AI-Powered Competitive Intelligence: Tools to Outsmart Rivals Today
- Introduction: Why AI is a Game Changer for Competitive Intelligence
- Introduction: Why AI is a Game Changer for Competitive Intelligence
- The Rising Stakes of Competitive Intelligence in Fast-Moving Markets
- From Manual Research to AI-Enabled Real-Time Insights
- The Challenge of Monitoring Vast, Diverse Data — And How AI Answers It
- Balancing Enthusiasm with Critical Perspective: AI is a Tool, Not a Magic Bullet
- Foundations of AI in Competitive Intelligence: Core Technologies and Methodologies
- Foundations of AI in Competitive Intelligence: Core Technologies and Methodologies
- Natural Language Processing: The Linguistic Swiss Army Knife
- Machine Learning: The Crystal Ball of Competitive Intelligence
- Data Mining: Unearthing Nuggets in a Mountain of Information
- Sentiment Analysis: Decoding the Market’s Emotional Pulse
- How AI Techniques Enable Automated CI: From Data Collection to Trend Detection
- AI-Assisted vs. Fully Automated Competitive Intelligence: Where Do We Draw the Line?
- Ethical Considerations and Data Quality: The Foundation of Trustworthy AI-Driven CI
- Technical Architecture and Specifications of Leading AI CI Platforms
- Technical Architecture and Specifications of Leading AI CI Platforms
- Data Ingestion Pipelines and Source Diversity
- AI Models and Real-Time Processing Capabilities
- Scalability, Integration, and Update Frequency
- User Interface Design and Usability
- Security and Privacy Considerations
- Performance Benchmarks and Limitations
- Practical Applications: How Businesses Leverage AI for Market and Competitor Monitoring
- Practical Applications: How Businesses Leverage AI for Market and Competitor Monitoring
- Real-World Use Cases: From Market Trend Analysis to Risk Detection
- Accelerating Decision Cycles: Battlecards, Daily Roundups, and Automated Alerts
- Industries Benefiting Most and Navigating Operational Challenges
- Workflow Integration Tips from the Field
- Comparative Analysis and Benchmarking of AI Competitive Intelligence Tools
- Comparative Analysis and Benchmarking of AI Competitive Intelligence Tools
- Data Source Coverage: Quantity Meets Quality
- AI Sophistication: From Data Collection to Actionable Intelligence
- Customization and Usability: Power Meets Accessibility
- Pricing Models: Transparency and Value
- Customer Support and Vendor Reliability
- Feature Matrix & Best-Use Scenarios
- Cutting Through the Hype: Reality Check on AI CI
- Addressing Gaps and Exploring Niche Solutions
- Future Trends and Emerging Innovations in AI-Driven Competitive Intelligence
- Future Trends and Emerging Innovations in AI-Driven Competitive Intelligence
- Generative AI and Real-Time Data Fusion: From Static Reports to Living Insights
- Predictive Analytics and Automation: Anticipating Moves Before Competitors Do
- Integration with Enterprise Knowledge Graphs and Decision Intelligence Platforms
- Ethical and Regulatory Challenges: Navigating a Complex Landscape
- How AI-Driven Competitive Intelligence Will Shape Market Dynamics
- Conclusion: Navigating Opportunities and Limitations of AI in Competitive Intelligence
- Conclusion: Navigating Opportunities and Limitations of AI in Competitive Intelligence
- The Transformative Potential—and Its Limits
- Ethical Considerations and Human Oversight
- Strategic Alignment and Proactive Experimentation
- Looking Ahead: AI’s Evolving Role and Societal Impact
- Key Takeaways for Practitioners
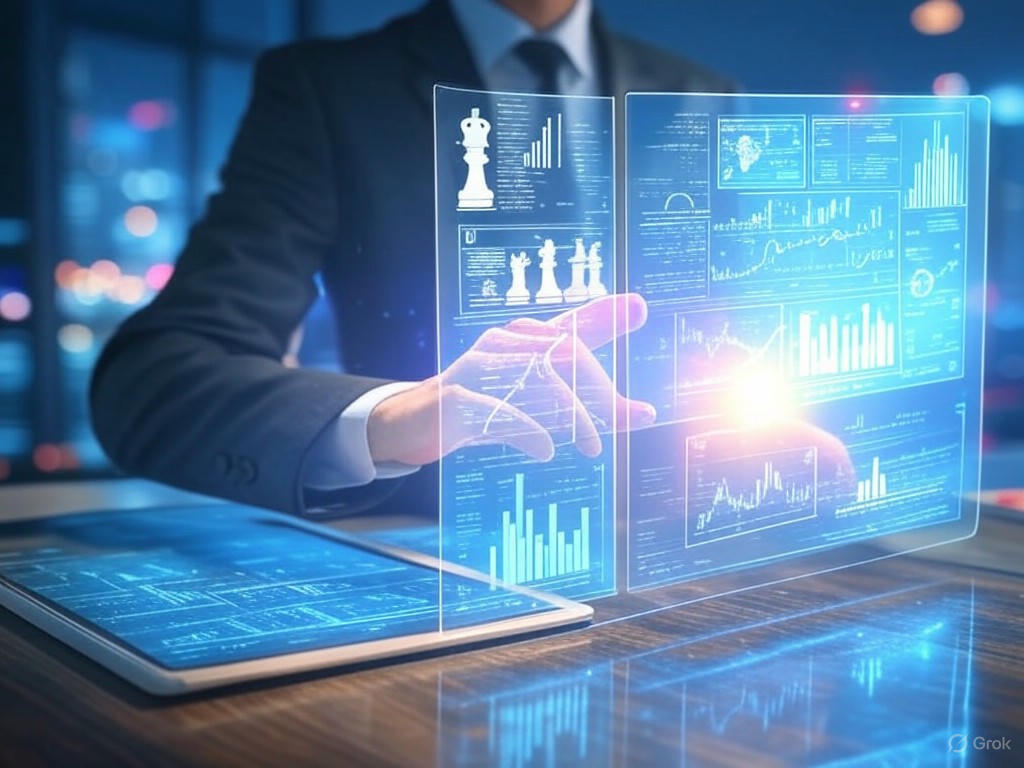
Introduction: Why AI is a Game Changer for Competitive Intelligence
Introduction: Why AI is a Game Changer for Competitive Intelligence
How do businesses stay ahead in markets that seem to shift overnight? The answer lies in competitive intelligence (CI)—but not as we once knew it. CI has evolved from a labor-intensive process of gathering reports and anecdotes into a high-velocity, data-driven discipline powered by artificial intelligence.
The Rising Stakes of Competitive Intelligence in Fast-Moving Markets
Competitive intelligence is now a strategic imperative rather than just a support function. According to AMPLYFI’s 2025 forecast, organizations that can detect subtle signals of market change early and act decisively will outpace their rivals. This holds especially true in sectors like pharmaceuticals, where marketing ROI hinges on sharper insights, and retail, where consumer preferences change daily.
Consider that 90% of Fortune 500 companies leverage CI tools to guide strategic planning (Umer, 2025). These tools do more than report static data; they uncover hidden patterns in competitor behavior, customer sentiment, and even SEO performance. For example, Ahrefs analyzes backlink profiles and keyword strategies to reveal competitors’ digital footprints—offering a powerful edge in digital marketing battles.
Today’s CI goes beyond tracking competitors; it bridges information and action. It enables companies to anticipate competitor moves, gauge customer sentiment, and identify innovation trends. This proactive intelligence translates into tangible benefits: smarter product launches, optimized pricing, and more effective marketing campaigns.
From Manual Research to AI-Enabled Real-Time Insights
If you still think of competitive intelligence as manually sifting through news articles or quarterly reports, it’s time to recalibrate. Traditional CI depended heavily on human analysts compiling static reports—an approach too slow for today’s accelerating pace.
AI is transforming this landscape dramatically. Strategic and Competitive Intelligence Professionals (SCIP) emphasize that CI is becoming smarter, faster, and bolder with AI integration. Instead of waiting for quarterly intel dumps, businesses now use AI-powered tools that continuously crawl millions of data points—from web pages to social networks—to deliver real-time alerts and concise summaries.
For instance, Visualping automates web page monitoring, sending AI-generated summaries when competitors update pricing or product features. Nimbleway aggregates data from hundreds of retail sources, turning static reports into dynamic dashboards that support immediate decisions. This automation not only saves time but uncovers insights that manual analysis might miss or deliver too late.
However, AI is a complement, not a substitute for human expertise. While AI excels at data gathering and pattern recognition, interpreting strategic implications requires the nuance and judgment of skilled analysts. The future of CI lies in this partnership—machines handling scale and speed, humans providing context and foresight.
The Challenge of Monitoring Vast, Diverse Data — And How AI Answers It
Why is AI indispensable for modern competitive intelligence? Because the data landscape is vast and noisy. Take social media: Facebook alone has over 3 billion users, while LinkedIn surpasses 800 million. Monitoring competitor mentions, sentiment, and emerging trends across such volumes is impossible without AI-driven filters, sentiment analysis, and automated alerts.
Beyond social media, companies must monitor competitor websites, pricing changes, regulatory filings, patent databases, and customer reviews. The sheer volume and diversity of data overwhelm traditional CI teams.
AI tackles these challenges on multiple fronts:
- Automated Data Aggregation: AI crawlers continuously scan millions of web pages, news feeds, and financial documents, collecting relevant data without human intervention.
- Advanced Filtering and Sentiment Analysis: Natural language processing (NLP) organizes information into meaningful categories—positive, negative, or neutral—helping prioritize attention.
- Real-Time Alerts: Customized notifications highlight critical competitor moves or market shifts as they occur, enabling swift responses.
- Integration with Business Systems: AI-powered CI tools increasingly integrate with CRM, ERP, and analytics platforms, embedding intelligence into daily workflows.
This technological leap shifts companies from reactive to proactive strategies, allowing them to spot emerging threats or opportunities that would have previously gone unnoticed.
Balancing Enthusiasm with Critical Perspective: AI is a Tool, Not a Magic Bullet
It’s tempting to see AI as a silver bullet for competitive intelligence. Yet, as pointed out by MIT Sloan Review, AI alone does not guarantee sustainable competitive advantage. As AI capabilities become more accessible and standardized, differentiation will increasingly depend on how organizations combine AI with human creativity, domain expertise, and ethical practices.
Moreover, challenges remain around data quality, algorithmic bias, and compliance with privacy laws. Ethical guidelines and responsible AI use are essential to build trust and avoid unintended consequences.
As we explore AI-powered CI tools, it’s important to maintain a balanced perspective: embrace the enormous efficiencies and insights AI offers, but remain vigilant about its limitations and the irreplaceable role of human judgment.
In the sections that follow, we will unpack how today’s AI technologies empower businesses to monitor their markets and rivals more effectively than ever before. From automated web scraping and social listening to predictive analytics, we’ll demystify the tools transforming competitive intelligence—and highlight how to harness them thoughtfully for strategic advantage.
Aspect | Description | Example/Detail |
---|---|---|
Competitive Intelligence Evolution | Shift from manual, labor-intensive reporting to AI-driven, real-time data analysis | Traditional reports vs. AI tools analyzing competitor behavior and market trends |
Strategic Importance | CI as a strategic imperative in fast-moving markets | 90% of Fortune 500 companies use CI tools; sectors like pharma and retail |
AI-Powered Tools | Use of AI to automate data collection and generate insights | Ahrefs for SEO analysis; Visualping for web monitoring; Nimbleway for retail data aggregation |
Data Challenges | Vast and diverse data sources making manual monitoring ineffective | Social media users, competitor websites, pricing, patents, regulatory filings |
AI Capabilities | Automated aggregation, filtering, sentiment analysis, real-time alerts, integration | AI crawlers, NLP categorization, customized notifications, CRM/ERP integration |
Human-AI Partnership | AI complements but does not replace human expertise | Machines handle scale and speed; humans provide context and foresight |
Limitations and Ethics | Challenges with data quality, bias, privacy; need for responsible AI use | MIT Sloan Review insights; importance of ethical guidelines and human judgment |
Foundations of AI in Competitive Intelligence: Core Technologies and Methodologies
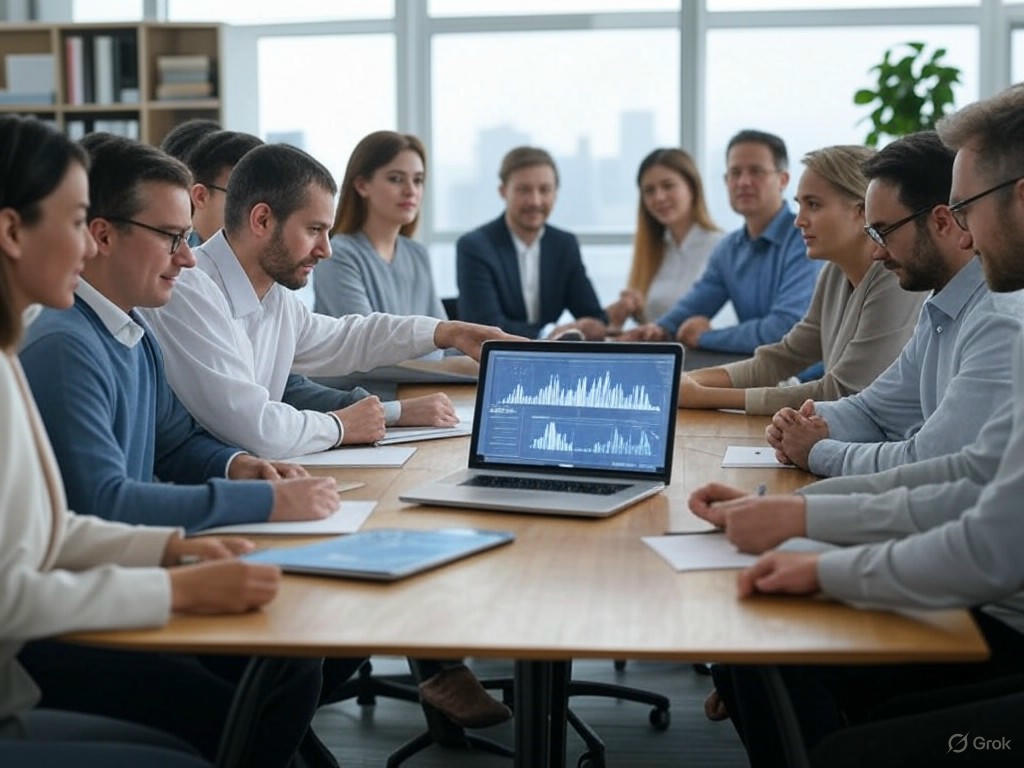
Foundations of AI in Competitive Intelligence: Core Technologies and Methodologies
What powers the AI tools that are revolutionizing how we monitor markets and competitors? At the core of modern Competitive Intelligence (CI) are advanced AI technologies—natural language processing (NLP), machine learning (ML), data mining, and sentiment analysis. These are not mere buzzwords; they form the foundational engines that automate data collection, reveal hidden patterns, and distill vast information into actionable insights.
Natural Language Processing: The Linguistic Swiss Army Knife
Imagine trying to manually read every article, report, social media post, and financial statement relevant to your industry every day—it’s an impossible task for any human. NLP acts like a linguistic Swiss Army knife, enabling machines to understand, interpret, and generate human language effectively across diverse formats and languages.
NLP’s role in CI is multifaceted:
- Automated Data Extraction: Tools like Semblian 2.0 scan industry reports and online data, pulling relevant competitor activity with precision.
- Summarization: Platforms such as Sebbly AI record and transcribe meetings, providing concise, real-time summaries that save hours of manual note-taking.
- Language Translation and Context Understanding: This capability extends monitoring across geographies and languages, breaking down traditional barriers.
- Sentiment Analysis: Algorithms analyze customer opinions and emotions embedded in text, revealing trends in public perception and market sentiment.
Advancements in NLP by 2025 focus on improved accuracy and deeper contextual awareness, allowing AI to grasp nuances beyond simple keyword matching. Yet, these capabilities also raise challenges: bias in training data can skew interpretations, and privacy concerns grow as AI sifts through sensitive information. Ethical awareness is critical when deploying NLP-driven CI tools, ensuring compliance with regulations like GDPR and CCPA.
Machine Learning: The Crystal Ball of Competitive Intelligence
Machine learning serves as a seasoned analyst who learns from every piece of data, spotting trends and making predictions with increasing accuracy. In fast-moving, volatile markets, ML models forecast competitor moves, customer lifetime value, and emerging opportunities, empowering companies to act proactively rather than reactively.
Think of ML as a detective who, by repeatedly examining clues (data), refines their intuition about where the competition is heading. Key processes include:
- Training models on historical and real-time data
- Recognizing subtle patterns invisible to humans
- Adapting dynamically to new information
The global ML market is booming, projected to reach $113 billion in 2025, underscoring its central role in CI and beyond. However, only about 12% of IT professionals have significant ML experience, pointing to a critical skills gap that organizations must address to fully leverage ML’s potential.
Data Mining: Unearthing Nuggets in a Mountain of Information
If ML is the detective, data mining is the prospector, digging through massive raw datasets to discover valuable patterns and correlations. In CI, data mining goes beyond mere extraction—it transforms raw data into strategic insights that can inform decision-making.
For instance, pharmaceutical companies utilize AI-driven data mining not only to track competitor drug pipelines but also to investigate anomalies and assess the credibility of sources with human oversight. This hybrid approach ensures that the intelligence gathered is both accurate and relevant.
Data mining’s effectiveness depends heavily on data quality. Poor data leads to misleading patterns and costly mistakes; thus, companies invest significantly in data validation technologies to ensure clean, reliable inputs for AI models.
Sentiment Analysis: Decoding the Market’s Emotional Pulse
While numbers tell one story, emotions tell another. Sentiment analysis uses NLP algorithms to interpret the emotional tone underlying customer reviews, social media chatter, and news articles. This insight helps businesses gauge public mood around brands, products, or market events.
Consider sentiment analysis as a market mood ring that signals when a competitor’s product launch is met with enthusiasm or skepticism. This real-time feedback informs marketing strategies, product development, and innovation pipelines.
However, capturing the nuances of human emotion—including sarcasm and cultural context—remains challenging. Sentiment models trained on biased or limited datasets may misinterpret sentiments, underscoring the necessity of continuous refinement and human validation.
How AI Techniques Enable Automated CI: From Data Collection to Trend Detection
At its core, AI-driven CI automates what was once painstaking manual work. Tools like Crayon and Kompyte monitor millions of sources—websites, social media, job postings, and review platforms—aggregating data in real time. This automation frees analysts to focus on strategic interpretation rather than data gathering.
Picture AI as a fleet of autonomous drones surveying a vast landscape. Each drone collects different data types—text, numbers, images—and relays them to a central hub where pattern recognition algorithms sift through the noise to identify meaningful signals.
Key AI capabilities in CI include:
- Automated Data Collection: AI crawlers and scrapers extract and unify data from diverse, often unstructured sources.
- Pattern Recognition: Machine learning models detect shifts in competitor behavior or emerging market trends.
- Summarization: NLP condenses large volumes of information into digestible, actionable reports.
- Trend Detection: Early signals of market changes are identified before they become obvious, enabling preemptive action.
Despite these advances, AI is not infallible. Data quality issues, biases, and noise can mislead automated systems. Hence, human expertise remains indispensable to interpret, verify, and contextualize AI outputs effectively.
AI-Assisted vs. Fully Automated Competitive Intelligence: Where Do We Draw the Line?
A crucial distinction in AI-driven CI is between AI-assisted and fully automated processes. AI-assisted systems augment human analysts by handling repetitive tasks such as data collection, preliminary analysis, and report generation, while leaving final interpretation and strategic decisions to humans.
Fully automated CI envisions AI agents autonomously collecting, analyzing, and acting on intelligence without human intervention. Although promising, this frontier faces current technological limitations and significant ethical concerns.
Human oversight is essential for:
- Assessing source credibility
- Investigating anomalies or unexpected results
- Detecting and mitigating bias
- Ensuring ethical data use and compliance
The future of CI likely lies in hybrid models that combine AI’s data-processing power with human judgment and strategic thinking. The pharmaceutical industry’s experience exemplifies this: AI accelerates intelligence gathering, but the most impactful insights emerge from human-AI collaboration.
Ethical Considerations and Data Quality: The Foundation of Trustworthy AI-Driven CI
AI systems are only as reliable as the data they consume. Poor data quality can lead to flawed insights, resulting in costly strategic errors. Organizations must invest in rigorous data validation, cleansing, and integration processes to maintain high-quality inputs.
Bias in AI models—stemming from skewed training data or algorithmic blind spots—can perpetuate unfair or inaccurate conclusions. For example, sentiment analysis might underrepresent minority voices if training data lacks diversity.
Ethical and legal considerations are equally critical. Privacy, transparency, and consent must be respected to avoid reputational damage and regulatory penalties. Responsible AI use involves:
- Implementing transparent AI methodologies
- Ensuring data privacy and regulatory compliance
- Maintaining human oversight to detect ethical pitfalls
- Regularly auditing AI outputs for bias and accuracy
In summary, the AI technologies underpinning competitive intelligence—NLP, machine learning, data mining, and sentiment analysis—offer unprecedented capabilities to monitor markets and rivals at scale. These tools are not magic; they require high-quality data, ethical application, and a collaborative human partnership to deliver true strategic advantage. Understanding their strengths, limitations, and responsibilities is the first step toward leveraging AI effectively in competitive intelligence.
AI Technology | Role in Competitive Intelligence | Key Capabilities | Challenges and Considerations | Examples/Tools |
---|---|---|---|---|
Natural Language Processing (NLP) | Enables machines to understand, interpret, and generate human language across formats and languages for data extraction and summarization. |
|
|
Semblian 2.0, Sebbly AI |
Machine Learning (ML) | Analyzes data to spot trends, make predictions, and forecast competitor moves for proactive decision-making. |
|
|
General ML frameworks; market projected $113B in 2025 |
Data Mining | Extracts and transforms raw data into strategic insights and identifies valuable patterns for decision-making. |
|
|
Pharmaceutical industry AI-driven mining |
Sentiment Analysis | Interprets emotional tone in text to gauge public mood and market sentiment. |
|
|
NLP-based sentiment algorithms |
AI-Assisted vs Fully Automated CI | Distinguishes between AI augmenting humans and AI operating autonomously in CI processes. |
|
|
Hybrid human-AI models in pharmaceuticals |
Ethical Considerations & Data Quality | Ensures trustworthy AI-driven CI by maintaining data integrity and ethical standards. |
|
|
N/A |
Technical Architecture and Specifications of Leading AI CI Platforms
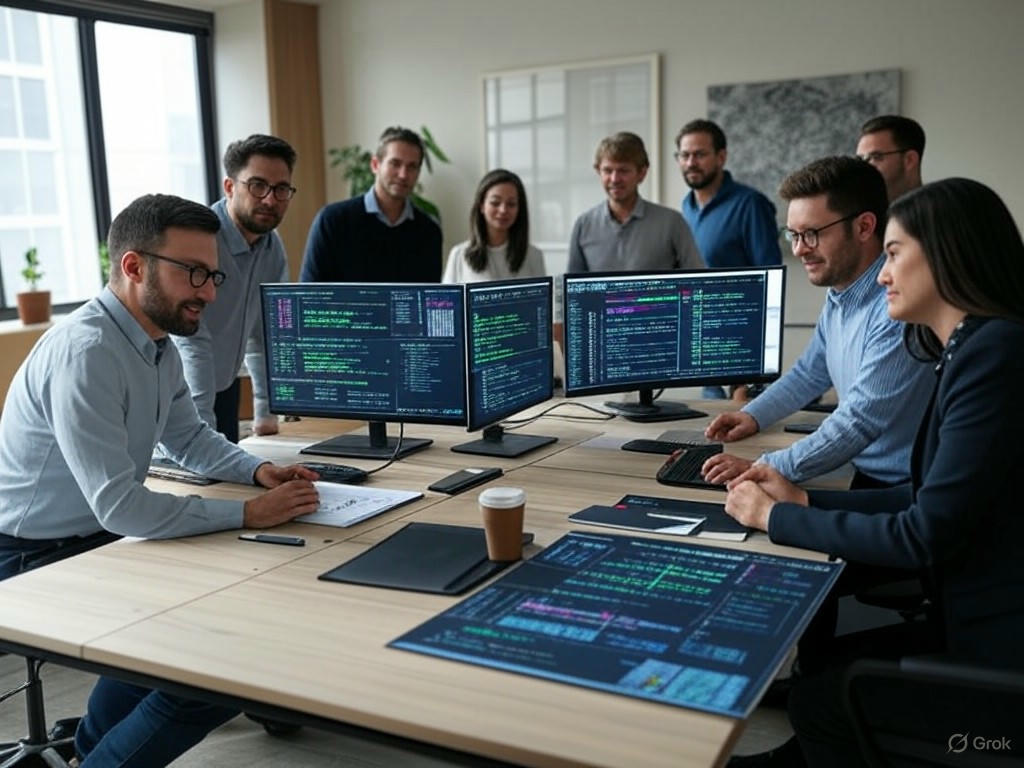
Technical Architecture and Specifications of Leading AI CI Platforms
What powers today’s competitive intelligence (CI) platforms beneath the surface? A closer look into the technical frameworks of top AI CI tools—such as Crayon, Klue, Kompyte, and Semrush—reveals how these systems convert vast, raw market data into timely, actionable insights. This section dissects their data ingestion mechanisms, AI models, real-time processing capabilities, scalability, integration options, and security features to present a clear understanding of their strengths and limitations.
Data Ingestion Pipelines and Source Diversity
At the heart of every CI platform is its ability to continuously gather and curate pertinent data. Leading tools rely on a comprehensive array of sources to capture market dynamics:
-
Crayon scans a vast spectrum of online and offline data, including competitor websites, social media channels, press releases, and financial reports. It monitors over seven million sources, delivering real-time market signals. While Crayon excels in focused coverage, it encounters scalability challenges when tracking extremely large competitor sets due to its ingestion pipeline’s optimization for depth over breadth.
-
Klue blends automation with human curation. Its data pipeline harvests information from websites, social media, product launches, press mentions, and reviews, enriched by user-added research insights. This hybrid approach enhances data quality and context, supporting tools like Klue’s AI-powered Review Insights, which analyzes buyer reviews to surface competitive differentiators and pain points.
-
Kompyte focuses on dynamic competitor tracking and alerts, pulling data from various marketing channels, including websites, social media, job listings, and review sites. Its emphasis is on enabling timely responses to competitor moves.
-
Semrush stands out for its massive data ingestion scale, sourcing from over 500,000 sources such as company websites, job portals, regulatory filings, social media, and news outlets. Its extensive and diverse dataset underpins comprehensive competitive and market intelligence, particularly in sectors like BFSI, pharmaceuticals, and IT.
These platforms employ a combination of web crawlers, APIs, RSS feeds, and partnerships with trusted data providers to ensure data freshness and coverage. For instance, Semrush leverages its SEO heritage with robust infrastructure for continuous web crawling and indexing, feeding its competitive analytics engine with up-to-date information.
AI Models and Real-Time Processing Capabilities
Transforming raw data into strategic insight relies heavily on advanced AI analysis. Most leading CI platforms utilize sophisticated Natural Language Processing (NLP) techniques, often grounded in transformer-based architectures such as BERT, GPT variants, or proprietary models fine-tuned specifically for competitive intelligence.
-
Transformer-based NLP models enable nuanced semantic understanding of unstructured text from sources like reviews, press releases, and social media chatter. This capability allows platforms like Klue and Crayon to automatically extract competitor strengths, weaknesses, product launches, and sentiment fluctuations with remarkable precision.
-
On top of these foundational models, custom classifiers and entity recognition components categorize data by competitor, product line, or market segment. For example, Klue’s Review Insights leverages these layers to analyze buyer feedback and highlight competitive advantages or challenges.
-
Real-time processing pipelines are critical, where data ingestion is immediately followed by AI-driven analysis. Crayon’s Sparks AI tool exemplifies this by converting data overload into prioritized, actionable intelligence, freeing analysts from manual sifting and enabling near-instantaneous alerts.
-
Scalability in AI inference is achieved through cloud-native deployments using containerized microservices or serverless functions. This elastic architecture accommodates surges in data volume or query load without performance degradation.
It is important to note that the accuracy of these AI models depends heavily on the quality and representativeness of the training data. Given the rapidly evolving competitive landscapes, continuous model retraining and validation are essential to maintain insight relevance and prevent biases or outdated conclusions.
Scalability, Integration, and Update Frequency
Managing vast volumes and high-velocity data is a defining challenge for CI platforms. Their approaches include:
-
Crayon and Semrush leverage robust, cloud-native architectures designed for global scalability. Crayon, now part of SoftwareOne, benefits from a platform capable of rapid updates and cost-effective scaling, serving enterprise clients with extensive monitoring requirements.
-
Semrush offers comprehensive APIs and integration points, facilitating seamless connectivity with CRM systems, analytics platforms, and marketing tools. This interoperability enables embedding competitive intelligence directly into business workflows, thereby accelerating decision-making processes.
-
Klue integrates natively with major enterprise communication tools like Salesforce and Slack, allowing real-time collaboration and intelligence sharing. Sales and product teams receive automated competitive alerts within their existing channels, enhancing responsiveness.
-
Update frequencies generally trend toward near-real-time or daily refresh cycles, depending on data source volatility. Semrush’s continuous web crawling ensures its SEO and keyword datasets remain current, while Klue and Crayon provide frequent intelligence updates to capture market dynamics promptly.
User Interface Design and Usability
A sophisticated backend requires an intuitive and efficient user experience to maximize value:
-
Klue emphasizes a centralized platform where competitive intelligence is automatically curated, analyzed, and shared across departments. Its user interface facilitates seamless navigation from high-level competitor overviews down to detailed insights such as quote comparisons and win-loss analyses.
-
Semrush organizes its tools into clearly segmented modules—SEO, PPC, content marketing, and competitive research—enabling users to handle complex data without overwhelm. Its dashboard is praised for balancing comprehensive depth with ease of use.
-
Crayon’s interface focuses on real-time market signals, featuring visual trend tracking and actionable notifications. It is positioned as a strategic enablement platform for sales and marketing teams, fostering timely responses to competitive moves.
Security and Privacy Considerations
Given the sensitivity and proprietary nature of competitive data, robust security and privacy measures are non-negotiable:
-
Klue complies with stringent privacy standards, including PIPEDA, GDPR, and CCPA. It employs the June 2021 Standard Contractual Clauses to ensure data protection across jurisdictions.
-
Crayon and Semrush maintain enterprise-grade security protocols, including encryption of data both in transit and at rest, role-based access controls, and regular third-party audits. These measures safeguard client information against unauthorized access.
-
Transparency around AI model usage and data provenance is increasingly emphasized to build user trust. Organizations seek assurances about the ethical sourcing of intelligence and the accuracy of AI-generated insights, especially when these insights influence strategic decisions.
Performance Benchmarks and Limitations
Detailed, independent performance benchmarks remain limited due to proprietary data and competitive confidentiality. Nevertheless, user feedback and industry observations offer valuable insights:
-
Semrush’s Organic Research dashboard is widely recognized as a leading tool for competitor keyword intelligence and market positioning, with responsiveness and data accuracy frequently highlighted.
-
Klue is lauded for automating the analysis of vast data points, significantly reducing manual workloads and accelerating insight delivery to sales and marketing teams.
-
Crayon’s Sparks AI tool represents a notable advancement in converting raw data into prioritized intelligence. However, its effectiveness may be constrained when monitoring extremely large competitor sets due to ingestion pipeline limitations.
It is vital for organizations to recognize that no platform provides a complete or flawless view. Data gaps, AI misinterpretations, and evolving market behaviors introduce inherent uncertainties. Consequently, these tools should be considered as augmentative aids complementing, rather than replacing, human analysis and domain expertise.
In summary, leading AI competitive intelligence platforms combine sophisticated, scalable data pipelines, advanced transformer-based NLP models, real-time processing, seamless integrations, and user-centric interfaces. While these capabilities empower businesses to gain timely and actionable market insights, balancing automation with accuracy, scalability with specificity, and integration with security remains an ongoing challenge. Understanding these architectural nuances helps enterprises make informed decisions when selecting and deploying AI-driven competitive intelligence tools.
Aspect | Crayon | Klue | Kompyte | Semrush |
---|---|---|---|---|
Data Sources | Online and offline data (websites, social media, press releases, financial reports); monitors 7M+ sources | Websites, social media, product launches, press mentions, reviews, user-added research | Websites, social media, job listings, review sites | 500,000+ sources including company websites, job portals, regulatory filings, social media, news |
Data Ingestion Methods | Web crawlers, APIs, RSS feeds | Automation plus human curation | Focus on dynamic tracking and alerts | Continuous web crawling and indexing leveraging SEO infrastructure |
AI Models | Transformer-based NLP (BERT, GPT variants), custom classifiers, entity recognition; Sparks AI for prioritization | Transformer-based NLP, Review Insights analyzing buyer feedback with custom classifiers | Not specified; focus on timely competitor alerts | Transformer-based NLP, proprietary models fine-tuned for CI |
Real-Time Processing | Near-instantaneous alerts via Sparks AI tool | Frequent updates with real-time collaboration | Enables timely responses to competitor moves | Continuous data crawling and updates |
Scalability | Cloud-native architecture, optimized for depth over breadth; challenges with very large competitor sets | Cloud-based; integrates with enterprise tools like Salesforce and Slack | Not detailed | Cloud-native, global scalability with cost-effective scaling |
Integration | Not specifically detailed | Native integrations with Salesforce, Slack for collaboration and alerts | Not specified | Comprehensive APIs for CRM, analytics, marketing tools |
Update Frequency | Frequent intelligence updates, near real-time | Near real-time or daily refresh cycles | Not specified | Continuous crawling ensures up-to-date SEO and keyword data |
User Interface Focus | Real-time market signals, visual trend tracking, actionable notifications | Centralized curation and analysis, detailed insights like quote comparisons, win-loss analyses | Not specified | Segmented modules for SEO, PPC, content marketing, competitive research; ease of use |
Security & Privacy | Enterprise-grade encryption, role-based access, third-party audits | Compliant with PIPEDA, GDPR, CCPA; uses June 2021 Standard Contractual Clauses | Not specified | Enterprise-grade encryption, role-based access, third-party audits |
Performance & Limitations | Effective prioritization but limited scalability for very large competitor sets | Automates analysis, reduces manual workload, accelerates insight delivery | Not specified | Highly regarded for organic research and market positioning accuracy |
Practical Applications: How Businesses Leverage AI for Market and Competitor Monitoring

Practical Applications: How Businesses Leverage AI for Market and Competitor Monitoring
What does it truly mean to harness AI for competitive intelligence in 2025? The answer lies not in futuristic fantasies but in concrete, real-world examples where AI tools are transforming how businesses track rivals, decode market signals, and sharpen decision-making. AI is shifting competitive intelligence from a manual, laborious chore into a streamlined, insight-rich process.
Real-World Use Cases: From Market Trend Analysis to Risk Detection
Consider the challenge of monitoring millions of data points daily—from social media chatter and job postings to pricing changes and product launches. AI-powered platforms like Crayon and Contify tackle this by scanning over seven million and more than a million vetted sources, respectively. Using machine learning and natural language processing (NLP), they distill vast raw data into actionable insights, enabling organizations to spot market trends long before they become obvious.
For instance, market trend analysis has evolved beyond sifting through endless industry reports. Tools like Semblian 2.0 automatically scan financial statements and online data, detecting subtle shifts that signal emerging opportunities or threats. Similarly, BuzzSumo employs AI algorithms to analyze content performance and social engagement metrics, helping marketers understand which narratives resonate best in real time.
Competitor campaign tracking has also transformed. Platforms such as Kompyte monitor competitors’ activities across websites, social media, job listings, and review sites. This multi-faceted surveillance allows companies to keep tabs on product positioning and promotional tactics effortlessly.
Pricing intelligence is another domain revolutionized by AI. Tools like Engage3 merge AI, behavioral science, and machine learning to help retailers optimize pricing strategies—not just to maximize margins but also to enhance their “price image,” a subtle yet critical factor in competitive positioning.
On the front of risk detection, AI flags anomalies such as sudden changes in competitor website designs, supply chain disruptions, or shifts in customer sentiment. Platforms like ChangeTower provide customizable alerts on these changes, enabling swift responses before risks escalate.
Accelerating Decision Cycles: Battlecards, Daily Roundups, and Automated Alerts
How do these insights translate into tangible business advantages? The key lies in speed and precision. Sales teams, for example, leverage AI-generated battlecards—concise, dynamic documents enriched with competitive intelligence—to win deals. Supported by platforms like Klue, these battlecards equip sales reps to counter objections with up-to-date facts and strategic messaging instantly.
Daily competitor roundups are another powerful feature. AI tools compile the latest competitor moves, market news, and relevant data into digestible summaries delivered via Slack or email. This keeps entire teams aligned and informed without manual research.
At the heart of proactive monitoring are automated alerts. Whether it’s a price change, new product launch, or a shift in customer reviews, AI systems notify stakeholders immediately. This reduces the lag between intelligence gathering and action, compressing decision cycles from days to mere minutes.
Take Steve, an AI-native competitive intelligence platform built by ex-Amazon and Apple veterans. It integrates directly into business communication tools, delivering fresh insights and automating research tasks on demand, exemplifying seamless AI adoption.
Industries Benefiting Most and Navigating Operational Challenges
Certain sectors reap outsized benefits from AI-driven competitive intelligence. The retail industry leads, with 75% of executives viewing generative AI as essential to revenue growth. Here, AI not only tracks pricing and inventory levels but also personalizes customer experiences and optimizes marketing campaigns.
Financial services leverage AI for risk assessment and market forecasting, gaining efficiency and precision. Similarly, technology, manufacturing, and healthcare sectors employ AI to monitor competitors’ innovations, regulatory changes, and customer feedback in real time.
Yet, AI CI tools face challenges. A primary concern is data overload: the digital universe generates upwards of 2.5 quintillion bytes daily, projected to reach 463 exabytes per day by 2025. Without robust filtering, businesses risk drowning in noise, missing critical signals or generating false positives.
Moreover, AI can sometimes flag irrelevant or misleading data, requiring human expertise for validation. Platforms like Contify address this by combining AI with human curation, ensuring the intelligence delivered is comprehensive and contextually accurate.
User experience is another critical factor. Seamless integration into existing workflows—whether CRM, Slack, or email—is essential for adoption and impact. Tools embedded directly into daily communication channels or automating mundane tasks tend to see higher engagement and faster ROI.
Workflow Integration Tips from the Field
Drawing from over 15 years of experience architecting AI systems, success with AI CI tools hinges on thoughtful integration:
- Embed AI outputs where decisions happen: Utilize Slack bots, email alerts, or direct CRM integrations to ensure timely delivery of insights without disrupting workflows.
- Customize alert thresholds: Calibrate notification sensitivity to business priorities to avoid alert fatigue and false alarms.
- Combine AI with domain expertise: While AI excels at pattern detection, human judgment remains vital to interpret nuance and strategic implications.
- Iterate and refine continuously: Competitive landscapes evolve rapidly; regularly calibrate AI models and data sources to maintain relevance.
- Train teams on AI literacy: Empower users to understand AI’s capabilities and limitations, fostering trust and smarter utilization.
In summary, AI-powered competitive intelligence transforms vast, complex data into focused, actionable insights that accelerate decision-making and sharpen competitive positioning. While operational challenges such as data overload and false positives remain, thoughtfully deployed AI tools—coupled with human oversight—represent a leap forward in strategic agility and efficiency. For businesses committed to integration and curation, AI is no longer optional; it’s a competitive imperative.
Application | AI Tools | Functionality | Key Benefits |
---|---|---|---|
Market Trend Analysis | Crayon, Contify, Semblian 2.0, BuzzSumo | Scan and analyze millions of data points, financial statements, and content performance | Early detection of market shifts, actionable insights from vast data, real-time understanding of trending narratives |
Competitor Campaign Tracking | Kompyte | Monitor competitors’ websites, social media, job listings, and review sites | Effortless tracking of product positioning and promotional tactics |
Pricing Intelligence | Engage3 | Optimize pricing strategies using AI, behavioral science, and machine learning | Maximize margins and enhance price image for competitive positioning |
Risk Detection | ChangeTower | Flag anomalies such as website changes, supply chain disruptions, customer sentiment shifts | Customizable alerts enabling swift risk response |
Decision Support (Battlecards) | Klue | Generate dynamic, AI-enriched battlecards for sales teams | Equip sales reps with up-to-date facts and strategic messaging to win deals |
Daily Competitor Roundups | Various AI tools | Compile competitor moves, market news, and relevant data into summaries | Keep teams aligned and informed without manual research |
Automated Alerts | Steve, ChangeTower, others | Immediate notifications on price changes, product launches, customer reviews | Compress decision cycles from days to minutes |
Industry Adoption | Multiple | Retail, Financial Services, Technology, Manufacturing, Healthcare | Track pricing, risk, innovations, regulatory changes, customer feedback |
Comparative Analysis and Benchmarking of AI Competitive Intelligence Tools
Comparative Analysis and Benchmarking of AI Competitive Intelligence Tools
What truly distinguishes an AI-powered competitive intelligence (CI) tool that drives strategic advantage from one that merely adds to the data noise? The difference lies in a rigorous, evidence-based evaluation of capabilities—beyond flashy features or marketing claims. In 2025, the CI tool landscape is vast and varied, spanning from highly specialized niche platforms to expansive, enterprise-grade ecosystems.
This section cuts through the hype to systematically compare leading AI CI platforms across key criteria: data source coverage, AI sophistication, customization, ease of use, pricing models, and customer support. We analyze each tool’s strengths and limitations relative to business needs and scale, provide a feature matrix, and recommend scenarios where each excels. Importantly, we emphasize transparency and critical assessment to separate marketing promises from real-world capabilities.
Data Source Coverage: Quantity Meets Quality
At the foundation of any CI tool is the data it ingests. But bigger isn’t always better—quality and relevance matter as much as volume.
-
Crayon monitors over seven million online and offline sources, providing real-time updates on competitors’ every move. Its vast coverage makes it a favorite for large enterprises requiring comprehensive market visibility.
-
Contify combines a proprietary crawling system with advanced natural language processing (NLP) and machine learning to sift through more than one million vetted sources. Contify’s focus on sectors like BFSI, IT, and consulting allows for tailored, industry-specific insights.
-
AlphaSense takes a quality-over-quantity approach by aggregating over 10,000 external data sources, including financial documents and expert research. This aligns well with analysts who prioritize depth and high-value content over sheer volume.
-
Similarweb offers extensive data on over 100 million websites and 4.7 million mobile apps but emphasizes digital performance and website traffic analysis, making it particularly strong for retail and digital marketing intelligence rather than holistic competitor tracking.
-
Semrush and SpyFu focus on SEO and PPC competitor data, serving digital marketing teams with specialized insights and user-friendly interfaces.
Key insight: Larger data coverage provides more raw input but demands sophisticated AI to filter signal from noise. Select platforms that align their data sources with your industry and intelligence objectives to avoid being overwhelmed by irrelevant information.
AI Sophistication: From Data Collection to Actionable Intelligence
AI sophistication goes beyond integrating the latest machine learning models—it’s about effectively converting vast data into timely, actionable insights.
-
Crayon and Contify employ advanced NLP and machine learning to detect competitor moves and market shifts in near real-time, supporting fast, informed decision-making.
-
Semblian 2.0 extends beyond aggregation by scanning financial statements and industry reports to flag emerging trends, thereby enabling strategic foresight.
-
BuzzSumo leverages AI algorithms to analyze social engagement and content performance, helping marketers decode competitive messaging dynamics.
-
AlphaSense stands out in the financial sector by combining AI-powered search with expert research libraries, accelerating insight discovery for analysts and researchers.
Despite these advances, AI remains a complement—not a substitute—for human judgment. As highlighted in the introduction, these tools amplify human decision-making capabilities but cannot fully replace nuanced strategic reasoning. Transparency and explainability remain critical, particularly given ongoing concerns about AI’s “black box” nature.
Customization and Usability: Power Meets Accessibility
No two organizations have identical CI needs, and the ability to customize workflows and integrate seamlessly is a major differentiator.
-
Klue offers robust integration capabilities, syncing competitive insights directly with Salesforce and Slack. This embedded intelligence supports medium to large enterprises seeking real-time distribution of insights within sales and marketing workflows.
-
SpyFu and SEMrush cater especially well to digital marketing teams with specialized SEO and keyword intelligence, providing tiered pricing and intuitive interfaces suited for small businesses and agencies.
-
Visualping provides a lightweight, scalable solution for website change monitoring, ideal for teams seeking precise, low-overhead alerts.
More powerful platforms like AlphaSense or Contify, while highly customizable and feature-rich, often require a steeper learning curve and dedicated analyst resources to realize their full potential.
Pricing Models: Transparency and Value
Pricing in AI CI often involves complex, tiered subscription models that can obscure true cost and value.
-
Seamless AI offers affordable entry points but has faced criticism for billing inconsistencies and data accuracy concerns, illustrating that low cost doesn’t always translate to cost-effectiveness.
-
Ahrefs and SEMrush provide free trials and scaled premium plans, making them accessible options for smaller teams exploring AI-powered CI.
-
Enterprise-grade platforms like Klue and Contify typically employ custom pricing models aligned with organizational scale and customization requirements, reflecting their comprehensive feature sets.
Businesses are advised to prioritize return on investment (ROI) by aligning platform capabilities with specific objectives rather than simply selecting the most feature-rich or expensive solution.
Customer Support and Vendor Reliability
Strong vendor support and reliability are vital, especially when integrating AI CI tools into complex enterprise workflows.
-
Platforms like NICE have earned recognition for technological excellence and customer impact, underscoring the value of robust post-sale services.
-
Conversely, mixed reviews around Seamless AI’s support highlight risks when rapid scaling outpaces service investment.
Vendors with established reputations, clear communication channels, and proactive customer success teams tend to foster better long-term partnerships—critical as AI tools evolve and require ongoing tuning and training.
Feature Matrix & Best-Use Scenarios
Tool | Data Coverage | AI Sophistication | Customization | Ease of Use | Pricing Model | Best Use Case |
---|---|---|---|---|---|---|
Crayon | 7M+ sources (online/offline) | Advanced NLP, real-time alerts | High, team-oriented | Moderate | Custom enterprise | Large enterprises needing real-time market moves |
Contify | 1M+ vetted sources | ML, NLP, proprietary crawling | Industry-specific customization | Moderate to complex | Custom, mid to enterprise | BFSI, IT, consulting industries |
AlphaSense | 10,000+ sources + expert docs | AI-powered search and analytics | Moderate | Moderate to complex | Custom | Financial analysts, researchers |
SEMrush | SEO-focused, keyword data | AI for SEO and content analysis | Moderate | High | Tiered subscriptions | Digital marketing and SEO teams |
Similarweb | 100M+ websites and apps | Traffic and engagement analytics | Moderate | High | Tiered subscriptions | Retail and digital performance benchmarking |
Klue | Aggregated competitor data | AI-driven insights | High, CRM integration | Moderate | Custom, enterprise | Sales enablement, competitive insight sharing |
SpyFu | SEO and PPC competitor data | Specialized AI for search trends | Limited | High | Flexible, affordable | Small to medium digital marketing teams |
Visualping | Website change monitoring | Simple AI for change detection | Low | Very high | Free and premium tiers | Lightweight monitoring for marketing/product teams |
Cutting Through the Hype: Reality Check on AI CI
Despite bold claims about AI’s transformative potential, adoption remains uneven and complex.
-
As of early 2024, only about 5.4% of firms had fully integrated generative AI into business processes (Google Cloud 2025 State of AI Infrastructure Report).
-
Many AI CI tools still face challenges in transparency, explainability, and consistent deliverability relative to their marketing hype.
-
Autonomous AI agents, often portrayed as fully independent problem solvers, remain tools that augment human expertise rather than replace it (IBM experts).
-
Data quality and ethical considerations continue to be pressing concerns; for example, Seamless AI experiences with billing and accuracy issues illustrate risks in less mature platforms.
The most effective AI CI solutions blend automated data aggregation with human strategic thinking, emphasizing transparency, bias mitigation, and ethical compliance.
Addressing Gaps and Exploring Niche Solutions
While AI CI tools have advanced considerably, notable gaps remain:
-
Industry Specialization: Deep vertical customization is limited outside finance, IT, and retail, leaving sectors such as manufacturing, healthcare, and niche B2B markets underserved.
-
Human-AI Collaboration: Current tools could better facilitate hybrid workflows, enabling analysts to validate, contextualize, and refine AI-generated insights through intuitive interfaces.
-
Ethical Transparency: There is a need for enhanced explainability, bias mitigation, and adherence to data privacy regulations as scrutiny intensifies globally.
-
Accessibility for Small Businesses: Although tiered pricing exists, genuinely affordable and effective AI CI solutions tailored to small enterprises remain scarce.
Emerging niche platforms like Visualping for precise website change monitoring and BuzzSumo for content performance intelligence provide valuable specialized capabilities often overlooked by larger, more generalized tools.
In summary, selecting an AI competitive intelligence platform requires a strategic balance of data relevance, AI capability, usability, and pricing aligned with your organizational scale and goals. Avoid the allure of “all-in-one” solutions without transparent, evidence-backed performance. Prioritize platforms that support human-in-the-loop workflows, ethical AI use, and offer clear, actionable insights—those are the tools that truly transform raw data into sustainable competitive advantage.
Tool | Data Coverage | AI Sophistication | Customization | Ease of Use | Pricing Model | Best Use Case |
---|---|---|---|---|---|---|
Crayon | 7M+ sources (online/offline) | Advanced NLP, real-time alerts | High, team-oriented | Moderate | Custom enterprise | Large enterprises needing real-time market moves |
Contify | 1M+ vetted sources | ML, NLP, proprietary crawling | Industry-specific customization | Moderate to complex | Custom, mid to enterprise | BFSI, IT, consulting industries |
AlphaSense | 10,000+ sources + expert docs | AI-powered search and analytics | Moderate | Moderate to complex | Custom | Financial analysts, researchers |
SEMrush | SEO-focused, keyword data | AI for SEO and content analysis | Moderate | High | Tiered subscriptions | Digital marketing and SEO teams |
Similarweb | 100M+ websites and apps | Traffic and engagement analytics | Moderate | High | Tiered subscriptions | Retail and digital performance benchmarking |
Klue | Aggregated competitor data | AI-driven insights | High, CRM integration | Moderate | Custom, enterprise | Sales enablement, competitive insight sharing |
SpyFu | SEO and PPC competitor data | Specialized AI for search trends | Limited | High | Flexible, affordable | Small to medium digital marketing teams |
Visualping | Website change monitoring | Simple AI for change detection | Low | Very high | Free and premium tiers | Lightweight monitoring for marketing/product teams |
Future Trends and Emerging Innovations in AI-Driven Competitive Intelligence
Future Trends and Emerging Innovations in AI-Driven Competitive Intelligence
Imagine a competitive intelligence (CI) system that not only reports yesterday’s data but anticipates tomorrow’s market shifts with dynamic, actionable insights. The AI-driven CI landscape is set for a profound transformation in 2025, propelled by key technological breakthroughs and strategic integrations. Yet, as these opportunities expand, so do the complexities involving ethics, privacy, and fairness.
Generative AI and Real-Time Data Fusion: From Static Reports to Living Insights
Generative AI has moved beyond concept to become an indispensable strategic asset for CI teams. No longer is intelligence gathering confined to manual sifting through reports and articles. Today’s generative AI models synthesize vast and disparate data streams, delivering contextual and nuanced insights tailored to specific business questions.
Platforms leveraging “Custom GPTs” are being fine-tuned for particular industries or competitive domains. They generate on-demand market trend summaries, competitor SWOT analyses, and scenario simulations, transforming AI from a passive data aggregator into an active “co-intelligence” partner that enhances human decision-making (The Hackett Group, 2025; Harvard Business Review, 2025).
At the same time, advances in real-time data fusion are dissolving information silos. These platforms integrate structured and unstructured data—from social media chatter to IoT sensor feeds and financial news—providing a comprehensive, timely view of the competitive landscape. The data fusion market is projected to grow at a compound annual growth rate (CAGR) exceeding 26% through 2032, driven by cloud adoption and the explosion of data volume and complexity (MarketResearchFuture, 2025).
This fusion capability is essential because CI in 2025 is not merely about collecting facts—it’s about connecting dots across multiple data types and sources to uncover hidden patterns or early warning signals. Andrew Lamb predicts 1,000 DataFusion-powered analytic systems will come online in 2025, signaling this technology’s shift from niche to mainstream (InfluxData, 2025).
Predictive Analytics and Automation: Anticipating Moves Before Competitors Do
Predictive analytics has matured into accessible tools embedded within CI workflows, empowering a broader range of business users. Next-generation predictive platforms utilize AI-driven forecasting to anticipate competitor pricing strategies, market disruptions, or shifts in customer sentiment.
Automation is evolving beyond mere delivery of predictions. AI CI tools now provide automated strategic recommendations—drafting competitive response plans, highlighting opportunities, and simulating outcomes of various tactics. This evolution bridges the gap between insight and action, enabling faster and more confident decision-making (TechTarget, 2025).
Consider generative AI assistants integrated into enterprise help desks or marketing platforms, which dramatically reduce task times—for example, cutting email drafting from one hour to ten minutes (IoT Analytics, 2025). Similar efficiencies are emerging in competitive intelligence, where AI handles routine monitoring and preliminary analyses, freeing human experts to focus on high-level strategy.
Integration with Enterprise Knowledge Graphs and Decision Intelligence Platforms
The full potential of AI-driven CI emerges through integration with broader AI ecosystems such as enterprise knowledge graphs (EKGs) and decision intelligence platforms (DIPs). EKGs provide a structured, semantic framework linking diverse organizational data—products, customers, competitors, regulations—creating a “single source of truth” for AI systems.
By grounding large language models (LLMs) in enterprise knowledge graphs, organizations can markedly enhance the factual accuracy, relevance, and explainability of AI-generated insights. This fusion transforms raw data into contextual intelligence, directly supporting complex decision-making processes (DaveAI, 2025; Hypermode, 2025).
Decision intelligence platforms build on this contextualized knowledge by enabling collaborative decision modeling, scenario testing, and outcome monitoring. Leading vendors like Cloverpop, Aera Technology, and Quantexa embed AI-driven optimization and automation into organizational workflows, facilitating smarter, data-informed decisions (Gartner, 2025).
Further integration with enterprise systems such as Salesforce, Slack, and CRM platforms—exemplified by tools like Klue and Crayon—enhances cross-functional collaboration. This ensures that competitive intelligence insights reach sales, marketing, product, and executive teams in real time, embedding intelligence across business functions (Improvado, 2025; Contify, 2025).
Ethical and Regulatory Challenges: Navigating a Complex Landscape
With increasing AI capabilities comes a heightened responsibility. AI CI tools processing vast volumes of data must contend with complex challenges related to data privacy, regulatory compliance, model transparency, and ethical use.
The 2025 privacy landscape is more intricate than ever. Cisco’s Data Privacy Benchmark Study reports that 86% of respondents support privacy legislation, with organizations recognizing that privacy investments often yield returns surpassing costs. Concurrently, regulations such as the EU AI Act, GDPR, and CCPA impose stringent requirements for data governance and AI accountability (Cisco, 2025; SC Media, 2025).
Explainability is a critical concern. Many advanced AI models, particularly deep learning architectures, operate as “black boxes,” complicating efforts to justify CI outputs to auditors or regulators. The field of Explainable AI (XAI) aims to develop techniques that make AI decisions transparent and interpretable—an indispensable foundation for trustworthy CI systems (Inbuilt.ai, 2025).
Ethical considerations extend beyond compliance. The deployment of AI in competitive intelligence can influence market dynamics and competitive fairness. There is a risk that firms with advanced AI CI capabilities may gain disproportionate advantages, potentially raising barriers to entry and concentrating market power.
Organizations must thus establish ethical frameworks emphasizing transparency, fairness, and respect for privacy. Early adopters embedding ethics into AI strategies find this approach to be a competitive differentiator—building customer trust and fostering long-term loyalty (Floqast, 2025; SecurePrivacy.ai, 2025).
How AI-Driven Competitive Intelligence Will Shape Market Dynamics
Looking forward, AI-driven competitive intelligence will transform not only internal decision-making but also broader market behaviors. Real-time, predictive, and automated insights will accelerate competitive responses, fueling faster innovation cycles and more dynamic pricing strategies.
However, these shifts may also intensify competitive pressures and market volatility. Firms that do not invest in cutting-edge AI CI risk falling behind, while questions of fairness and ethical boundaries will increasingly dominate regulatory discourse.
The future of AI in competitive intelligence hinges not just on technology, but on how organizations balance capability, responsibility, and strategic vision. Harnessing AI’s potential while safeguarding trust and fairness will be key to sustaining competitive advantage in an evolving marketplace.
Key Takeaways:
- Generative AI and real-time data fusion enable dynamic, context-rich, and predictive competitive insights.
- Integration with enterprise knowledge graphs and decision intelligence platforms amplifies AI CI’s impact by embedding insights into broader strategic workflows.
- Ethical AI use, regulatory compliance, and explainability are foundational to sustainable AI CI adoption.
- The rise of AI-driven CI will intensify competitive dynamics but also offers opportunities for fairer, more transparent market intelligence practices.
Navigating this evolving landscape requires more than technology adoption—it demands thoughtful stewardship, ensuring AI serves as a powerful tool to illuminate the competitive horizon rather than obscure it.
Trend / Innovation | Description | Impact / Notes | References / Projections |
---|---|---|---|
Generative AI and Real-Time Data Fusion | AI models synthesize diverse data streams to deliver contextual, nuanced insights; platforms use Custom GPTs tailored for industries; real-time fusion integrates structured and unstructured data. | Transforms AI from passive aggregator to active co-intelligence partner; dissolves information silos; uncovers hidden patterns and early warning signals. | Data fusion market CAGR >26% through 2032; 1,000 DataFusion-powered analytic systems expected by 2025 (MarketResearchFuture, 2025; InfluxData, 2025). |
Predictive Analytics and Automation | AI-driven forecasting tools embedded in CI workflows predict competitor strategies, market disruptions, and customer sentiment; automation provides strategic recommendations and simulates tactical outcomes. | Bridges insight and action; accelerates decision-making; reduces task times, e.g., email drafting down from 1 hour to 10 minutes. | Efficiency improvements in CI workflows; integration with generative AI assistants (TechTarget, 2025; IoT Analytics, 2025). |
Integration with Enterprise Knowledge Graphs (EKGs) and Decision Intelligence Platforms (DIPs) | EKGs link organizational data creating a single source of truth; LLMs grounded in EKGs enhance AI insight accuracy and explainability; DIPs enable collaborative decision modeling and automation. | Supports complex decisions; embeds AI-driven optimization into workflows; improves cross-functional collaboration via integration with Salesforce, Slack, CRM. | Vendors: Cloverpop, Aera Technology, Quantexa; Tools: Klue, Crayon (DaveAI, 2025; Hypermode, 2025; Gartner, 2025; Improvado, 2025; Contify, 2025). |
Ethical and Regulatory Challenges | AI CI tools face privacy, compliance, transparency, and ethical use hurdles; Explainable AI (XAI) addresses black-box model concerns; risk of unfair competitive advantages. | Necessitates ethical frameworks emphasizing transparency, fairness, privacy; ethical AI is a competitive differentiator fostering trust and loyalty. | 86% support privacy laws; regulations include EU AI Act, GDPR, CCPA (Cisco, 2025; SC Media, 2025; Floqast, 2025; SecurePrivacy.ai, 2025; Inbuilt.ai, 2025). |
Market Dynamics Shaped by AI-Driven CI | Real-time, predictive, automated insights accelerate responses, innovation, and pricing strategies; raises competitive pressures and market volatility. | Firms must balance AI capability with responsibility and ethics to sustain advantage; non-adopters risk falling behind. | Future success depends on stewardship and ethical use. |
Conclusion: Navigating Opportunities and Limitations of AI in Competitive Intelligence
Conclusion: Navigating Opportunities and Limitations of AI in Competitive Intelligence
What does the future hold for AI in competitive intelligence, and how should organizations approach this transformative yet complex technology? Rapid advancements—from real-time market monitoring to predictive analytics—are undeniably reshaping how businesses gather and interpret competitor data. However, the path forward requires a balanced approach that embraces AI’s transformative potential while acknowledging its inherent uncertainties and ethical complexities.
The Transformative Potential—and Its Limits
AI promises to revolutionize competitive intelligence by automating data collection and synthesis at an unprecedented scale. Tools like Crayon and Kompyte scan millions of data points across websites, social media, and industry reports, delivering alerts about market shifts and competitor activities in minutes rather than weeks. This agility is critical: McKinsey’s 2025 report estimates the AI opportunity at $4.4 trillion, with 92% of companies planning increased AI investments soon.
Yet, AI technology remains in its early stages of maturity. Only about 1% of organizations consider themselves “AI-mature,” revealing a significant gap between aspirations and effective execution. The 2025 United Nations Technology and Innovation Report highlights that infrastructure, data quality, and specialized skills are persistent bottlenecks. Without diverse, representative, and well-structured data, AI outputs risk being biased or incomplete. Even the most advanced AI models require continuous human interpretation and strategic judgment to convert insights into competitive advantage.
Ethical Considerations and Human Oversight
The power of AI comes with ethical responsibilities that competitive intelligence professionals must not overlook. Algorithmic bias, often rooted in skewed or incomplete datasets, remains a persistent threat. Harvard Business School stresses that systematically addressing these biases through diverse data inputs is not only ethical but also essential for reliable AI-generated intelligence.
Transparency and trust underpin effective AI deployment. Organizations need clear data governance structures, strong privacy safeguards, and open communication with stakeholders—especially when AI tools influence decisions about markets or competitors. According to the Boston Consulting Group, human oversight is far more than a compliance checkbox; it is a sophisticated, integrated process encompassing ongoing testing, evaluation, and escalation mechanisms designed to detect AI pitfalls early and maintain decision quality.
Strategic Alignment and Proactive Experimentation
Successful AI adoption in competitive intelligence requires more than simply deploying tools. It demands tight alignment of AI capabilities with organizational strategic goals. As emphasized by PwC and The Strategy Institute, businesses should regularly assess their AI maturity across adoption and development dimensions, investing in AI literacy to understand both the strengths and limitations of their systems.
Proactive experimentation, guided by clear objectives, is crucial. Rather than pursuing every emerging AI tool, companies should focus on use cases where AI augments human expertise—such as automating repetitive data gathering or surfacing early signals of competitor innovation. For instance, Microsoft’s integration of generative AI has demonstrated how thoughtfully applied AI can triple returns on investment by streamlining workflows and enhancing decision-making.
Looking Ahead: AI’s Evolving Role and Societal Impact
Moving beyond 2025, AI is expected to transition from a passive tool to a collaborative teammate. Gartner predicts that by 2028, autonomous agents will handle at least 15% of daily work decisions, indicating a shift toward AI systems that reason, learn, and adapt in real time. This evolution opens exciting possibilities for competitive intelligence—imagine AI agents not just reporting competitor moves but simulating market scenarios and recommending strategic actions.
However, this promising future also raises critical societal questions. The demand for vast computational resources challenges sustainability goals, while ethical frameworks and governance models must evolve alongside AI’s expanding influence. Organizations face a clear choice: lead with trust, invest in human-AI collaboration, and embed ethical safeguards to ensure AI advances equitable and transparent business outcomes.
Key Takeaways for Practitioners
- Adopt AI tools with a critical eye: Evaluate vendors based on data quality, multi-channel monitoring capabilities, and strategic alignment.
- Invest in AI literacy and skills development: Equip teams to understand, prompt, and validate AI outputs effectively.
- Maintain robust human oversight: Implement integrated review processes to detect biases, inaccuracies, and scope drift before decisions are made.
- Prioritize ethical governance: Commit to transparency, data privacy, and bias mitigation as foundational principles.
- Experiment with clear objectives: Use AI to augment—not replace—human intuition and contextual knowledge.
- Stay adaptable: The AI landscape will evolve rapidly; flexibility and continuous learning are your best defenses against hype and obsolescence.
In summary, AI in competitive intelligence offers a powerful lens to decode your market and rivals—but it is neither a crystal ball nor a set-and-forget solution. By blending technological rigor with ethical mindfulness and strategic clarity, organizations can harness AI not only to outpace competition but also to foster a more informed, equitable business ecosystem.
Aspect | Details |
---|---|
Transformative Potential | Automates large-scale data collection and synthesis; tools like Crayon and Kompyte enable real-time monitoring; AI opportunity valued at $4.4 trillion by 2025; only 1% of organizations AI-mature; requires high-quality diverse data and human interpretation. |
Ethical Considerations | Risks of algorithmic bias; importance of diverse datasets; emphasis on transparency, data governance, privacy; human oversight as ongoing process for quality assurance; ethical use essential for reliability. |
Strategic Alignment | Align AI capabilities with organizational goals; assess AI maturity; invest in AI literacy; focus on augmenting human expertise; example: Microsoft’s generative AI tripling ROI by enhancing workflows. |
Future Outlook | Shift towards AI as collaborative teammate by 2028; autonomous agents handling 15% of daily decisions; potential for AI to simulate market scenarios and recommend strategies; challenges include sustainability and evolving governance frameworks. |
Key Practitioner Takeaways | Critical evaluation of AI tools; invest in skills development; maintain human oversight; prioritize ethical governance; experiment with clear objectives; remain adaptable to rapid AI evolution. |
Summary | AI is a powerful yet complex tool for competitive intelligence, requiring balanced integration of technology, ethics, and strategy to outpace rivals and foster equitable business practices. |