Mastering Personal Finance with AI: Budgeting & Investing Tips
- Introduction: Harnessing AI to Transform Your Personal Finances
- Harnessing AI to Transform Your Personal Finances
- The Transformative Power of AI in Personal Finance
- Clear Goals AI Can Help You Achieve
- Balancing AI Capabilities with Human Oversight
- The Imperative of Data Privacy and Ethics
- Looking Ahead
- Prerequisites: Understanding the Technical Foundations and Financial Literacy Needed
- Prerequisites: Understanding the Technical Foundations and Financial Literacy Needed
- Mastering the Basics of Financial Literacy
- Grasping the AI Technologies Behind Personal Finance Tools
- Technical and Security Foundations for Using AI in Personal Finance
- Bridging the Gap Between Technology and Financial Savvy
- Preparing Your Financial Data and Selecting the Right AI Tools
- Preparing Your Financial Data and Selecting the Right AI Tools
- Gathering and Organizing Your Financial Data Securely
- Evaluating and Selecting AI Financial Tools: What Really Matters?
- Popular AI Budgeting and Investing Platforms Compared
- Final Thoughts on Data Preparation and Tool Selection
- Step-by-Step AI-Driven Budgeting: From Data Input to Personalized Insights
- Step-by-Step AI-Driven Budgeting: From Data Input to Personalized Insights
- Importing and Organizing Transaction Data: The Foundation of AI Budgeting
- Automated Expense Categorization: Demystifying the Machine Learning Magic
- Setting Realistic Budget Goals with Predictive Analytics
- Receiving AI-Generated Alerts: Staying Ahead of Overspending and Saving Opportunities
- How AI Adapts Budgets Dynamically in Response to User Behavior and External Factors
- In Summary
- Leveraging AI for Intelligent Investing: Automated Strategies and Risk Management
- Leveraging AI for Intelligent Investing: Automated Strategies and Risk Management
- How AI Evaluates Risk and Manages Your Investment Portfolio
- Using Generative AI for Scenario Simulation: Peering into Alternative Futures
- Algorithmic Trading and Market Prediction: Behind the AI Curtain
- The Vital Role of Human Judgment Amid AI’s Rise
- Key Takeaways
- Troubleshooting and Mitigating Risks in AI-Based Financial Management
- Troubleshooting and Mitigating Risks in AI-Based Financial Management
- Common Challenges in AI-Driven Financial Tools
- Practical Steps to Verify and Interpret AI Insights
- Protecting Your Privacy and Data Security
- Recognizing and Responding to AI Limitations
- Balancing Enthusiasm with Caution
- Advanced Techniques: Optimizing AI Use for Personalized Financial Growth and Ethical Considerations
- Advanced Techniques: Optimizing AI Use for Personalized Financial Growth and Ethical Considerations
- Integrating and Customizing AI Tools for Holistic Financial Planning
- Leveraging Behavioral Finance Algorithms to Correct Cognitive Biases
- Ethical Considerations: Transparency, Data Ownership, and Societal Impact
- Staying Ahead: Continuous Learning and Adaptation
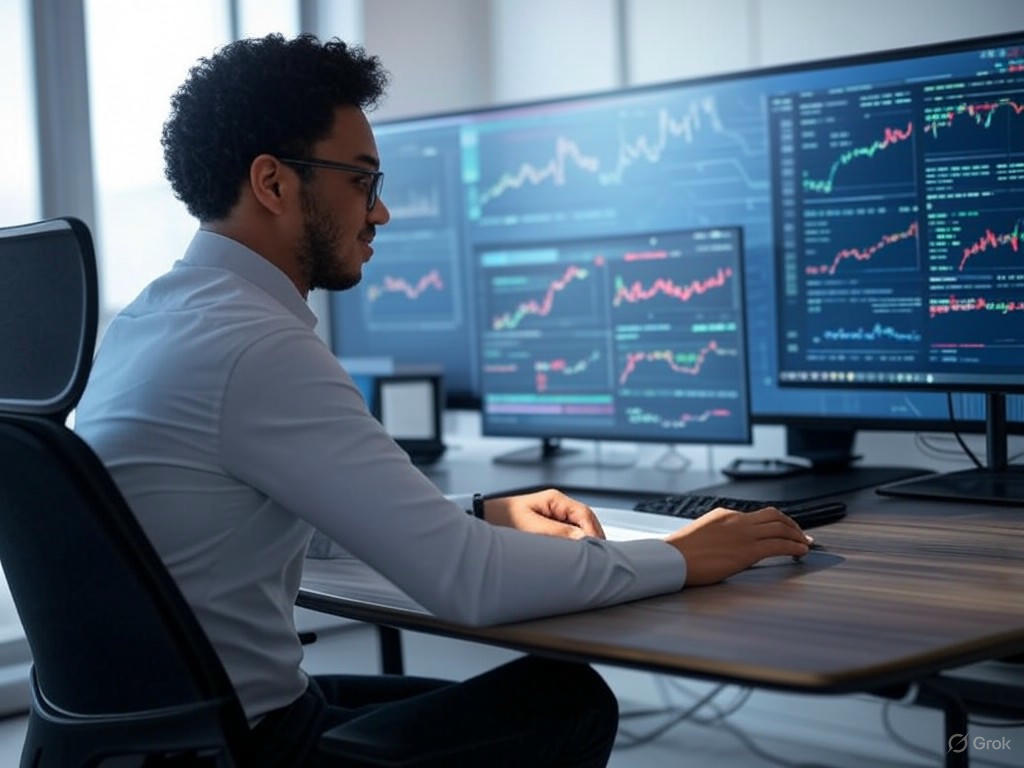
Introduction: Harnessing AI to Transform Your Personal Finances
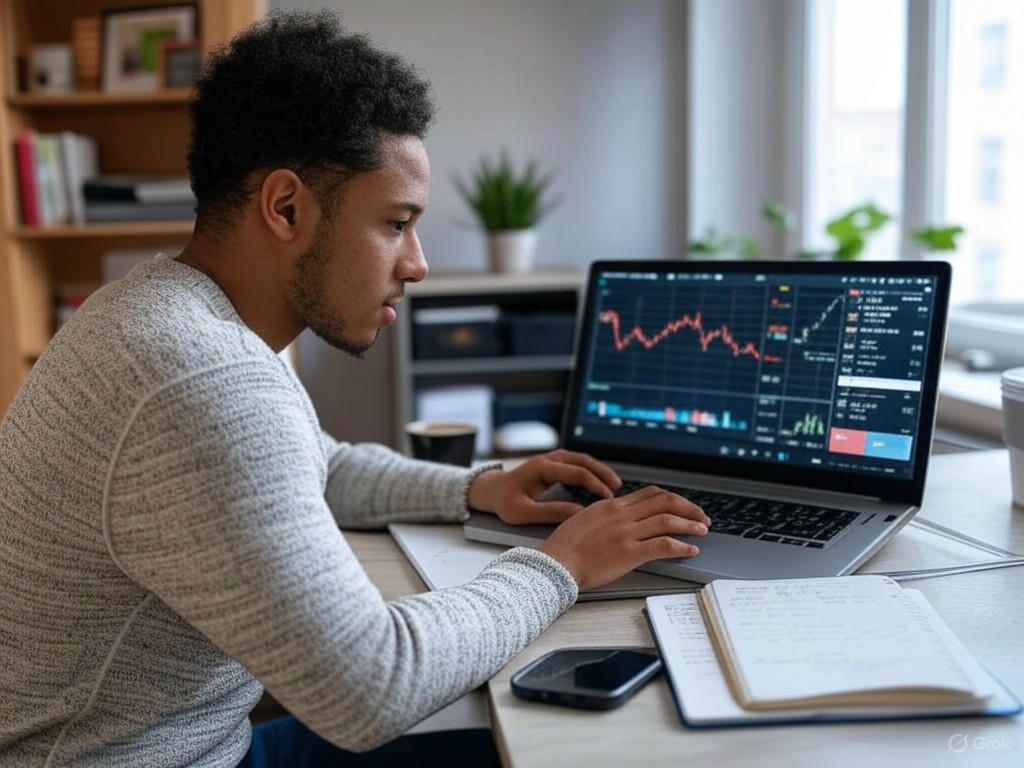
Harnessing AI to Transform Your Personal Finances
Have you ever wondered how artificial intelligence—once the stuff of science fiction—is quietly revolutionizing the way we manage our money? Today, AI-driven tools are no longer exclusive to Wall Street firms or fintech startups. They have become accessible partners in everyday personal finance, offering capabilities that can fundamentally reshape how you budget, save, and invest.
The Transformative Power of AI in Personal Finance
At its core, AI excels at processing vast amounts of data, detecting patterns, and forecasting trends that often escape human intuition. In personal finance, this means AI can analyze your spending habits, income streams, and investment opportunities with remarkable precision and speed—capabilities unimaginable just a decade ago.
For example, AI-powered budgeting apps like YNAB and Mint do more than simply track expenses. They learn from your behavior, anticipate upcoming costs, and suggest personalized budgets tailored to your lifestyle rather than imposing rigid, one-size-fits-all plans. Tools such as Trim take this a step further by automatically identifying and canceling unwanted subscriptions, optimizing your spending without any extra effort from you.
On the investing side, AI is transforming portfolio management. According to the World Economic Forum, by 2027, AI-driven investment tools are projected to become the primary advisors for retail investors. These platforms process extensive datasets to craft strategies personalized to your risk tolerance and financial goals. However, this evolution doesn’t replace human advisors; instead, AI empowers them to provide more informed and nuanced recommendations by blending computational rigor with human judgment.
Clear Goals AI Can Help You Achieve
What does this mean for your financial life? AI-driven tools aim to deliver tangible benefits such as:
-
Improved Financial Literacy: AI-powered chatbots and virtual assistants simplify complex financial jargon, helping you grasp concepts that once required specialized knowledge.
-
Personalized Budgeting: By analyzing your actual income and spending patterns, AI crafts budgets that evolve with you, offering better control and reducing frustration from unrealistic targets.
-
Automated Savings: Features like automatic round-ups, offered by apps like Chime, save spare change from everyday transactions, nudging you effortlessly toward your savings goals.
-
Data-Driven Investment Strategies: Robo-advisors apply predictive analytics to balance risk and growth dynamically, adjusting portfolios based on market conditions and your preferences.
These capabilities not only optimize your finances but also encourage a more engaged and informed approach to money management.
Balancing AI Capabilities with Human Oversight
Despite the impressive advances in AI, it’s important to remember these tools are assistants—not oracles. Trust remains foundational in successful financial advice, whether human or machine-driven. Over 80% of investors are open to AI-supported portfolio management, yet they still value human oversight to interpret context, emotions, and ethical nuances that AI cannot fully capture.
Moreover, AI’s effectiveness depends on the quality of data and algorithms behind it. Algorithmic bias—where AI decisions reflect prejudiced or incomplete data—poses significant risks that require vigilant design and continuous monitoring. Ethical AI deployment involves addressing these biases, ensuring inclusivity, and guarding against manipulation or exploitation.
The Imperative of Data Privacy and Ethics
Handling sensitive financial data demands rigorous privacy protections. The Cisco 2025 Data Privacy Benchmark Study highlights growing public expectations for strong privacy legislation and corporate transparency. Since AI tools require access to your spending patterns, income, and investments, they must adhere to stringent security standards to maintain your trust.
Ethically, the boundary between offering helpful financial suggestions and unduly influencing decisions is delicate. Both developers and users must advocate for transparency in AI recommendations, clear disclosures about capabilities and limitations, and a commitment to using AI to empower—not exploit—consumers.
Looking Ahead
AI’s integration into personal finance is not a distant vision—it’s happening now, enhancing how individuals understand and manage their money. Yet, as with any powerful technology, this promise carries responsibility. By thoughtfully embracing AI tools, combining their strengths with human wisdom, and prioritizing ethical standards and privacy, we can unlock a new era of financial empowerment for everyone.
AI Capability | Description | Example Tools |
---|---|---|
Improved Financial Literacy | Simplifies complex financial jargon through chatbots and virtual assistants. | Various AI-powered chatbots |
Personalized Budgeting | Analyzes income and spending to create evolving, tailored budgets. | YNAB, Mint |
Automated Savings | Automatically rounds up transactions to save spare change. | Chime |
Data-Driven Investment Strategies | Uses predictive analytics to balance risk and growth dynamically. | Robo-advisors |
Prerequisites: Understanding the Technical Foundations and Financial Literacy Needed
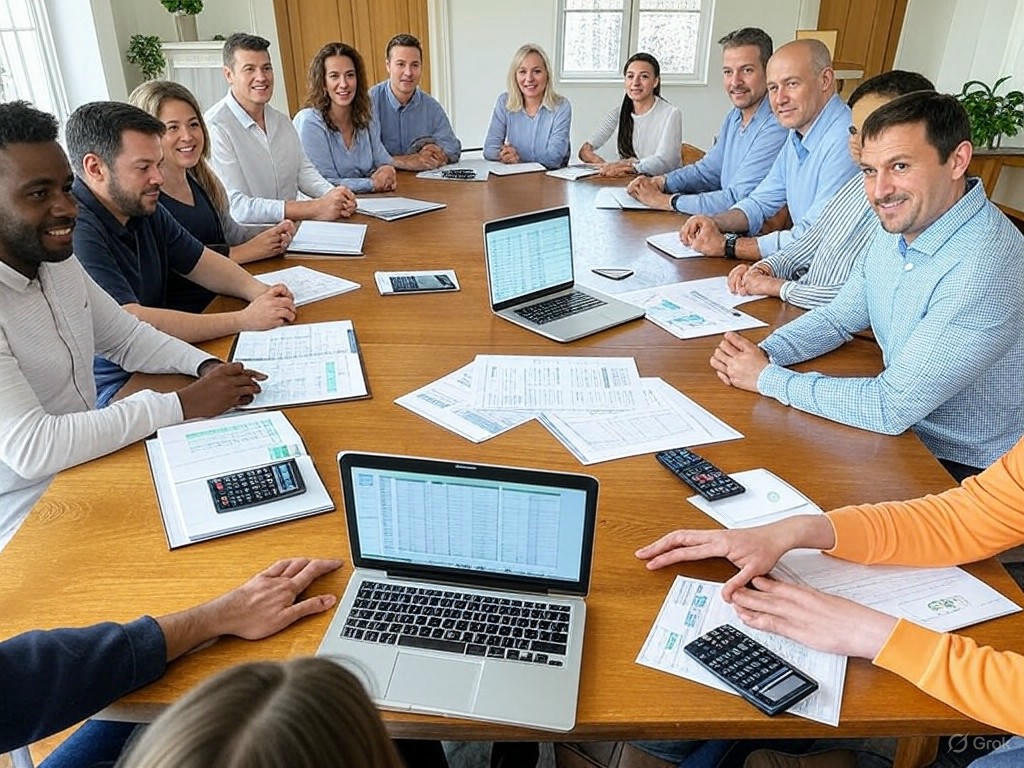
Prerequisites: Understanding the Technical Foundations and Financial Literacy Needed
Before you let AI steer your personal finances, it’s essential to build a strong foundation in both financial literacy and the technical underpinnings of AI technologies. Think of this as tuning your engine before a long journey—without this preparation, even the most advanced AI tools can lead you astray.
Mastering the Basics of Financial Literacy
Budgeting, investing, and understanding your risk tolerance aren’t just buzzwords—they are the pillars of any sound financial strategy. Budgeting goes beyond simply tracking expenses; it’s about organizing your financial life to achieve key goals like paying off debt, saving for retirement, or building an emergency fund.
Financial experts recommend saving 10-15% of your gross income for retirement when possible. Equally important is establishing an emergency fund that covers six months of living expenses, providing a critical safety net against unexpected events.
One nuance often overlooked is that successful budgeting requires finding a system that suits your personality and lifestyle. Whether you prefer a zero-based budgeting approach like YNAB or a more flexible model, consistency is key. Studies demonstrate that optimistic budgeting can reduce spending by nearly 22% compared to not budgeting at all, with lasting effects over months.
AI-powered budgeting apps can help by automatically categorizing expenses and highlighting opportunities to cut back. Tools like Mint, YNAB, and PocketGuard analyze your spending patterns and provide real-time alerts, but your fundamental grasp of budgeting principles remains indispensable.
When it comes to investing, the classic 60/40 stock-to-bond allocation continues to be a balanced strategy for navigating diverse economic conditions. But diversification involves more than just asset allocation; it includes understanding instruments such as Treasury Inflation-Protected Securities (TIPS) to hedge inflation risk or dividend-paying stocks for steadier income streams.
Your risk tolerance—the level of market volatility and potential loss you’re comfortable with—is dynamic. It evolves with your life circumstances and financial goals. AI tools can assist in assessing and adjusting your risk profile over time, but no AI can replace the judgment you develop by understanding these financial fundamentals.
Grasping the AI Technologies Behind Personal Finance Tools
What powers smart budgeting apps and robo-advisors? Several AI technologies transform raw financial data into actionable insights:
-
Machine Learning (ML): ML algorithms analyze thousands of data points—from spending habits to credit history—to offer personalized recommendations. Banks increasingly use ML beyond traditional credit scores to make smarter lending decisions, enhancing fairness and accuracy.
-
Natural Language Processing (NLP): NLP enables conversational interactions with AI financial assistants through chatbots or voice commands. This technology powers virtual assistants that answer questions, explain spending trends, or guide you through investment options in real time.
-
Data Privacy and Security: Since AI platforms process sensitive financial data, understanding data privacy principles is critical. Compliance with regulations such as GDPR and CCPA, adoption of privacy-by-design frameworks, and transparency about data usage are non-negotiable. Users should be vigilant about app permissions and prefer services employing strong encryption and multi-factor authentication.
AI’s role in personal finance extends beyond automation—it augments human decision-making with data-driven insights. Still, the complexity of these technologies means users benefit from a basic knowledge of how AI processes data and the potential risks involved, including algorithmic bias or inadvertent data exposure.
Technical and Security Foundations for Using AI in Personal Finance
To fully leverage AI’s benefits, you typically need to integrate your digital banking data with AI-driven tools. This integration brings important technical and security considerations:
-
Access to Digital Banking Data: Most AI-powered budgeting and investment apps connect securely to your bank accounts, credit cards, and investment platforms via APIs or open banking protocols. This enables real-time transaction tracking, spending analysis, and portfolio monitoring.
-
Familiarity with AI-Driven Platforms: Leading personal finance apps like Simplifi, Quicken, and Monarch Money use AI to predict expenses, detect spending anomalies, and provide tailored advice. Familiarizing yourself with these platforms’ features—such as customizing alerts and linking accounts—enhances your ability to maximize their value.
-
Security Best Practices: Given the sensitivity of financial data, protecting it is paramount. Employ two-factor authentication, regularly update passwords, and review app permissions. Stay alert to phishing attempts and social engineering tactics that could compromise your credentials.
-
Regulatory Landscape and Compliance: Financial institutions and app providers operate under strict data protection regulations. Staying informed about your rights—such as those granted by the Consumer Financial Protection Bureau’s Personal Financial Data Rights Rule finalized in October 2024—is essential for maintaining control over your information.
Bridging the Gap Between Technology and Financial Savvy
Success in using AI for budgeting and investing depends on the intersection of solid financial knowledge and an understanding of the enabling technology. AI is a powerful copilot but can never replace your financial intuition or ethical considerations.
Being proactive about learning—whether that means brushing up on investing basics, understanding how machine learning algorithms work, or knowing how your data is stored and secured—empowers you to harness AI’s capabilities while minimizing risks.
In this rapidly evolving landscape, adopting a skeptical yet open mindset works best: trust but verify, and always keep your financial goals and personal privacy front and center. This dual foundation prepares you not only to use AI tools effectively today but also to adapt alongside tomorrow’s innovations.
Category | Key Points |
---|---|
Financial Literacy Basics | Budgeting organizes finances to meet goals; save 10-15% for retirement; emergency fund for 6 months expenses; consistency in budgeting methods; classic 60/40 stock-to-bond investment allocation; diversification includes TIPS and dividend stocks; risk tolerance is dynamic and personal judgment is crucial. |
AI Technologies in Personal Finance | Machine Learning analyzes data for personalized advice; Natural Language Processing enables conversational AI assistants; Data privacy requires compliance with GDPR, CCPA; strong encryption and multi-factor authentication recommended; awareness of algorithmic bias and data exposure risks. |
Technical & Security Foundations | Secure integration with banking data via APIs/open banking; familiarity with apps like Simplifi, Quicken, Monarch Money; use two-factor authentication, strong passwords, review permissions; stay informed on regulations like CFPB’s Personal Financial Data Rights Rule. |
Bridging Technology & Financial Savvy | Combine financial knowledge with tech understanding; AI augments but does not replace intuition; continual learning on investing and AI processes; maintain privacy and verify AI recommendations; adopt skeptical yet open mindset for evolving tools. |
Preparing Your Financial Data and Selecting the Right AI Tools
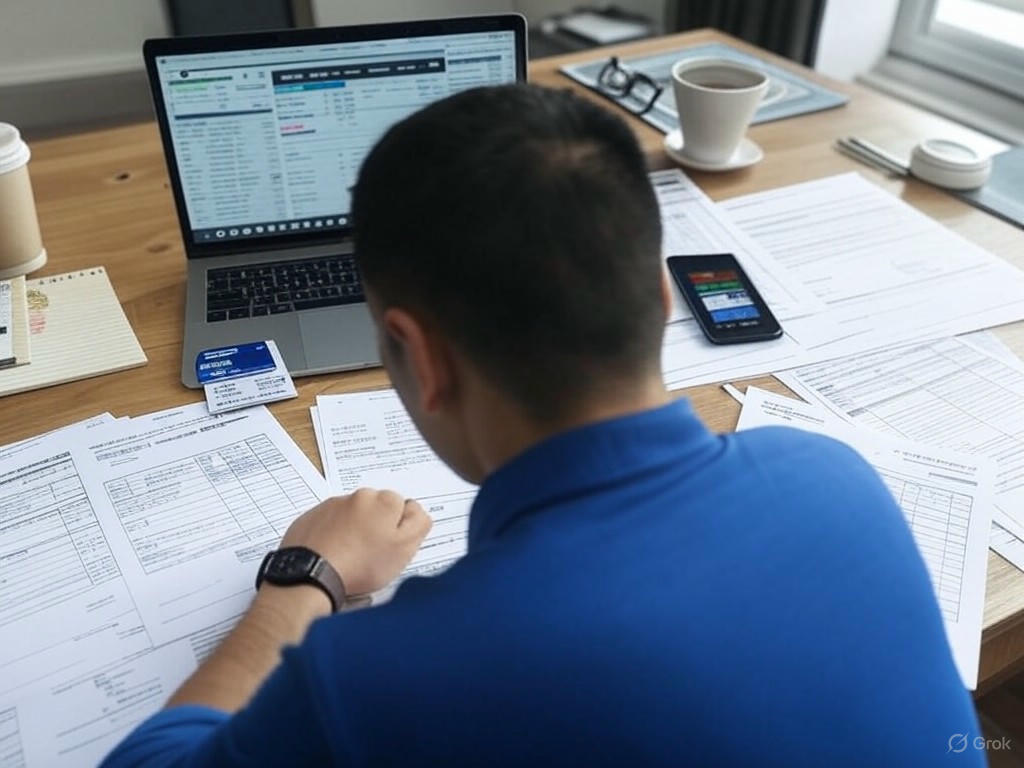
Preparing Your Financial Data and Selecting the Right AI Tools
How do you transform a jumble of financial statements, bank accounts, credit cards, and investment portfolios into a coherent dataset ready for AI-driven analysis? The answer lies in careful preparation paired with a discerning selection of AI tools that respect your privacy while delivering meaningful value.
Gathering and Organizing Your Financial Data Securely
Before you unleash AI on your finances, start by gathering data from diverse sources: checking and savings accounts, credit cards, investment portfolios, and spending histories. This step is not merely about collecting numbers; it’s about creating a clean, consistent, and secure foundation for AI to provide accurate insights.
Today’s financial institutions operate under rigorous consent and privacy regulations, such as the Consumer Financial Protection Bureau’s Personal Financial Data Rights Rule finalized in October 2024. These rules empower you with greater control, requiring explicit permission before AI budgeting or investing tools can access your sensitive data.
Approach this linking process with care by:
- Reviewing consent and privacy policies thoroughly. Understand how the platform collects, uses, and stores your data.
- Choosing tools compliant with major privacy laws like GDPR, CCPA, and the upcoming Minnesota Consumer Data Privacy Act effective mid-2025.
- Preferring platforms that use strong encryption and anonymization techniques to safeguard data during transmission and storage.
- Considering emerging decentralized consent management systems that provide granular control over data sharing, expected to gain traction in 2025.
Think of your financial data like personal health records—deeply sensitive and requiring meticulous handling. RegTech solutions, which automate compliance processes, are increasingly important to minimize manual data handling and reduce risks. As you organize your data, aim for accuracy and completeness. AI insights depend heavily on the quality of input data—garbage in, garbage out still applies.
Evaluating and Selecting AI Financial Tools: What Really Matters?
The AI landscape for personal finance is crowded, ranging from free budgeting apps to sophisticated robo-advisors. How can you cut through the noise and pick the right tool for your unique financial needs?
Focus on these four critical criteria:
1. Algorithm Transparency
AI systems often operate as black boxes, making decisions without clear explanations. Transparency goes beyond buzzwords—it’s about building trust. Can the platform clearly explain how it generates budget recommendations or constructs investment portfolios?
For example, a robo-advisor that openly shares its risk models and portfolio construction principles instills more confidence than one that simply outputs a “best portfolio” without context. Transparency helps users identify potential biases and understand AI-driven advice.
2. Personalization Capabilities
Your financial life is unique. Select AI tools that tailor insights to your income, spending habits, financial goals, and risk tolerance. For instance, YNAB excels at detailed budgeting, while Personal Capital combines budgeting with investment tracking for a holistic financial view.
On the investing side, AI-driven platforms increasingly use machine learning to adapt portfolio strategies in real time, offering dynamic management beyond static, rule-based approaches.
3. Security Measures
Security is non-negotiable when handling financial data. Leading platforms implement multi-layered protection, including identity intelligence, network resilience, and AI-based security fortifications.
Cisco’s 2025 Data Privacy Benchmark reveals that 86% of users trust global providers to protect their data, but vigilance remains essential. When evaluating platforms, verify if they employ:
- End-to-end encryption
- Continuous fraud monitoring
- Compliance certifications like SOC2 and ISO 27001
- Regular third-party security audits
4. User Interface and Experience
Even the most powerful AI tools are ineffective if they’re difficult to use. A clean, intuitive user interface encourages regular engagement and better financial understanding.
Apps like Mint offer free, easy-to-navigate budgeting with AI-powered insights, while Trim’s AI identifies unwanted subscriptions and can cancel them automatically, reducing friction and saving money effortlessly.
Popular AI Budgeting and Investing Platforms Compared
Here’s a snapshot of leading platforms for 2025, focusing on data integration, features, and trustworthiness:
Platform | Data Integration | Key Features | Trustworthiness & Security |
---|---|---|---|
YNAB (You Need A Budget) | Connects bank accounts and credit cards | Detailed budgeting, goal tracking | Strong privacy policies, transparent fees |
Mint | Broad aggregation of financial accounts | Free budgeting, bill reminders, credit score monitoring | Owned by Intuit, robust security and data policies |
Personal Capital | Combines budgeting with investment accounts | Investment tracking, retirement planning | High security standards, clear disclosures |
Betterment | Investment accounts, some bank syncing | Robo-advisor with personalized portfolios | SEC-registered, encrypted data storage |
Schwab Intelligent Portfolios | Deep brokerage integration | Automated portfolio management, tax-loss harvesting | Regulated broker, strong compliance framework |
Trim | Transaction-level bank data | Subscription cancellation, spending analysis | Focus on data privacy, encrypted communications |
Acorns | Bank and credit card integration | Automatic round-ups, micro-investing | Transparent fees, regulated investment platform |
Zeta | Family and joint account tracking | Designed for couples and families, budgeting | GDPR and CCPA compliant, secure data handling |
This list exemplifies the diverse approaches available. Some platforms prioritize streamlined budget management, while others specialize in AI-driven investing or subscription management.
Final Thoughts on Data Preparation and Tool Selection
Preparing your financial data carefully is the foundation for high-quality AI insights. Equally important is choosing AI tools that are transparent, personalized, secure, and easy to use.
In a world buzzing with AI hype, remember no tool is perfect. Regulatory landscapes evolve rapidly, and AI models—especially complex ones—may sometimes offer recommendations that require your human judgment. Treat AI as a powerful assistant, not a crystal ball.
Start small: connect a single account, explore the tool’s features, and monitor how it handles your data. Prioritize platforms with a strong commitment to privacy and regulatory compliance. With thoughtful preparation and careful tool selection, AI can become a trustworthy partner on your journey toward smarter budgeting and investing.
Platform | Data Integration | Key Features | Trustworthiness & Security |
---|---|---|---|
YNAB (You Need A Budget) | Connects bank accounts and credit cards | Detailed budgeting, goal tracking | Strong privacy policies, transparent fees |
Mint | Broad aggregation of financial accounts | Free budgeting, bill reminders, credit score monitoring | Owned by Intuit, robust security and data policies |
Personal Capital | Combines budgeting with investment accounts | Investment tracking, retirement planning | High security standards, clear disclosures |
Betterment | Investment accounts, some bank syncing | Robo-advisor with personalized portfolios | SEC-registered, encrypted data storage |
Schwab Intelligent Portfolios | Deep brokerage integration | Automated portfolio management, tax-loss harvesting | Regulated broker, strong compliance framework |
Trim | Transaction-level bank data | Subscription cancellation, spending analysis | Focus on data privacy, encrypted communications |
Acorns | Bank and credit card integration | Automatic round-ups, micro-investing | Transparent fees, regulated investment platform |
Zeta | Family and joint account tracking | Designed for couples and families, budgeting | GDPR and CCPA compliant, secure data handling |
Step-by-Step AI-Driven Budgeting: From Data Input to Personalized Insights
Step-by-Step AI-Driven Budgeting: From Data Input to Personalized Insights
How does AI transform a chaotic jumble of bank transactions into a clear, actionable budget? The journey starts with seamlessly importing and organizing your financial data—a process often more technical and security-sensitive than most users realize.
Importing and Organizing Transaction Data: The Foundation of AI Budgeting
Leading AI budgeting tools like Mint, YNAB, and PocketGuard excel because they connect directly to your financial accounts through secure APIs or encrypted data feeds. This means no more tedious manual entry or spreadsheet headaches; your transaction data flows automatically into the app, updated in near real-time.
Behind the scenes, these connections rely on bank-grade security protocols—such as multi-factor authentication and end-to-end encryption—to safeguard your sensitive financial information. In 2025, with growing consumer awareness around privacy, trustworthiness and compliance with regulations like GDPR and CCPA are essential for any reputable app.
For example, platforms like Monarch Money and Quicken Simplifi balance robust data integration with strong encryption, offering a comprehensive and secure view of all your accounts in one place.
Automated Expense Categorization: Demystifying the Machine Learning Magic
Once your raw transactions are imported, AI steps in to categorize every expense. Traditional budgeting apps might assign categories based on merchant names or locations—“Amazon” purchases go to “Shopping,” Starbucks to “Dining Out.” But this rule-based approach is often brittle and error-prone.
Today’s AI budgeting tools use sophisticated machine learning models trained on millions of transaction records. These models analyze not just where you spent money, but what you bought. For instance, SparkReceipt leverages advanced natural language processing (NLP) powered by models like OpenAI’s ChatGPT to parse individual receipt items. This enables “Amazon” expenses to be broken down into finer categories such as “Electronics,” “Books,” or “Household Supplies” based on actual purchases.
This granular classification dramatically improves accuracy, enabling the AI to detect unusual spending patterns or recurring subscriptions you might have missed. Over time, the system learns your unique habits, refining categories and minimizing false positives.
Setting Realistic Budget Goals with Predictive Analytics
AI budgeting tools evolve from passive record-keepers into proactive financial advisors through predictive analytics. By analyzing your historical spending, income patterns, and external economic factors—like inflation or upcoming bills—these systems recommend realistic budget targets tailored to your lifestyle.
For example, Quicken Simplifi and FinPlan AI use algorithms to forecast your monthly expenses and suggest personalized savings goals. They adapt continuously based on your actual financial behavior and future projections.
Imagine your AI assistant noticing a spike in utility bills as winter approaches, adjusting your heating budget accordingly. Or detecting increased dining out during holidays and recommending a temporary boost in your entertainment budget to keep you on track.
This dynamic budgeting approach replaces static budgets that quickly become outdated. AI models continuously recalibrate your targets, making financial planning more responsive and less stressful.
Receiving AI-Generated Alerts: Staying Ahead of Overspending and Saving Opportunities
Automation shines when it frees you from constant vigilance. Leading AI budgeting platforms send real-time alerts to warn of potential overspending or highlight savings opportunities.
For instance, PocketGuard offers a “safe-to-spend” balance by subtracting upcoming bills and savings goals from your available funds, alerting you when you’re close to exceeding it. Similarly, Trim’s AI analyzes your subscriptions, identifying redundant services and offering to cancel them automatically—putting money back in your pocket without any extra effort.
These alerts are powered by anomaly detection algorithms that flag unusual transactions or spikes in spending, similar to fraud detection systems banks use. This dual function not only protects your budget but adds a layer of security.
How AI Adapts Budgets Dynamically in Response to User Behavior and External Factors
The real strength of AI budgeting lies in its adaptability. Financial lives are fluid—unexpected expenses, changes in income, or market fluctuations can disrupt budgets. Unlike manual budgeting, AI tools continuously ingest new data and adjust recommendations accordingly.
This dynamic adaptation depends on time-series forecasting models and reinforcement learning algorithms that evaluate how you respond to prior budgets and external signals. For example, if you consistently underspend your dining budget, the AI might reallocate that surplus towards building your emergency fund or boosting investment contributions.
Moreover, some advanced platforms incorporate macroeconomic indicators—such as interest rate changes, inflation trends, or geopolitical events—to forecast their potential impact on your finances. This integration helps you prepare proactively, not just reactively, to shifts in the broader economic landscape.
In Summary
- Data Import: Secure API connections automatically pull transaction data, eliminating manual input.
- Expense Categorization: Machine learning models analyze purchase details, vastly improving accuracy over rule-based methods.
- Budget Goals: Predictive analytics create tailored financial targets that adapt to your behavior and external factors.
- AI Alerts: Real-time notifications warn about overspending risks and uncover savings opportunities.
- Dynamic Adaptation: Continuous learning algorithms update budgets as your financial situation and the economy evolve.
By understanding the technical foundations and practical benefits of AI-driven budgeting, you can harness these tools not merely for tracking but for actively managing your financial future with confidence and precision. Choosing solutions that balance powerful automation with privacy and transparency is key—because when it comes to money, trust is everything.
Step | Description | Example Tools/Platforms | Key Technologies/Features |
---|---|---|---|
Data Import | Secure API connections automatically pull transaction data, eliminating manual input and spreadsheet headaches. | Mint, YNAB, PocketGuard, Monarch Money, Quicken Simplifi | Secure APIs, Multi-factor authentication, End-to-end encryption, GDPR & CCPA compliance |
Expense Categorization | Machine learning models analyze purchase details to categorize expenses more accurately than rule-based methods. | SparkReceipt | Machine learning, Natural Language Processing (NLP), Fine-grained categorization, Pattern detection |
Budget Goals | Predictive analytics create tailored financial targets that adapt based on historical spending, income, and external factors. | Quicken Simplifi, FinPlan AI | Predictive analytics, Forecasting algorithms, Adaptive budgeting |
AI Alerts | Real-time notifications warn about potential overspending and identify savings opportunities. | PocketGuard, Trim | Anomaly detection, Subscription analysis, Safe-to-spend calculations, Automated subscription cancellations |
Dynamic Adaptation | Continuous learning algorithms update budgets dynamically in response to user behavior and external economic factors. | Advanced AI budgeting platforms | Time-series forecasting, Reinforcement learning, Integration of macroeconomic indicators |
Leveraging AI for Intelligent Investing: Automated Strategies and Risk Management
Leveraging AI for Intelligent Investing: Automated Strategies and Risk Management
How do AI-powered robo-advisors and investment platforms build portfolios tailored to your financial goals? At their core, these platforms employ algorithmic asset allocation strategies that integrate your risk tolerance, investment horizon, and personal preferences with comprehensive market data.
According to Morningstar’s 2025 Robo-Advisor Report, leaders like Betterment and Vanguard Digital Advisor distinguish themselves not only through low fees but also via transparent, data-driven portfolio construction. Betterment marries sleek technology with clear communication about asset allocation, while Vanguard leverages its suite of key ETFs to craft personalized retirement plans.
These platforms continuously monitor market conditions and your portfolio, executing automatic rebalancing to preserve the desired risk-return profile. This real-time adjustment surpasses traditional periodic rebalancing by incorporating machine learning models that detect subtle shifts in market volatility and correlations across assets. Investment platforms such as E-Trade Core Portfolios and Fidelity Go offer these dynamic capabilities, often coupling algorithmic management with optional human advisor access. This hybrid approach blends automation with personalized guidance, ensuring you receive tailored support.
How AI Evaluates Risk and Manages Your Investment Portfolio
Risk assessment in AI-driven investing goes far beyond simple volatility calculations. It involves sophisticated models processing multi-dimensional data streams—from price movements and economic indicators to social sentiment and geopolitical developments.
Advanced AI techniques like deep learning neural networks and reinforcement learning algorithms empower robo-advisors to update risk scores dynamically. This adaptive capability allows them to respond to sudden market shocks or evolving user preferences effectively.
For example, firms such as Two Sigma and BlackRock’s Aladdin platform employ AI-powered portfolio optimization techniques. These systems use factor analysis and scenario simulations to forecast how portfolios might perform under diverse economic conditions, helping to balance growth opportunities with downside protection.
Still, no AI model is infallible. Financial markets are influenced by unpredictable human behaviors and rare black swan events that even the most advanced models struggle to anticipate. That’s why platforms emphasize continuous rebalancing and integrate safeguards—like risk limits and compliance checks—to prevent excessive exposure and protect your investments.
Using Generative AI for Scenario Simulation: Peering into Alternative Futures
A cutting-edge frontier in AI-driven investing is generative AI’s ability to simulate multiple potential investment outcomes. Unlike traditional predictive models that offer a single forecast, generative AI synthesizes historical data, macroeconomic trends, and emerging variables to create a range of plausible market scenarios.
Financial institutions such as JPMorgan Chase and BlackRock have integrated generative AI tools analyzing data from millions of financial instruments daily. These tools generate rich scenario analyses, enabling investors to explore “what-if” questions, for example:
- How would a sudden interest rate hike impact my portfolio?
- What if geopolitical tensions escalate unexpectedly?
This approach enhances strategic planning by quantifying potential risks and rewards across diverse futures.
However, these simulations are illustrative rather than definitive. The complexity of financial markets means even the most sophisticated generative models carry inherent uncertainty. Human judgment remains essential to interpret scenarios, assess assumptions’ plausibility, and align investment recommendations with your unique goals and values.
Algorithmic Trading and Market Prediction: Behind the AI Curtain
Algorithmic trading powers much of today’s automated investing, leveraging AI to execute trades at speeds and scales beyond human capability. The fundamental principle is straightforward: predefined rules and machine learning models analyze market conditions and execute buy or sell orders to optimize returns while minimizing risks.
Machine learning techniques like Long Short-Term Memory (LSTM) networks excel at modeling time-series data such as stock prices. By learning complex patterns and dependencies within historical market data, these models improve short-term price movement forecasts. Pioneers like Renaissance Technologies and Two Sigma harness vast datasets and AI to refine trading strategies continuously.
A critical technical challenge in this domain is mitigating bias in AI models. Biases can arise from skewed training data, overfitting to past trends, or inadvertently replicating market inefficiencies. For instance, an AI trained primarily on bull market data may underestimate risks during downturns. To counteract this, developers apply diverse data sourcing, regular model retraining, and fairness-aware algorithms to ensure that financial recommendations remain balanced and robust.
The Vital Role of Human Judgment Amid AI’s Rise
Despite AI’s impressive advances, it cannot fully replace human intuition and ethical reasoning in personalized investing. AI excels at processing data and identifying patterns but lacks the ability to comprehend your broader life context—your values, evolving priorities, and emotional responses to market fluctuations.
As highlighted by the World Economic Forum and other thought leaders, the future of wealth management is hybrid: AI equips advisors with deeper insights and enhances operational efficiency, while humans provide empathy, trust, and strategic oversight. This partnership is crucial to avoid pitfalls such as overreliance on opaque “black-box” AI systems or neglecting qualitative factors that influence sound investment decisions.
Moreover, human vigilance remains essential regarding AI’s limitations in bias and transparency. While AI can reduce costs and improve scalability, it may also perpetuate systemic biases or obscure decision-making logic. Investors should insist on clear explanations of how AI recommendations are generated and maintain an active role in reviewing and adjusting their strategies.
Key Takeaways
- Robo-advisors use AI-driven algorithms to construct portfolios aligned with your preferences and dynamically rebalance them in response to market changes.
- Advanced risk assessment models integrate diverse data sources, enabling continuous portfolio optimization but cannot foresee every market shock.
- Generative AI provides powerful scenario simulations that enrich investment planning but require human interpretation to contextualize uncertainties.
- Algorithmic trading leverages machine learning models like LSTM to forecast market movements; however, bias mitigation is crucial for trustworthy recommendations.
- Human judgment remains indispensable for interpreting AI insights, incorporating ethical considerations, and ensuring investment strategies reflect your unique goals.
In summary, AI is transforming personal investing from a largely manual, intuition-driven activity into a sophisticated, data-powered discipline. Like any tool, its effectiveness depends on thoughtful and transparent integration into the decision-making process. For investors willing to engage critically, AI offers a compelling ally to navigate today’s complex markets.
Aspect | Details | Examples / Platforms |
---|---|---|
AI-Powered Portfolio Construction | Algorithmic asset allocation integrating risk tolerance, investment horizon, and preferences; continuous automatic rebalancing using machine learning | Betterment, Vanguard Digital Advisor, E-Trade Core Portfolios, Fidelity Go |
Risk Assessment and Management | Multi-dimensional data processing including price, economic indicators, social sentiment, geopolitical factors; dynamic risk scoring using deep learning and reinforcement learning | Two Sigma, BlackRock Aladdin |
Generative AI for Scenario Simulation | Simulates multiple potential market outcomes using historical data and macroeconomic trends to answer “what-if” questions | JPMorgan Chase, BlackRock |
Algorithmic Trading & Market Prediction | Machine learning (e.g. LSTM networks) for time-series forecasting; bias mitigation via diverse data and fairness-aware algorithms | Renaissance Technologies, Two Sigma |
Human Judgment Role | Essential for interpreting AI insights, ethical considerations, emotional and contextual factors, avoiding overreliance on black-box AI | World Economic Forum emphasis on hybrid AI-human wealth management |
Key Takeaways | – AI enables dynamic portfolio rebalancing – Advanced risk models cannot predict all shocks – Generative AI enriches planning but requires human interpretation – Bias mitigation critical in algorithmic trading – Human judgment indispensable for ethical and personalized investing |
Troubleshooting and Mitigating Risks in AI-Based Financial Management
Troubleshooting and Mitigating Risks in AI-Based Financial Management
What happens when the AI tools that help manage your money get it wrong? Despite impressive advances in AI-powered personal finance apps, users can face persistent challenges—from inaccurate data interpretations to privacy concerns—that may impact their financial well-being. Navigating these risks effectively requires a combination of technical savvy, critical thinking, and human judgment.
Common Challenges in AI-Driven Financial Tools
AI excels at recognizing patterns and processing vast datasets rapidly, but it is not infallible. A frequent issue is inaccurate data interpretation. For instance, an AI budgeting app might flag a normal transaction as suspicious or recommend investment moves based solely on historical trends, without understanding your unique financial context or goals. Blindly following such alerts can lead to misguided decisions.
Another significant risk is overreliance on automated advice. Robo-advisors and budgeting apps efficiently handle routine tasks but lack the emotional intelligence and ethical judgment essential in finance. As one expert insight highlights, “Financial advice isn’t just about numbers—it’s about what those numbers mean to you.” AI cannot fully grasp your personal values, risk tolerance, or life changes.
Algorithmic bias is another thorny problem. AI models trained on historical financial data may perpetuate existing inequalities, especially in lending and credit assessments. Studies demonstrate that lending discrimination disproportionately affects Black and Brown communities, underscoring the ethical responsibility of both developers and users to scrutinize AI decision-making.
Practical Steps to Verify and Interpret AI Insights
How can you avoid blindly trusting AI-generated financial advice? The first rule is verification. Treat AI outputs as one input among many. Always cross-check recommendations against your financial goals and other trusted sources.
If an AI tool suggests reallocating your investments or drastically cutting budget categories, pause and ask yourself:
- Does this align with my long-term financial objectives?
- Have I factored in recent life events or changes the AI might not know about?
- What assumptions is the AI making based on my data?
When AI alerts seem off or conflict with your understanding, seek human advisory. Certified financial planners and advisors provide essential context, empathy, and fiduciary responsibility that AI cannot replicate. The future of wealth management lies in hybrid approaches where AI augments human expertise, rather than replacing it.
Protecting Your Privacy and Data Security
AI tools process your most sensitive financial information, making privacy not just a feature but a necessity. Unfortunately, many AI-powered apps operate with limited transparency about how your data is collected, stored, or shared, potentially exposing you to risks like identity theft or unauthorized profiling.
To safeguard your financial data:
- Choose AI financial apps from reputable providers with clear, robust data handling policies.
- Use strong, unique passwords and enable two-factor authentication.
- Regularly review app permissions and revoke access to unnecessary data.
- Be cautious about sharing sensitive documents or biometric data unless absolutely necessary.
- Keep your devices updated with the latest security patches to guard against cyberattacks.
Emerging best practices include data minimization—sharing only essential information—and sandboxing sensitive data to isolate it from broader AI training datasets. As generative AI models grow more complex, understanding how your data may be used or incorporated into training sets is critical.
Recognizing and Responding to AI Limitations
AI tools like robo-advisors and smart wallets are powerful but have blind spots. They rely heavily on historical data patterns and often struggle with unpredictable events such as market crashes or personal emergencies. For example, AI may fail to detect emotional spending triggered by stress—something a human advisor could address with empathy.
When you notice discrepancies between AI outputs and your financial goals, take these steps:
- Pause and analyze: Identify where the AI’s recommendation diverges from your priorities.
- Gather more information: Seek external data or opinions to validate or challenge the AI’s advice.
- Consult a financial professional: A human expert can contextualize AI insights and tailor advice to your situation.
- Adjust AI settings or inputs: Many tools allow customization to better reflect your risk tolerance or preferences.
- Report anomalies: If you suspect bias or malfunction, notify the provider. Transparent companies will investigate and improve their models.
Balancing Enthusiasm with Caution
AI is reshaping personal finance with remarkable capabilities—from real-time fraud detection to hyper-personalized budgeting. But it is not a magic bullet. Users must remain vigilant, combining AI’s analytical power with their own judgment and trusted human advice.
Think of AI as a sophisticated co-pilot rather than an autopilot. It can alert you to financial risks, suggest optimizations, and free up your time—but ultimate control rests with you. By verifying AI insights, integrating human expertise, securing your data, and critically interpreting AI outputs, you can harness this transformative technology safely and effectively. This thoughtful approach turns potential pitfalls into opportunities for smarter money management.
Category | Challenges/Risks | Mitigation Strategies |
---|---|---|
Data Interpretation | Inaccurate data interpretations; false alerts; recommendations based on historical trends without personal context | Verify AI outputs; cross-check with financial goals and trusted sources; consider personal context and recent life events |
Overreliance on Automation | Lack of emotional intelligence and ethical judgment; blind following of robo-advisor advice | Incorporate human advisory; combine AI insights with human expertise and judgment |
Algorithmic Bias | Bias in lending and credit assessments; perpetuation of existing inequalities | Scrutinize AI decision-making; report anomalies; advocate for ethical AI development and transparency |
Privacy & Data Security | Limited transparency on data handling; risks of identity theft and unauthorized profiling | Choose reputable apps; use strong passwords and two-factor authentication; review app permissions; practice data minimization; keep devices updated |
AI Limitations | Dependence on historical data; inability to handle unpredictable events; failure to detect emotional spending | Pause and analyze discrepancies; gather external data; consult financial professionals; adjust AI settings; report malfunctions |
User Approach | Potential overenthusiasm or blind trust in AI | Maintain vigilance; treat AI as a co-pilot; combine AI’s analytical power with personal judgment and human advice |
Advanced Techniques: Optimizing AI Use for Personalized Financial Growth and Ethical Considerations
Advanced Techniques: Optimizing AI Use for Personalized Financial Growth and Ethical Considerations
How can you move beyond basic budgeting apps and make AI a truly strategic ally in managing your personal finances? For experienced users, the next frontier involves integrating multiple AI-powered tools, customizing AI models to fit your unique financial profile, and leveraging behavioral finance algorithms that help identify and correct cognitive biases. Alongside these advancements, it’s essential to engage with AI ethically—understanding transparency, data ownership, and the broader societal impact of democratizing financial access.
Integrating and Customizing AI Tools for Holistic Financial Planning
Today’s market offers a rich ecosystem of AI-driven financial tools, each specializing in areas like budgeting, investing, credit risk assessment, fraud detection, and bookkeeping automation. Instead of relying on a single app, you can orchestrate a suite of AI services tailored to your financial goals.
For example, platforms like Rows enable users to connect multiple financial accounts and services through over 50 native integrations powered by APIs such as Plaid. This creates a unified dashboard where AI analyzes your cash flow, detects spending patterns, and predicts upcoming expenses. Meanwhile, AI investment platforms like Betterment and Wealthfront bring goal-based investing within reach by offering tax-efficient strategies, 24/7 accessibility, and unbiased advice—even for those without deep financial expertise.
For technically savvy users, APIs from AWS AI Services, IBM Watson, and Cohere provide the flexibility to build or customize AI financial assistants. These assistants can understand natural language queries, analyze market conditions, or process financial documents using computer vision. Such customization allows you to:
- Tailor insights and recommendations based on your specific risk tolerance and lifestyle.
- Automate complex tasks such as fraud detection and identity verification.
- Integrate diverse data streams in real-time for dynamic portfolio adjustments.
This modular approach mirrors the hybrid IT strategies adopted by financial institutions, combining on-premise systems with multicloud APIs to enhance resilience and agility. It’s not just about using AI—it’s about crafting an adaptable AI ecosystem that grows with you.
Leveraging Behavioral Finance Algorithms to Correct Cognitive Biases
Despite AI’s power to analyze vast datasets, human financial behavior often remains irrational. Cognitive biases like overconfidence, loss aversion, and anchoring can undermine even the most disciplined investor’s decisions. Combining AI with behavioral finance offers a promising solution.
Emerging AI systems now detect cognitive biases by analyzing your transaction history, investment choices, and even the language you use in financial planning conversations. For instance, JPMorgan’s Moneyball AI tool helps portfolio managers identify when they fall prey to such biases by comparing their behavior with historical market patterns. This feedback loop is invaluable for improving decision-making.
Beyond bias detection, AI platforms increasingly incorporate gamification and personalized nudges to encourage better financial habits. Imagine an AI assistant that not only alerts you when your spending deviates from your budget but also explains the emotional triggers behind those deviations and suggests practical corrective actions.
The future lies in hybrid models where AI augments human judgment rather than replaces it. While AI excels at pattern recognition and predictive analytics, it still lacks intuition for unprecedented events. Personal finance success remains a careful balance between machine insights and human wisdom.
Ethical Considerations: Transparency, Data Ownership, and Societal Impact
As AI becomes deeply embedded in managing personal finances, ethical questions become more urgent and practical. Who owns the data your AI tools collect? How transparent are the decision-making processes behind investment recommendations? What societal effects arise when AI democratizes financial access?
Data ownership is a critical battleground in 2025. Unlike the earlier decade’s scramble for attention, today’s technology providers focus on building trusted data relationships. However, regulatory frameworks remain fragmented, and many users are unaware of how their data is monetized or shared. Advocates call for clearer governance models that balance innovation with privacy and fairness.
Transparency in AI decision-making is equally crucial. Techniques such as SHAP (SHapley Additive exPlanations) and LIME (Local Interpretable Model-agnostic Explanations) help explain which features influence AI outputs, fostering trust and accountability. Without such clarity, users risk becoming passive recipients of opaque advice—especially dangerous in finance, where errors can have significant consequences.
On a societal level, AI-powered financial tools are lowering barriers to entry, enabling underserved populations to access budgeting assistance, credit scoring, and investment opportunities once reserved for the affluent. While this democratization is commendable, it also raises concerns about algorithmic bias, digital literacy gaps, and the risk of over-reliance on automated systems without sufficient human oversight.
Staying Ahead: Continuous Learning and Adaptation
The AI landscape in personal finance is evolving rapidly. To harness its full potential and navigate its challenges, ongoing education is essential. Engage with AI communities, webinars, and the latest research to stay informed about new capabilities, limitations, and ethical frameworks.
- Experiment with integrating new AI APIs and tools to refine your personal financial ecosystem.
- Stay updated on behavioral finance advancements and incorporate bias-correcting algorithms.
- Monitor regulatory changes and advocate for transparent, responsible AI practices.
Approach AI as a dynamic partner that requires your active involvement and critical thinking. By doing so, you position yourself not only to optimize your finances but also to contribute to a more equitable and trustworthy financial future.
In summary, mastering AI-driven personal finance today means building a tailored, integrated system informed by behavioral insights and grounded in ethical awareness. It is a sophisticated interplay between technology and humanity that can unlock unprecedented financial growth while safeguarding your autonomy and values.
Technique | Description | Examples / Tools | Benefits | Considerations |
---|---|---|---|---|
Integrating and Customizing AI Tools | Use multiple AI-driven financial tools tailored to individual goals and profiles, combining budgeting, investing, fraud detection, and more. | Rows (with Plaid integration), Betterment, Wealthfront, AWS AI Services, IBM Watson, Cohere | Unified dashboard, personalized insights, automation of complex tasks, real-time portfolio adjustments | Requires technical know-how for customization; balancing multiple APIs and data streams |
Behavioral Finance Algorithms | AI systems detect and correct cognitive biases affecting financial decisions by analyzing behavior and transaction data. | JPMorgan’s Moneyball, AI nudges and gamification tools | Improves decision-making, encourages better financial habits, addresses irrational behavior | AI augments but does not replace human intuition; requires user engagement |
Ethical Considerations | Focus on transparency, data ownership, and societal impact as AI becomes central in personal finance. | SHAP, LIME for explainability; regulatory frameworks for data governance | Builds trust and accountability; protects privacy; democratizes financial access | Fragmented regulations; risks of algorithmic bias and over-reliance on automation |
Continuous Learning and Adaptation | Ongoing education and experimentation to stay current with AI advancements and ethical practices. | AI communities, webinars, research, new AI APIs | Maintains optimized and responsible AI use; adapts to evolving landscape | Requires time and active involvement; need to monitor regulatory changes |