Guarding Your Privacy: Practical Defense Against AI Surveillance
- Introduction: Why Privacy Protection Matters in the Age of AI Surveillance
- Introduction: Why Privacy Protection Matters in the Age of AI Surveillance
- The Expanding Reach and Power of AI Surveillance
- The Stakes: What’s at Risk for Individuals and Society?
- Balancing AI’s Promise With Pragmatic Privacy Protection
- Prerequisites: Understanding AI Surveillance, Data Ecosystems, and Privacy Concepts
- Prerequisites: Understanding AI Surveillance, Data Ecosystems, and Privacy Concepts
- AI Surveillance Modalities: Beyond Facial Recognition
- Understanding Data Collection Pipelines and AI Data Dependencies
- Core Privacy Principles: Data Minimization, Anonymization, and Consent
- The Necessary Technical Foundation: Data Flows, Encryption, and Network Security
- Preparation: Assessing Your Digital Footprint and Privacy Exposure
- Preparation: Assessing Your Digital Footprint and Privacy Exposure
- Taking Inventory: Mapping Your Digital Presence
- Recognizing Implicit Data Collection: The Invisible Harvest
- Tools and Techniques for Data Inventory and Policy Evaluation
- Why Establishing a Privacy Baseline Matters
- Step-by-Step Instructions for Protecting Privacy Against AI Surveillance and Data Collection
- Step-by-Step Instructions for Protecting Privacy Against AI Surveillance and Data Collection
- 1. Minimize Data Sharing by Adjusting Device and App Settings
- 2. Employ Technical Tools to Disrupt AI Data Collection
- 3. Use Data Masking and Pseudonymization Where Applicable
- 4. Leverage AI-Aware Privacy Features: Opt-Out Mechanisms and Consent Management
- 5. Regularly Update Software and Security Practices
- Technical Explanations: Why These Privacy Measures Work Against AI Surveillance
- Technical Explanations: Why These Privacy Measures Work Against AI Surveillance
- Encryption: The Digital Lock on Your Data
- Anonymization: Blurring the Fingerprints AI Uses to Identify You
- Data Minimization: Giving AI Less to Work With, Limiting Its Reach
- Balancing AI Capabilities and Privacy Protections
- Troubleshooting Common Issues in Implementing AI Privacy Protections
- Troubleshooting Common Issues in Implementing AI Privacy Protections
- Navigating Incomplete Data Control and App Incompatibilities
- Balancing Privacy Protections with Usability and Managing Consent Fatigue
- Detecting Data Leakage and Monitoring Privacy Protection Effectiveness
- Final Thoughts
- Advanced Techniques and Future-Proofing Your Privacy in an Evolving AI Landscape
- Advanced Techniques and Future-Proofing Your Privacy in an Evolving AI Landscape
- Cutting-Edge Privacy-Enhancing Technologies: Promise and Limitations
- Navigating the Evolving Regulatory Landscape: GDPR, AI Acts, and Beyond
- Cultivating Proactive Privacy Habits: Staying Ahead of AI’s Curve
- Looking Ahead: Privacy as a Dynamic, Layered Endeavor
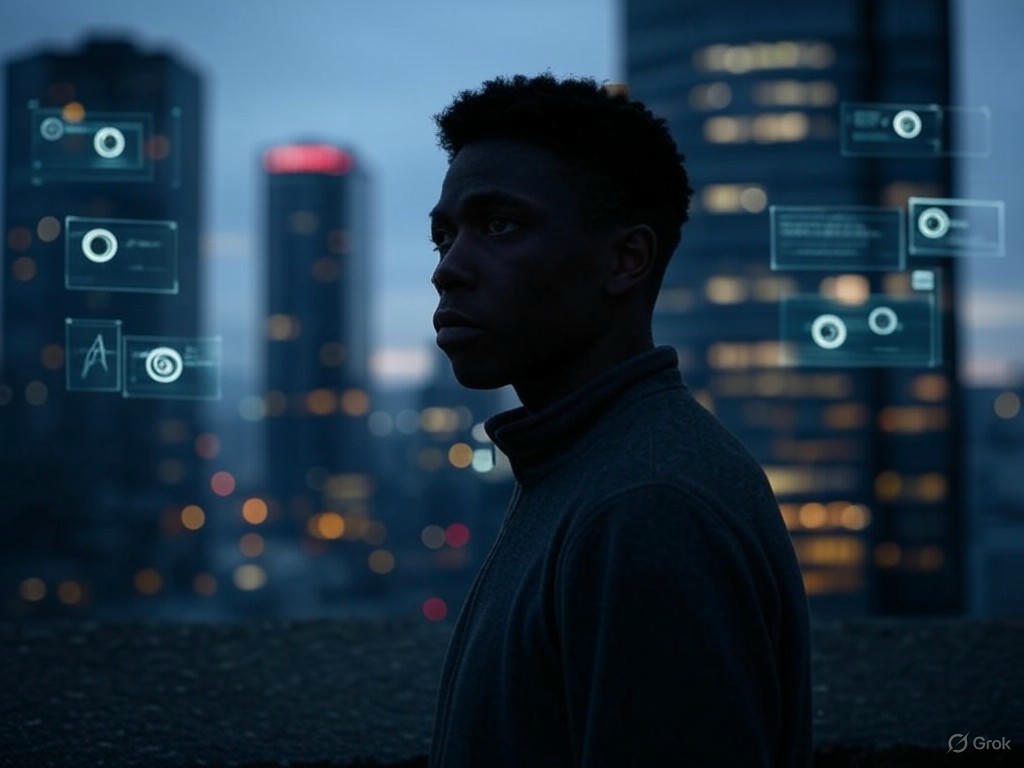
Introduction: Why Privacy Protection Matters in the Age of AI Surveillance
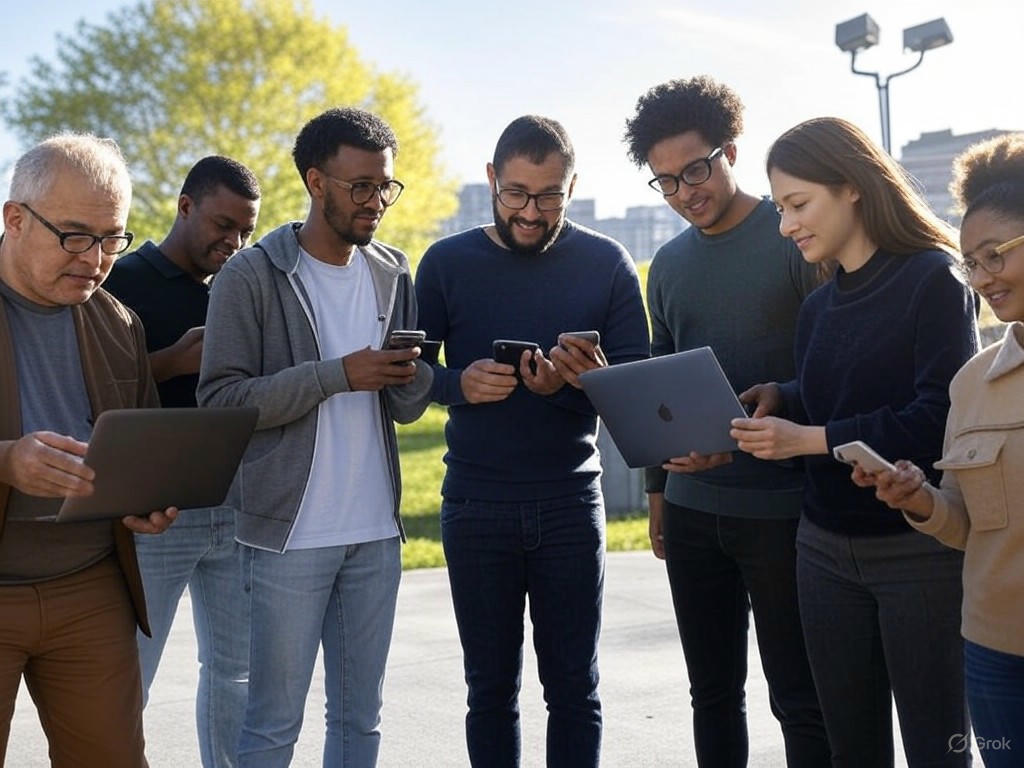
Introduction: Why Privacy Protection Matters in the Age of AI Surveillance
Imagine a world where every move you make, every interaction online or offline, is quietly observed, analyzed, and stored by intelligent systems far more capable than any human. In 2025, this is not science fiction—it is the reality shaped by the rapid advancement of AI surveillance and data collection technologies.
The Expanding Reach and Power of AI Surveillance
AI-driven surveillance has reached unprecedented levels of sophistication, powered by breakthroughs in computer vision, behavioral analytics, and cloud computing. Modern video analytics extend well beyond basic motion detection; they identify objects, track complex patterns, and analyze behaviors in real time.
For example, airports deploy facial recognition systems to expedite passenger screening, boasting accuracy rates exceeding 97% in controlled environments. Law enforcement agencies leverage AI to identify suspects and locate missing persons, often incorporating “liveness detection” to prevent spoofing attempts using deepfakes or masks, with accuracy surpassing 98%.
However, AI surveillance is not limited to cameras. Governments, particularly in the U.S., utilize AI to monitor social media activities of travelers and immigrants by scanning vast troves of public and semi-public data to flag anomalies or potential threats. Meanwhile, businesses invest billions in generative AI technologies that depend on enormous datasets, frequently collected without explicit user awareness or consent.
The scale of data collection is staggering. Organizations aggregate, clean, label, and even synthetically augment data to train AI models that influence nearly every aspect of daily life—from personalized marketing to predictive policing. As generative AI tools are embedded in 90% of companies worldwide, the footprint of AI surveillance and data collection grows exponentially.
The Stakes: What’s at Risk for Individuals and Society?
This vast, interconnected data ecosystem raises profound privacy concerns. AI systems that collect and process sensitive personal data—such as biometric identifiers, behavioral patterns, and social interactions—open the door to potential misuse and abuse.
Key risks include:
-
Opaque Data Practices: Individuals often lack visibility into what data is collected, how it is used, or with whom it is shared. This opacity erodes trust and leaves people vulnerable to profiling and manipulation.
-
Algorithmic Bias and Discrimination: AI trained on biased or incomplete datasets can perpetuate inequalities, disproportionately affecting marginalized communities in areas like law enforcement and employment.
-
Unauthorized Use and Security Breaches: AI models and the data they handle are targets for hacking, leading to leaks and malicious exploitation of personal information.
-
Legal and Ethical Ambiguities: Privacy regulations vary widely across jurisdictions, and rapid AI innovation frequently outpaces legislation, resulting in a fragmented patchwork of protections that can be challenging to navigate.
The consequences are tangible. Targeted advertising can verge on invasive profiling. Identity theft risk escalates when biometric data is compromised. Mass surveillance threatens fundamental freedoms and democratic values.
Balancing AI’s Promise With Pragmatic Privacy Protection
Despite these risks, AI’s transformative benefits are undeniable. From enhancing security operations with autonomous monitoring to streamlining business workflows through data-driven insights, AI delivers significant value.
The challenge is to strike a balance—embracing AI’s advantages while vigilantly safeguarding privacy. This requires:
-
Privacy-by-Design: Integrating privacy safeguards into AI systems from the outset, minimizing data collection to only what is strictly necessary.
-
Transparency and Consent: Empowering users with clear understanding of data flows and meaningful control over their personal information.
-
Robust Security Measures: Implementing strong encryption, multi-factor authentication, and real-time threat detection to protect sensitive data.
-
Ethical Governance and Accountability: Establishing frameworks that address bias, fairness, and compliance with evolving regulations like GDPR, CCPA, and emerging U.S. state laws.
This article aims to demystify the technical underpinnings of AI surveillance and data collection, cutting through hype and fear to provide actionable, evidence-based strategies. Whether you are a privacy advocate, a technology professional, or an everyday user, understanding these dynamics is essential for navigating a world where data is currency and AI is the banker.
Ultimately, protecting your privacy in 2025 and beyond is not about rejecting AI outright. It is about informed engagement—leveraging AI’s benefits while minimizing risks through smart, proactive choices. The following sections will equip you with both the technical insight and practical tools necessary to safeguard your personal data in this complex and evolving landscape.
Aspect | Description | Examples / Details | Impact / Risks | Mitigation Strategies |
---|---|---|---|---|
AI Surveillance Capabilities | Advanced monitoring using AI technologies | Facial recognition with >97% accuracy, liveness detection >98%, social media monitoring | Mass data collection, potential for misuse | Privacy-by-Design, transparency, consent |
Data Collection Scale | Aggregation and augmentation of huge datasets for AI training | Data labeling, synthetic augmentation, 90% company adoption of generative AI | Opaque data practices, increased risk of profiling | Clear data use policies, user empowerment |
Privacy Risks | Potential abuse of personal data | Algorithmic bias, unauthorized use, security breaches | Discrimination, identity theft, erosion of trust | Robust security, ethical governance, compliance with regulations |
Legal and Ethical Challenges | Fragmented and evolving privacy regulations | GDPR, CCPA, emerging U.S. state laws | Complex compliance landscape, legal ambiguities | Accountability frameworks, continuous monitoring |
Balancing AI Benefits and Privacy | Leveraging AI advantages while protecting privacy | Autonomous security, data-driven business insights | Potential privacy invasion if unchecked | Privacy-by-Design, user control, strong security |
Prerequisites: Understanding AI Surveillance, Data Ecosystems, and Privacy Concepts
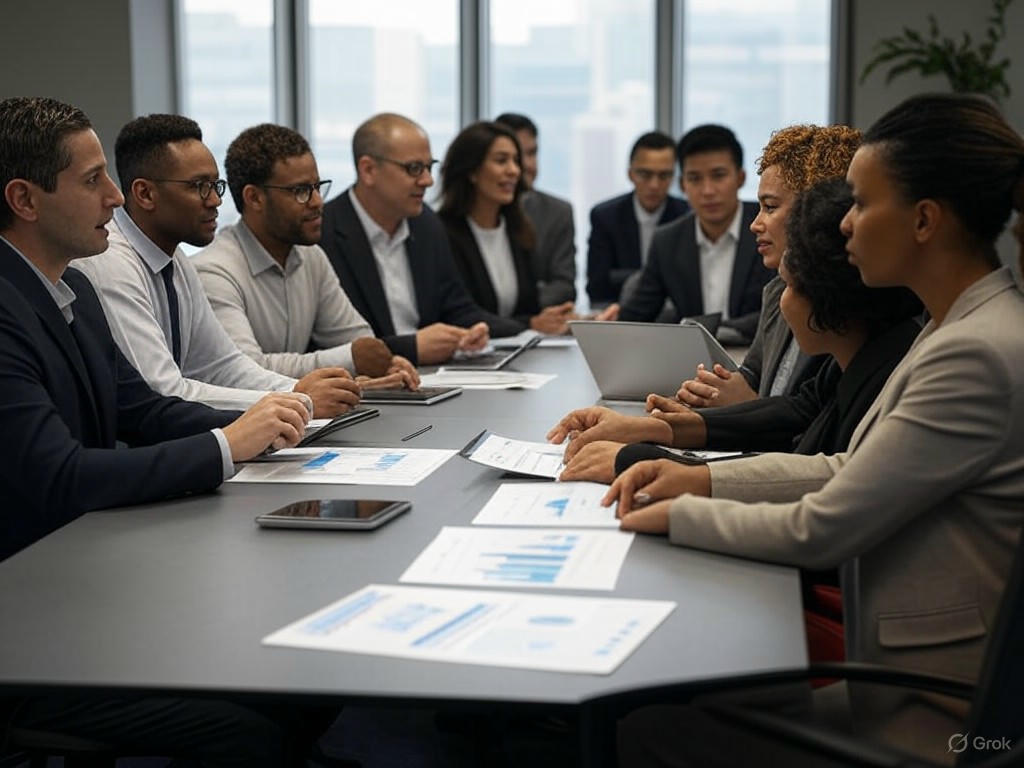
Prerequisites: Understanding AI Surveillance, Data Ecosystems, and Privacy Concepts
Before diving into privacy protection strategies, it’s essential to clarify what we mean by AI surveillance, data ecosystems, and key privacy concepts. These terms are often used broadly, but understanding their technical foundations empowers you to better assess risks and defenses in today’s AI-driven environment.
AI Surveillance Modalities: Beyond Facial Recognition
Facial recognition is perhaps the most familiar AI surveillance technology. It identifies or verifies individuals by scanning distinctive facial features, widely used in airports for passenger screening, law enforcement for suspect identification, and banking for secure authentication. For example, the U.S. Department of Homeland Security reports a 97% success rate in face matching across demographic groups in controlled airport settings, highlighting both its accuracy and extensive deployment.
However, challenges remain. Facial recognition systems exhibit documented biases, disproportionately affecting people of color, women, and nonbinary individuals, raising concerns about fairness and privacy. Many companies claim trade secrecy to withhold performance data, which limits transparency and independent validation.
Beyond facial recognition, behavioral analytics is a growing surveillance modality that leverages AI to monitor patterns of movement, interactions, or online activities. By analyzing sequences and anomalies in real time, these systems infer intent or detect suspicious behavior. Similarly, video analytics use advanced motion detection and object recognition to support proactive crowd management and security threat identification.
These AI surveillance technologies depend on vast, varied data inputs, meaning privacy concerns extend beyond the algorithms to the underlying data collection and processing infrastructure.
Understanding Data Collection Pipelines and AI Data Dependencies
Think of a data pipeline as a factory assembly line—but instead of producing physical goods, it processes data. These automated workflows collect, clean, transform, and route data from raw sources to AI models or actionable insights.
Modern AI data pipelines handle enormous volumes of both structured and unstructured data, including images, videos, GPS signals, and sensor readings. For instance, autonomous vehicles generate massive real-time streams that require sub-second latency processing, demanding highly scalable and reliable pipelines.
AI models rely heavily on training datasets—curated collections teaching the AI to recognize patterns or make predictions. These datasets often contain personal identifiable information (PII) such as full names, Social Security numbers, biometric data, and related metadata like timestamps or geolocation tags.
PII can be sensitive (e.g., financial or medical records) or non-sensitive (e.g., publicly available phone numbers). Mishandling this data increases risks of identity theft and fraud, as starkly illustrated by incidents like the 2017 Equifax breach exposing 147 million records or the Cambridge Analytica scandal affecting over 50 million Facebook users.
Therefore, data pipelines must be designed not only for efficiency but also with robust privacy safeguards, ensuring responsible handling and secure storage of PII and metadata.
Core Privacy Principles: Data Minimization, Anonymization, and Consent
How much data should organizations collect? The principle of data minimization advises collecting only the data strictly necessary for a defined purpose. This approach reduces exposure to breaches, limits bias, and simplifies regulatory compliance, in line with the European GDPR’s purpose limitation.
Anonymization goes further by transforming data to prevent identification of individuals, even when datasets are combined. Techniques include differential privacy, data masking, synthetic data generation, and structural transformations. However, achieving true anonymization is technically challenging due to risks like linkage or re-identification attacks.
At the ethical core is consent—individuals must be informed and voluntarily agree to how their data is collected and used. Modern consent management platforms (CMPs) have evolved beyond cookie banners into sophisticated systems that manage, audit, and enforce user permissions in compliance with laws like GDPR and the California Consumer Privacy Act (CCPA).
In regulated sectors such as finance, consent is often combined with biometric authentication—with 87% of global banks employing biometrics alongside multi-layered consent frameworks to meet stringent regulatory requirements.
The Necessary Technical Foundation: Data Flows, Encryption, and Network Security
To fully understand and implement effective privacy protections against AI surveillance and data collection, a foundational grasp of data flows and security technologies is essential.
Data flows describe the pathways data takes—from your devices, through servers, networks, and cloud platforms, to AI systems. Each stage represents a potential vulnerability where data could be intercepted or misused.
Encryption acts as a digital lockbox, encoding data so only authorized parties can access it. It protects data both in transit (e.g., via TLS when sending messages or payments) and at rest (stored on servers or devices). Emerging threats like quantum computing challenge current encryption standards, prompting experts to advocate for transitioning to quantum-resistant algorithms to future-proof data security.
Network security employs multiple layers of defense including software firewalls, intrusion detection systems, and access controls. The foundational CIA triad—Confidentiality, Integrity, and Availability—guides these efforts to ensure data remains private, unaltered, and accessible only to legitimate users.
Newer practices such as micro-segmentation (dividing networks into secure zones) and zero trust architectures (assuming no implicit trust, verifying every access) are increasingly adopted to strengthen defenses in complex cloud and hybrid environments.
Understanding these technical and ethical fundamentals is more than academic. It equips you to critically evaluate how your data is collected, processed, and protected in an AI-pervasive world. This foundation sets the stage for practical strategies to safeguard your privacy against increasingly sophisticated AI surveillance and data collection practices.
Category | Details | Examples / Notes |
---|---|---|
AI Surveillance Modalities | Technologies using AI to monitor and identify individuals or behaviors | Facial recognition (97% accuracy in airports), Behavioral analytics, Video analytics |
Challenges in AI Surveillance | Bias, lack of transparency, privacy concerns | Bias affecting minorities and women, trade secrecy limiting validation |
Data Collection Pipelines | Automated workflows processing raw data into usable AI inputs | Handles structured/unstructured data like images, GPS, sensor data; requires scalability and reliability |
AI Data Dependencies | Training datasets often containing PII and metadata | PII includes names, SSNs, biometrics; metadata includes timestamps, geolocation |
Privacy Risks | Identity theft, fraud due to mishandling of sensitive data | Equifax breach (147 million records), Cambridge Analytica (50 million users) |
Core Privacy Principles | Data minimization, anonymization, consent | GDPR purpose limitation, differential privacy, consent management platforms (CMPs) |
Consent and Authentication | Informed user consent and biometric authentication for regulated sectors | 87% of global banks use biometrics with multi-layered consent |
Technical Foundations | Understanding data flows, encryption, network security | Encryption in transit and at rest; quantum-resistant algorithms; CIA triad; micro-segmentation; zero trust |
Preparation: Assessing Your Digital Footprint and Privacy Exposure
Preparation: Assessing Your Digital Footprint and Privacy Exposure
How well do you really understand the digital shadow you cast every day? Before you can effectively shield yourself from AI surveillance and pervasive data collection, it’s essential to perform a comprehensive audit of your digital footprint. This process reveals where your personal information resides—often invisibly—and highlights potential vulnerabilities.
Taking Inventory: Mapping Your Digital Presence
Your digital footprint extends far beyond what you explicitly share online. It encompasses every click, login, app permission, and device interaction—forming a complex mosaic of data. Experts estimate that by 2025, the average individual’s data trail spans multiple devices, platforms, and cloud services, each representing a potential point of exposure.
Begin your audit by cataloging:
- Devices: Smartphones, laptops, tablets, smart TVs, wearables, and Internet of Things (IoT) gadgets.
- Online accounts: Social media profiles, email addresses, cloud storage, e-commerce platforms, subscription services, and professional networks.
- Apps and permissions: Review the personal data your installed apps request. Regularly check privacy settings and revoke permissions that aren’t necessary.
Additionally, routinely searching your name on major search engines and setting up alerts (such as Google Alerts) can help you monitor what personal information is publicly accessible. This vigilance is crucial; for instance, one in ten university admissions officers reportedly rescinded offers based on inappropriate social media content. Curating a professional and privacy-conscious online presence is therefore not only wise but can also have significant real-world consequences.
Recognizing Implicit Data Collection: The Invisible Harvest
Not all data collection is transparent or explicit. AI systems embedded within IoT devices, smart assistants, and social media algorithms collect vast amounts of passive data, frequently without your direct awareness.
Consider the following examples:
-
IoT devices: By 2025, over 40 billion connected IoT devices—including smart fridges and home security cameras—will be operational worldwide. Many of these devices lack standardized security protocols, making them vulnerable points for data collection. Isolating such devices on separate networks and limiting their access permissions can mitigate risk.
-
Smart assistants and sensors: Devices like voice-activated assistants continuously listen or monitor environmental cues, generating extensive behavioral data. While they offer convenience, they also feed AI systems that analyze your habits, preferences, and routines silently in the background.
-
Social media platforms: AI algorithms on platforms such as Instagram, TikTok, and LinkedIn track detailed metadata—including content viewing duration, engagement patterns, and location data. For example, Instagram and TikTok monitor how long you view specific content, while LinkedIn tailors feeds based on your interaction history.
Understanding these implicit data flows is critical because conventional privacy settings often fail to guard against such passive surveillance. It’s akin to locking your front door but leaving the windows wide open.
Tools and Techniques for Data Inventory and Policy Evaluation
Establishing a privacy baseline requires not only knowing what data exists but also comprehending who controls it and how it is shared. Here’s a systematic approach to help you build that baseline:
-
Leverage privacy checkup tools: Major platforms offer built-in tools—Google Dashboard, Facebook’s Privacy Checkup, and others—that allow you to review and adjust data collection and sharing preferences. Regular audits of these settings help minimize unnecessary exposure.
-
Utilize data inventory software: Although traditionally designed for enterprises, tools like DataGrail and BigID now cater to individuals and small businesses by providing automated data discovery and mapping. These solutions classify data types, track vendor risks, and identify security or compliance gaps.
-
Scrutinize privacy policies and data-sharing agreements: Such documents specify what data is collected, how it’s used, and with whom it’s shared. Given the complex regulatory landscape—including California’s CPRA, the EU’s GDPR, and emerging laws in Montana and Texas—it’s vital to understand your rights and the obligations of data controllers.
Key aspects to watch for in these policies include:
- Data retention durations and rights to deletion
- Third-party data sharing and selling practices
- Security measures and breach notification protocols
- Opt-out provisions and data access request procedures
-
Set a personal data retention policy: Decide which types of personal information you are comfortable having stored and for how long. Many services offer options for automatic data deletion or allow you to download and erase your data entirely.
Why Establishing a Privacy Baseline Matters
Without a clear understanding of your data landscape, privacy efforts are essentially a shot in the dark. A well-defined privacy baseline provides:
-
Visibility: Knowing what data exists and where it resides is your first line of defense against unauthorized use or breaches.
-
Control: Awareness empowers informed decisions—whether that means deleting accounts, tightening app permissions, or opting for privacy-focused alternatives.
-
Compliance: For professionals and businesses, adherence to evolving regulations depends on accurate data inventories and governance frameworks.
Think of this process as conducting a home security audit. You wouldn’t install alarms and locks before identifying which doors and windows are vulnerable. Similarly, assessing your digital footprint lays the foundation upon which all effective privacy protections are built.
Armed with this inventory and understanding, you are now prepared to implement concrete technical and behavioral measures to shield yourself from intrusive AI surveillance and data exploitation. But every journey begins with knowing your digital self.
Category | Examples / Details | Notes |
---|---|---|
Devices | Smartphones, laptops, tablets, smart TVs, wearables, IoT gadgets | Multiple devices represent potential exposure points |
Online Accounts | Social media profiles, email addresses, cloud storage, e-commerce platforms, subscription services, professional networks | Catalog all accounts to know where data resides |
Apps and Permissions | Installed apps with data access requests | Regularly review and revoke unnecessary permissions |
Implicit Data Collection | IoT devices, smart assistants, social media AI algorithms | Often passive and invisible data harvesting |
Privacy Checkup Tools | Google Dashboard, Facebook Privacy Checkup | Built-in tools for reviewing and adjusting settings |
Data Inventory Software | DataGrail, BigID | Automated data discovery and mapping tools |
Privacy Policies to Scrutinize | Data retention, third-party sharing, security, opt-out provisions | Understand rights and vendor obligations |
Personal Data Retention Policy | Decide data storage duration and deletion preferences | Set personal limits for data retention |
Step-by-Step Instructions for Protecting Privacy Against AI Surveillance and Data Collection
Step-by-Step Instructions for Protecting Privacy Against AI Surveillance and Data Collection
AI surveillance and data collection are no longer distant concerns—they are deeply embedded in the devices and services we use every day. However, by taking deliberate, phased actions, you can disrupt these pervasive data flows and regain control over your personal information. This practical plan, rooted in 2025’s latest privacy research and technological advances, outlines clear steps to protect your privacy effectively.
1. Minimize Data Sharing by Adjusting Device and App Settings
Many users underestimate how much personal data their smartphones and apps share by default. Consider that the personal data harvested from smartphones fuels a $14 billion market, incentivizing companies to collect as much information as possible.
Key steps to reduce exposure include:
-
Regularly audit app permissions. Many apps request access to sensitive features like your microphone, camera, precise location, and contacts without clear justification. For example, weather apps rarely require exact location data; switching these to “approximate” or disabling them entirely limits unnecessary data sharing. Both iOS and Android provide granular controls under Settings > Privacy > Permissions.
-
Disable personalized ads and tracking. Both Google and Apple offer settings to opt out of ad personalization and limit activity tracking. On Android, turning off “Web & App Activity” and opting out of personalized ads curtails data collection for targeted advertising.
-
Restrict location services. Location data is particularly valuable for AI models analyzing behavioral patterns. Disable location access for apps that don’t explicitly need it. On iOS, navigate to Settings > Privacy & Security > Location Services; Android provides comparable settings.
-
Use privacy-focused password managers. Avoid saving passwords in browsers that synchronize with cloud services, which may expose your credentials. Tools like Bitwarden and 1Password offer encrypted vaults without tracking your usage, enhancing security.
Tightening these settings effectively closes the “windows” through which AI systems collect detailed user profiles, reducing the granularity and volume of data they can harvest.
2. Employ Technical Tools to Disrupt AI Data Collection
Device and app settings form the foundation, but layering technical tools adds robust defense by obscuring and encrypting your digital footprint.
Recommended privacy tools:
-
Use a reputable VPN service. VPNs encrypt your internet traffic and mask your IP address, preventing AI systems and trackers from linking your online activity to your identity or location. Leading 2025 options include NordVPN, ExpressVPN, and Proton VPN, all audited for strong no-logs policies and high-speed connections. For instance, NordVPN’s NordLynx protocol combines rapid speeds with advanced encryption.
-
Adopt encrypted messaging apps. Signal remains the gold standard with end-to-end encryption and minimal metadata retention. Unlike WhatsApp or Telegram, Signal does not store your contact list or message history on servers. For users seeking enhanced anonymity, decentralized apps like Briar or Session provide communication without centralized data storage.
-
Switch to privacy-focused browsers. Browsers such as Brave and Firefox (enhanced with privacy extensions) block trackers, fingerprinting, and ads by default. Brave’s Basic Attention Token (BAT) system even compensates users for opting into privacy-respecting ads, reversing the traditional surveillance model. For highest anonymity, Tor Browser routes traffic through volunteer nodes, obscuring IP addresses and browsing habits.
-
Install tracker and script blockers. Extensions like uBlock Origin and Privacy Badger prevent unseen trackers from monitoring your web activity, limiting AI’s ability to build behavioral profiles.
These technical tools act like digital cloaks, significantly complicating AI systems’ attempts to profile or surveil you.
3. Use Data Masking and Pseudonymization Where Applicable
In professional or research contexts, where data sharing is unavoidable, data masking and pseudonymization provide critical privacy safeguards.
-
Data masking replaces sensitive information (e.g., names, social security numbers) with realistic but fictitious data. This allows AI models to analyze trends without exposing actual personal details.
-
Pseudonymization substitutes identifiers with pseudonyms, enabling data analysis while breaking direct identity links.
Platforms like K2view dynamically mask sensitive data during testing and analytics, aligning with regulatory demands such as GDPR’s purpose limitation and data minimization principles.
While these techniques do not eliminate re-identification risks entirely, they introduce meaningful barriers, reducing the likelihood of misuse or leaks by AI systems.
4. Leverage AI-Aware Privacy Features: Opt-Out Mechanisms and Consent Management
As AI systems evolve, so do privacy tools designed to give users granular control over data collection and usage.
-
Exercise opt-out options. Many AI platforms now provide settings to exclude your data from model training or delete interaction histories. For example, some generative AI services allow you to opt out of contributing your data to their training datasets.
-
Use AI-powered consent management platforms (CMPs). Tools like Google’s Consent Mode, OneTrust, and Enzuzo automatically detect cookies and trackers, block non-essential ones until you grant permission, and maintain compliance audit trails. These platforms simplify navigating complex consent dialogs and reduce “consent fatigue” caused by repetitive prompts.
-
Adopt user-centric consent experiences. AI-powered CMPs tailor consent requests to your preferences and context, helping prevent blind acceptance of invasive tracking and ensuring your privacy choices are informed and respected.
This shift toward dynamic, AI-aware privacy controls counters indiscriminate data harvesting and strengthens your autonomy over personal information.
5. Regularly Update Software and Security Practices
Outdated software is the easiest gateway for unauthorized data collection and AI surveillance breaches. The interval between vulnerability disclosure and exploitation has shrunk dramatically—sometimes to just hours.
-
Keep your operating system, applications, and firmware up to date. Updates patch security flaws that attackers or AI surveillance systems could exploit.
-
Avoid fake update scams. Always update software through official channels to prevent malware disguised as legitimate patches.
-
Use reputable security software. Modern antivirus and endpoint protection suites incorporate AI-driven threat detection to identify emerging attack vectors swiftly.
-
Implement multi-factor authentication (MFA). MFA adds layers of defense, reducing the risk of unauthorized access even if passwords are compromised.
Consistent vigilance in software maintenance and security hygiene acts as fortified locks and alarms on your digital environment, protecting against sophisticated AI-driven intrusions.
Each of these steps builds upon the others, creating a layered defense against AI surveillance and data collection. While no single measure guarantees absolute privacy, together they substantially elevate the barriers, limiting AI systems’ ability to track, profile, or exploit your data without your knowledge.
In 2025, protecting your privacy is both a technical necessity and an ethical commitment. Armed with these strategies and tools, you can reclaim agency in a data-driven world increasingly shaped by artificial intelligence.
Step | Description | Key Actions / Tools | Benefits |
---|---|---|---|
1. Minimize Data Sharing by Adjusting Device and App Settings | Reduce personal data exposure by managing permissions and settings on devices and apps. |
|
Limits data collection windows; decreases granularity and volume of shared data |
2. Employ Technical Tools to Disrupt AI Data Collection | Use software and services to obscure and encrypt digital footprints. |
|
Encrypts traffic; blocks trackers; prevents profiling and surveillance |
3. Use Data Masking and Pseudonymization Where Applicable | Protect sensitive information in professional or research data sharing. |
|
Reduces risk of identity exposure; aligns with GDPR and data minimization |
4. Leverage AI-Aware Privacy Features: Opt-Out Mechanisms and Consent Management | Use AI-driven privacy controls to manage consent and data usage preferences. |
|
Enhances control over data sharing; prevents blind acceptance of tracking |
5. Regularly Update Software and Security Practices | Maintain software hygiene to close vulnerabilities exploited by AI surveillance. |
|
Protects against unauthorized access; reduces risk from exploits and scams |
Technical Explanations: Why These Privacy Measures Work Against AI Surveillance
Technical Explanations: Why These Privacy Measures Work Against AI Surveillance
What really stops AI systems from snooping on your data? The answer lies in how privacy measures disrupt the AI data pipeline—from the moment data leaves your device, to how it’s stored and analyzed. Understanding the technical foundation of encryption, anonymization, and data minimization reveals why these protections remain critical even as AI capabilities advance rapidly.
Encryption: The Digital Lock on Your Data
Encryption transforms readable data into an unreadable format using complex mathematical algorithms and secret keys—much like sending a letter in a secret code only the recipient can decipher. It protects your data both in transit and at rest:
-
In transit: When you send messages, make payments, or upload files, encryption protocols such as TLS (Transport Layer Security) scramble the data. This ensures that AI surveillance systems monitoring network traffic see only indecipherable gibberish, keeping your communications confidential.
-
At rest: Data stored on servers or devices is encrypted, so even if an unauthorized AI system gains access, the information remains unintelligible without the corresponding decryption keys.
Modern encryption standards—such as those mandated by PCI DSS 4.0.1—require strong algorithms and regular key rotation to close vulnerabilities. Weak or outdated encryption invites AI-powered attackers to exploit cracks and reconstruct sensitive information.
Exciting advancements like Fully Homomorphic Encryption (FHE) enable AI models to perform computations directly on encrypted data without decrypting it first. For example, researchers at NYU demonstrated high-resolution object detection on encrypted images, allowing AI to analyze content without exposing it. While promising for privacy-preserving AI services, FHE remains computationally intensive and is not yet widely adopted.
Still, encryption is not invulnerable. The rise of quantum computing threatens to break current public-key cryptography, driving the need for quantum-resistant algorithms. Additionally, client-side scanning proposals—where AI inspects data before encryption—pose potential privacy trade-offs by exposing data prior to protection.
Anonymization: Blurring the Fingerprints AI Uses to Identify You
AI surveillance thrives by linking disparate data points to profile individuals. Anonymization breaks these links by removing or obscuring personally identifiable information (PII), making it nearly impossible for AI to connect datasets back to specific people.
Effective anonymization goes beyond simply deleting names or IDs. Advanced techniques include:
-
Differential Privacy: Adds carefully calibrated statistical noise to datasets, ensuring that the presence or absence of any individual’s data does not significantly affect analysis outcomes. This approach is increasingly integrated into AI frameworks like Google’s TensorFlow Privacy and adopted by organizations such as the US Census Bureau and Uber.
-
Data Masking and Synthetic Data: Tools like Informatica Persistent Data Masking replace sensitive data with realistic but fictitious values. Similarly, synthetic data replicates the statistical properties of real data without containing actual personal information, enabling AI training without compromising privacy.
-
Structural Transformations: Platforms such as K2view restructure data to remove direct identifiers while retaining analytical usefulness, striking a balance between utility and privacy.
However, anonymization is a continual arms race. Linkage attacks—where anonymized data is cross-referenced with other datasets—pose re-identification risks. Hence, combining multiple anonymization methods, adhering to regulatory compliance, and ongoing monitoring are essential defenses.
Anonymization is particularly crucial in sensitive sectors like healthcare and finance, where balancing data utility and privacy is mandatory for responsible AI use.
Data Minimization: Giving AI Less to Work With, Limiting Its Reach
Reducing the amount of personal data collected and stored is one of the most effective privacy strategies. Data minimization limits AI’s exposure to sensitive information, thereby lowering privacy risks and mitigating bias in AI models.
The European Union’s GDPR enshrines principles of purpose limitation and data minimization, requiring organizations to justify data collection and restrict usage to what is strictly necessary. For instance, life sciences companies using real-world evidence data for AI training must ensure data relevance and minimal scope.
From a technical standpoint, data minimization offers several benefits:
-
Reduces attack surface: Less data means fewer opportunities for adversaries to exploit or for AI to infer private details.
-
Limits bias amplification: AI models trained on excessive or irrelevant data can inherit or amplify biases, leading to unfair outcomes. Minimization emphasizes data quality over quantity.
-
Simplifies compliance and accountability: Clear data governance and documentation ease regulatory audits and risk management.
Nonetheless, AI systems are not passive recipients of data. Adversarial techniques such as data poisoning—maliciously injecting biased or corrupted data into training sets—can manipulate AI behavior. Other threats like prompt injection and obfuscation tactics (e.g., zero-width characters, emoji smuggling) aim to trick AI into revealing or misusing protected data.
The practical impact of such attacks varies, but they highlight the importance of robust, layered defenses combining technical safeguards, continuous monitoring, and human oversight.
Balancing AI Capabilities and Privacy Protections
AI’s power to process vast datasets and detect subtle patterns makes it a formidable surveillance tool—but also a double-edged sword for privacy.
-
Encryption and anonymization disrupt AI’s data ingestion pipelines by rendering data unreadable or unlinkable.
-
Data minimization restricts unnecessary data flow to AI models, curbing their ability to learn sensitive information.
However, no technique is foolproof. Advances in AI-driven de-anonymization, side-channel attacks, or metadata exploitation can erode these protections.
The path forward involves combining cryptographic innovations like FHE, rigorous anonymization standards leveraging differential privacy, and principled data governance enforcing minimization and purpose limitation.
Think of these privacy measures as layers of fog obscuring AI’s view. They don’t guarantee invisibility but make surveillance exponentially harder and more resource-intensive. For individuals and organizations seeking to stay ahead, understanding how these technical barriers shape AI’s limits is essential for informed privacy defense.
By grasping the interplay between AI capabilities and privacy technologies, we cut through hype and gain realistic expectations. Encryption builds your data fortress, anonymization erases your footprints, and data minimization narrows AI’s hunting grounds. Together, they form a resilient shield—one demanding ongoing vigilance but offering tangible protection in an era defined by AI’s reach.
Privacy Measure | How It Works | Technical Details / Examples | Limitations / Threats |
---|---|---|---|
Encryption | Transforms readable data into unreadable format using algorithms and keys |
|
|
Anonymization | Removes or obscures personally identifiable information (PII) to break data links |
|
|
Data Minimization | Limits amount of personal data collected and stored |
|
|
Troubleshooting Common Issues in Implementing AI Privacy Protections
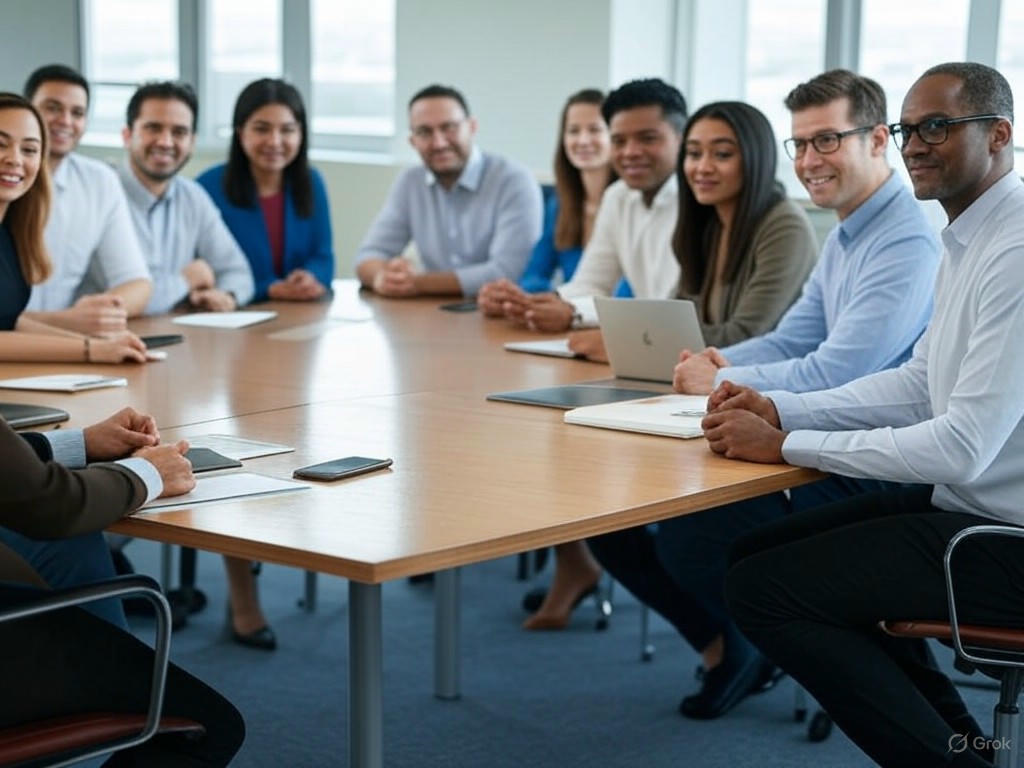
Troubleshooting Common Issues in Implementing AI Privacy Protections
Deploying AI-powered privacy protections often reveals that theoretical frameworks meet complex, real-world challenges. Issues such as incomplete data control on third-party platforms, the tension between privacy and usability, and detecting hidden data leaks are more than minor hurdles—they critically influence the success of privacy strategies. This section unpacks these common obstacles and offers practical solutions grounded in 2025 realities.
Navigating Incomplete Data Control and App Incompatibilities
A major challenge is the fragmented regulatory landscape and the sprawling web of third-party platforms involved in data handling. In the United States, the absence of a comprehensive federal privacy law means compliance depends on a patchwork of sector-specific and state-level regulations. California’s Consumer Privacy Act (CPRA), effective since 2023, grants broad rights and responsibilities to data controllers and processors. Likewise, states such as Utah, Montana, Texas, and Iowa have enacted diverse privacy laws that businesses must navigate carefully.
This mosaic complicates enforcement and, crucially, limits the control individuals or organizations have once data leaves their immediate environment. Third-party vendors often operate under different legal frameworks, making consistent privacy policy implementation difficult.
Adding to this complexity are technical challenges. Many privacy tools face app incompatibilities; for example, a browser extension may block trackers effectively on desktop browsers but falter on mobile apps due to platform constraints. Recent studies highlight these gaps, revealing that some privacy-enhancing technologies (PETs) fail to provide uniform protection across all user touchpoints.
To mitigate these issues:
-
Adopt comprehensive third-party risk management (TPRM) platforms. Tools like UpGuard and OneTrust offer automated scanning and continuous risk profiling to assess third-party compliance and vulnerabilities effectively.
-
Leverage advanced PETs employing cryptographic methods and data masking. These technologies secure data shared with external parties, reducing exposure even when direct control is limited.
-
Prefer vendors with strong privacy scores and transparent data practices. Conduct thorough due diligence, request detailed documentation, and reconsider partnerships with non-compliant or opaque vendors.
-
Stay current with jurisdictional regulations and align compliance strategies accordingly. For instance, the extraterritorial scope of the EU AI Act imposes strict standards on companies serving EU residents regardless of their base location.
The essential takeaway: Privacy protections are only as robust as the weakest link in the data chain. Vigilant management of third-party risks and ensuring tool compatibility are critical.
Balancing Privacy Protections with Usability and Managing Consent Fatigue
Protecting privacy should not come at the cost of user frustration. Consent fatigue—a phenomenon where users become overwhelmed by frequent cookie pop-ups and data permission requests—undermines meaningful control. When consent prompts become routine annoyances, users often dismiss them carelessly, inadvertently enabling extensive data collection.
To address consent fatigue while maintaining privacy goals, organizations should:
-
Implement granular, user-friendly consent mechanisms. Offer clear, concise options that empower users to make informed, selective choices rather than broad, all-or-nothing consents.
-
Utilize intelligent consent management tools such as Google Consent Mode v2, which aligns with GDPR and the Digital Markets Act to minimize repetitive prompts by managing consent intelligently across Google and third-party platforms.
-
Explore gamified user experience designs. Incorporating game elements—like points, progress indicators, and interactive visualizations—has shown promise in enhancing user engagement and comprehension during consent interactions.
-
Maintain transparency and build user trust. Clearly communicate why data is collected and how it will be used, reassuring users and reducing skepticism.
Beyond consent, detecting hidden or unauthorized data collection remains vital. AI-driven OSINT tools enable organizations to monitor digital footprints proactively, uncovering obscure or unauthorized data harvesting that traditional privacy controls might miss.
Ultimately, privacy measures must respect user experience to be sustainable. Overburdening users with frequent consent requests risks desensitization and erosion of trust, while thoughtful, transparent approaches encourage active, informed participation.
Detecting Data Leakage and Monitoring Privacy Protection Effectiveness
Data leakage is a subtle yet costly threat. Gartner estimates that poor data quality and undetected leaks cost organizations an average of $15 million annually, with 68% of data issues remaining unnoticed until they cause significant harm.
AI systems themselves are both targets and potential sources of leakage. Vulnerabilities or misconfigurations can expose sensitive information, while malicious actors may poison AI training data to corrupt model outputs or extract confidential data.
To stay ahead of these risks, organizations should:
-
Deploy advanced data leak detection solutions capable of monitoring both internal and third-party environments. These tools scan dark web sources, public code repositories, and other channels for exposed credentials or sensitive information. Leading platforms include UpGuard, BitSight, and Recorded Future.
-
Implement AI-powered anomaly detection agents that analyze data flows to flag suspicious activities proactively, enabling rapid response before breaches escalate.
-
Conduct regular audits of AI models for fairness, bias, and privacy compliance. Tools like Qualys support automated privacy-by-design practices and help maintain ethical AI use throughout the data lifecycle.
-
Incorporate multi-layered security controls. Foundational measures such as encryption, multi-factor authentication, and intrusion detection remain essential and are increasingly enhanced by AI’s real-time monitoring and automated response capabilities.
-
Continuously monitor evolving AI-driven privacy threats using threat intelligence platforms and governance frameworks. The cybersecurity landscape is dynamic, with adversaries leveraging AI to bypass traditional defenses, making adaptive monitoring indispensable.
Verification of privacy protections is not a one-time task but an ongoing process. Organizations must cultivate intelligent, self-governing data ecosystems that adapt to emerging threats while preserving privacy and compliance.
Final Thoughts
Implementing AI privacy protections is a complex, multifaceted endeavor involving legal, technical, and human factors. Success requires a holistic approach that includes managing third-party risks, designing user-centric consent models, deploying state-of-the-art leak detection technologies, and maintaining vigilant, continuous monitoring.
There are no silver bullets. Organizations must blend robust technology, clear policies, and adaptive governance to safeguard privacy effectively in an AI-driven world. Acknowledging and proactively addressing these complexities is essential to building sustainable trust and resilience against AI surveillance and data collection risks.
Issue | Challenges | Practical Solutions |
---|---|---|
Navigating Incomplete Data Control and App Incompatibilities |
|
|
Balancing Privacy Protections with Usability and Managing Consent Fatigue |
|
|
Detecting Data Leakage and Monitoring Privacy Protection Effectiveness |
|
|
Advanced Techniques and Future-Proofing Your Privacy in an Evolving AI Landscape
Advanced Techniques and Future-Proofing Your Privacy in an Evolving AI Landscape
How can you protect your privacy as AI surveillance and data collection grow more complex and pervasive? The answer goes beyond traditional safeguards. It increasingly depends on sophisticated cryptographic methods, intelligent data governance, and staying proactive amid evolving regulations. This section unpacks leading-edge technologies and strategic approaches shaping privacy protection in 2025—and how you can start applying them today to remain resilient.
Cutting-Edge Privacy-Enhancing Technologies: Promise and Limitations
Differential Privacy offers a mathematically rigorous way to extract insights from datasets while obscuring individual data points. Imagine sharing a noisy whisper that reveals general trends without exposing your exact words. This technique, now supported by finalized guidelines from the National Institute of Standards and Technology (NIST), is actively used by organizations like the US Census Bureau and companies such as Uber to protect sensitive driver and rider information.
The core strength of differential privacy lies in its formal guarantees: it restricts the number of data queries to prevent re-identification of individuals. However, deploying it effectively requires specialized expertise and careful calibration to strike a balance between data utility and privacy protection. For many government agencies and enterprises, differential privacy remains a frontier technology that demands robust protocols and skilled personnel to implement successfully.
Federated Learning (FL) represents a paradigm shift in AI training by keeping raw data on user devices, sending only model updates to centralized servers. Think of it as collecting homework summaries from students rather than their notebooks. FL shines in privacy-sensitive sectors like healthcare and finance, where centralizing data raises significant concerns.
Nevertheless, FL faces notable technical challenges. It must contend with security threats such as Byzantine attacks that manipulate model updates, handle heterogeneous datasets that may be non-representative, and manage the communication overhead of coordinating multiple devices. Despite these hurdles, ongoing research and industry investments—by companies including Google, IBM, and NVIDIA—are advancing defenses and optimizations. FL is poised to become a cornerstone of privacy-centric AI, particularly as 6G networks enable higher bandwidth and lower latency.
Homomorphic Encryption (HE) takes privacy protection a step further by enabling computations directly on encrypted data without decrypting it. It’s akin to cooking a meal inside a sealed container without ever opening it. This approach protects data during processing—a phase traditionally vulnerable despite encryption at rest or in transit.
HE holds transformative potential for secure, real-time collaboration and analytics across industries. However, it remains computationally intensive and demands advanced cryptographic knowledge to deploy safely. The introduction of noise into ciphertext enhances security but complicates calculations. Practical adoption requires rigorous key management and layered security measures. IBM’s open-source HE libraries and academic advancements signal progress, yet widespread enterprise use is still emerging.
Navigating the Evolving Regulatory Landscape: GDPR, AI Acts, and Beyond
As AI privacy challenges mount, regulatory frameworks are striving to keep pace, though the landscape remains fragmented and dynamic.
In the European Union, the European Data Protection Board (EDPB) is intensifying enforcement through its 2025 Coordinated Enforcement Framework (CEF), focusing especially on the “right to erasure” (the “right to be forgotten”) across all 30 member states. Organizations must be prepared to promptly delete personal data upon request, with minimal tolerance for delay.
The General Data Protection Regulation (GDPR), nearly a decade old, continues to evolve with active enforcement emphasizing transparency, accuracy in privacy disclosures, and organizational accountability. GDPR now intersects closely with newer EU digital laws like the AI Act, which introduces specific obligations around AI transparency, risk management, and ethical deployment. Together, these regulations are shaping privacy strategies for any organization processing personal data with AI.
In the United States, privacy regulation is highly decentralized. Hundreds of AI-related bills have been proposed in 2025 at the state level, focusing on consumer protections, transparency, and liability. Illinois’ requirement for AI risk assessments every 90 days exemplifies this trend. Without a comprehensive federal privacy law, businesses must navigate a mosaic of statutes, from California’s Consumer Privacy Act (CCPA) to newer laws in Tennessee and New Jersey. This patchwork demands adaptable governance frameworks capable of scaling with evolving legal requirements.
Beyond formal laws, agencies like the Federal Trade Commission (FTC) actively scrutinize privacy practices, including biometric data usage and third-party data brokers. The overriding message is clear: privacy can no longer be treated as a mere compliance checkbox but must be a strategic priority demanding continuous legal awareness and operational adjustment.
Cultivating Proactive Privacy Habits: Staying Ahead of AI’s Curve
The rapid evolution of technology and regulation requires individuals and organizations to cultivate ongoing vigilance and proactive habits to build privacy resilience.
-
Stay Informed: Regularly follow updates from reputable privacy organizations, regulatory bodies, and industry experts. For example, during Data Privacy Week 2025, leaders emphasized that data privacy is a “day-zero imperative,” underscoring the need for continuous attention rather than reactive fixes.
-
Engage in Collective Governance: Participate in community-driven data stewardship initiatives or industry consortia. Collaborative governance efforts help shape privacy norms, balance innovation with accountability, and amplify individual voices in standard-setting.
-
Advocate for Ethical AI: Promote transparency, fairness, and user control in AI development. Encourage mechanisms that provide users with clear visibility into how AI systems process their data and empower them with meaningful choices.
-
Adapt Privacy Measures Continuously: As technologies like federated learning mature and homomorphic encryption becomes more practical, integrate these tools into your data workflows where appropriate. Regularly reassess data minimization policies to ensure collection is limited to what is strictly necessary.
-
Prepare for Regulatory Flux: Build flexible, modular privacy governance frameworks capable of accommodating new regulations without costly overhauls. Embrace internationally recognized standards such as ISO/IEC 27701 (soon to be updated) and security models like Zero Trust principles to strengthen organizational defenses.
Looking Ahead: Privacy as a Dynamic, Layered Endeavor
Protecting privacy in an AI-driven world is not a one-time fix but a continuous process of adaptation and vigilance. Emerging technologies such as differential privacy and federated learning offer powerful tools for safeguarding data, but their complexities and limitations require expertise and thoughtful deployment.
Meanwhile, regulators worldwide are sharpening their focus, striving to balance innovation with individual rights. Organizations and individuals must move beyond reactive compliance toward proactive stewardship—embedding privacy and ethical considerations into the core of AI development and data governance.
In this evolving landscape, the most effective defense combines advanced technical safeguards, regulatory awareness, and a strong ethical compass. Privacy in the age of AI is not guaranteed; it must be actively earned and vigilantly maintained through layered, informed, and adaptive strategies.
Technology | Description | Strengths | Limitations | Notable Users / Developers |
---|---|---|---|---|
Differential Privacy | Extracts insights from datasets while obscuring individual data points. | Mathematically rigorous guarantees; restricts data queries to prevent re-identification. | Requires specialized expertise; balancing data utility and privacy is challenging. | US Census Bureau, Uber; supported by NIST guidelines |
Federated Learning (FL) | AI training paradigm keeping raw data on user devices, sending only model updates. | Enhances privacy by decentralizing data; useful in healthcare and finance. | Vulnerable to Byzantine attacks; handles heterogeneous data; communication overhead. | Google, IBM, NVIDIA |
Homomorphic Encryption (HE) | Allows computation on encrypted data without decryption. | Protects data during processing; enables secure collaboration and analytics. | Computationally intensive; requires advanced cryptography knowledge; complex key management. | IBM open-source libraries, academic research |
Region | Regulatory Focus | Key Regulations / Frameworks | Enforcement / Trends | Implications for Organizations |
---|---|---|---|---|
European Union | Data erasure rights, AI transparency, risk management | GDPR, AI Act, EDPB 2025 Coordinated Enforcement Framework (CEF) | Intensified enforcement on “right to be forgotten”; evolving GDPR enforcement | Must ensure prompt data deletion; integrate AI transparency and ethics into privacy strategies |
United States | Consumer protections, transparency, AI risk assessments | State laws (CCPA, Illinois AI risk assessments, Tennessee, New Jersey laws), FTC oversight | Decentralized regulation; hundreds of AI-related bills; active FTC scrutiny | Requires adaptable governance; navigate patchwork of laws; proactive legal awareness needed |
Habit | Description | Purpose / Benefit |
---|---|---|
Stay Informed | Follow updates from privacy organizations, regulators, experts. | Maintain continuous attention; avoid reactive fixes. |
Engage in Collective Governance | Participate in data stewardship initiatives and consortia. | Shape privacy norms; balance innovation with accountability. |
Advocate for Ethical AI | Promote transparency, fairness, user control in AI systems. | Empower users with data processing visibility and choices. |
Adapt Privacy Measures Continuously | Integrate advanced tools like FL and HE; regularly reassess data minimization. | Ensure privacy tools remain effective and data collection is minimal. |
Prepare for Regulatory Flux | Build flexible, modular governance frameworks; adopt standards (ISO/IEC 27701, Zero Trust). | Accommodate new laws efficiently; strengthen organizational defenses. |