustainable deployment.
-
Workforce Readiness Differs from Leadership Expectation: McKinsey’s research reveals that employees are generally more prepared and eager to adopt AI than leadership anticipates, underscoring the need for transparent communication and training.
-
Investment vs. ROI Matters: Developing or integrating AI can range from tens of thousands of dollars for pilots to millions for large-scale systems. Strategic budgeting and clear KPIs aligned with business goals are essential to justify investments.
This article provides a grounded, nuanced framework to navigate these choices. We analyze both open-source and commercial AI options across technical performance, strategic fit, and societal implications. By understanding trade-offs with concrete examples and data, you will be equipped to select AI tools that serve your project’s goals instead of chasing the latest buzz.
What to Expect Ahead
In the following sections, we will explore:
-
How open-source AI democratizes innovation but requires savvy governance and skilled infrastructure management to mitigate risks.
-
The strengths and limitations of commercial AI platforms in delivering rapid, enterprise-grade, and compliant solutions.
-
Cost-benefit considerations and how to align AI investments with your project’s scale, expertise, and long-term strategy.
-
Security, compliance, and ethical frameworks critical to trustworthy AI deployment, including adherence to regulations like GDPR and the EU AI Act.
-
Broader societal impacts such as workforce transformation, ethical AI governance, and the imperative of transparency and accountability.
Making an informed, context-driven AI choice is not merely a technical decision—it is a strategic and ethical imperative. While the stakes are high, so too are the opportunities when decisions are grounded in evidence and clarity rather than hype.
Dimension | Open-Source AI | Commercial AI |
---|---|---|
Innovation and Customization | Enables rapid innovation, fine-tuning on proprietary data, adaptable architectures (e.g., Meta’s Llama 4 MoE) | Provides bundled advanced features, faster deployment but limited deep customization |
Cost and Resource Allocation | No licensing fees, supports incremental scaling, but requires managing infrastructure, training, compliance, and security internally | Upfront and ongoing fees for managed services, professional support, and simplified operations |
Performance and Reliability | Requires engineering effort for reliability but can outperform in domain-specific needs (e.g., healthcare, finance) | Rigorously tested and optimized for robust, standard use case performance |
Security and Compliance | Transparent for thorough audits; demands internal expertise for data privacy and risk management | Built-in compliance and security protocols; proprietary algorithms limit auditability and data sovereignty |
Ethical Considerations | Community scrutiny fosters transparency, fairness, accountability; supports iterative improvement | Vendors assume ethical safeguards but restrict visibility into inner workings |
Technical Foundations and Specifications of Open-Source and Commercial AI Tools
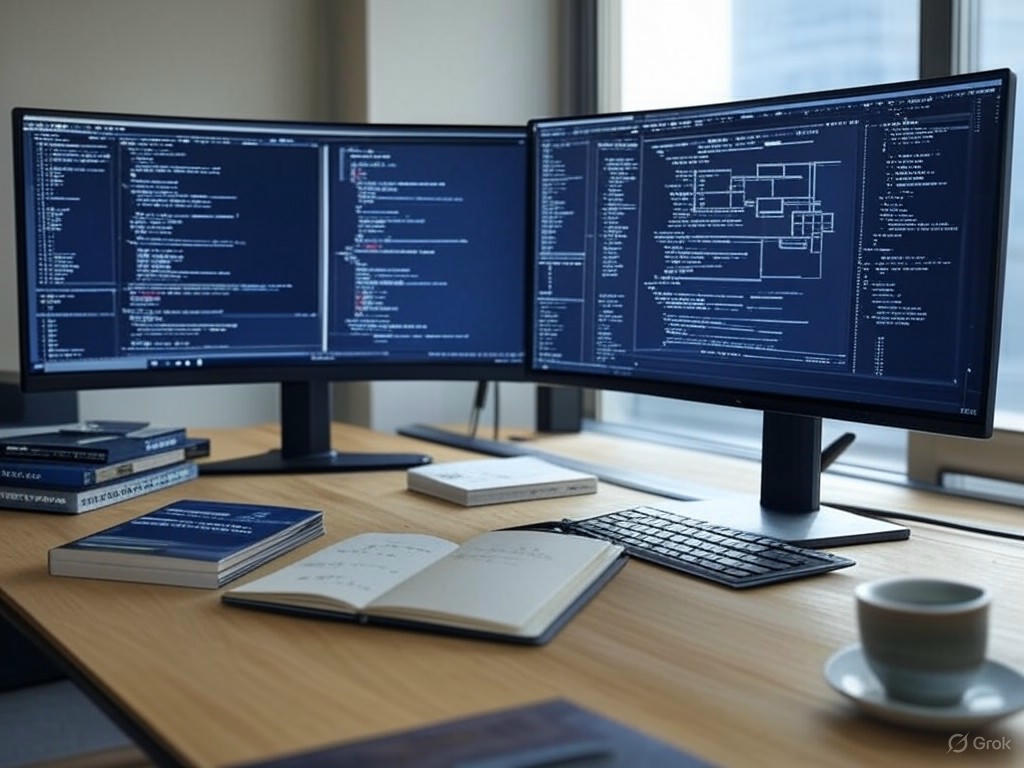
Technical Foundations and Specifications of Open-Source and Commercial AI Tools
What fundamentally distinguishes open-source AI tools such as Meta’s Llama 4 series or Stability AI’s Stable Diffusion from commercial platforms like OpenAI’s GPT-4 or Google Cloud AI lies beneath the surface—in their architectural design, licensing models, hardware requirements, and software ecosystems. Grasping these technical foundations is essential for project leaders aiming to align AI adoption with their unique organizational needs.
Architecture Types, Model Sizes, and Training Data Scope
Open-source AI models have dramatically advanced, challenging the perception that only proprietary solutions can deliver cutting-edge performance. Meta’s Llama 4 series exemplifies this progress with its Mixture-of-Experts (MoE) architecture. In this design, multiple specialized “experts” focus on distinct data patterns, enabling a 288-billion-parameter model with 16 experts—Llama 4 Maverick—that scales intelligence efficiently while managing compute costs effectively. Moreover, Llama 4 is natively multimodal, capable of processing text, images, and video frames through early fusion techniques that blend these inputs seamlessly. This modular specialization marks a shift from earlier dense transformer models like Llama 3, which primarily scaled by increasing parameter counts without such architectural nuance.
Commercial AI platforms also push boundaries with massive dense Transformer architectures. OpenAI’s GPT-4 supports multimodal inputs and handles context windows exceeding 25,000 words, providing extensive language understanding and generation capabilities. Google Cloud’s Gemini models leverage sophisticated Transformer backbones enhanced by deep integration with Google’s data ecosystems and hardware accelerators, notably their latest Ironwood TPUs, which deliver nearly 30 times the efficiency of original TPU generations.
Regarding training data, commercial platforms typically access vast, proprietary datasets, continuously curated for safety, relevance, and multilingual coverage. Open-source models often rely on publicly available corpora or collaborative partnerships, yet recent Meta releases exhibit significant improvements in multilingual and multimodal training scopes, narrowing the gap in data quality and diversity.
Licensing models serve as a critical distinction. Open-source AI tools like Llama 4 and Stable Diffusion adopt permissive licenses—such as CreativeML OpenRAIL-M for Stable Diffusion—allowing users to freely use, modify, and redistribute the models under responsible use clauses. This openness fosters community-driven innovation but places compliance and risk management squarely on users. Conversely, commercial AI operates under strict API usage agreements with controlled access, offering users clearer legal terms and vendor-managed compliance at the expense of customization freedom.
Hardware Requirements: Deployment and Fine-Tuning Demands
Hardware considerations significantly influence the feasibility of deploying sophisticated AI models, especially for open-source tools where infrastructure responsibilities fall on the user.
For example, deploying larger Llama 3 variants requires GPUs with at least 16GB VRAM for smaller models, scaling up to over 160GB VRAM for the 70-billion-parameter version. This typically involves multi-GPU setups using NVIDIA A100 80GB cards. Fine-tuning these models—requiring backpropagation and gradient computation—adds complexity. Techniques like QLoRA (Quantized Low-Rank Adaptation) have emerged to dramatically reduce memory footprints to between 9 and 14GB VRAM, enabling fine-tuning on consumer-grade GPUs or cloud instances with less overhead.
Llama 4, with its MoE layers and extended context windows up to 10 million tokens, demands specialized compute environments such as the Bizon ZX5500 workstation, featuring multiple high-end GPUs and advanced cooling systems. Its use of 8-bit floating point (FP8) precision optimizes throughput and memory efficiency—doubling performance compared to 16-bit floating point models on comparable hardware.
In contrast, commercial AI platforms like OpenAI and Azure OpenAI abstract these hardware challenges. Users interact via APIs without managing VRAM or GPU configurations. This accessibility comes with operational constraints such as usage quotas and rate limits—for instance, Azure OpenAI enforces tokens-per-minute and requests-per-minute caps. Microsoft’s Azure also offers regional deployment options and fine-tuning capabilities in select regions, balancing flexibility with managed service reliability.
Software Capabilities: Customization, API Access, and Ecosystem Maturity
Open-source AI tools excel in customization and flexibility. With open access to source code and pretrained model weights, developers can fine-tune models on proprietary datasets, modify architectures, and integrate novel functionalities. For instance, Llama 4’s open weights empower organizations to build tailored AI assistants that comply with specific regulatory or domain requirements. Similarly, Stable Diffusion’s modular design and CreativeML OpenRAIL-M license facilitate custom image generation styles and integration of control mechanisms like ControlNet for fine-grained output management.
However, this flexibility demands considerable technical expertise, infrastructure investment, and ongoing maintenance—barriers that smaller teams or organizations with limited AI experience may find challenging.
Commercial AI platforms prioritize ease of integration and ecosystem maturity. OpenAI’s GPT-4 API provides straightforward access complemented by extensive documentation, SDKs, and industry-specific tooling, supporting applications from customer support to software development. Google Cloud’s Vertex AI ecosystem offers pre-built models, deployment frameworks, and an Agent Development Kit leveraging the Model Context Protocol (MCP) to enable seamless integration of data and tools across platforms like Notion, Google Workspace, and Slack.
Both ecosystems evolve rapidly. Open-source AI benefits from vibrant communities driving frequent updates and innovations, though release schedules may be less predictable. Commercial vendors deliver regular, managed updates with robust support and service-level agreements (SLAs), critical for enterprise-grade reliability and compliance.
Model Transparency, Update Cadence, and Vendor Support
Transparency is a notable strength of open-source AI. Full access to model weights, training data descriptions, and development processes allows users to audit models for ethical compliance, bias mitigation, and alignment with organizational values. Meta’s Llama 4, for example, is accompanied by a Developer Use Guide emphasizing AI safety and responsible deployment, reflecting community and corporate commitments to ethical AI.
Commercial AI models often function as black boxes, with limited transparency into proprietary training data or internal architectures. OpenAI’s Model Spec initiative represents progress toward disclosing intended model behaviors and safety guidelines, but users must still rely on vendor trust and certifications.
Update cadence favors commercial platforms with predictable, frequent releases and performance optimizations, delivered under SLAs. Open-source projects rely on community contributions and corporate sponsorships, resulting in variable update schedules that may lag behind commercial releases.
Vendor support is another differentiator. Commercial AI providers supply dedicated support teams, integration assistance, and compliance certifications—essential for mission-critical deployments. Open-source AI users typically depend on community forums, third-party consultants, or internal AI expertise, which may introduce support variability.
In summary, the technical landscape between open-source and commercial AI tools reflects a fundamental trade-off: open-source offers unparalleled control, transparency, and customization at the cost of requiring significant technical and hardware investments. Commercial platforms provide turnkey access to advanced AI capabilities with robust vendor support and managed infrastructure, but impose constraints on customization, transparency, and potential vendor lock-in.
Selecting the right approach demands a careful assessment of your project’s technical requirements, compliance obligations, budget, and long-term AI strategy. Neither path is inherently superior; rather, they represent complementary options empowering organizations to harness AI’s transformative potential responsibly and effectively.
Aspect | Open-Source AI Tools | Commercial AI Platforms |
---|---|---|
Examples | Meta Llama 4 series, Stability AI Stable Diffusion | OpenAI GPT-4, Google Cloud AI Gemini |
Architecture | Mixture-of-Experts (MoE), modular specialization, multimodal early fusion | Massive dense Transformer, multimodal, integrated with proprietary data and hardware accelerators (e.g., Ironwood TPUs) |
Model Size | Up to 288 billion parameters with specialized experts (Llama 4 Maverick) | Large-scale models with extensive context windows (e.g., GPT-4 >25,000 words) |
Training Data | Publicly available corpora, collaborative partnerships, improving multilingual/multimodal scope | Vast proprietary datasets, continuously curated for safety, relevance, multilingual coverage |
Licensing | Permissive licenses (e.g., CreativeML OpenRAIL-M), user responsible for compliance | Strict API usage agreements, controlled access, vendor-managed compliance |
Hardware Requirements | High VRAM GPUs (16GB to 160GB+), multi-GPU setups, specialized workstations (e.g., Bizon ZX5500), QLoRA for fine-tuning on consumer GPUs | Abstracted hardware via APIs, no user management of VRAM or GPUs, subject to usage quotas and rate limits |
Fine-Tuning | Possible with source code and weights; requires substantial compute and expertise | Available in select regions with managed services and support |
Software Customization | Full access to code and weights, enables tailored models and integration of novel features | APIs with extensive documentation, SDKs, industry tooling, easier integration |
Ecosystem Maturity | Vibrant, community-driven updates, less predictable release schedules | Regular managed updates, robust support, SLAs for enterprise reliability |
Transparency | Full access to model weights and training data descriptions, ethical guidelines available | Limited transparency, black-box models, ongoing initiatives for model behavior disclosure |
Update Cadence | Variable, community and corporate sponsored | Frequent, predictable, SLA-backed |
Vendor Support | Community forums, third-party consultants, internal expertise needed | Dedicated support teams, integration assistance, compliance certifications |
Performance Benchmarks and Evaluation Metrics: Accuracy, Latency, and Scalability
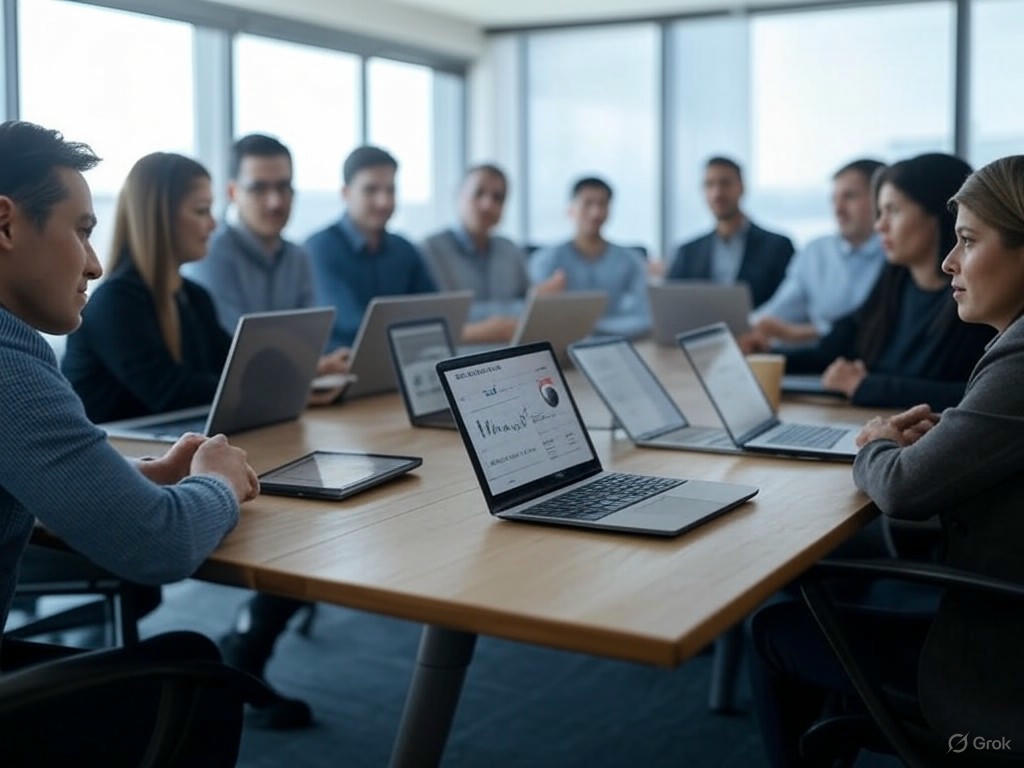
Performance Benchmarks and Evaluation Metrics: Accuracy, Latency, and Scalability
Accuracy: How Do Open-Source and Commercial AI Tools Stack Up?
The competition between open-source and commercial AI solutions on raw accuracy is closer than many anticipate. For example, the open-source Mistral Small 3 model, licensed under Apache 2.0, achieves impressive throughput—processing roughly 150 tokens per second, which is over three times faster than Llama 3.3 70B when run on identical hardware. In contrast, commercial leaders like OpenAI’s GPT series and Google’s Gemma 3, which supports context windows up to 128,000 tokens, continue to lead in advanced language understanding and generation capabilities.
Standardized benchmarks such as GLUE and MLPerf confirm that commercial AI models maintain a slight edge in specialized NLP tasks and multimodal integrations. Nevertheless, open-source models are rapidly narrowing this gap, especially when combined with community-driven fine-tuning and optimizations. This dynamic is reflected in hybrid deployment strategies, where organizations leverage open-source tools for customization and control, while relying on commercial APIs for convenience and reliability.
In computer vision, similar patterns emerge. Proprietary solutions often provide highly optimized pipelines tailored to specific industry applications, like real-time object detection with YOLO variants or infrastructure inspection powered by specialized models. However, open-source frameworks such as Stable Diffusion XL (SDXL) and other diffusion models have demonstrated remarkable generative prowess, at times surpassing commercial counterparts in adaptability and extensibility.
It is important to recognize that benchmark scores only capture part of the performance story. Real-world robustness and generalization to diverse, out-of-distribution datasets remain challenging. For instance, some open-source models show greater variability when exposed to unfamiliar data, whereas commercial vendors often mitigate such risks through extensive proprietary data curation and continuous model refinement.
Latency and Throughput: Meeting Real-World Demand
While accuracy is fundamental, production environments prioritize latency and throughput as key determinants of user experience and system viability. Open-source models deployed on optimized local or private infrastructure can deliver outstanding performance. For instance, Intel’s 6th-gen Xeon CPUs and the latest NVIDIA GPUs have enabled systems achieving more than 800 tokens per second on very large models like Llama 3.1 405B, as measured by MLPerf Inference v5.0.
However, open-source deployments often grapple with “cold start” latency—delays when loading models after periods of inactivity or at startup. This latency is more pronounced in private setups due to explicit resource allocation requirements. In contrast, commercial cloud APIs amortize startup costs across many users, offering near-zero startup latency. For latency-critical use cases, edge AI deployments favor compact or quantized models to minimize inference delays.
Commercial AI services typically provide highly optimized infrastructure featuring load balancing and auto-scaling, ensuring consistent latency under concurrent multi-user loads. This reliability comes with trade-offs, including vendor lock-in and potentially escalating API usage fees at scale.
Tools like Apache JMeter and Gatling remain essential for enterprises to conduct load testing and evaluate AI system responsiveness under realistic demand scenarios. Early consideration of these performance metrics during architecture design is crucial to avoid costly downtime and degraded user experience.
Scalability: Fine-Tuning, Robustness, and Customization at Scale
Scalability in AI encompasses more than just hardware capacity—it includes how models adapt to evolving data, increasing user volumes, and growing task complexity. Fine-tuning techniques have become central to achieving such adaptability. Parameter-Efficient Fine-Tuning (PEFT) methods, including LoRA and QLoRA, enable substantial customization with reduced memory and compute overhead. These approaches make it feasible to tailor large models on modest GPU clusters or hybrid cloud/on-premises infrastructures.
Open-source models uniquely offer extensive control and flexibility, allowing enterprises to fine-tune AI systems for niche domains or to meet regulatory requirements. For example, financial institutions use on-premises fine-tuning to protect sensitive data while leveraging state-of-the-art architectures. Conversely, commercial providers often limit fine-tuning capabilities, favoring prompt engineering or constrained customization via APIs.
Robustness and generalization remain critical scalability indicators. Commercial AI platforms benefit from large-scale proprietary datasets and continuous retraining pipelines, enhancing model stability. Meanwhile, open-source communities increasingly contribute diverse datasets and validation benchmarks to improve robustness. Nevertheless, performance variability can arise due to differences in deployment environments, hardware accelerators, and software stacks; an AI model optimized for cloud TPU clusters may underperform on local GPU hardware without careful tuning.
As AI models grow in complexity, infrastructure scalability becomes a bottleneck. Emerging server technologies such as PCIe Gen5/6, Compute Express Link (CXL) memory expansion, and high-throughput networking are vital to sustain multi-user, low-latency deployments. Organizations must evaluate the total cost of ownership when deciding between investing in cutting-edge infrastructure or leveraging commercial cloud AI services.
Quantitative Insights: Benchmark Highlights
Metric | Open-Source Example | Commercial Example | Notes |
---|---|---|---|
Tokens/sec (NLP) | Mistral Small 3: ~150 TPS | CoreWeave Llama 3.1 405B: 800 TPS | Commercial models excel in raw throughput on large-scale hardware |
Context Window Size | Llama 3.3: ~8,192 tokens | Google Gemma 3: 128,000 tokens | Larger context windows facilitate long-form language tasks |
Fine-Tuning Time (6B model) | ~1 hour on single A100 GPU | Limited or no fine-tuning on API models | Open-source enables deeper model customization |
Real-World Latency | Cold start delays in private deployments | Near-zero startup latency via managed APIs | Latency-sensitive applications may favor commercial platforms |
Final Thoughts
Selecting between open-source and commercial AI tools requires a nuanced understanding of performance metrics including accuracy, latency, and scalability. Open-source AI shines in customization, cost control, and scalability but demands substantial in-house expertise, infrastructure investment, and governance to match commercial-grade latency and robustness.
Commercial AI solutions provide turnkey performance with low latency and seamless scaling, supported by dedicated security teams and compliance assurances. However, these benefits come at the cost of reduced flexibility, potential vendor lock-in, and ongoing usage expenses.
Hybrid strategies are increasingly prevalent, allowing organizations to optimize workloads by deploying open-source models on-premises or at the edge for sensitive or high-volume tasks, while utilizing commercial cloud services for generic, bursty workloads.
Ultimately, the right choice depends on your project’s specific requirements, resource availability, and strategic priorities. Rigorous benchmarking and environment-specific evaluation remain indispensable to ensure AI deployments deliver meaningful, reliable outcomes in 2025 and beyond.
Metric | Open-Source Example | Commercial Example | Notes |
---|---|---|---|
Tokens/sec (NLP) | Mistral Small 3: ~150 TPS | CoreWeave Llama 3.1 405B: 800 TPS | Commercial models excel in raw throughput on large-scale hardware |
Context Window Size | Llama 3.3: ~8,192 tokens | Google Gemma 3: 128,000 tokens | Larger context windows facilitate long-form language tasks |
Fine-Tuning Time (6B model) | ~1 hour on single A100 GPU | Limited or no fine-tuning on API models | Open-source enables deeper model customization |
Real-World Latency | Cold start delays in private deployments | Near-zero startup latency via managed APIs | Latency-sensitive applications may favor commercial platforms |
Security, Privacy, and Regulatory Compliance Challenges
Security, Privacy, and Regulatory Compliance Challenges
How secure is your AI tool of choice? Whether you favor open-source frameworks or commercial AI solutions, data security and privacy concerns have never been more critical. AI’s insatiable demand for data, paired with increasingly sophisticated adversarial attacks, means selecting the right tool goes beyond performance metrics—it requires a deep understanding of security risks and regulatory requirements.
Data Security and Privacy Safeguards: Open-Source vs. Commercial
Open-source AI tools power approximately 97% of applications worldwide, underscoring their ubiquity and adaptability. Yet, their security often hinges on volunteer-maintained ecosystems, which can be fragile. For instance, the notorious xz Utils backdoor attack highlighted how a single compromised maintainer can introduce supply chain vulnerabilities that affect countless downstream projects. This risk is compounded by the emergence of AI-generated fake identities used by attackers to infiltrate trusted communities and manipulate open-source repositories.
In contrast, commercial AI offerings typically include dedicated security teams and enterprise-grade protections. Vendor Service Level Agreements (SLAs) often guarantee prompt patching of vulnerabilities and robust data encryption protocols. However, these controlled environments are not infallible. They may obscure operational details, raising valid concerns about transparency and the auditability of models and data flows.
Both open-source and commercial AI tools face distinct challenges from adversarial attacks—malicious inputs crafted to deceive AI models. The National Institute of Standards and Technology (NIST) finalized guidelines categorizing these threats and recommending mitigation strategies, though the landscape remains dynamic. Open-source projects benefit from extensive community scrutiny, accelerating vulnerability detection, but often lack rapid incident response capabilities. Commercial vendors implement advanced protections and swift responses but may limit customer visibility into internal defenses.
Navigating Compliance: GDPR, HIPAA, and Beyond
Compliance with data protection regulations like the European Union’s GDPR and the U.S. HIPAA is a decisive factor in AI tool selection, especially in sectors such as healthcare, finance, and any industry handling sensitive personal data. The European Data Protection Board’s Opinion 28/2024 emphasizes that organizations must demonstrate the necessity of personal data processing and ensure that legitimate interests do not override fundamental rights. This makes due diligence on AI providers’ data protection compliance an absolute necessity.
In the United States, HIPAA’s 2025 updates intensify requirements for safeguarding Protected Health Information (PHI), compelling healthcare providers and vendors to strengthen security policies. Although AI-specific HIPAA rules have not yet been issued, regulatory guidance signals increasing scrutiny over AI’s role in managing patient data. Vendors failing to meet these evolving standards risk legal penalties and, critically, erosion of trust in AI-powered healthcare solutions.
Enterprise-grade AI compliance platforms have emerged as essential tools. Solutions like SAS Viya and Wiz AI-SPM offer real-time monitoring, automated risk assessments, and workflow automation tailored to complex regulatory frameworks. These platforms help organizations map AI compliance to sector-specific laws while maintaining operational resilience. Yet, surveys indicate that fewer than half of organizations feel confident in their ability to fully comply with AI-related regulations, revealing a significant compliance gap that must be addressed.
Transparency, Auditability, and the Trade-Off Between Control and Convenience
Open-source AI’s greatest strength lies in its transparency. The ability to audit codebases, data pipelines, and model behavior provides a crucial advantage when handling sensitive information. Organizations can customize security protocols, host models on-premises, and maintain strict control over data flows—minimizing exposure to third-party risks and regulatory pitfalls such as those posed by the U.S. Cloud Act.
However, this level of control comes at a cost. Deploying and securing open-source models demands substantial in-house expertise and infrastructure investment. It can also be financially burdensome and operationally complex, especially at scale. Commercial AI solutions offer convenience through managed services, contractual data protections, and vendor accountability, but often at the expense of relinquishing some control and visibility.
Google’s approach exemplifies this trade-off: consumer-facing AI services extensively leverage user data, while enterprise offerings contractually commit not to use client data beyond the service scope. This segmentation illustrates the growing differentiation between free, convenience-focused AI tools and paid, privacy-conscious commercial products.
Industry-Specific Regulatory Impacts on AI Tool Choice
Regulatory pressures heavily shape AI tool adoption in regulated industries. Consider these examples:
-
Healthcare: The California Health Care Services AI Act mandates clear disclosure of generative AI use in patient communications. Compliance with HIPAA’s enhanced 2025 requirements further restricts data handling practices. Healthcare providers often choose commercial AI platforms with certified compliance or opt for self-hosted open-source models to maintain data sovereignty and transparency.
-
Finance: Financial institutions operate under layered regulations emphasizing fairness, transparency, and data security. AI tools must integrate with existing risk management frameworks and support auditability mandates. Vendor SLAs and AI compliance platforms help mitigate operational and legal risks in this tightly regulated environment.
-
Public Safety: Deployments in real-time threat detection or emergency dispatch demand stringent controls to protect sensitive citizen data and ensure system integrity. Transparency, robustness against adversarial manipulation, and rapid incident response capabilities are essential.
Across these sectors, organizations must balance the convenience of managed commercial AI services with the granular control offered by open-source models. The “AI Data Trade-Off” ultimately involves weighing privacy and security against operational efficiency and innovation velocity.
Key Takeaways
-
Open-source AI tools provide unparalleled transparency and control but require significant investment in security governance and infrastructure to manage supply chain vulnerabilities and adversarial threats.
-
Commercial AI solutions deliver enterprise-grade security protocols, compliance assurances via SLAs, and dedicated support but may limit auditability, data sovereignty, and operational transparency.
-
Regulatory compliance with frameworks such as GDPR and HIPAA is a non-negotiable factor influencing AI tool selection, especially in sensitive sectors like healthcare and finance.
-
A hybrid approach—combining open-source transparency with commercial security and support—can often strike the optimal balance between control and convenience.
-
Proactive AI governance is critical: organizations must implement rigorous oversight policies, conduct thorough vendor due diligence, and continuously monitor compliance to navigate the rapidly evolving security and regulatory landscape.
Ultimately, choosing between open-source and commercial AI tools is a strategic decision that extends beyond technical capabilities. It demands careful consideration of your project’s risk tolerance, compliance obligations, and long-term trustworthiness objectives.
Aspect | Open-Source AI | Commercial AI |
---|---|---|
Security Model | Volunteer-maintained ecosystems; vulnerable to supply chain attacks (e.g., xz Utils backdoor); extensive community scrutiny but slower incident response | Dedicated security teams; enterprise-grade protections; rapid patching and incident response; potential lack of transparency |
Data Privacy | Full transparency and auditability; customizable security protocols; on-premises hosting reduces third-party risks | Contractual data protections; vendor accountability; possible limitations on data visibility and sovereignty |
Regulatory Compliance | Requires substantial in-house expertise to meet GDPR, HIPAA, and industry regulations; benefits from transparency for audits | Often includes compliance assurances via SLAs and specialized AI compliance platforms; helps navigate complex regulations |
Adversarial Attack Mitigation | Community-driven vulnerability detection; limited rapid response capabilities | Advanced protections; swift incident response; less customer visibility into defenses |
Operational Complexity | High; needs significant infrastructure and expertise investment | Lower; managed services provide convenience but reduce control |
Transparency & Auditability | High transparency; full code and model auditability | Limited transparency; auditability may be restricted by vendor policies |
Industry Use Cases | Preferred for data sovereignty in healthcare; used where transparency is critical | Favored in finance, healthcare, and public safety for compliance and support |
Trade-Off | Maximizes control and customization at cost of complexity and risk management burden | Maximizes convenience and support at cost of reduced control and potential data exposure |
Practical Use Cases and Industry-Specific Implications
Practical Use Cases and Industry-Specific Implications
How do organizations navigate the choice between open-source AI tools and commercial solutions, each offering distinct benefits and inherent risks? The decision fundamentally depends on factors such as project scale, domain specificity, and resource availability. A clear understanding of these real-world trade-offs explains why startups often embrace open-source AI frameworks for agility and innovation, while large enterprises typically prefer commercial platforms for reliability, support, and compliance.
Organizational Choices: Scale, Specificity, and Resources
Startups and small businesses frequently adopt open-source AI tools like PyTorch, TensorFlow, and LangChain. These frameworks come with no licensing fees and foster rapid experimentation, enabling resource-constrained teams to build and customize models that align closely with their unique data and objectives. For example, early-stage startups developing niche AI applications—such as personalized recommendation engines or specialized natural language processing (NLP) services—leverage open-source flexibility to iterate quickly without vendor lock-in.
In contrast, large enterprises are statistically twice as likely to deploy AI technologies (ExplodingTopics, 2025). These organizations prioritize commercial AI solutions for their enterprise-grade security, dedicated support teams, and seamless integration with existing systems. Financial institutions and healthcare providers, for instance, often require vendor certifications, compliance guarantees, and service-level agreements (SLAs) that commercial offerings provide. While the upfront costs are higher, the reduced operational risk and accelerated time-to-value justify the investment.
Resource availability is also paramount. Companies with in-house AI expertise and robust infrastructure confidently adopt open-source AI models, sometimes contributing improvements back to the community. Conversely, organizations lacking deep AI talent or operating under strict regulatory scrutiny tend to prefer commercial solutions, which bundle expertise, compliance features, and ongoing maintenance, reducing the burden on internal teams.
Sector-Specific Nuances: Healthcare, Finance, and Technology
Industry characteristics deeply influence AI tool selection, with sectors such as healthcare, finance, and technology exhibiting distinct adoption patterns.
-
Healthcare: The healthcare sector exemplifies the complexity of choosing between commercial and open-source AI foundation models. According to a report by VKTR and the National Academy of Medicine, this choice is situational, requiring careful evaluation of model provenance, data privacy, and clinical validation. Providers and pharmaceutical companies often favor commercial AI tools backed by proprietary datasets and regulatory compliance frameworks. For example, AstraZeneca’s AI-driven oncology research utilizes commercial-grade AI platforms to ensure data integrity and patient safety. However, open-source AI is gaining traction in experimental domains like federated learning, which allows decentralized model training on sensitive patient data without compromising privacy.
-
Finance: Financial institutions balance the need for innovation with stringent regulatory compliance. Commercial AI solutions offer hardened security protocols, audit trails, and compliance features essential for regulated environments. Yet, open-source AI is increasingly applied in areas like algorithmic trading, fraud detection, and customer segmentation where customization and transparency are critical. Accenture advocates for a mindset shift in banks from “why we can’t” to “how might we” adopt AI, indicating openness to hybrid approaches that combine proprietary and open-source tools.
-
Technology: The technology sector leads in open-source AI adoption, with 70% of organizations deploying open-source models across their stacks (McKinsey, 2025). Companies like Google and Meta release robust open-source frameworks that fuel global innovation cycles. The agility and transparency of open-source AI accelerate product development, particularly for startups and scale-ups targeting rapid market entry.
Startups vs. Enterprises: Strategic Advantages and ROI
Startups benefit from open-source AI’s low entry barriers, enabling them to stretch limited budgets and innovate swiftly. They can tailor models to niche markets, achieving competitive differentiation without the overhead of vendor contracts. However, startups bear the responsibility for maintenance, security, and compliance—challenges that can be nontrivial without sufficient expertise.
Enterprises emphasize reliability, risk management, and governance. PwC’s 2025 AI Business Predictions highlight trust as central to AI adoption at scale. Commercial AI vendors provide transparency about model training, liability protections, and ongoing support, critical when AI impacts mission-critical operations or regulated data.
From a return-on-investment perspective, the data is compelling. A global survey found that 92% of early AI adopters report their investments are paying off (insideAI News, 2025). Enterprises often realize faster ROI with commercial solutions due to reduced time-to-market and comprehensive vendor support. Startups, on the other hand, may achieve higher ROI over time by customizing open-source AI to disrupt markets—provided they effectively manage implementation risks.
Case Studies: Successes and Challenges in Implementation
-
Netflix’s Recommendation Engine: Netflix employs a hybrid AI approach, integrating proprietary algorithms with open-source components to analyze viewing habits and personalize content. This blend accelerates innovation and maintains user engagement, demonstrating how combining open and closed systems can be advantageous.
-
Airbus Predictive Maintenance: Airbus uses commercial AI platforms for predictive maintenance of aircraft sensors, emphasizing robustness and regulatory compliance in safety-critical applications.
-
Healthcare AI Startups: Many healthcare AI startups prefer open-source frameworks for their flexibility and cost-effectiveness. However, scaling in highly regulated environments remains challenging without clear AI governance and compliance strategies. The National Academy of Medicine stresses the importance of prudent model selection aligned with clinical and regulatory needs.
-
Commercial Real Estate Innovation: Startups and firms in commercial real estate utilize open-source AI tools to develop custom mortgage calculators, case study generators, and chatbots. Simultaneously, established players adopt commercial AI APIs like Anthropic’s Claude or OpenAI’s GPT to gain immediate access to advanced NLP capabilities, balancing speed with customization.
Balancing Act: The Hybrid Approach
The distinction between open-source and commercial AI is increasingly blurred as organizations adopt hybrid strategies. Many leverage open-source AI for core development and customization, while deploying commercial solutions for critical production workloads requiring enterprise support, compliance, and scalability.
Key considerations when adopting a hybrid approach include:
-
Scalability: Open-source AI often demands significant engineering effort to scale securely. Commercial platforms offer elastic infrastructure, simplifying scalability.
-
Security & Compliance: Proprietary vendors provide certifications and compliance documentation essential for regulated industries, while open-source requires internal governance.
-
Cost Management: Open-source reduces licensing fees but may increase operational overhead for infrastructure and maintenance. Commercial solutions offer predictable pricing but risk vendor lock-in.
-
Innovation Velocity: Open-source thrives on community-driven collaboration and rapid experimentation. Commercial products prioritize stability, polish, and enterprise-grade reliability.
Final Thoughts
Choosing between open-source and commercial AI tools is less about identifying a universally superior option and more about aligning technology choices with organizational goals, risk tolerance, and sector-specific demands. Startups harness open-source AI for rapid innovation and market disruption, while enterprises rely on commercial solutions to secure reliable, compliant, and scalable AI deployments.
In sectors such as healthcare, finance, and technology, regulatory landscapes, data sensitivity, and operational priorities shape AI adoption patterns distinctly. The strategic advantage lies in understanding these nuances and crafting tailored AI strategies that leverage the strengths of both open-source and commercial ecosystems.
Emerging trends like federated learning, hybrid AI architectures, and ethical AI frameworks underscore the importance of flexibility and responsibility in AI deployments. Organizations that adeptly navigate the open-source versus commercial AI landscape will unlock not only technological ROI but also sustainable competitive differentiation in an AI-driven future.
Aspect | Open-Source AI | Commercial AI |
---|---|---|
Typical Users | Startups, small businesses, companies with in-house AI expertise | Large enterprises, regulated industries, organizations lacking deep AI talent |
Cost | No licensing fees, but potential operational and maintenance overhead | Higher upfront costs, predictable pricing, vendor lock-in risk |
Flexibility & Customization | High; enables rapid experimentation and model tailoring | Limited customization; focuses on out-of-the-box enterprise solutions |
Support & Maintenance | Community-driven; internal teams handle maintenance | Dedicated vendor support, SLAs, compliance guarantees |
Security & Compliance | Requires internal governance and expertise | Enterprise-grade security, certifications, regulatory compliance |
Scalability | Requires engineering efforts for secure scaling | Elastic infrastructure simplifying scalability |
Innovation Velocity | Rapid innovation fueled by community collaboration | Stable, polished, focused on reliability |
Industry Examples | Startups in healthcare AI, algorithmic trading, real estate tools | Financial institutions, healthcare providers, aerospace (e.g., Airbus) |
Use Case Examples | Netflix’s open-source components, healthcare federated learning experiments | Netflix proprietary algorithms, AstraZeneca oncology research |
ROI Considerations | Higher ROI potential with risk of maintenance challenges | Faster ROI due to reduced time-to-market and vendor support |
Hybrid Approaches | Core development and customization often open-source | Production workloads with enterprise support and compliance |
Comparative Analysis: Strengths, Limitations, and Hybrid Approaches
Comparative Analysis: Strengths, Limitations, and Hybrid Approaches
Choosing between open-source AI tools and commercial AI solutions is a complex decision—one that depends on technical capabilities, organizational priorities, and evolving market realities. Rather than a binary choice, this decision involves balancing flexibility, innovation speed, cost, support, security, and compliance.
This section synthesizes key insights on the advantages and challenges of each approach and introduces hybrid models that blend their strengths. We also provide a practical decision matrix to help you assess which path aligns best with your project requirements.
The Power and Pitfalls of Open-Source AI
Open-source AI has emerged as a transformative force democratizing AI innovation. According to insights from Red Hat and IBM, open-source projects accelerate discovery by harnessing a global, collaborative developer community. This collective intelligence fosters rapid evolution of models and tools, with contributions from academia, startups, and enterprises.
Notable frameworks like TensorFlow, PyTorch, and Hugging Face Transformers exemplify this openness by enabling developers to build and fine-tune models tailored precisely to their use cases—without proprietary constraints. This flexibility is a strategic asset for organizations seeking deep customization and ownership over their AI solutions.
However, this flexibility demands substantial internal expertise. Open-source AI systems often require skilled teams to deploy, maintain, and scale effectively. Unlike commercial solutions that include vendor-managed infrastructure and professional support, open-source projects rely heavily on community forums and self-driven troubleshooting. For organizations without dedicated AI expertise, this can pose significant operational challenges.
Security and compliance are critical considerations. McKinsey data reveals that 62% of enterprises express concerns about cybersecurity risks when adopting open-source AI, underscoring the need for robust governance frameworks. Additionally, regulatory compliance and intellectual property management require careful attention in open-source deployments.
From a cost perspective, open-source AI eliminates licensing fees, making it attractive for startups and cost-conscious teams. Yet, complexity translates into hidden expenses—such as the engineering talent required for customization and the infrastructure necessary for training and deployment. Open source offers a powerful sandbox but demands skilled players to navigate its complexities.
Commercial AI Solutions: Convenience and Control
Commercial AI platforms provide turnkey usability, supported by managed infrastructure, vendor certifications, and professional services. Microsoft reports that 85% of Fortune 500 companies leverage their AI offerings, often realizing $3.70 in value for every dollar invested. These platforms typically offer polished user interfaces, seamless integration with enterprise systems, and built-in compliance assurances.
The key strength of commercial AI lies in accelerating time to value. Organizations can prototype and scale AI workflows rapidly without the overhead of infrastructure management or deep technical lift. This is especially vital in regulated industries such as finance and healthcare, where enterprise-grade security protocols and vendor-backed compliance reduce operational friction.
However, these conveniences come with trade-offs. Vendor lock-in remains a significant risk. As highlighted by LeanIX and Writer, reliance on proprietary ecosystems can make switching vendors or integrating alternative models costly and technically challenging.
Pricing structures based on licenses or usage can escalate unpredictably, particularly at scale. Furthermore, commercial AI platforms may limit customization options. Organizations requiring highly specialized AI behavior or granular control over model internals may find proprietary solutions constraining.
Hybrid AI Models: Blending the Best of Both Worlds
Recognizing the trade-offs inherent in both approaches, hybrid AI models are gaining traction as a pragmatic compromise. These models combine open-source foundational architectures with proprietary enhancements to balance flexibility, security, and support.
IBM’s watsonx.ai platform exemplifies this hybrid strategy, allowing enterprises to train and fine-tune open-source models within a managed environment that includes governance, compliance tools, and professional support. Similarly, Cytel’s East Horizon Platform integrates open-source AI code with commercial clinical trial software, enabling domain-specific customization without sacrificing regulatory rigor.
Hybrid models enable organizations to:
- Leverage cost-effective open-source cores to reduce upfront development expenses.
- Apply proprietary augmentation to meet specialized domain requirements.
- Mitigate full vendor lock-in by retaining control over critical AI components.
- Benefit from professional support and compliance frameworks provided by commercial vendors.
Implementing hybrid AI requires deliberate architectural planning and a clear understanding of where customization adds value versus where off-the-shelf solutions suffice. This approach reflects the nuanced reality that AI adoption often involves a spectrum rather than exclusive choices.
Decision Checklist: Choosing the Right Path for Your Project
To assist in evaluating which AI approach suits your project, consider the following decision matrix:
Factor | Open Source | Commercial AI | Hybrid Approach |
---|---|---|---|
Flexibility & Control | High — full access to code, models, and customization | Moderate — customization limited by vendor | High — open core with proprietary tuning |
Cost Model | Low upfront costs; variable operational expenses | Licensing and usage fees; potentially high scale | Balanced — reduced development costs; some licensing fees |
Time to Deployment | Longer — requires in-house AI expertise and setup | Faster — vendor-managed, ready-to-use solutions | Moderate — depends on integration complexity |
Support & Maintenance | Community-driven support; variable responsiveness | Professional 24/7 vendor support | Combination of community and vendor support |
Security & Compliance | Requires internal governance and risk management | Enterprise-grade security and compliance | Managed security with customizable controls |
Scalability | Scalable but demands complex infrastructure management | Scalable with vendor’s elastic cloud resources | Flexible — hybrid deployment options |
Risk of Vendor Lock-in | Minimal — open standards and code transparency | High — proprietary ecosystems and contracts | Reduced — control over core components |
Innovation Pace | Rapid — community-driven, enabling experimentation | Controlled — vendor roadmap and priorities | Accelerated — open innovation plus vendor enhancements |
Final Thoughts
Selecting the appropriate AI toolchain demands a strategic alignment of your organization’s capabilities, budget, regulatory environment, and long-term goals. Open-source AI offers ownership, transparency, and agility but requires committed investment in expertise and governance. Commercial AI provides streamlined deployment, robust support, and compliance assurances at a premium, with some loss of control.
Hybrid approaches are increasingly recognized as the most pragmatic path forward. They harness open-source innovation while leveraging commercial reliability and governance, helping organizations manage complexity, cost, and risk effectively.
Ultimately, the best choice transcends marketing hype. It hinges on rigorous evaluation of your project’s unique requirements, readiness to invest in necessary skills and infrastructure, and a commitment to responsible AI adoption that balances innovation with ethical and operational imperatives.
Factor | Open Source | Commercial AI | Hybrid Approach |
---|---|---|---|
Flexibility & Control | High — full access to code, models, and customization | Moderate — customization limited by vendor | High — open core with proprietary tuning |
Cost Model | Low upfront costs; variable operational expenses | Licensing and usage fees; potentially high scale | Balanced — reduced development costs; some licensing fees |
Time to Deployment | Longer — requires in-house AI expertise and setup | Faster — vendor-managed, ready-to-use solutions | Moderate — depends on integration complexity |
Support & Maintenance | Community-driven support; variable responsiveness | Professional 24/7 vendor support | Combination of community and vendor support |
Security & Compliance | Requires internal governance and risk management | Enterprise-grade security and compliance | Managed security with customizable controls |
Scalability | Scalable but demands complex infrastructure management | Scalable with vendor’s elastic cloud resources | Flexible — hybrid deployment options |
Risk of Vendor Lock-in | Minimal — open standards and code transparency | High — proprietary ecosystems and contracts | Reduced — control over core components |
Innovation Pace | Rapid — community-driven, enabling experimentation | Controlled — vendor roadmap and priorities | Accelerated — open innovation plus vendor enhancements |
Conclusion and Recommendations: Making an Evidence-Based Choice for Your AI Project
Conclusion and Recommendations: Making an Evidence-Based Choice for Your AI Project
Choosing between open-source and commercial AI tools is seldom a simple decision—there is no universal solution that fits every project or organization. The optimal choice depends on a nuanced alignment with your project’s specific goals, technical capabilities, security posture, and ethical commitments. Navigating this complexity requires a deliberate, evidence-based approach that transcends hype and generic heuristics.
Aligning AI Tool Choice with Your Project’s Unique Needs
Successful AI integration begins with clear objectives. Whether the focus is automating routine tasks, enhancing customer experiences, or advancing predictive analytics, your AI toolset must directly support these goals. For example, Atlassian highlights the value of setting precise AI objectives to unlock efficiencies in project management workflows, such as automating task assignments or optimizing resource allocation. Likewise, CTOs emphasize the importance of defining KPIs aligned with broader business strategies to meaningfully measure AI’s impact.
Equally critical is evaluating your team’s technical maturity. Open-source AI solutions demand substantial in-house expertise for customization, integration, and ongoing maintenance. They thrive in organizations capable of investing in developer skill-building and managing the complexities of continuous updates, security patches, and compliance. In contrast, commercial AI platforms typically offer turnkey usability with vendor-backed support, lowering the barrier for teams with limited AI experience or infrastructure.
Security and ethical considerations must also guide your selection. Open-source models provide transparency that facilitates auditing and regulatory compliance, a vital advantage in sectors such as healthcare and finance. However, this openness carries risks; security experts warn about adversarial threats like data poisoning and backdoors, necessitating vigilant monitoring and defense strategies. Commercial vendors often embed hardened security protocols, service-level agreements, and compliance certifications, but this can come at the cost of reduced visibility into underlying algorithms and data management practices.
Pragmatic Evaluation: Total Cost of Ownership, Scalability, and Sustainability
Beyond license fees or the allure of zero-cost open-source licenses, it is essential to assess the total cost of ownership. Hidden expenses abound in AI projects—data acquisition and labeling alone can range from $10,000 for pilot initiatives to over $1 million for large-scale deployments. Development costs vary widely; custom AI systems generally start around $50,000 and can escalate into the hundreds of thousands depending on complexity, integration depth, and domain-specific requirements.
Open-source components can reduce development costs by 15–30%, yet they require ongoing investment in skilled personnel and infrastructure management. Commercial AI solutions, while often involving higher upfront and subscription fees, bundle support, maintenance, and integration services that may lower operational overhead and simplify compliance.
Scalability is another pivotal consideration. AI workloads typically experience annual growth of 40–60% in data volume and user demands. Open-source frameworks like PyTorch and TensorFlow offer flexibility to tailor scaling strategies to organizational needs but demand significant infrastructure expertise. Commercial platforms provide elastic cloud resources and managed services that simplify scaling but may introduce vendor lock-in and limit customization.
Long-term sustainability requires attention to energy consumption and environmental impact. Training a single large generative AI model can consume over 1,200 megawatt-hours of electricity, contributing substantially to carbon footprints. Organizations committed to sustainability should weigh such factors carefully, potentially favoring solutions optimized for energy efficiency or adopting hybrid approaches that balance compute demands with performance and environmental responsibility.
Staying Ahead: Continuous Monitoring and Ethical Stewardship
The AI ecosystem in 2025 is characterized by rapid innovation and evolving standards. Breakthroughs—from advanced agentic AI capable of multi-step reasoning to novel model context protocols—emerge frequently. Staying informed via industry conferences such as Nvidia GTC, authoritative resources like the Stanford AI Index, and ongoing research is critical. This vigilance enables AI architects to anticipate shifts, assess emerging risks, and seize innovation opportunities proactively.
Equally paramount is embracing ethical responsibility. The choice between open-source and commercial AI tools transcends technical and financial factors; it shapes broader societal impact. Open-source AI democratizes access, fostering global innovation and inclusion. However, it also raises concerns about misuse, bias, and data privacy. Commercial AI may embed stronger controls and compliance assurances but risks vendor lock-in and opacity in decision-making processes.
AI architects and organizational leaders must champion transparency, fairness, and accountability. This entails ensuring AI systems comply with ethical standards and legal frameworks, instituting proactive governance structures such as AI steering committees, contractual safeguards, risk mitigation strategies, and continuous ethical audits. Embedding these principles reduces liability and builds trust with users and stakeholders alike.
Key Takeaways
-
No universal winner: Select AI tools based on alignment with your project’s goals, technical capacity, security requirements, and ethical standards.
-
Assess total costs: Consider development, maintenance, scaling, and environmental impact—not just licensing fees.
-
Balance flexibility and support: Open-source AI offers customization and transparency; commercial solutions provide ease of use, robust security, and compliance guarantees.
-
Monitor ecosystem developments: AI innovation is accelerating; staying informed is essential to adapt and protect your investments.
-
Prioritize ethical AI governance: Transparency, fairness, and regulatory compliance are critical for sustainable AI adoption and public trust.
Ultimately, choosing between open-source and commercial AI tools is a strategic act of stewardship. It involves designing solutions that not only perform effectively but also align with the values and responsibilities incumbent upon AI architects shaping the future of technology and society.
Criteria | Open-Source AI | Commercial AI |
---|---|---|
Alignment with Project Goals | Requires clear objectives and technical maturity for customization | Turnkey usability aligned with business KPIs |
Technical Expertise | High in-house expertise needed for integration and maintenance | Lower barrier with vendor-backed support |
Security and Transparency | High transparency enabling auditing; risks of adversarial attacks | Hardened security, SLAs, compliance; less algorithm visibility |
Total Cost of Ownership | Lower development costs (15–30% savings) but ongoing personnel and infrastructure investment | Higher upfront/subscription fees; includes support and maintenance |
Scalability | Flexible scaling via frameworks (e.g., PyTorch, TensorFlow); requires infrastructure expertise | Elastic cloud resources and managed services; potential vendor lock-in |
Environmental Impact | Depends on infrastructure and model efficiency; requires careful management | Often optimized for energy efficiency; hybrid approaches possible |
Innovation & Ecosystem | Rapid innovation, community-driven updates | Proprietary advancements with vendor-led innovation |
Ethical Considerations | Democratizes access; risks of misuse and bias | Stronger controls and compliance; risk of opacity and lock-in |
Governance | Requires proactive ethical audits and governance structures | Includes contractual safeguards and compliance frameworks |