AI in Telecom: Mastering Network Management with Machine Learning
- Introduction: Why AI and Machine Learning are Game Changers for Telecom Networks
- Introduction: Why AI and Machine Learning are Game Changers for Telecom Networks
- The Growing Complexity and Data Volumes Driving AI/ML Adoption
- The Convergence of 5G, IoT, and Cloud-Native Architectures
- How Machine Learning Optimizes Network Operations, Resource Management, and Customer Experience
- Foundations of Machine Learning in Telecommunications: Key Concepts and Techniques
- Foundations of Machine Learning in Telecommunications: Key Concepts and Techniques
- Core Machine Learning Paradigms in Telecom: Supervised, Unsupervised, and Reinforcement Learning
- Essential Data Sources Feeding Telecom ML Models
- Feature Engineering Challenges and Model Training Considerations in Telecom
- AI-Driven Network Management: From Automation to Predictive Optimization
- AI-Driven Network Management: From Automation to Predictive Optimization
- Machine Learning Transforming Network Planning and Real-Time Traffic Management
- Fault Detection and Predictive Maintenance: From Reactive to Proactive
- Algorithms Powering Anomaly Detection and Capacity Forecasting
- Integrating Machine Learning with SDN/NFV for Automated Network Control
- Balancing Innovation with Operational Realities
- Looking Ahead
- Enhancing Customer Experience and Operational Efficiency with AI
- Enhancing Customer Experience and Operational Efficiency with AI
- Personalization through Customer Segmentation and Churn Prediction
- AI-Powered Customer Support: Chatbots, Virtual Assistants, and Sentiment Analysis
- Driving Operational Efficiency: Call Center Optimization, Fraud Detection, and Revenue Assurance
- Ethical Considerations: Privacy, Bias, and Trust
- Benchmarking AI Solutions in Telecom: Performance Metrics and Comparative Analyses
- Benchmarking AI Solutions in Telecom: Performance Metrics and Comparative Analyses
- Key Performance Indicators for Telecom ML Models
- Comparing Machine Learning Frameworks and AI Platforms in Telecom
- Scalability Challenges and Cost-Benefit Considerations
- Final Thoughts
- Challenges, Risks, and Responsible AI Adoption in Telecom Networks
- Challenges, Risks, and Responsible AI Adoption in Telecom Networks
- Technical Challenges: Data Quality, Model Interpretability, and Dynamic Environments
- Cybersecurity Risks and Adversarial Threats
- Responsible AI Adoption: Governance, Transparency, and Trust
- Future Outlook: AI, 6G, and the Evolution of Intelligent Telecom Networks
- Future Outlook: AI, 6G, and the Evolution of Intelligent Telecom Networks
- AI as the Backbone of 6G: Ubiquitous Connectivity and Autonomous Network Slicing
- Frontier AI Models: Large Language Models and Agentic AI in Telecom Automation and Customer Experience
- Emerging Trends: Quantum Machine Learning, Edge AI, and Societal Implications
- Key Takeaways

Introduction: Why AI and Machine Learning are Game Changers for Telecom Networks
Introduction: Why AI and Machine Learning are Game Changers for Telecom Networks
Telecommunications networks today confront an unprecedented challenge: managing an exploding volume of data alongside a vast and intricate web of devices, all while delivering seamless, reliable service. By 2024, global data creation is projected to reach 149 zettabytes, soaring to over 394 zettabytes by 2028. To contextualize, a zettabyte equals one trillion gigabytes — a scale that outstrips traditional network management approaches. Layer in the rapid adoption of 5G, the proliferation of billions of IoT devices, and the shift toward cloud-native network architectures, and we see a perfect storm of complexity demanding smarter, more adaptive solutions.
The Growing Complexity and Data Volumes Driving AI/ML Adoption
Why is AI no longer optional for telecom operators? The answer lies in the sheer complexity and scale of modern networks, which have outpaced manual and rule-based management strategies. The telecommunications industry, poised to generate revenues exceeding $1.53 trillion in 2024, increasingly depends on AI to remain competitive and profitable.
The midpoint of the 5G rollout in 2024 represents a critical inflection. With 6G anticipated around 2030, networks must evolve to be not only faster but also more efficient and sustainable. For example, 6G aims to reduce energy consumption per gigabyte by about 90% — a goal that traditional management tools cannot reliably achieve without AI-driven optimization.
Market signals underscore this urgency: over 15 global telecom companies have announced plans to build their own generative AI data centers, signaling a shift toward AI-first infrastructure. Additionally, mergers and acquisitions in data centers have doubled from 2019 to 2022, reflecting strategic moves to harness AI capabilities at scale.
This vast data landscape, coupled with escalating customer expectations, compels operators to rethink foundational infrastructure. Automating operations reduces costs and enhances service agility. The combined operations support systems (OSS) and business support systems (BSS) market alone is projected to reach $70 billion by 2025, driven largely by AI integration.
The Convergence of 5G, IoT, and Cloud-Native Architectures
How do 5G, IoT, and cloud-native models accelerate AI adoption in telecom? Their synergy intensifies network complexity and capability.
5G delivers more than faster speeds; it enables ultra-reliable, low-latency connections essential for critical enterprise applications across manufacturing, transportation, and energy sectors. Managing such diverse, dynamic use cases requires networks capable of self-optimization and real-time decision-making.
AI-powered self-organizing networks (SONs) capitalize on machine learning to dynamically adjust network parameters based on traffic patterns, device types, and environmental factors. This real-time adaptability is crucial to support the explosive growth of IoT — with forecasts exceeding 75 billion connected devices by 2025 and surpassing 29 billion by 2030.
Cloud-native architectures complement this by enabling scalable, flexible deployment of network functions through virtualization and containerization. This approach allows operators to deploy AI-driven analytics and automation closer to the network edge, reducing latency and improving responsiveness.
The race to integrate AI with 5G and cloud architectures is intensifying. Telecom providers are embracing AI not only for network optimization but also to unlock new revenue streams by offering personalized, hyper-localized services. For instance, AI-driven predictive analytics can anticipate network congestion or hardware failures, enabling preemptive actions that minimize downtime and enhance customer satisfaction.
How Machine Learning Optimizes Network Operations, Resource Management, and Customer Experience
What tangible benefits does machine learning deliver in telecom networks today? The impact spans multiple domains:
-
Network Operations: AI-powered predictive analytics identify potential outages and bottlenecks before they affect service. Operators like Vodafone use AI to automate fault detection and resolution, minimizing human intervention and accelerating recovery times. This capability is vital, as telecom experiences some of the fastest-growing outage rates among technology sectors.
-
Resource Management: Machine learning models optimize spectrum allocation, energy consumption, and traffic routing. AI algorithms contribute to reducing carbon footprints by enhancing energy efficiency in network operations — a growing priority as telecoms pursue sustainability goals aligned with 6G.
-
Customer Experience: AI-driven personalization tailors offers and services to individual subscribers, significantly reducing churn. Generative AI-powered virtual assistants and chatbots handle customer queries across multiple channels with speed and accuracy, elevating service levels while lowering operational costs.
Despite these promising advances, it is crucial to maintain a balanced perspective. AI in telecom is not a silver bullet. Data quality issues, integration challenges, workforce readiness, and ethical considerations such as AI transparency and security remain significant hurdles. For example, nearly 57% of telecom companies express concern over security attacks targeting physical assets, highlighting the need for robust, AI-driven security frameworks.
Moreover, while AI automates many tasks, human expertise remains essential to interpret insights, govern AI models responsibly, and guide strategic decisions. Operators must invest in building AI literacy among employees and adopt transparent governance structures to foster trust among customers and regulators.
In summary, the convergence of exploding data volumes, the expansion of 5G and IoT, and the adoption of cloud-native architectures have propelled AI and machine learning from promising tools to essential pillars of modern telecom networks. Their ability to optimize complex operations, manage scarce resources efficiently, and enhance customer experiences is transforming how networks are built and operated. Yet, this transformation demands a cautious, evidence-based approach that acknowledges AI’s limitations and underscores the critical human role in steering its deployment.
As this article explores further, understanding both the capabilities and constraints of AI in telecom will be key for operators seeking to harness its full potential without succumbing to hype or unintended risks.
Aspect | Details |
---|---|
Global Data Creation (2024) | 149 zettabytes |
Global Data Creation (2028 Forecast) | 394+ zettabytes |
Telecom Industry Revenue (2024) | $1.53 trillion |
5G Rollout Midpoint | 2024 |
6G Anticipated | Around 2030 |
6G Energy Consumption Reduction Goal | ~90% per gigabyte |
Global Telecom Companies Planning AI Data Centers | 15+ |
Data Center Mergers & Acquisitions Growth | Doubled from 2019 to 2022 |
OSS/BSS Market Projection (2025) | $70 billion |
IoT Connected Devices Forecast (2025) | 75 billion+ |
IoT Connected Devices Forecast (2030) | 29 billion+ |
Telecom Security Concern (Physical Asset Attacks) | 57% of companies |
Foundations of Machine Learning in Telecommunications: Key Concepts and Techniques
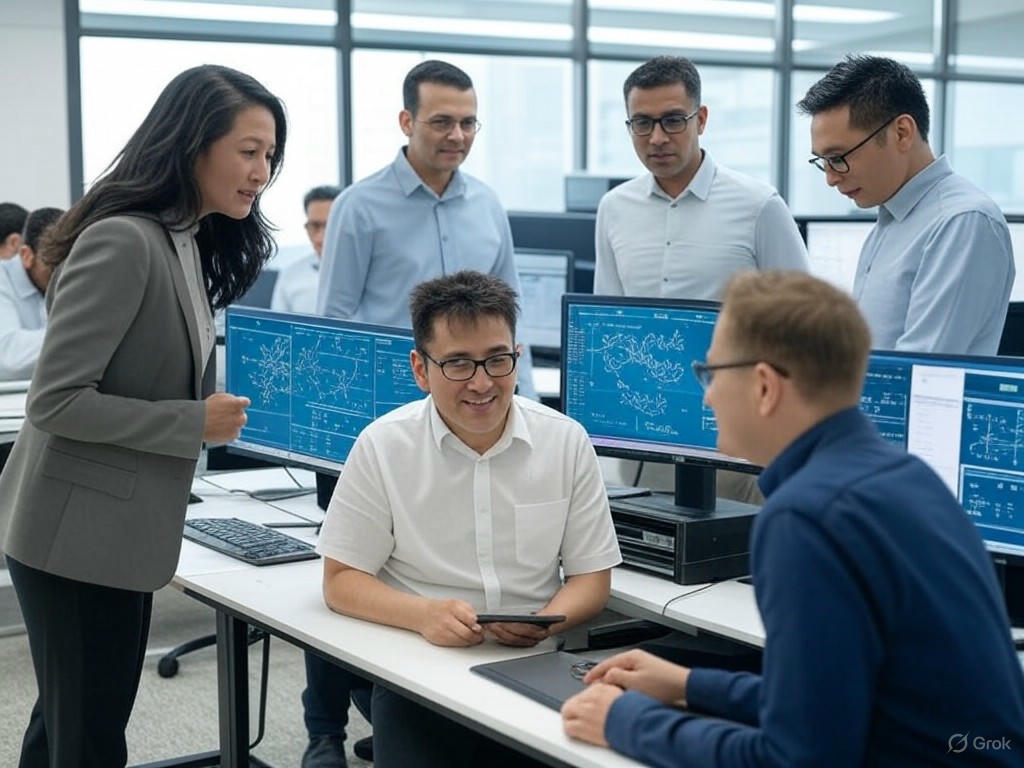
Foundations of Machine Learning in Telecommunications: Key Concepts and Techniques
What does machine learning (ML) truly mean for telecommunications beyond the buzzwords? At its core, ML in telecom leverages vast streams of data—from network activity to customer behavior—to automate decisions, optimize operations, and detect anomalies faster than any human team could manage. To grasp how these systems function, we must unpack the foundational ML paradigms and the unique characteristics of telecom data that shape modeling strategies.
Core Machine Learning Paradigms in Telecom: Supervised, Unsupervised, and Reinforcement Learning
Telecom networks provide a rich environment for all three major ML approaches, each tailored to distinct operational challenges.
-
Supervised Learning: This approach relies on labeled data to train models for prediction or classification tasks. For example, fraud detection in telecom uses extensive historical datasets tagging fraudulent versus legitimate traffic. Models trained on such data enable real-time flagging of suspicious activity, helping reduce global fraud losses, which amounted to nearly $39 billion in 2023. Another vital application is customer churn prediction, where models identify subscribers likely to leave, allowing operators to launch proactive retention campaigns.
-
Unsupervised Learning: When labeled data is scarce or unavailable, unsupervised learning explores data structure to uncover patterns or anomalies. Telecom generates massive unlabeled datasets—from network telemetry to call logs. Clustering and anomaly detection techniques can identify unusual network behaviors indicating faults or cyberattacks. For instance, self-organizing networks (SONs) employ unsupervised methods to dynamically adjust configuration parameters based on real-time conditions, improving network efficiency with minimal human intervention.
-
Reinforcement Learning (RL): At the frontier of telecom ML, RL enables agents to learn optimal policies through trial and error, guided by reward signals. Imagine AI “players” interacting with the network environment to optimize resource allocation, power consumption, or routing decisions dynamically. Deep RL algorithms are increasingly deployed in managing complex 5G and emerging 6G networks, fostering adaptive infrastructure that responds to fluctuating demand and evolving user needs with minimal manual tuning.
Additionally, semi-supervised learning—a hybrid combining limited labeled data with abundant unlabeled data—is gaining traction. This approach balances efficiency and accuracy, particularly important in telecom where labeling is costly and time-consuming.
Essential Data Sources Feeding Telecom ML Models
Machine learning’s effectiveness hinges on data quality and diversity. In telecom, the data ecosystem is uniquely complex and varied. Understanding these sources is crucial for designing accurate and efficient models.
-
Network Telemetry: Telemetry acts as the nervous system of telecom networks. Devices emit continuous real-time metrics such as flow statistics, packet headers, device health indicators, and signal quality. Standardized protocols like NetFlow, IPFIX, and sFlow facilitate this data collection, providing the granular visibility needed for predictive maintenance, fault localization, and capacity planning. Industry leaders like Cisco emphasize telemetry’s critical role in network observability.
-
Customer Usage Patterns: Beyond technical data, operators analyze how customers engage with services—call durations, data consumption, app usage, and mobility patterns. Such behavioral data feeds ML models for churn forecasting, personalized offers, and network provisioning optimization. Verizon’s AI-driven customer service, for example, leverages these insights to reduce response times by 61%, handling significantly more inquiries without increasing workforce size.
-
Service Logs and Event Data: These include system events, error reports, and configuration changes across network elements. Mining these logs with ML helps identify root causes of outages, predict failures before escalation, and automate troubleshooting workflows. The surge of 5G and IoT devices has led to exponential growth in service logs, necessitating scalable and efficient processing architectures.
Collecting, integrating, and cleansing these heterogeneous datasets is non-trivial. Telecom data is often noisy, incomplete, and inconsistently formatted, requiring sophisticated preprocessing pipelines to ensure model reliability.
Feature Engineering Challenges and Model Training Considerations in Telecom
If data is the raw material, feature engineering is the craftsman’s workshop where signals become actionable insights. Telecom data presents distinctive challenges in this regard:
-
Handling Massive Scale and Velocity: Operators manage millions of devices generating continuous streams of high-dimensional data. Feature creation must capture meaningful patterns without overburdening computational resources. This balance requires careful aggregation, sampling, and dimensionality reduction techniques to maintain efficiency.
-
Addressing Data Quality and Heterogeneity: Noise, missing values, and variable signal quality are common in telecom datasets. Feature engineering must incorporate robust imputation methods and normalization strategies to ensure model resilience across different network conditions and geographies.
-
Latency Constraints: Many telecom applications demand near real-time inference. Tasks like adaptive beamforming or dynamic spectrum allocation require feature extraction pipelines optimized for low latency, sometimes leveraging edge computing to preprocess data closer to the source.
-
Domain-Specific Feature Design: Features must reflect telecom-specific knowledge. Examples include aggregating packet loss rates over sliding windows, encoding mobility patterns as spatiotemporal vectors, or quantifying user Quality of Experience (QoE) through composite metrics. SoftBank’s pioneering work on Large Telecom Models (LTMs)—large language models trained on telecom-specific data—demonstrates how tailored feature sets can unlock superior inference capabilities.
Model training at telecom scale also demands distributed computing frameworks and incremental learning approaches to accommodate continuous data inflows and dynamic network environments. Cloud platforms like Google Cloud’s Vertex AI provide scalable infrastructure and integrated tools for model management and deployment, supporting these complex workflows.
In summary, machine learning in telecommunications is a nuanced, multi-faceted endeavor. It combines supervised, unsupervised, and reinforcement learning to tackle diverse challenges, powered by a rich tapestry of telemetry, customer, and service data. The transformative potential lies in converting this data into meaningful features and deploying models that meet stringent performance and latency requirements. As AI platforms, edge computing, and domain-specific models advance, the telecom future promises networks that are not only faster but smarter, more resilient, and deeply aligned with user needs.
Category | Details | Examples / Notes |
---|---|---|
Core ML Paradigms | Supervised Learning | Fraud detection using labeled data; Customer churn prediction; Real-time suspicious activity flagging; $39B global fraud losses in 2023 |
Unsupervised Learning | Clustering and anomaly detection on unlabeled data; Self-organizing networks (SONs) for dynamic configuration; Detecting faults and cyberattacks | |
Reinforcement Learning | Dynamic resource allocation; Optimizing power consumption and routing; Managing 5G/6G networks; Adaptive infrastructure with minimal manual tuning | |
Semi-Supervised Learning | Combines limited labeled and abundant unlabeled data; Balances accuracy and efficiency; Useful when labeling is costly | |
Essential Data Sources | Network Telemetry | Real-time metrics (flow stats, packet headers, device health, signal quality); Protocols: NetFlow, IPFIX, sFlow; Used for predictive maintenance, fault localization, capacity planning |
Customer Usage Patterns | Call durations, data consumption, app usage, mobility; Used for churn forecasting, personalized offers, network provisioning; Example: Verizon reduces response times by 61% | |
Service Logs and Event Data | System events, error reports, configuration changes; Used for root cause analysis, failure prediction, troubleshooting automation; Growth driven by 5G and IoT devices | |
Feature Engineering Challenges | Scale and Velocity | Millions of devices streaming high-dimensional data; Requires aggregation, sampling, dimensionality reduction |
Data Quality and Heterogeneity | Noise, missing values, variable signal quality; Needs imputation and normalization for model resilience | |
Latency Constraints | Near real-time inference needed for adaptive beamforming, spectrum allocation; Edge computing used for preprocessing | |
Domain-Specific Feature Design | Packet loss rates over sliding windows; Mobility as spatiotemporal vectors; QoE composite metrics; SoftBank’s Large Telecom Models (LTMs) | |
Model Training Considerations | Distributed Computing & Incremental Learning | Handles continuous data inflows and dynamic environments; Platforms like Google Cloud Vertex AI support scalable model management and deployment |
AI-Driven Network Management: From Automation to Predictive Optimization
AI-Driven Network Management: From Automation to Predictive Optimization
Imagine a telecom network that not only detects faults but autonomously resolves them before users even experience any disruption. This capability, once the realm of science fiction, is rapidly becoming a reality through the deep integration of machine learning (ML) into network management. The surge in data traffic from 5G deployment and the proliferation of IoT devices compel telecom operators to move beyond reactive approaches toward predictive and automated strategies.
Machine Learning Transforming Network Planning and Real-Time Traffic Management
Traditional network planning often resembles steering a ship through dense fog—decisions are largely based on historical data and static assumptions, which limits agility and operational efficiency. Machine learning helps lift this fog by enabling the creation of Network Digital Twins, virtual replicas that simulate complex, dynamic network environments in real time. These digital counterparts allow operators to experiment with various configurations, optimizing resource allocation and capacity ahead of demand spikes.
Predictive analytics models forecast traffic surges, facilitating proactive capacity adjustments that prevent congestion. This dynamic approach reduces costly over-provisioning and enhances investment precision. For instance, Flycomm forecasts that by 2025, AI-driven network planning will dominate mobile network management, propelled by automation and SaaS platforms that streamline decision-making.
In real-time traffic management, AI continuously ingests and analyzes streaming telemetry data to balance network loads, reroute packets, and enforce quality of service (QoS) protocols. These systems dynamically adapt to traffic fluctuations caused by events such as live sports broadcasts or emergency situations, ensuring consistent and seamless connectivity.
Fault Detection and Predictive Maintenance: From Reactive to Proactive
Historically, fault detection in telecom networks has been reactive—issues are addressed only after service degradation occurs. AI is reversing this trend by enabling early anomaly detection and predictive maintenance, significantly reducing downtime.
Advanced ML algorithms analyze vast amounts of telemetry and NetFlow data to identify subtle patterns indicative of impending faults. Deep learning models, including Recurrent Neural Networks (RNNs) and Convolutional Neural Networks (CNNs), are particularly effective at detecting previously unseen anomalies by learning complex statistical signatures of normal network behavior.
The benefits are substantial: predictive maintenance can reduce unexpected downtime by up to 40%, saving telecom providers approximately $13,000 per minute in avoided losses. AI agents now continuously monitor network health, automatically isolating faults and triggering incident management workflows with minimal human intervention.
A concrete example is the rise of self-healing networks. These systems leverage AI to detect failures and execute autonomous recovery actions, such as traffic rerouting or resource reallocation. Public sector agencies employing self-healing architectures report enhanced resilience to cyberattacks and natural disasters, often restoring connectivity within seconds.
Algorithms Powering Anomaly Detection and Capacity Forecasting
At the core of AI-driven network management are sophisticated algorithms tailored for network anomaly detection and capacity forecasting. Conventional machine learning techniques like decision trees and support vector machines (SVMs) offer simplicity but may struggle with the high-dimensional and complex data typical in telecom environments.
Deep learning models, especially RNNs and CNNs, are increasingly preferred due to their ability to model temporal sequences and spatial relationships within network traffic data. These models detect subtle deviations from baseline patterns, enabling early identification of security threats or performance degradations.
Capacity forecasting leverages time series analysis and regression models enhanced by machine learning to predict future network loads based on historical usage, emerging trends, and external factors such as new user deployments or viral content. This foresight allows operators to efficiently scale infrastructure, balancing cost and performance.
Integrating Machine Learning with SDN/NFV for Automated Network Control
The full potential of AI in telecommunications is realized when combined with Software Defined Networking (SDN) and Network Functions Virtualization (NFV)—programmable network architectures that provide the agility and granularity required for AI-driven automation.
ML models feed actionable insights directly into SDN controllers, which dynamically adjust routing, bandwidth allocation, and security policies via APIs. This closed-loop automation transforms networks into adaptive ecosystems capable of self-optimization and self-healing.
One notable application is energy-efficient Radio Access Network (RAN) management, where AI modulates power consumption based on real-time traffic demands. This approach drastically reduces the carbon footprint of mobile networks without compromising user experience. For example, Vodafone UK and Ericsson conducted trials demonstrating AI-driven enhancements in 5G energy efficiency, marking crucial steps toward sustainable telecom infrastructure.
The synergy between AI and SDN/NFV is also evident in self-healing networks. By continuously monitoring network state, AI anticipates faults, isolates affected segments, and triggers autonomous reconfigurations, reducing operational overhead and improving uptime—critical for mission-critical communication services.
Balancing Innovation with Operational Realities
While AI holds immense promise, integrating it into telecom’s SDN/NFV infrastructure is not without challenges. Operators must rethink workflows and invest in workforce training, as successful deployment requires a fusion of telecom domain expertise and cloud-native development skills. This transformation demands both technological upgrades and cultural shifts.
Data quality remains a fundamental concern. AI systems require rich, accurate, and well-labeled telemetry to function effectively. Incomplete or noisy data can lead to false positives or missed anomalies, undermining trust in automated systems.
Looking Ahead
By 2025, AI-powered network management will become foundational rather than optional. Operators that embrace ML-driven planning, fault detection, and automated control will unlock unprecedented gains in efficiency, reliability, and sustainability.
However, automation must be paired with transparency and human oversight. AI is not a replacement for human expertise but an augmentation—providing tools that empower smarter, more timely decisions. Ethical AI practices, including fairness, accountability, and robust security frameworks, will be as essential as the underlying algorithms.
In the evolving telecom landscape, AI acts as the nervous system that enables networks to think, adapt, and heal in real time. Those mastering this transformation will lead the next wave of connectivity innovation.
Aspect | Description | Examples / Benefits |
---|---|---|
Network Digital Twins | Virtual replicas simulating real-time dynamic network environments for planning and optimization. | Optimize resource allocation and capacity ahead of demand spikes. |
Predictive Analytics | Forecast traffic surges to enable proactive capacity adjustments and prevent congestion. | Reduces over-provisioning; Flycomm predicts dominance by 2025. |
Real-Time Traffic Management | AI analyzes streaming telemetry data to balance loads, reroute packets, and enforce QoS protocols. | Adapts to events like live broadcasts or emergencies for seamless connectivity. |
Fault Detection & Predictive Maintenance | ML algorithms detect anomalies early and predict faults to reduce downtime. | Up to 40% reduction in downtime; saves ~$13,000 per minute in losses. |
Deep Learning Models | RNNs and CNNs identify complex anomalies by learning statistical signatures of normal network behavior. | Detect previously unseen faults and security threats. |
Self-Healing Networks | AI-driven systems autonomously detect failures and execute recovery actions. | Enhanced resilience; restores connectivity within seconds in public sector. |
Anomaly Detection Algorithms | Decision trees, SVMs, and increasingly deep learning models for detecting deviations and threats. | Improved detection of security and performance issues. |
Capacity Forecasting | Time series analysis and regression enhanced by ML predict network load and trends. | Efficient infrastructure scaling balancing cost and performance. |
Integration with SDN/NFV | ML insights fed into programmable network architectures for automated control and optimization. | Dynamic routing, bandwidth, security adjustments; energy-efficient RAN management. |
Energy-Efficient RAN Management | AI modulates power consumption based on real-time demand. | Reduces carbon footprint; Vodafone UK & Ericsson 5G trials. |
Operational Challenges | Need for workflow changes, workforce training, and data quality assurance. | Requires telecom and cloud-native expertise fusion; critical for trust in AI systems. |
Future Outlook | AI will be foundational by 2025, augmenting human expertise with transparency and ethics. | Enables smarter decisions, network adaptability, and sustainability. |
Enhancing Customer Experience and Operational Efficiency with AI
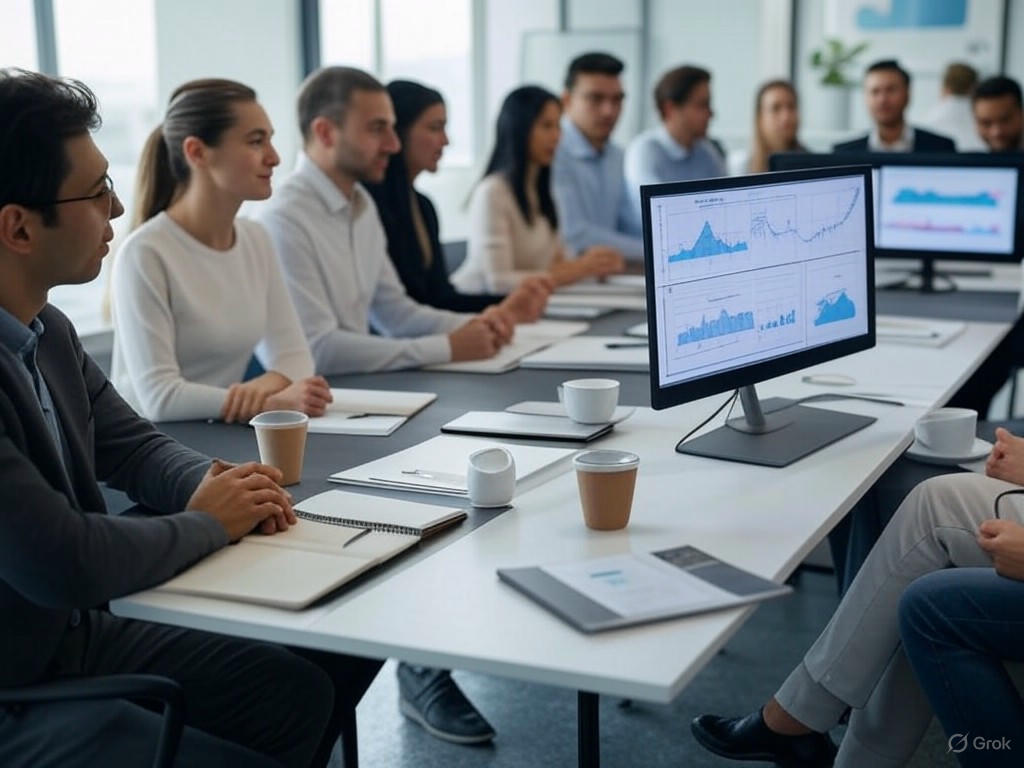
Enhancing Customer Experience and Operational Efficiency with AI
How can telecommunications companies transform vast amounts of customer data into proactive, personalized service? The key lies in machine learning-driven customer segmentation and churn prediction models that enable hyper-personalization—anticipating user needs before they even arise.
Personalization through Customer Segmentation and Churn Prediction
Telecom operators possess some of the richest datasets in the digital economy, including call records, usage patterns, device types, and location data. The challenge is to extract actionable insights that inform customer segmentation and identify subscribers at risk of churn.
For instance, Onix’s churn prediction models analyze diverse data points to flag customers likely to leave, enabling timely interventions to retain them. Research presented at recent IEEE conferences confirms that optimizing segmentation and churn prediction directly enhances customer retention and operational efficiency.
Segmentation is evolving beyond static demographic categories. Leading firms like Rapid Innovation employ AI agents that dynamically analyze behavioral patterns, yielding granular, actionable insights. This dynamic approach aligns with the shift toward hyper-personalization, where AI anticipates customer desires and engages them via the optimal channel—whether with a special offer or a network upgrade notification. Insider’s analysis underscores that hyper-personalization is no longer optional but essential for competitiveness.
The efficacy of these AI models depends heavily on integrating real-time and external data sources, which significantly improves predictive accuracy. However, this raises ethical concerns around data privacy and transparency. Telecoms must balance the benefits of personalization with maintaining customer trust, ensuring AI-driven segmentation respects privacy norms and avoids perpetuating biases embedded in historical data.
AI-Powered Customer Support: Chatbots, Virtual Assistants, and Sentiment Analysis
Long wait times and repetitive customer service interactions have driven telecoms to adopt AI-powered chatbots and virtual assistants as frontline support solutions. The global market for generative AI chatbots in telecom is projected to grow from $302 million in 2024 to over $428 million in 2025, with forecasts nearing $10 billion by 2034. This growth reflects business urgency, as 46% of telecom customers switch providers following poor service experiences.
AI chatbots effectively manage routine inquiries, freeing human agents to address complex issues. T-Mobile’s deployment of AI-powered virtual agents has notably improved response times and customer satisfaction. These chatbots operate 24/7 across websites, apps, and social platforms, offering multilingual support, although language proficiency gaps remain a challenge.
Beyond scripted Q&A, sentiment analysis tools enhance support by detecting caller emotions—such as frustration or happiness—through voice and text cues. These real-time insights enable call centers to adjust responses empathetically, boosting satisfaction. CallMiner’s AI Assist exemplifies this trend by combining natural language processing with real-time analytics to optimize agent-customer interactions.
Integrating AI-driven support does present challenges. Legacy systems and data security concerns complicate deployment. Telecoms must design AI workflows carefully to ensure seamless handoffs between bots and human agents, preserving service quality and customer trust.
Driving Operational Efficiency: Call Center Optimization, Fraud Detection, and Revenue Assurance
Machine learning is revolutionizing operational aspects of telecom, transforming call centers from cost centers into strategic assets through AI automation. According to CallMiner, AI can increase individual agent productivity by 30–45% by automating repetitive tasks, delivering real-time insights, and adding empathetic interactions via agentic AI systems—AI designed to act autonomously in customer engagements.
Fraud detection is another critical domain where AI excels. The telecom fraud landscape is increasingly sophisticated, with threats such as AI-generated deepfake audio and SIM closure fraud. Platforms like DataDome employ real-time machine learning at the network edge to detect and block fraudulent activities within milliseconds. This rapid response is vital as fraudsters adapt quickly, circumventing traditional methods like SMS one-time passwords.
Revenue assurance benefits from AI’s capacity to analyze vast data streams, identifying leakage points and anomalies. Automating these processes reduces financial losses and improves compliance. A McKinsey report highlights that leading telecoms are building centralized AI platforms to scale such efficiencies while maintaining governance and control.
Ethical Considerations: Privacy, Bias, and Trust
These AI advances come with significant ethical complexities. Telecom operators wield immense power through their access to sensitive data, making privacy a paramount concern. The World Economic Forum and Accenture emphasize the necessity of transparent data governance frameworks to sustain customer trust.
Algorithmic bias remains a pressing risk. If training datasets are skewed—reflecting historical inequities or underrepresenting minority groups—AI systems may perpetuate unfair treatment. Ethical AI practices require diverse datasets, rigorous bias testing, and continuous model audits.
Moreover, AI-driven customer interactions must avoid misinformation and inappropriate responses. Surveys indicate that 43% of users fear AI could propagate falsehoods, while 41% worry about biased outputs. Designing AI systems with fairness, accountability, and transparency at their core is not only an ethical imperative but essential for maintaining customer loyalty and regulatory compliance.
In conclusion, machine learning is reshaping telecommunications by enabling hyper-personalized customer experiences, smarter support systems, and enhanced operational efficiency. Yet, realizing this potential depends on responsible deployment that respects privacy, mitigates bias, and builds trust. Telecoms that effectively balance innovation with ethical governance will improve their bottom line and solidify their role as foundational infrastructure in the AI-powered economy.
Category | AI Application | Details | Examples / Companies | Challenges / Considerations |
---|---|---|---|---|
Customer Experience | Customer Segmentation & Churn Prediction | Machine learning models analyze rich telecom datasets to identify at-risk customers and enable hyper-personalization. | Onix, Rapid Innovation | Data privacy, ethical use of data, avoiding bias in segmentation |
AI-Powered Customer Support | Chatbots and virtual assistants handle routine queries; sentiment analysis detects caller emotions for better responses. | T-Mobile, CallMiner | Language proficiency gaps, seamless bot-human handoff, data security | |
Hyper-Personalization | Dynamic behavioral analysis for personalized offers and engagement via optimal channels. | Insider (analysis) | Balancing personalization with customer trust and privacy | |
Operational Efficiency | Call Center Optimization | AI automates repetitive tasks and provides real-time insights to boost agent productivity by 30-45%. | CallMiner | Integration with legacy systems, maintaining service quality |
Fraud Detection | Real-time machine learning detects and blocks fraud such as deepfake audio and SIM closure attempts. | DataDome | Rapidly evolving threats require constant model updates | |
Revenue Assurance | AI analyzes data streams to identify revenue leakage and anomalies, improving compliance and reducing losses. | Leading telecom firms (per McKinsey) | Governance and control of centralized AI platforms | |
Ethical Considerations | Privacy, Bias, and Trust | Transparent data governance, bias mitigation, fair and accountable AI to maintain trust and comply with regulations. | World Economic Forum, Accenture | Algorithmic bias, misinformation, customer trust, regulatory compliance |
Benchmarking AI Solutions in Telecom: Performance Metrics and Comparative Analyses
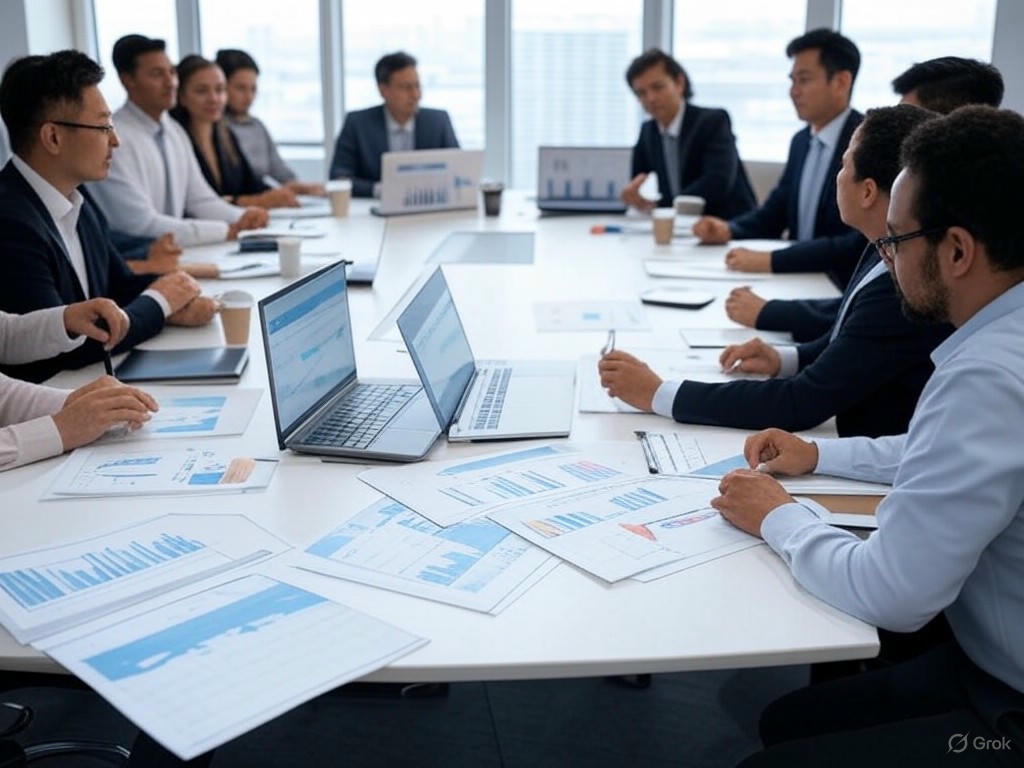
Benchmarking AI Solutions in Telecom: Performance Metrics and Comparative Analyses
When telecom operators deploy machine learning (ML) models to manage increasingly complex networks, rigorous benchmarking is essential to verify that these models deliver real-world value. Such evaluation moves beyond simple accuracy metrics, encompassing latency, robustness, scalability, and more, to ensure dependable and efficient operation within dynamic telecom environments.
Key Performance Indicators for Telecom ML Models
While accuracy often headlines ML project results, telecommunications demands a broader set of key performance indicators (KPIs) tailored to its unique operational challenges:
-
Accuracy and Recall: In customer churn prediction—a quintessential telecom use case—models demonstrating 85% accuracy in controlled testing environments can see production accuracy drop to around 70%. Addressing this requires sophisticated validation strategies such as stratified k-fold cross-validation and techniques like SMOTE to handle class imbalance. These methods have proven effective in raising production recall to 79%, underscoring that precision and recall aligned with business goals often better reflect operational impact than accuracy alone.
-
Latency: Real-time network management and customer-facing applications like personalized recommendations or conversational interfaces place stringent requirements on inference latency. Large language models (LLMs) and foundational generative AI introduce latency bottlenecks that challenge traditional cloud-only deployments. To combat this, telecom infrastructure innovations emphasize on-premise and edge AI deployments, leveraging 5G and Multi-access Edge Computing (MEC) to reduce latency and enable faster, localized decision-making.
-
Robustness: Telecom networks are highly dynamic and subject to noisy, heterogeneous data streams as well as adversarial conditions. Evaluating model robustness—how well models maintain performance under varied and potentially hostile scenarios—is critical to preventing catastrophic failures and ensuring security. Techniques such as adversarial training and robustness benchmarks are gaining prominence to mitigate risks unique to AI in telecom.
-
Scalability and Throughput: AI models must handle exponential data growth and unpredictable traffic surges without degradation. Scalability encompasses both architectural design—supporting distributed training and inference—and execution efficiency, ensuring AI solutions can keep pace with network demands across multiple geographic regions and device types.
Balancing these KPIs involves trade-offs; for example, reducing latency with edge deployment can increase infrastructure complexity. Operators must tailor these balances to their operational context, guided by continuous performance monitoring and adaptive management.
Comparing Machine Learning Frameworks and AI Platforms in Telecom
The selection of ML frameworks and AI platforms profoundly influences performance, deployment agility, and long-term scalability for telecom providers:
-
Popular Frameworks: Usage statistics show that Scikit-learn (35%) and PyTorch (32%) are the most widely adopted frameworks among telecom practitioners, with TensorFlow at 8%. Scikit-learn’s simplicity and comprehensive coverage of classical ML algorithms make it ideal for prototyping and feature engineering on medium-sized structured datasets. PyTorch’s dynamic computation graph architecture enables rapid experimentation and supports complex deep learning models favored in research and innovation contexts. TensorFlow offers a robust, production-grade ecosystem with extensive deployment tools like TensorFlow Extended (TFX) and TensorBoard, but its steeper learning curve and rigidity sometimes deter smaller teams or rapid prototyping.
-
Agentic AI Frameworks: As telecoms integrate generative AI and autonomous agents, frameworks such as LangChain, AutoGen (Microsoft), and CrewAI gain traction. These platforms facilitate building sophisticated AI agents capable of autonomous decision-making, collaboration, and workflow automation—core capabilities for next-generation network management and customer engagement.
-
Commercial AI Solutions: Vendors like Whale Cloud provide AI-powered network operation suites designed for Communication Service Providers (CSPs), while hyperscalers such as Google Cloud offer integrated AI platforms (e.g., Vertex AI, Gemini) that enable real-time diagnostics, predictive maintenance, and large-scale data processing. These managed platforms deliver enterprise-grade security, scalability, and faster time-to-value but may introduce vendor lock-in and limit customization.
-
On-Premise vs. Cloud Deployments: Deployment strategy remains a critical decision point. On-premise AI solutions offer low latency and tighter integration with legacy infrastructure, meeting strict data sovereignty and compliance requirements. Conversely, cloud deployments provide elastic scalability, access to cutting-edge AI capabilities, and simplified maintenance. Hybrid cloud models, blending both approaches, are increasingly favored—surveys indicate nearly 75% of network decision-makers regard hybrid cloud as essential for AI strategy, citing improved security, privacy, and flexibility.
Scalability Challenges and Cost-Benefit Considerations
Scaling AI across sprawling telecom networks presents multifaceted challenges that operators must address strategically:
-
Scaling AI Use Cases: Despite growing recognition of AI’s potential, fragmented efforts with isolated pilots remain common. McKinsey research reveals that 42% of telco executives prioritize scaling agentic AI across functions by 2025. Centralized AI platforms that consolidate reusable modules, standardize tools, and enforce governance guardrails are emerging as best practices to accelerate deployment, reduce duplication, and maintain quality.
-
Cost-Benefit Dynamics: Initial investments in AI infrastructure, particularly for generative AI workloads, are substantial. Over 15 global telecom operators have announced plans to build dedicated generative AI data centers, emphasizing strategic control over foundational AI capabilities. However, most telcos are expected to adopt hybrid deployment models or partner with cloud providers to balance cost, complexity, and innovation velocity.
-
Generative AI Impact: Beyond operational cost savings, generative AI unlocks new revenue opportunities and operational efficiencies. Use cases range from automating customer interactions with context-aware chatbots to enhancing network planning through predictive analytics. Success relies on robust data governance, adherence to ethical AI principles, and clear alignment with business objectives to mitigate risks such as hallucinations and bias.
-
Sustainability and Efficiency: The forthcoming 6G networks promise transformative energy efficiency gains, targeting up to a 90% reduction in energy consumption per gigabyte compared to 5G. AI will be instrumental in optimizing network resource allocation, enabling telecoms to meet stringent sustainability goals while supporting unprecedented throughput and low-latency requirements.
Final Thoughts
Effective benchmarking of AI solutions in telecommunications requires a comprehensive approach that goes beyond raw accuracy to include latency, robustness, scalability, and operational integration. The choice of machine learning frameworks and deployment architectures profoundly shapes the ability to embed AI into existing workflows, scale across diverse environments, and maintain compliance with evolving regulations.
As generative and agentic AI capabilities mature, telecom providers stand at a strategic crossroads: whether to invest heavily in in-house AI infrastructure or leverage hybrid cloud ecosystems offering flexibility and innovation. Both paths entail distinct trade-offs in cost, control, agility, and risk.
Ultimately, CSPs that build centralized, modular AI platforms, embed strong ethical governance, and foster cross-functional AI expertise will be best positioned to harness AI’s transformative potential. By doing so, they can evolve from traditional connectivity providers into agile, AI-powered digital experience architects—shaping the telecom landscape for the decade ahead.
Performance Metric | Description | Telecom-Specific Considerations |
---|---|---|
Accuracy and Recall | Measures correctness and completeness in predictions. | Production accuracy can drop to ~70%; use stratified k-fold cross-validation and SMOTE to handle class imbalance; recall improved to 79% to align with business goals. |
Latency | Time delay in model inference and response. | Critical for real-time network management and customer applications; edge AI and MEC with 5G reduce latency bottlenecks from large models. |
Robustness | Model performance stability under noisy, dynamic, and adversarial conditions. | Essential to prevent failures and security risks; adversarial training and robustness benchmarks are increasingly used. |
Scalability and Throughput | Ability to handle data growth and traffic surges efficiently. | Requires distributed training/inference architectures; must support diverse geographies and device types without degradation. |
ML Framework / Platform | Usage Share | Strengths | Considerations |
---|---|---|---|
Scikit-learn | 35% | Simplicity, comprehensive classical ML, ideal for prototyping and medium-sized structured data. | Less suited for deep learning and large-scale deployments. |
PyTorch | 32% | Dynamic computation graph, rapid experimentation, supports complex deep learning models. | Favored in research; may require more effort for production deployment. |
TensorFlow | 8% | Robust production ecosystem; tools like TFX and TensorBoard aid deployment and monitoring. | Steeper learning curve; less flexible for rapid prototyping. |
Agentic AI Frameworks (LangChain, AutoGen, CrewAI) | Emerging | Enable autonomous AI agents for decision-making, collaboration, workflow automation. | Newer technologies; integration and maturity still evolving. |
Commercial AI Solutions (e.g., Whale Cloud, Google Vertex AI) | Varies | Enterprise-grade security, scalability, real-time diagnostics, predictive maintenance. | Potential vendor lock-in; limited customization. |
Deployment Type | Advantages | Challenges |
---|---|---|
On-Premise | Low latency, tight legacy integration, data sovereignty compliance. | Higher infrastructure complexity, limited scalability. |
Cloud | Elastic scalability, access to advanced AI capabilities, simplified maintenance. | Potential data privacy concerns, vendor dependency. |
Hybrid Cloud | Combines benefits of on-premise and cloud; improved security, privacy, flexibility. | Complex management; requires sophisticated orchestration. |
Challenges, Risks, and Responsible AI Adoption in Telecom Networks
Challenges, Risks, and Responsible AI Adoption in Telecom Networks
What unfolds when the transformative power of AI encounters the intricate, fast-evolving domain of telecom networks? The promise of AI-driven automation and optimization is enormous, yet the journey is laden with complex technical and operational challenges. Addressing these requires not only cutting-edge solutions but also ethical foresight and strong governance to ensure sustainable success.
Technical Challenges: Data Quality, Model Interpretability, and Dynamic Environments
Data forms the foundation of AI systems, but in telecommunications, it often presents as a double-edged sword. The sector grapples with enormous, heterogeneous data streams—from real-time network telemetry to customer usage patterns—often fragmented, inconsistent, or locked within legacy infrastructures. This fragmentation introduces noise, bias, and gaps that can cripple AI models before deployment.
The economic impact of poor data quality is staggering; recent analyses estimate a $3.1 trillion annual loss to the U.S. economy alone. Telecom operators that treat data as a strategic product, embedding robust data quality management into their architectures and governance, gain not only improved AI reliability but also competitive advantage.
Emerging AI-powered automation tools now enable continuous monitoring, cleansing, and validation of data at scale, transforming previously manual bottlenecks into strategic assets. However, data quality is just one facet of the technical challenge.
Model interpretability remains a formidable hurdle in telecom AI. Many machine learning applications rely on complex deep neural networks or ensemble models that excel at pattern recognition but operate as “black boxes.” Given that AI decisions can affect millions of users and critical infrastructure, explainability is non-negotiable.
Telecom-specific interpretability techniques—such as layer-wise relevance propagation and surrogate modeling—are gaining traction to illuminate these opaque models. Yet, operators must carefully balance accuracy with transparency, especially when AI influences network prioritization, fault management, or customer interactions.
Maintaining AI models in dynamic telecom environments adds another layer of complexity. Networks are continuously evolving due to technological upgrades (e.g., 5G, edge computing), fluctuating traffic loads, and new service demands. Models trained on historical data degrade rapidly without ongoing retraining and validation.
This necessitates sophisticated model lifecycle management practices, including automated retraining pipelines, real-time performance monitoring, and anomaly detection mechanisms to detect model drift promptly.
Cybersecurity Risks and Adversarial Threats
The stakes of AI system vulnerabilities in telecom networks are exceptionally high, with potential consequences extending to national economies and societal functions. The telecom sector is a prime target for nation-state actors and cybercriminal groups. Recent reports from 2024 and early 2025 document sustained cyberattacks linked to Russia, China, Iran, and North Korea targeting critical telecom infrastructure, threatening large-scale communication disruptions.
AI introduces novel dimensions to this threat landscape. Adversarial attacks—where attackers subtly manipulate input data to deceive AI models—pose unique risks. For instance, crafted noise injected into network telemetry can trigger false alarms or conceal genuine intrusions, undermining security operations.
Traditional cybersecurity defenses, designed for slower, signature-based threat landscapes, often fall short against these sophisticated AI-targeted attacks. Promising innovations like the Low-Rank Iterative Diffusion (LoRID) method have emerged, purifying inputs to remove adversarial perturbations with unprecedented accuracy, offering a robust defense line for telecom AI systems.
AI is a double-edged sword in cybersecurity. While AI-powered tools enable real-time threat detection, anomaly analysis, and automated incident response far exceeding human capabilities, adversaries also leverage AI to craft adaptive, evasive attacks. AI-driven distributed denial-of-service (DDoS) campaigns and rapid zero-day exploitations are increasingly prevalent.
This escalating arms race underscores the imperative for telecom operators to integrate AI defensively as a core component of their cybersecurity strategies, moving beyond add-on solutions to embedded, adaptive defense architectures.
Responsible AI Adoption: Governance, Transparency, and Trust
Given these technical and security complexities, how can telecom providers implement AI responsibly at scale? The answer lies in establishing robust governance frameworks that intertwine technical rigor with ethical stewardship.
AI governance is more than a compliance checklist; it demands ongoing collaboration among engineers, ethicists, policymakers, and customers to define and enforce policies ensuring fairness, accountability, and privacy. While governance models vary across regions and organizations, several common pillars emerge:
-
Transparency: Operators must provide clear, accessible explanations of AI decision-making processes, especially in customer-facing and critical network functions. Explainability is transitioning from a regulatory requirement to a competitive differentiator.
-
Human Oversight: Balancing automation with human-in-the-loop or human-on-the-loop controls is essential to prevent unchecked AI actions and enable timely, informed interventions.
-
Ethical Data Practices: Commitment to diverse, representative datasets and rigorous bias testing mitigates discriminatory outcomes and promotes inclusivity.
-
Privacy and Security: Data governance policies must safeguard sensitive user information, ensuring compliance with regulations such as GDPR and CCPA, thereby fostering customer trust.
Telecom leaders increasingly favor incremental AI deployments through pilot projects that validate feasibility, demonstrate return on investment, and employ clear success metrics to maintain organizational alignment and stakeholder buy-in. This pragmatic approach effectively manages risk while showcasing AI’s tangible benefits.
Moreover, as AI becomes integral to telecom infrastructure, providers are uniquely positioned to act as trusted custodians of the AI era—delivering transparent, reliable services that respect user rights and societal norms.
In summary, the transition to AI-powered telecom networks is a multifaceted balancing act. Technical challenges—spanning data quality, model interpretability, and dynamic model maintenance—require cutting-edge solutions and disciplined operations. Cybersecurity risks mandate vigilant, proactive defense strategies that harness AI’s capabilities defensively. Above all, responsible AI adoption grounded in transparent governance, ethical data practices, and human oversight will be pivotal to sustainable success and public trust in this transformative chapter of telecommunications.
Category | Challenges / Risks | Details | Solutions / Approaches |
---|---|---|---|
Technical Challenges | Data Quality |
|
|
Model Interpretability |
|
|
|
Dynamic Environments |
|
|
|
Cybersecurity Risks | Adversarial Threats |
|
|
AI-driven Cyberattacks |
|
|
|
Responsible AI Adoption | Governance, Transparency, and Trust |
|
|
Future Outlook: AI, 6G, and the Evolution of Intelligent Telecom Networks
Future Outlook: AI, 6G, and the Evolution of Intelligent Telecom Networks
What will the telecom landscape look like once 6G networks become a reality? If 5G marked a significant leap in connectivity, 6G promises a quantum shift—not just in speed but in creating a fully AI-native ecosystem that transforms how networks operate, interact, and serve society. At the core of this transformation is artificial intelligence, evolving from a supportive tool to the fundamental fabric of network design, automation, and user experience.
AI as the Backbone of 6G: Ubiquitous Connectivity and Autonomous Network Slicing
6G networks are expected to seamlessly and intelligently connect hundreds of billions of devices—from smartphones and sensors to autonomous vehicles and robots. Managing this scale and complexity with manual or traditional rule-based systems is no longer feasible.
AI will enable:
- Ubiquitous connectivity through real-time, dynamic adaptation of network resources, ensuring uninterrupted service even in highly congested or challenging environments.
- Autonomous network slicing, where AI orchestrates multiple virtual networks customized for specific applications—whether ultra-reliable low-latency communication for remote surgery or high-throughput slices for immersive augmented reality experiences.
The AI-native network slicing architecture is a pivotal innovation. Research from the University of Waterloo and others shows AI deeply embedded across the entire network slicing lifecycle—from predicting service demand using recurrent neural networks (RNNs) to real-time resource allocation with reinforcement learning algorithms like Deep Deterministic Policy Gradient (DDPG).
For example, consider a network slice dedicated to road safety applications that leverages AI to guarantee latency below 100 milliseconds. The system continuously monitors data collection, model training, and inference phases, optimizing resource distribution across base stations and UAVs to meet stringent quality-of-service requirements. This synergy of AI and network slicing maximizes efficiency, reduces operational costs, and enhances security—with studies projecting up to 40% efficiency improvements and 50% cost reductions.
Frontier AI Models: Large Language Models and Agentic AI in Telecom Automation and Customer Experience
Beyond network management, large language models (LLMs) are emerging as transformative agents in telecom operations and customer interactions. SoftBank’s development of a Large Telecom Model (LTM), trained specifically on telecom data, achieves over 90% accuracy in predicting network configurations, accelerating automation and minimizing human errors.
These models also enable agentic AI systems—intelligent agents capable of autonomously detecting network anomalies, generating resolution playbooks, and proactively predicting disruptions before they affect users. NVIDIA’s collaboration with leading telecom players illustrates this trend; their AI agents, powered by specialized LLMs, are being integrated to manage Radio Access Networks (RAN) and security operations, with projections that AI will autonomously handle up to 75% of network security tasks by 2025.
On the customer side, AI-driven personalization is poised to revolutionize engagement. At Mobile World Congress 2025, companies demonstrated AI-native infrastructure that self-optimizes and personalizes connectivity and service delivery. Innovations like Deutsche Telekom’s AI-powered phone, Vodafone’s partnership with IBM on quantum-safe technologies, and Microsoft’s telecom-specific AI data models signal an era where AI fosters customer loyalty through hyper-personalized, anticipatory services.
Emerging Trends: Quantum Machine Learning, Edge AI, and Societal Implications
Looking further ahead, two rising AI trends are set to reshape telecom networks and their societal impact: quantum machine learning (QML) and edge AI.
Quantum computing, once theoretical, is now rapidly advancing. Events like IEEE Quantum Week 2025 and specialized workshops emphasize research into quantum-enhanced algorithms capable of tackling complex optimization and security problems beyond classical computing’s reach. For telecom, this translates into quantum-powered network optimization, ultra-secure communication protocols, and accelerated AI model training.
Meanwhile, edge AI—processing data locally on devices or at network edges—addresses critical challenges of latency, bandwidth constraints, and privacy. By reducing reliance on centralized cloud processing, edge AI supports real-time analytics and decision-making essential for applications such as autonomous vehicles, smart cities, and industrial IoT. Retail environments, for instance, are deploying edge AI for facial recognition and crowd analytics to enhance customer experiences without compromising privacy.
These technological advances raise profound societal questions:
- Digital inclusion: AI-driven 6G networks offer the potential to close connectivity gaps by intelligently allocating resources to underserved regions, but realizing this requires deliberate, equity-focused deployment strategies.
- Workforce transformation: As AI automates routine network operations, the telecom workforce will evolve, emphasizing roles in AI oversight, ethical governance, and strategic innovation.
Policymakers and organizations must carefully manage this transition to harness AI’s benefits while mitigating risks such as job displacement and exacerbated digital divides.
Key Takeaways
- AI will be the driving force behind 6G’s promise, enabling ubiquitous, efficient, and secure connectivity through autonomous network slicing and ultra-low latency applications.
- Large language models and agentic AI are already revolutionizing network automation and customer interaction, heralding a future where networks self-manage and personalize at scale.
- Emerging fields like quantum machine learning and edge AI will introduce new capabilities and complexities, necessitating thoughtful integration to ensure technology serves societal interests.
- The evolution of intelligent telecom networks is not just a technical challenge but also a societal one, demanding ethical foresight alongside engineering expertise.
In summary, the journey to 6G is as much about reimagining network intelligence as it is about redefining the role of AI in shaping a connected, inclusive, and resilient future.
Aspect | Description | Examples / Details | Impact / Benefits |
---|---|---|---|
AI as Backbone of 6G | AI enables ubiquitous connectivity and autonomous network slicing for intelligent resource management | Network slices for remote surgery (ultra-low latency), augmented reality (high throughput); AI models like RNNs and DDPG for demand prediction and resource allocation | Uninterrupted service, 40% efficiency improvements, 50% cost reductions, enhanced security |
Frontier AI Models | Large Language Models (LLMs) and agentic AI automate telecom network operations and customer experience | SoftBank’s Large Telecom Model (90%+ accuracy); NVIDIA’s AI agents managing RAN and security; AI-driven personalization at Mobile World Congress 2025 | Accelerated automation, minimized human error, up to 75% network security tasks automated by 2025, hyper-personalized customer engagement |
Emerging Trends | Quantum Machine Learning and Edge AI reshape telecom capabilities and societal impact | Quantum-enhanced algorithms for optimization and security; Edge AI for local data processing in autonomous vehicles, smart cities, retail facial recognition | Improved optimization, ultra-secure communication, reduced latency, enhanced privacy, real-time analytics |
Societal Implications | Challenges and opportunities related to digital inclusion and workforce transformation | AI-driven resource allocation to underserved regions; evolving telecom workforce roles in AI oversight and ethics | Potential to close connectivity gaps; need for ethical governance; managing job displacement risks |
Key Takeaways | Summary of AI’s transformative role in future telecom networks | 6G driven by AI-native networks, autonomous slicing, LLMs in automation, quantum and edge AI emergence | Efficient, secure, inclusive, and personalized telecom future requiring ethical foresight |