Balancing AI Automation and Personalization in Customer Support
- Introduction: Why Automate Customer Support with AI Without Losing Personalization?
- Introduction: Why Automate Customer Support with AI Without Losing Personalization?
- The Business Imperative: Scale Meets the Human Touch
- Debunking the Myth: Automation Does Not Mean Depersonalization
- Strategic Integration: Designing AI to Enhance Personalization
- Why This Matters Now
- Prerequisites: Technical Foundations and Organizational Readiness for AI-Driven Support Automation
- Prerequisites: Technical Foundations and Organizational Readiness for AI-Driven Support Automation
- Technical Foundations: Beyond Simple Chatbots
- Organizational Readiness: The Critical, Often Overlooked Pillar
- Integration and Ethical Considerations: Connecting the Dots Securely and Responsibly
- Bringing It All Together
- Designing Personalized AI Customer Support Systems: Core Components and Architectures
- Designing Personalized AI Customer Support Systems: Core Components and Architectures
- Core AI Components for Personalization and Automation
- Selecting Models and Designing Data Pipelines for Scalable AI Support
- Embedding Personalization Through Customer Profiling and Dynamic Content
- Synthesizing Technology and Humanity for Effective AI Support
- Step-by-Step Implementation Guide: Building and Deploying AI Automation Without Sacrificing Personalization
- Step-by-Step Implementation Guide: Building and Deploying AI Automation Without Sacrificing Personalization
- Data Preparation and Annotation: The Foundation of Personalization
- Model Training, Fine-Tuning, and Multi-Channel Integration: Tailoring AI to Your Brand
- Setting Up Feedback Loops and Human-in-the-Loop Systems: Safeguarding Trust and Continuous Improvement
- Final Thoughts: Balancing Efficiency with Humanity
- Technical Evaluation and Benchmarking: Measuring Effectiveness and Personalization in AI Customer Support
- Technical Evaluation and Benchmarking: Measuring Effectiveness and Personalization in AI Customer Support
- Defining Key Performance Indicators (KPIs) for AI Customer Support
- Comparing Leading AI Customer Support Platforms: Capabilities, Scalability, and Personalization
- Benchmarking Methodologies and A/B Testing Frameworks for Continuous Improvement
- Closing Thoughts: Evidence-Based AI Automation with a Human Touch
- Troubleshooting Common Challenges: Maintaining Balance Between Automation Efficiency and Personalized Experience
- Troubleshooting Common Challenges: Maintaining Balance Between Automation Efficiency and Personalized Experience
- Loss of Context: Why Conversations Go Off the Rails
- Over-Automation: When Efficiency Undermines Experience
- Bias in AI Responses: Ethical and Practical Implications
- Integration Complexities: The Hidden Roadblocks
- Final Thoughts: Transparency and Trust as Cornerstones
- Advanced Techniques and Future Directions: Enhancing Personalization in AI Support with Emerging Technologies
- Advanced Techniques and Future Directions: Enhancing Personalization in AI Support with Emerging Technologies
- Multimodal AI: Beyond Text to Richer, Contextual Understanding
- Real-Time Emotional Intelligence and Predictive Analytics: Proactive, Empathetic Support
- Federated Learning: Personalization Without Compromising Privacy
- Explainable AI: Building Trust Through Transparency
- Navigating the Regulatory Landscape: Preparing for Ethical, Scalable AI
- Recommendations for Building Scalable, Ethical AI Support Systems
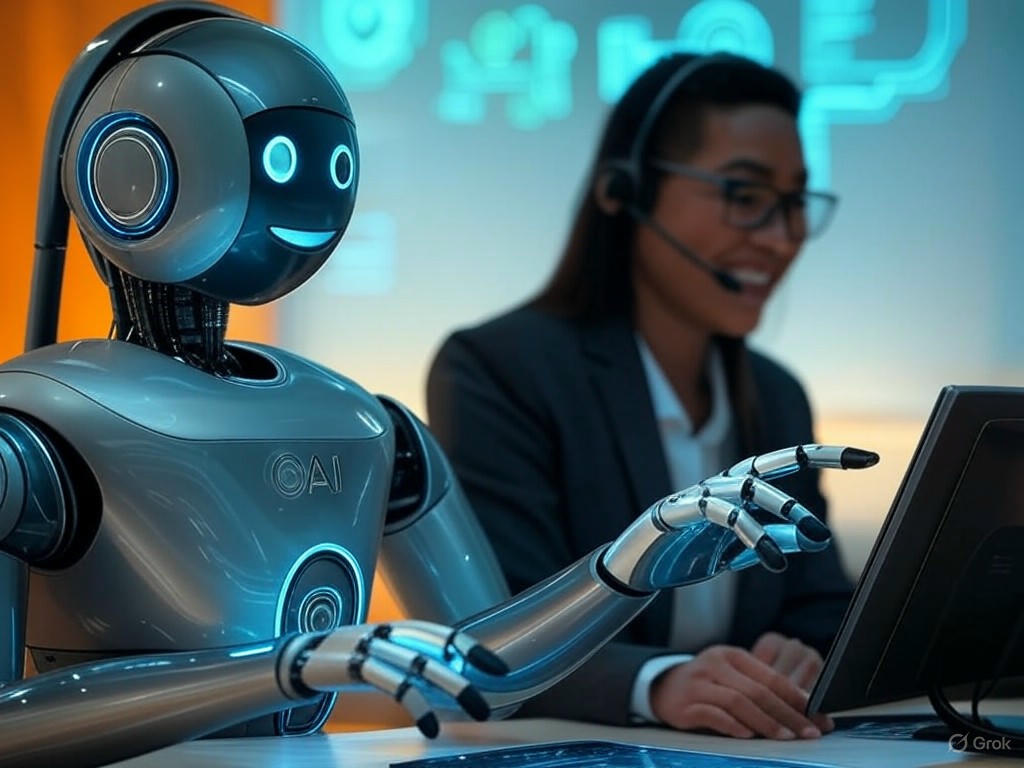
Introduction: Why Automate Customer Support with AI Without Losing Personalization?
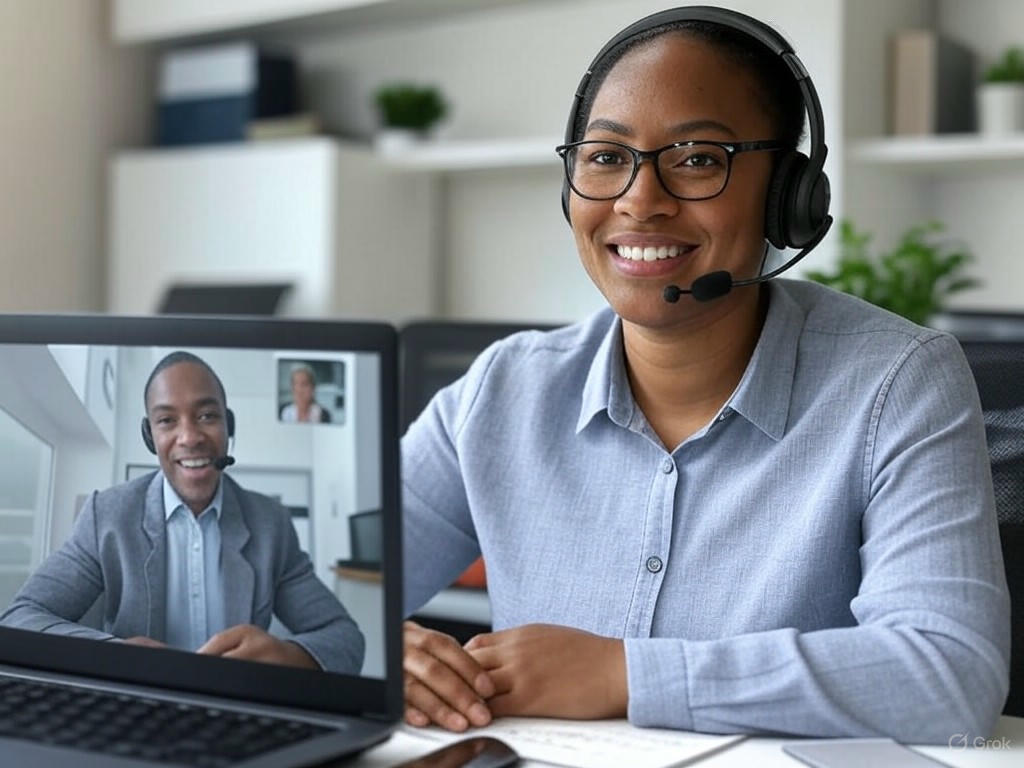
Introduction: Why Automate Customer Support with AI Without Losing Personalization?
Why is automating customer support with AI a pressing priority today—and why does preserving personalization remain non-negotiable? Businesses face a classic tension: the urgent need to scale support efficiently to meet soaring demand while maintaining the human connections that foster loyalty and satisfaction.
The Business Imperative: Scale Meets the Human Touch
The data underscores this dual mandate. By 2025, AI is projected to handle 70-95% of customer interactions, with AI chatbots alone expected to save companies roughly $8 billion annually (NICE, Desk365). Importantly, this evolution is not about replacing human agents but amplifying their impact by automating routine queries and freeing them to focus on complex or sensitive issues.
Operational efficiency has shifted from a “nice-to-have” to a critical business imperative. As Intercom highlights, scaling customer service is essential for growth, and AI tools enable companies to deliver faster, more cost-effective support without proportional headcount increases (Intercom).
However, this quest for efficiency must not come at the expense of personalization. Customer expectations have matured—they demand not only rapid responses but contextually aware, empathetic interactions. AI’s role is to deliver these experiences at scale, enhancing rather than diluting the human touch.
Debunking the Myth: Automation Does Not Mean Depersonalization
A widespread misconception is that automation inherently reduces personalization. Contrary to this, modern AI excels at hyper-personalizing interactions by leveraging extensive customer data to anticipate needs, tailor responses, and dynamically adjust tone (Zendesk). For instance, Zendesk’s Tone Shift tool empowers agents to instantly calibrate message formality or friendliness, seamlessly blending automation with human nuance.
Advances in natural language processing (NLP) and generative AI further enable AI systems to simulate empathetic, human-like conversations once thought impossible (CallMiner). These AI agents can detect sentiment, support multiple languages, and escalate with context-aware handoffs when human intervention is needed.
That said, the technology is not flawless. Real concerns persist around AI bias, misinformation, and data privacy that must be addressed through transparent, ethical AI design and governance (The Future of Commerce). Automation should augment human judgment rather than supplant it.
Strategic Integration: Designing AI to Enhance Personalization
How can architects and support leaders harness AI automation without losing the personal touch? The answer lies in thoughtful, strategic integration that balances broad “one-to-many” automation with deep “all-to-one” personalization.
Key strategies include:
-
Data-Driven Intelligence: Using AI to analyze customer behavior, sentiment, and history to proactively tailor support (Zendesk, Openwave).
-
Hybrid Models: Combining AI for routine queries with smooth transitions to skilled human agents for complex or emotional cases, preserving empathy and trust (Sprout Social).
-
Context-Aware Process Automation: Employing agentic AI platforms that dynamically learn and optimize workflows in real time, reducing inefficiencies while maintaining personalized conversations (CallMiner, Teneo).
-
Ethical AI and Transparency: Implementing explainable AI (XAI) and federated learning techniques to ensure decisions are auditable, fair, and privacy-respecting, thereby fostering customer trust (millermedia7).
-
Continuous Agent Training: Equipping human agents with AI-powered assistive tools that deliver real-time insights, suggested responses, and sentiment analysis to elevate performance (Zendesk).
This article aims to provide a pragmatic, technically grounded guide for AI architects and customer support leaders navigating these complex trade-offs. We will cut through the hype to demonstrate how to design AI-driven automation that scales support operations while deepening, not diluting, personalized customer experiences.
Why This Matters Now
The AI revolution in customer support is no longer theoretical; it is unfolding rapidly. Gartner predicts that by 2026, 20-30% of service agents will be replaced or augmented by generative AI technologies (The Future of Commerce). This transformation will redefine customer loyalty and competitive advantage.
Success will favor companies that view AI as a sophisticated partner—not a blunt instrument for cost-cutting. Those who blend automation with authentic human connection will best meet evolving customer expectations in an increasingly digital world.
In the sections ahead, we will explore specific technical strategies, architectures, and tools that make this balance achievable. The goal is clear: automation at scale without sacrificing the personal touch that turns customers into lifelong advocates.
Strategy | Description | References |
---|---|---|
Data-Driven Intelligence | Using AI to analyze customer behavior, sentiment, and history to proactively tailor support. | Zendesk, Openwave |
Hybrid Models | Combining AI for routine queries with smooth transitions to skilled human agents for complex or emotional cases, preserving empathy and trust. | Sprout Social |
Context-Aware Process Automation | Employing agentic AI platforms that dynamically learn and optimize workflows in real time, reducing inefficiencies while maintaining personalized conversations. | CallMiner, Teneo |
Ethical AI and Transparency | Implementing explainable AI (XAI) and federated learning techniques to ensure decisions are auditable, fair, and privacy-respecting, fostering customer trust. | millermedia7 |
Continuous Agent Training | Equipping human agents with AI-powered assistive tools that deliver real-time insights, suggested responses, and sentiment analysis to elevate performance. | Zendesk |
Prerequisites: Technical Foundations and Organizational Readiness for AI-Driven Support Automation
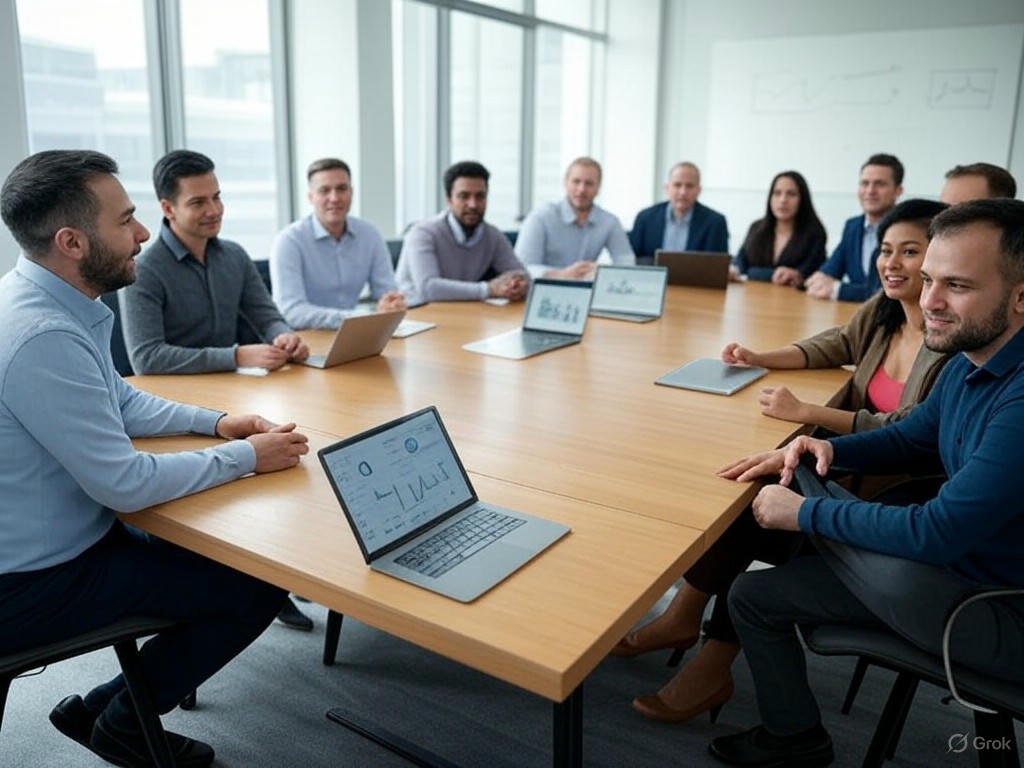
Prerequisites: Technical Foundations and Organizational Readiness for AI-Driven Support Automation
What foundational work must organizations accomplish before embarking on AI-driven customer support automation? This is a multifaceted challenge. Achieving automation that enhances personalization demands a solid blend of advanced technologies, high-quality data, well-mapped workflows, and an organizational culture primed to adopt AI as a collaborative partner—not merely a tool.
Technical Foundations: Beyond Simple Chatbots
Natural Language Processing (NLP) sits at the core of AI-powered customer support, enabling machines to interpret, understand, and generate human language. Modern NLP is far beyond basic keyword matching; it leverages sophisticated deep learning transformer models like GPT-4 and its successors. These models deliver nuanced language understanding, powering chatbots and virtual assistants capable of engaging in natural, context-aware conversations.
To appreciate the significance, the global NLP market is projected to reach nearly $157 billion by 2030, reflecting its pivotal role in business transformation. Advanced NLP integrates text, speech, and image processing to holistically grasp customer queries, including multilingual capabilities that break down language barriers. However, handling rare languages or domain-specific jargon remains a constant challenge, requiring ongoing model refinement and data enrichment.
Machine learning models require orchestration to function cohesively. AI orchestration platforms such as Haystack, Vue.ai, and Akka have emerged as essential tools, managing complex workflows, coordinating multiple AI agents, and integrating seamlessly with existing IT infrastructures. These platforms act like conductors, ensuring each AI component—from intent recognition to response generation and ticket routing—performs harmoniously.
Underlying this is robust data infrastructure, the silent powerhouse of AI systems. High-quality, well-governed data streams are indispensable for training models, tuning responses, and enabling real-time analytics. Scalable cloud resources paired with automated pipelines for continuous model retraining and deployment—practices encapsulated in MLOps frameworks—prevent AI systems from becoming brittle or degrading in performance over time.
Organizational Readiness: The Critical, Often Overlooked Pillar
Technical capabilities alone do not guarantee success. According to McKinsey, while 92% of companies plan to increase AI investments, only about 1% consider their AI deployment mature. A major barrier is organizational readiness—do you have the right data, workflows, and personnel prepared to integrate AI effectively?
-
Data Availability and Quality: Comprehensive, consistent, and clean customer support data is foundational. Tools for data profiling, cleansing, validation, and enrichment can reduce manual cleaning efforts by up to 40% and improve decision-making speed by roughly 30%. Poor-quality or biased data leads to subpar AI performance and threatens customer trust.
-
Support Workflow Mapping: Critically analyze current support processes. Understand ticket flows, identify bottlenecks, and evaluate the balance between automation and human intervention. AI should augment human agents by handling repetitive queries while escalating complex or sensitive issues to humans who provide empathy and nuanced judgment.
-
Team Readiness and Culture: Employees are often more open to AI adoption than leadership assumes. Implementing training and change management programs focused on upskilling agents to collaborate with AI tools fosters acceptance and reduces resistance. Gartner recommends embedding AI literacy across departments to transition from pilots to full-scale deployments.
Integration and Ethical Considerations: Connecting the Dots Securely and Responsibly
Effective AI-driven customer support automation does not operate in isolation. Seamless integration with existing systems—Customer Relationship Management (CRM) platforms like Salesforce Einstein and HubSpot, ticketing systems, and knowledge bases—is vital to enable context-rich, continuous interactions.
For example, AI-powered CRMs use historical customer data to personalize responses and predict needs, while AI chatbots alleviate agent workload by handling routine inquiries 24/7. Integration ensures that AI complements human agents, providing a unified, personalized experience.
Security and privacy are the ethical backbones of AI deployment. With AI processing sensitive personal and behavioral data, organizations must embed privacy-by-design principles. This entails encrypting and anonymizing data, conducting regular audits to detect bias and ensure fairness, and complying with regulations such as the European Union’s GDPR.
Moreover, establishing formal AI policies is essential. Effective policies define acceptable AI uses, data governance frameworks, bias mitigation strategies, and accountability structures. These often involve senior oversight roles like Chief AI Officers (CAIOs) and Chief Information Security Officers (CISOs). Continuous monitoring and auditing ensure AI systems remain transparent, fair, and aligned with organizational values, fostering customer trust.
Bringing It All Together
Automating customer support with AI without sacrificing the human touch requires more than flipping a switch. It demands:
-
Mastery of NLP and machine learning models powered by robust AI orchestration platforms and scalable data infrastructure.
-
High-quality, governed data coupled with a thorough understanding of existing customer support workflows.
-
A culture of AI readiness supported by comprehensive training, change management, and leadership alignment.
-
Seamless integrations with CRM systems, ticketing platforms, and knowledge bases to deliver context-aware, personalized service.
-
Rigorous security, privacy, and ethical frameworks to build and maintain customer trust.
Only when these prerequisites are firmly established can organizations fully harness AI’s potential to deliver faster, smarter, and deeply personalized customer experiences at scale. Skipping these foundational steps risks not only technical failure but also erosion of the very customer relationships AI aims to strengthen.
Category | Key Components | Details | Examples/Tools |
---|---|---|---|
Technical Foundations | Natural Language Processing (NLP) | Advanced deep learning transformer models enabling nuanced language understanding, context-aware conversations, multilingual support | GPT-4, successors |
AI Orchestration Platforms | Manage complex AI workflows, coordinate multiple AI agents, integrate with IT infrastructure | Haystack, Vue.ai, Akka | |
Data Infrastructure | High-quality, well-governed data streams for training and tuning; scalable cloud resources; automated pipelines; MLOps frameworks | Cloud platforms, MLOps tools | |
Market Significance | Growth and importance of NLP in business transformation | Projected $157 billion NLP market by 2030 | |
Organizational Readiness | Data Availability and Quality | Consistent, clean customer support data; tools for profiling, cleansing, validation, enrichment; reduces manual effort and improves decision speed | Data profiling and cleansing tools |
Support Workflow Mapping | Analyze ticket flows, identify bottlenecks, balance automation and human intervention | Workflow analysis frameworks | |
Team Readiness and Culture | Training and change management to upskill agents, foster acceptance, embed AI literacy | Training programs, Gartner recommendations | |
Integration and Ethical Considerations | System Integration | Seamless connection with CRM, ticketing, knowledge bases for context-rich interactions | Salesforce Einstein, HubSpot |
Security and Privacy | Privacy-by-design: data encryption, anonymization, audits, GDPR compliance | Encryption tools, compliance frameworks | |
AI Policies and Governance | Define AI use cases, data governance, bias mitigation, accountability; senior oversight roles; continuous monitoring | Chief AI Officer (CAIO), Chief Information Security Officer (CISO) |
Designing Personalized AI Customer Support Systems: Core Components and Architectures
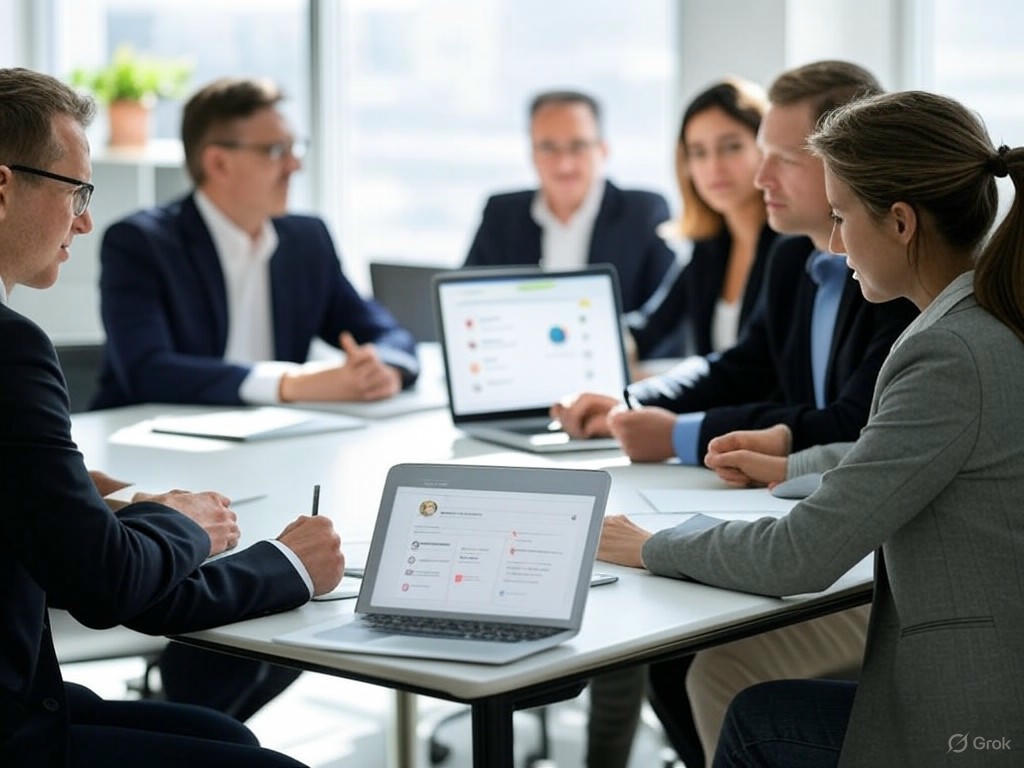
Designing Personalized AI Customer Support Systems: Core Components and Architectures
Building an AI customer support system that delivers a genuinely personalized experience—one that avoids feeling cold or robotic—requires a careful blend of advanced technical design and human-centered principles. The core challenge is to ensure the AI not only automates routine tasks but also understands and adapts to each customer’s unique needs in real time.
Core AI Components for Personalization and Automation
At the foundation of any AI-driven customer support platform is a suite of intelligent components working together to interpret customer inputs, maintain context, and respond with appropriate nuance.
-
Intent Recognition: Accurately identifying what a customer wants is critical. Modern systems use transformer-based natural language understanding (NLU) models, such as GPT-4 and similar large language models (LLMs), trained on vast conversational datasets. These models decode user intents even when queries are fragmented, misspelled, or complex. For example, financial institutions like banks and credit unions implement pre-trained NLU models to automate routine requests while continuously monitoring missed intents to refine their systems—a feedback loop essential to prevent customer frustration caused by irrelevant or incomplete responses.
-
Sentiment Analysis: Understanding the emotional tone of customer messages has become indispensable. Advanced AI sentiment analysis tools provide real-time insights into customer mood and satisfaction, enabling the system to adjust its tone and urgency dynamically. Hospitality companies like Hilton deploy AI-powered sentiment analysis across thousands of properties to transform raw feedback into actionable insights, enhancing empathy in automated interactions.
-
Contextual Memory: Unlike traditional chatbots that reset after each interaction, next-generation AI agents employ layered memory architectures that retain conversation history, user preferences, and brand persona. This approach supports coherent, context-aware dialogues over multiple interactions. Effective memory management involves selective forgetting and continuous updating to avoid overwhelming the system or sounding disconnected—striking this balance is crucial to maintaining a natural, human-like conversation flow.
-
Interaction Orchestration: Efficient support demands managing multi-turn conversations, appropriate escalation, and seamless AI-to-human transitions. Interaction orchestration frameworks route requests based on intent complexity, sentiment signals, and customer profiles, ensuring AI handles high-volume, low-complexity queries while human agents focus on nuanced cases.
-
Human-Agent Handoff: Recognizing the limits of AI is vital. Smooth, context-rich handoffs preserve conversation continuity and customer trust. Leading platforms now support bi-directional handoffs, where AI can escalate to humans and later resume interactions post-human intervention, optimizing overall service quality.
Selecting Models and Designing Data Pipelines for Scalable AI Support
The choice of AI models and the design of data pipelines are pivotal to building scalable, adaptable, and responsive customer support systems.
-
Transformer-Based NLP Models: The advent of transformer architectures—epitomized by models like GPT-4—has revolutionized natural language processing. These models excel at contextual understanding, coherent response generation, and multilingual support, which are essential for diverse customer bases. Their extensive pre-training on massive datasets enables handling a broad spectrum of queries with high accuracy.
-
Fine-Tuning and Transfer Learning: While pre-trained models offer strong general capabilities, fine-tuning on domain-specific datasets—such as company FAQs, product details, and historical support tickets—is essential to align AI responses with brand voice and expertise. Parameter-efficient techniques like LoRA and QLoRA facilitate this fine-tuning with reduced computational demands, making customization feasible even for resource-constrained teams.
-
Data Pipeline Architecture: Real-time AI inference requires robust data pipelines that ingest, clean, and update data from multiple channels—web, mobile apps, social media, and CRM systems—while ensuring high data quality, privacy, and compliance. Modern AI stacks integrate structured and unstructured data with sub-second latency in response generation. Continuous monitoring frameworks detect model drift promptly, preventing degradation of response accuracy and maintaining customer satisfaction.
-
Retrieval-Augmented Generation (RAG): To enhance factual accuracy and mitigate hallucinations, many AI support systems employ RAG frameworks that combine generative models with retrieval mechanisms. By dynamically fetching relevant documents or past interactions, RAG ensures AI responses are grounded in up-to-date, authoritative information.
Embedding Personalization Through Customer Profiling and Dynamic Content
Scaling automation without sacrificing the personal touch hinges on embedding rich personalization capabilities throughout the AI support system.
-
Unified Customer Profiles: AI systems leverage comprehensive, unified profiles that consolidate behavioral data, purchase history, preferences, and prior interactions. Platforms like Segment and Gainsight exemplify tools that synthesize multi-channel data into actionable profiles, enabling AI to generate tailored responses and proactive recommendations.
-
Dynamic Content Generation: Moving beyond static, scripted replies, generative AI enables real-time creation of personalized messages, offers, and troubleshooting steps. This dynamic content generation adapts to the customer’s current context and historical interactions, creating conversations that feel natural and uniquely relevant.
-
Predictive Analytics and Proactive Engagement: Advanced AI anticipates customer needs by analyzing behavioral patterns and sentiment trends. Proactive outreach—such as flagging potential issues before the customer contacts support—enhances satisfaction and reduces friction. These capabilities transform reactive service into anticipatory engagement.
-
Human-in-the-Loop (HITL) and Escalation Strategies: Despite AI advances, human empathy and judgment remain irreplaceable for complex or sensitive cases. Intelligent handoff mechanisms ensure seamless transitions to human agents when required, with AI supplying contextual information and suggested responses to aid human decision-making. This collaboration enhances operational efficiency without compromising personalization.
Synthesizing Technology and Humanity for Effective AI Support
The architecture of personalized AI customer support demands a thoughtful integration of cutting-edge technology with human sensibility. Transformer-based NLP models, layered contextual memory systems, and sentiment-aware intent recognition form the technical backbone. Yet, without embedding rich customer profiles, dynamic content generation, and fluid human-agent collaboration, automation risks alienating customers rather than engaging them.
Business leaders and AI architects must invest not only in powerful models but also in data governance, continuous performance evaluation, and user-centric design. The objective is not to replace human agents but to empower them—scaling personalized support in ways previously unimaginable. When executed effectively, AI-powered support systems do more than answer questions—they forge lasting customer relationships.
Core Component | Description | Example Use Case |
---|---|---|
Intent Recognition | Uses transformer-based NLU models (e.g., GPT-4) to accurately identify customer intents, even with fragmented or complex queries. | Banks use pre-trained NLU models to automate routine requests and refine systems via feedback loops. |
Sentiment Analysis | Analyzes emotional tone in real-time to adjust AI responses with appropriate tone and urgency. | Hilton uses sentiment analysis to transform feedback into empathetic automated interactions. |
Contextual Memory | Retains conversation history, user preferences, and brand persona for coherent multi-turn dialogues with selective forgetting. | Next-gen AI agents maintain context across sessions to enable natural conversations. |
Interaction Orchestration | Manages multi-turn conversations, escalation, and AI-to-human transitions based on intent, sentiment, and profiles. | Routes simple queries to AI and complex ones to human agents efficiently. |
Human-Agent Handoff | Ensures smooth, context-rich transitions between AI and human agents with bi-directional handoffs. | AI escalates difficult cases to humans and resumes interaction after intervention. |
Model or Technique | Description | Benefits |
---|---|---|
Transformer-Based NLP Models | Pre-trained on massive datasets to handle diverse queries with contextual understanding and multilingual support. | High accuracy and broad query handling capabilities. |
Fine-Tuning and Transfer Learning (LoRA, QLoRA) | Customizes pre-trained models on domain-specific data efficiently with reduced compute needs. | Aligns AI responses with brand voice and expertise. |
Data Pipeline Architecture | Ingests, cleans, and updates multi-channel data with sub-second latency ensuring quality and compliance. | Enables real-time AI inference and prevents model drift. |
Retrieval-Augmented Generation (RAG) | Combines generative models with retrieval of relevant documents and past interactions. | Enhances factual accuracy and reduces hallucinations. |
Personalization Feature | Description | Example Tools or Approaches |
---|---|---|
Unified Customer Profiles | Consolidates behavioral data, purchase history, preferences, and prior interactions into actionable profiles. | Segment, Gainsight |
Dynamic Content Generation | Generates real-time personalized messages and offers adapting to customer context and history. | Generative AI models for personalized conversations |
Predictive Analytics and Proactive Engagement | Analyzes patterns and sentiment to anticipate needs and enable proactive outreach. | Flagging potential issues before customer contacts support |
Human-in-the-Loop (HITL) and Escalation | Seamless AI-human transitions with AI providing contextual info and suggested responses. | Intelligent handoff mechanisms |
Step-by-Step Implementation Guide: Building and Deploying AI Automation Without Sacrificing Personalization
Step-by-Step Implementation Guide: Building and Deploying AI Automation Without Sacrificing Personalization
How do you automate customer support with AI without reducing your brand’s voice to a soulless script? The key is a carefully staged, balanced approach that combines technical precision with ethical oversight, ensuring AI enhances rather than diminishes the personal connection customers expect. Below is a detailed, phased workflow aligned with best practices and emerging trends for 2025 and beyond.
Data Preparation and Annotation: The Foundation of Personalization
AI’s ability to deliver truly personalized support hinges on training data that is meticulously prepared and accurately annotated. Approximately half of businesses leveraging large datasets report that without robust annotation strategies, even advanced AI models struggle to grasp the nuances of customer interactions.
Essential steps and best practices include:
-
Curate Brand-Specific Datasets: While public datasets serve as useful baselines, personalization demands datasets tailored to your brand’s unique customer language, products, and service scenarios. Custom annotation captures subtle domain-specific cues, enabling AI responses to feel authentic and aligned with brand tone.
-
Implement Multi-Modal Annotation: Customer support data spans multiple formats—text, voice recordings, images, and sometimes video. Utilize annotation tools capable of handling these modalities, tagging not only intent and entities but also sentiment and contextual signals. This rich, layered input is critical for building models with deep contextual awareness.
-
Leverage AI-Assisted Annotation Platforms: Solutions like SuperAnnotate and Shaip offer AI-assisted labeling that accelerates the annotation process without compromising quality. This hybrid approach reduces manual effort while maintaining data integrity and consistency.
-
Develop Clear Annotation Guidelines: Consistency is paramount. Establish detailed protocols addressing edge cases, ambiguous expressions, and complex customer language to minimize model confusion and bias.
-
Enforce Data Privacy and Security: Adhere strictly to privacy laws by anonymizing personally identifiable information (PII) wherever possible. While external vendors can aid in compliance, internal processes must enforce rigorous data handling controls to protect customer trust.
By executing these steps, your AI models will gain a nuanced understanding of customer needs, laying a solid foundation for personalized, context-aware support.
Model Training, Fine-Tuning, and Multi-Channel Integration: Tailoring AI to Your Brand
Pre-trained, general-purpose AI models are powerful but risk delivering generic, off-brand responses without fine-tuning. Customizing these foundation models with your specific data sharpens relevance and enhances personalization.
Key considerations for this phase:
-
Select Efficient Fine-Tuning Techniques: Parameter-efficient methods like LoRA and QLoRA have become standard in 2025, enabling adaptation of large models using limited computational resources. These approaches maintain cost-effectiveness and speed while avoiding overfitting.
-
Perform Supervised Fine-Tuning: Incorporate your annotated, domain-specific datasets into supervised fine-tuning pipelines. This process refines the model’s grasp of your customer interactions, improving accuracy in resolving unique queries and reflecting your brand’s tone.
-
Adopt Hybrid Infrastructure: For sensitive or regulated data, conduct fine-tuning on-premises using dedicated GPUs to ensure compliance. For scalability and agility, leverage cloud platforms such as AWS SageMaker or Azure AI Foundry. A hybrid architecture balances security with operational flexibility.
-
Integrate Across Customer Support Channels: Deploy AI-powered automation seamlessly across chatbots, voice assistants, email workflows, and social media platforms. Tools like LiveX AI and Talkative facilitate multi-channel integration, ensuring consistent yet channel-appropriate personalization.
-
Embed Contextual Memory: Implement conversational memory and context-awareness to sustain natural, human-like dialogues across multiple interactions. This reduces repetitive or robotic exchanges, enhancing customer engagement.
-
Automate Routine Tasks While Empowering Human Agents: Use AI to address FAQs, route tickets intelligently, and handle simple requests. This frees human agents to focus on complex, empathy-driven issues, preserving the human touch where it matters most.
This phase transforms AI from a generic assistant into a brand-savvy support agent capable of nuanced, personalized engagement.
Setting Up Feedback Loops and Human-in-the-Loop Systems: Safeguarding Trust and Continuous Improvement
AI in customer support is not “set and forget.” Maintaining personalization, trust, and empathy requires robust feedback mechanisms and human oversight.
Core components to implement:
-
Establish Real-Time Feedback Loops: Continuously capture and analyze customer interactions to identify pain points and successes. Platforms like DevRev convert every conversation into actionable insights, enabling prompt refinement of AI responses.
-
Deploy Human-in-the-Loop (HITL) Frameworks: Integrate human review strategically for complex, sensitive, or high-risk cases. HITL ensures AI augments human judgment, reducing errors, mitigating ethical risks, and maintaining empathetic service.
-
Design Clear Fallback and Escalation Strategies: Define escalation protocols where AI defers to human agents upon detecting uncertainty, customer dissatisfaction, or emotional complexity. This prevents frustration and preserves trust by ensuring human empathy when needed.
-
Ensure Transparency and Obtain Consent: Inform customers upfront about AI usage and data handling practices. Provide options to opt-out or request human interaction, fostering transparency and compliance with regulations such as GDPR and CCPA.
-
Implement Continuous Monitoring and Ethical Auditing: Regularly audit AI behavior for bias, fairness, and performance drift using monitoring dashboards and alerting systems. Early detection of issues prevents adverse customer experiences.
-
Close the Feedback Loop with Customers: Communicate improvements or changes driven by customer input, reinforcing a collaborative relationship and responsiveness.
Embedding these safeguards ensures AI remains a trustworthy, respectful partner in your support ecosystem.
Final Thoughts: Balancing Efficiency with Humanity
The promise of AI in customer support is not automation at the expense of personalization, but automation that elevates the human experience. By handling routine tasks at scale, AI empowers human agents to focus on what machines cannot replicate—genuine empathy, complex problem-solving, and nuanced judgment.
Through meticulous data preparation, targeted model fine-tuning, seamless multi-channel integration, and robust human-in-the-loop frameworks, businesses can unlock AI’s full potential without sacrificing the personal touch customers demand.
In 2025 and beyond, success hinges on viewing AI as a collaborative partner—an augmentation of human capability rather than a replacement—crafting customer journeys that are both efficient and deeply human.
Phase | Key Steps / Best Practices | Tools / Techniques | Goals / Outcomes |
---|---|---|---|
Data Preparation and Annotation |
|
|
|
Model Training, Fine-Tuning, and Multi-Channel Integration |
|
|
|
Setting Up Feedback Loops and Human-in-the-Loop Systems |
|
|
|
Technical Evaluation and Benchmarking: Measuring Effectiveness and Personalization in AI Customer Support
Technical Evaluation and Benchmarking: Measuring Effectiveness and Personalization in AI Customer Support
How can you confidently assess whether your AI-driven customer support is achieving its goals without sacrificing the human connection? The answer lies in a comprehensive, data-driven evaluation framework that balances operational efficiency with genuine personalization. This section breaks down the essential KPIs, platform capabilities, and benchmarking methodologies critical to successful AI customer support automation in 2025.
Defining Key Performance Indicators (KPIs) for AI Customer Support
Focusing solely on response speed or cost reduction is an incomplete view of AI success. To truly measure both effectiveness and personalization, a multidimensional set of KPIs is necessary. Here are the key indicators that should be closely monitored:
-
Response Time and Latency: Speed remains paramount. Measuring the time between a customer query and the AI’s response directly impacts customer satisfaction. Fast, sub-3-second response times not only reduce frustration but enable real-time support in high-volume channels such as call centers or chatbots (Multimodal.dev).
-
Resolution Rates: The percentage of issues resolved without human escalation reflects the AI system’s problem-solving autonomy. For instance, Algomo reports up to 85% of queries resolved automatically, highlighting AI’s potential to handle routine interactions effectively (Unite.ai).
-
Customer Satisfaction (CSAT): The definitive metric for customer experience. Leading AI tools now enhance CSAT assessment by analyzing not only survey scores but also conversation transcripts and agent-specific performance, capturing nuanced sentiment trends for a richer understanding (Crescendo.ai).
-
Personalization Effectiveness:
- Relevance of Recommendations: AI should tailor responses and offers based on user data and behavioral patterns, driving higher engagement and conversion rates (B2Brocket.ai).
- Sentiment Alignment: Advanced natural language processing (NLP) models detect customer mood and adjust tone accordingly, adding an empathetic dimension to automated conversations (CallMiner).
-
Knowledge Base Accuracy and Update Frequency: The AI’s knowledge repository needs to be current and precise. Outdated or inconsistent data can lead to misinformation and unnecessary escalations, impacting customer trust (Verloop.io).
-
Compliance and Transparency Metrics: With 63% of consumers concerned about AI bias and discrimination, monitoring adherence to data privacy regulations such as GDPR, and ensuring explainability of AI decisions, is essential for building and maintaining customer trust (Sobot.io).
Tracking these KPIs collectively offers a holistic view of how AI systems perform both operationally and emotionally, ensuring balance between efficiency and human-centric support.
Comparing Leading AI Customer Support Platforms: Capabilities, Scalability, and Personalization
Selecting the right AI platform requires aligning your business objectives with a solution’s strengths in automation and personalization. Here’s an overview of prominent platforms shaping the AI customer support landscape in 2025:
-
Zendesk: Integrates decades of customer service data with generative AI to assist agents by summarizing tickets and suggesting replies. Its omnichannel reach and AI-assisted workflows support scalability without losing conversational context (Zendesk.com).
-
Algomo: Excels in multilingual support and robust automation, handling up to 85% of queries without human intervention. This is especially valuable for global enterprises aiming to maintain localized personalization at scale (Unite.ai).
-
Freshworks’ Freddy AI: Embedded within their chat and enterprise plans, Freddy automates routine tasks and enhances agent responses with context-aware AI, exemplifying how AI can augment rather than replace human agents (Zendesk.com).
-
Moveworks: An agentic AI platform that orchestrates multiple AI tools to empower workforce productivity by delivering frictionless support experiences and dynamic workflow automation (Moveworks.com).
-
Zowie and Forethought: These platforms go beyond reactive automation by proactively taking actions across systems—such as updating customer relationship management (CRM) records or predicting customer intent—to deliver timely, personalized support (GetZowie.com).
When evaluating scalability, consider how well these platforms integrate with existing systems, their ability to handle peak loads, and adaptability to emerging channels like voice, chat, or video. Personalization capabilities hinge on real-time data integration, sentiment analysis, and predictive analytics that enable contextually relevant interactions.
Benchmarking Methodologies and A/B Testing Frameworks for Continuous Improvement
Ensuring that AI-driven support evolves with customer expectations requires ongoing benchmarking and experimentation:
-
Baseline Benchmarking: Establish initial measurements for response time, CSAT, and resolution rates. Set targets informed by industry standards—for example, aiming for response times under 3 seconds and first-contact resolution rates above 70%.
-
Iterative Query Refinement: Leverage generative AI to refine query handling through follow-up questions and context expansion, improving accuracy and relevance over time (PrajnaAI.medium.com).
-
A/B Testing Personalization Elements: Utilize tools like AB Tasty, Dynamic Yield, and Split.io to run controlled experiments on conversational tone, recommendation algorithms, or escalation triggers. This data-driven approach reveals what resonates best with distinct customer segments (VWO.com).
-
Hybrid Experimentation: Combine traditional experimentation with AI-driven personalization to gain granular insights—such as measuring the impact of AI tone adjustments on CSAT or resolution speed (SiteSpect.com).
-
Human-in-the-Loop Feedback: Continuously integrate feedback from human agents and customers to prevent AI drift into impersonal or inaccurate behavior. Case studies demonstrate that scaling AI successfully requires empowering human agents rather than replacing them (HiverHQ.com).
-
Data Quality and Governance: Robust benchmarking depends on clean, consistent data. Poor data governance leads to siloed, error-prone datasets that compromise AI model accuracy and personalization outcomes (Sobot.io).
Closing Thoughts: Evidence-Based AI Automation with a Human Touch
Automating customer support without losing personalization is a delicate balance achieved through strategic KPI selection, careful platform choice, and continuous experimentation. These technical evaluation and benchmarking practices act as a compass to steer AI initiatives toward measurable success.
Remember, AI’s true power lies in augmenting human agents by swiftly handling routine tasks while delivering personalized, empathetic interactions grounded in data-driven insights. Without transparent performance metrics, thoughtful vendor selection, and iterative testing, AI risks becoming a blunt instrument rather than a precision tool.
The future belongs to those who treat AI not as a mysterious black box but as a rigorously monitored, continuously refined partner in crafting exceptional customer experiences.
KPI | Description | Impact / Measure | Example / Source |
---|---|---|---|
Response Time and Latency | Time between customer query and AI response | Sub-3-second response times improve satisfaction and enable real-time support | Multimodal.dev |
Resolution Rates | Percentage of issues resolved without human escalation | Up to 85% queries resolved automatically indicates strong autonomy | Unite.ai |
Customer Satisfaction (CSAT) | Measures overall customer experience | Includes survey scores and transcript sentiment analysis for nuanced insights | Crescendo.ai |
Personalization Effectiveness: Relevance of Recommendations | Tailoring responses and offers based on user data and behavior | Drives higher engagement and conversion rates | B2Brocket.ai |
Personalization Effectiveness: Sentiment Alignment | NLP detects customer mood and adjusts tone accordingly | Adds empathetic dimension to automated conversations | CallMiner |
Knowledge Base Accuracy and Update Frequency | Ensures AI repository is current and precise | Prevents misinformation and unnecessary escalations | Verloop.io |
Compliance and Transparency Metrics | Monitors adherence to data privacy and explainability | Builds customer trust by addressing AI bias concerns | Sobot.io |
Platform | Key Capabilities | Scalability Features | Personalization Features | Source |
---|---|---|---|---|
Zendesk | Generative AI assists agents by summarizing tickets and suggesting replies | Omnichannel reach and AI-assisted workflows support scalability without losing context | Context-aware AI supports personalized conversational continuity | Zendesk.com |
Algomo | Multilingual support; robust automation handling up to 85% queries automatically | Suitable for global enterprises with localized personalization | Localized personalization at scale | Unite.ai |
Freshworks’ Freddy AI | Automates routine tasks, enhances agent responses with context-aware AI | Embedded in chat and enterprise plans for easy scaling | Augments human agents with personalized, context-aware assistance | Zendesk.com |
Moveworks | Orchestrates multiple AI tools for frictionless support and workflow automation | Dynamic workflow automation boosts workforce productivity | Delivers seamless, personalized support experiences | Moveworks.com |
Zowie and Forethought | Proactively updates CRM and predicts customer intent | Supports proactive actions across systems for timely support | Enables predictive, personalized customer interactions | GetZowie.com |
Methodology | Description | Purpose / Outcome | Example / Source |
---|---|---|---|
Baseline Benchmarking | Establish initial KPIs like response time, CSAT, resolution rates | Set targets aligned with industry standards (e.g., <3s response, >70% resolution) | Industry standard benchmarking |
Iterative Query Refinement | Use generative AI for follow-up questions and context expansion | Improves query accuracy and relevance over time | PrajnaAI.medium.com |
A/B Testing Personalization Elements | Run controlled experiments on tone, recommendations, escalation triggers | Identify best approaches for different customer segments | VWO.com |
Hybrid Experimentation | Combine traditional testing with AI-driven personalization | Gain granular insights on AI adjustments’ impact on CSAT and speed | SiteSpect.com |
Human-in-the-Loop Feedback | Integrate agent and customer feedback continuously | Prevent AI drift and maintain empathetic, accurate AI behavior | HiverHQ.com |
Data Quality and Governance | Ensure clean, consistent datasets for AI training and benchmarking | Avoid siloed or error-prone data to improve accuracy and personalization | Sobot.io |
Troubleshooting Common Challenges: Maintaining Balance Between Automation Efficiency and Personalized Experience
Troubleshooting Common Challenges: Maintaining Balance Between Automation Efficiency and Personalized Experience
Deploying AI to automate customer support is a delicate balancing act between operational efficiency and preserving the genuine, personalized experience that customers expect. This tightrope walk is fraught with common pitfalls that can undermine trust and satisfaction if not carefully managed. In this section, we explore frequent challenges organizations face and actionable strategies to troubleshoot them, ensuring AI amplifies — rather than diminishes — the human touch.
Loss of Context: Why Conversations Go Off the Rails
One of the most significant issues in AI-driven support is the loss of conversational context, which leads to interactions feeling robotic or fragmented. For example, customers often need to repeat their issues because the AI fails to remember or connect previous details, resulting in frustration and eroding trust.
Typical root causes include:
- Insufficient intent recognition: AI models struggle to accurately interpret nuanced customer requests.
- Fragmented data silos: Disconnected systems prevent AI from accessing comprehensive interaction histories.
- Limited chatbot memory: Many bots reset context after each interaction or lack session state tracking.
Effective troubleshooting strategies include:
-
Refining intent models through prompt engineering: Craft precise, context-rich prompts that help AI understand subtle customer intents. Research from OpenPrompt Labs shows that assigning roles or personas to AI agents can boost user satisfaction by up to 29%.
-
Enhancing training datasets with real-world dialogues: Incorporate diverse, annotated conversations covering complex and edge-case scenarios to improve the AI’s contextual awareness.
-
Implementing session persistence and cross-channel integration: Enable AI systems to access and update unified customer profiles in real-time, ensuring prior context informs every new query.
By addressing context loss directly, brands can make AI-powered interactions more coherent and empathetic, reducing the risk of alienating customers.
Over-Automation: When Efficiency Undermines Experience
While AI automation promises rapid responses and 24/7 availability—with projections indicating up to a 50% reduction in resolution times—the risk of over-automation looms large. “Chatbot fatigue” occurs when customers feel trapped in repetitive, scripted loops lacking meaningful resolution or empathy.
A cautionary example is Air Canada’s chatbot, which inaccurately promised bereavement refunds contrary to policy, leading to its removal and a loss of customer trust.
To mitigate over-automation pitfalls:
-
Establish clear escalation protocols: Define precise thresholds for AI to transfer interactions to human agents, especially for emotionally charged or complex issues. Solutions like Rapid Innovation’s Inquiry Routing Agent effectively prioritize urgent cases.
-
Monitor AI confidence scores continuously: Allow AI to respond autonomously only when confidence is high; otherwise, route queries to human agents to prevent errors.
-
Implement robust feedback loops: Regularly collect customer satisfaction metrics and qualitative feedback to detect friction caused by automation and iteratively refine AI behavior.
The objective is to augment human support, not replace it, ensuring seamless handoffs that preserve both efficiency and empathy.
Bias in AI Responses: Ethical and Practical Implications
Bias in AI manifests subtly but carries profound consequences. AI models trained on skewed data risk perpetuating harmful stereotypes or unfair treatment. For instance, Amazon’s AI recruiting tool downgraded resumes containing the word “women’s,” while Twitter’s image-cropping algorithm favored white faces, reflecting broader societal biases.
Unchecked AI bias can:
- Erode trust among marginalized groups.
- Cause unfair prioritization or misclassification in support workflows.
- Expose companies to reputational and legal risks.
Mitigation approaches include:
-
Curating diverse and representative training data: Ensure datasets encompass varied demographics, languages, and cultural contexts, following data-centric AI development principles.
-
Human-in-the-loop evaluation: Conduct regular audits with diverse teams to detect and correct biased outputs.
-
Leveraging bias detection tools: Employ platforms like Amazon SageMaker Clarify and Microsoft Fairlearn to assess and mitigate bias systematically.
Ethical AI is far more than a compliance checkbox—it’s a strategic differentiator. PwC reports that 85% of customers are more likely to trust companies that deploy AI responsibly, underscoring the critical business imperative for fairness and transparency.
Integration Complexities: The Hidden Roadblocks
Integrating AI into existing customer support infrastructure—often built on legacy systems—presents significant challenges. Legacy applications may lack architecture suitable for modern AI workloads, leading to data inconsistencies, security vulnerabilities, or operational bottlenecks.
Such integration issues can result in:
- Degraded data quality impacting AI accuracy.
- Increased security risks exposing sensitive customer information.
- Service interruptions during transitional phases.
To navigate these complexities:
-
Adopt incremental integration strategies: Begin with deploying AI in a single support queue for simple queries before scaling across channels, following recommendations from eesel AI.
-
Modernize legacy systems with expert support: Engage companies like Integrass to retrofit AI capabilities without disrupting ongoing operations.
-
Implement robust data governance and privacy safeguards: Ensure transparency about data collection and AI decision-making. Help Scout advocates clearly informing customers when they interact with AI bots to maintain ethical transparency.
Final Thoughts: Transparency and Trust as Cornerstones
The ultimate success of AI-powered customer support hinges on transparency and ethical rigor alongside technical proficiency. Customers expect to be informed when AI is involved, understand its limitations, and have unobstructed access to human assistance.
Best practices to uphold trust include:
- Explicitly disclosing AI involvement during interactions.
- Providing understandable explanations of AI decisions.
- Offering straightforward opt-out or human escalation options.
Balancing automation efficiency with personalized experience is an ongoing journey requiring continuous refinement, ethical vigilance, and human-centric design. When executed thoughtfully, AI does not replace the human element; it empowers it to deliver support that is faster, fairer, and more empathetic.
Challenge | Root Causes / Issues | Troubleshooting Strategies | Examples / Notes |
---|---|---|---|
Loss of Context |
|
|
OpenPrompt Labs: up to 29% boost in user satisfaction by persona assignment |
Over-Automation |
|
|
Air Canada chatbot removed after false bereavement refund promises |
Bias in AI Responses |
|
|
|
Integration Complexities |
|
|
|
Transparency and Trust |
|
|
Key to balancing automation efficiency with personalized experience |
Advanced Techniques and Future Directions: Enhancing Personalization in AI Support with Emerging Technologies
Advanced Techniques and Future Directions: Enhancing Personalization in AI Support with Emerging Technologies
Imagine an AI customer support agent that can see your frustration, hear the subtle stress in your voice, and anticipate your needs before you even ask. This is not a distant sci-fi vision but the trajectory of AI-driven customer support as we approach 2025 and beyond. To balance scaling automation with deep personalization, enterprises are increasingly adopting a sophisticated blend of emerging technologies—combining multimodal data, real-time emotional intelligence, predictive analytics, and privacy-preserving learning methods.
These innovations are reshaping customer support by enhancing context, empathy, and proactive engagement without sacrificing the human touch. Let’s explore how these advanced techniques are transforming the landscape.
Multimodal AI: Beyond Text to Richer, Contextual Understanding
Customer interactions are evolving beyond traditional typed chats or scripted phone calls. Multimodal AI systems—those that integrate text, voice, and visual inputs—are becoming essential to delivering richer, more context-aware support experiences.
-
Why multimodal? Single-mode AI models often miss critical context. For example, an AI that processes only the text of a customer email but ignores accompanying screenshots or the caller’s tone may provide generic or irrelevant responses. Multimodal AI synthesizes these diverse signals to generate tailored, accurate replies.
-
Practical examples: Convin’s AI Phone Calls exemplify this approach by analyzing not just what customers say, but how they say it, through tone and sentiment analysis. This results in empathetic, natural conversations that dynamically adjust to emotional cues.
Similarly, NexGen Cloud’s Hyperstack platform “reads” documents like a human, recognizing tables, signatures, and logos to streamline compliance and customer service simultaneously. By 2025, it is projected that multimodal AI will underpin 100% of customer interactions in forward-looking service organizations.
-
Challenges: Despite its promise, multimodal AI raises complex issues around privacy, bias, and data interpretation. Training these models fairly and accurately demands significant effort and ethical vigilance.
Real-Time Emotional Intelligence and Predictive Analytics: Proactive, Empathetic Support
Advances in emotional AI are enabling machines to move from reactive problem-solving to proactive, empathetic engagement.
-
Emotional intelligence in action: AI now detects customer sentiment in real-time by analyzing natural language, voice tone, and even facial expressions where permitted. This allows AI agents to respond to feelings—calming frustration, acknowledging confusion, or celebrating satisfaction—rather than just words.
-
Market significance: The emotional AI market is expected to reach $91.67 billion by 2025, highlighting its growing operational and commercial importance.
-
Predictive analytics: Complementing emotional insights, predictive models analyze historical and behavioral data to anticipate customer issues before they arise. For instance, Slack’s intelligent assistant, Slackbot, uses personalized prompts during onboarding to reduce friction proactively.
-
Business impact: Organizations employing these techniques report conversion rates boosted by 30-40% and nearly 50% higher revenue per chat hour, driven by timely, relevant, and emotionally attuned interactions.
-
Privacy safeguards: Given the sensitive nature of emotional and behavioral data, privacy-preserving methods are crucial. Federated learning plays a key role here.
Federated Learning: Personalization Without Compromising Privacy
How can AI deliver hyper-personalized support without centralized data collection that risks privacy breaches? Federated learning (FL) offers a compelling solution.
-
What is federated learning? FL trains AI models locally on users’ devices, sharing only model updates—not raw data—with a central aggregator. This approach keeps personal data on-device, significantly enhancing privacy.
-
Innovative combinations: Recent advances integrate FL with multimodal large language models to enable personalized yet privacy-aware AI systems. This balances avoiding overfitting to individual data with maintaining broad applicability.
-
Real-world implications: For customer support, FL allows AI agents to learn from interactions across millions of users without exposing any single user’s data. This enables hyper-personalized assistance while aligning with stringent data protection laws like GDPR and California’s AB 1008, which explicitly cover AI-generated personal information.
-
Trade-offs and challenges: FL presents complexities in communication overhead, model convergence, and fairness across diverse populations. Organizations must manage these carefully to maintain high-quality, equitable service.
Explainable AI: Building Trust Through Transparency
No matter how advanced, AI is only as effective as the trust customers place in it. Explainable AI (XAI) is becoming a cornerstone technology to foster transparency and confidence.
-
The importance of explainability: Today, only 17% of organizations actively address AI explainability, yet customers and regulators increasingly demand clarity on how AI decisions are made, especially in sensitive domains like finance, healthcare, and customer support.
-
Enhancing user experience: XAI demystifies AI recommendations, allowing users to understand the rationale behind actions—such as why a particular troubleshooting step was suggested. This reduces skepticism and helps customers feel in control, not at the mercy of a “black box.”
-
Industry benefits: Transparent AI models help reduce disputes and complaints, improving overall customer satisfaction. Leading companies are adopting XAI frameworks offering model interpretability, post-hoc explanations, and compliance support.
-
Ongoing challenges: Deep learning models remain complex and hard to interpret fully. Standardized XAI frameworks are still emerging, requiring businesses to navigate evolving best practices carefully.
Navigating the Regulatory Landscape: Preparing for Ethical, Scalable AI
The rapid adoption of AI in customer support coincides with an increasingly complex and evolving regulatory environment.
-
Emerging regulations: U.S. states like California, Colorado, and Illinois are pioneering AI-specific legislation focusing on bias mitigation, data privacy, and transparency. California’s AB 1008, for example, extends privacy protections explicitly to AI-generated personal data.
-
Organizational imperatives: Successful AI deployment demands cross-functional collaboration among IT, legal, HR, and business units to audit AI systems proactively for fairness and compliance. Transparency and explainability are not just ethical imperatives but critical risk management tools.
-
Strategic alignment: Building scalable AI roadmaps requires balancing innovation with responsible governance. Embedding ethical principles, continuous monitoring, and adaptability into AI deployments from the outset is essential.
-
Competitive advantage: Organizations that prioritize ethical AI use and compliance are better positioned to differentiate themselves, build lasting customer trust, and withstand regulatory scrutiny.
Recommendations for Building Scalable, Ethical AI Support Systems
To effectively leverage these advanced technologies while preserving personalization, businesses should:
-
Adopt multimodal AI platforms that integrate text, voice, and visual data, enabling richer context and more responsive customer support.
-
Invest in emotional AI and predictive analytics to shift from reactive support to proactive, empathetic engagement.
-
Implement federated learning architectures to deliver personalized experiences while rigorously protecting user privacy and complying with data protection laws.
-
Prioritize explainable AI frameworks to enhance transparency, build customer trust, and facilitate adherence to evolving regulatory requirements.
-
Foster cross-disciplinary collaboration across legal, technical, and business teams to embed ethical considerations and compliance into AI lifecycle management.
-
Develop flexible AI roadmaps that align business objectives with societal values, anticipating future regulation and customer expectations.
By thoughtfully integrating these emerging technologies and ethical frameworks, organizations can automate customer support at scale—without losing the personal touch that defines exceptional service. The future of AI in customer support is not about replacing human connection but augmenting it intelligently and responsibly.
Advanced Technique | Description | Benefits | Challenges | Examples / Notes |
---|---|---|---|---|
Multimodal AI | Integrates text, voice, and visual inputs for richer, context-aware support | Tailored, accurate replies; empathetic and natural conversations | Privacy concerns, bias, complex data interpretation | Convin’s AI Phone Calls; NexGen Cloud’s Hyperstack platform; projected 100% adoption by 2025 |
Real-Time Emotional Intelligence & Predictive Analytics | Detects sentiment and predicts customer issues proactively | Improved conversion rates (30-40%), higher revenue per chat hour (~50%), empathetic engagement | Privacy of emotional/behavioral data; need for privacy-preserving methods | Slackbot personalized onboarding prompts; emotional AI market projected $91.67B by 2025 |
Federated Learning | Trains AI locally on user devices, sharing only model updates to protect privacy | Hyper-personalization without data exposure; compliance with GDPR, AB 1008 | Communication overhead, model convergence, fairness challenges | Combined with multimodal large language models for privacy-aware AI |
Explainable AI (XAI) | Provides transparency on AI decision-making processes | Builds customer trust; reduces disputes; supports regulatory compliance | Deep learning complexity; emerging standards and frameworks | Only 17% of organizations actively address XAI; vital in sensitive domains |
Regulatory Landscape Navigation | Compliance with evolving AI-specific laws focusing on bias, privacy, transparency | Risk management; ethical AI deployment; competitive advantage through trust | Complex cross-functional coordination; continuous auditing needed | California AB 1008, Colorado, Illinois AI legislation; ethical AI principles integration |