AI in Sports: Unveiling Performance Analytics & Coaching Insights
- Introduction: Why AI is a Game-Changer in Sports Analytics and Coaching
- Introduction: Why AI is a Game-Changer in Sports Analytics and Coaching
- Transforming Player Performance and Coaching Strategies
- Injury Prevention: A New Frontier in Athlete Care
- Revolutionizing Fan Engagement and Ethical Considerations
- A Balanced Perspective on AI’s Role in Sports
- Foundations of AI in Sports: Core Technologies and Data Infrastructure
- Foundations of AI in Sports: Core Technologies and Data Infrastructure
- Core AI Technologies Shaping Sports Analytics
- Critical Data Sources: The Fuel for AI in Sports
- Navigating Data Quality, Preprocessing, and Integration Challenges
- Key Performance Metrics and Evaluation Criteria in AI Models
- Technical Deep Dive: Machine Learning Models and Algorithms Driving Performance Insights
- Technical Deep Dive: Machine Learning Models and Algorithms Driving Performance Insights
- Supervised Learning: Injury Prediction and Performance Forecasting
- Reinforcement Learning: Strategy Optimization on the Field
- Unsupervised Learning: Discovering Hidden Patterns
- Model Training Workflows: From Data to Deployment
- Real-Time Data Processing: The Final Frontier
- Concrete Example: Liverpool FC’s AI-Driven Recruitment and Performance Systems
- Balancing Hype with Reality
- AI Tools and Platforms: Evaluating Leading Solutions in Sports Analytics
- AI Tools and Platforms: Evaluating Leading Solutions in Sports Analytics
- Technical Specifications and Core Functionalities
- Integration Capabilities and Real-Time Feedback
- Benchmarking Accuracy, Scalability, and User Adoption
- Key Takeaways
- Practical Applications: Enhancing Coaching, Injury Prevention, and Fan Engagement
- Practical Applications: Enhancing Coaching, Injury Prevention, and Fan Engagement
- Smarter Coaching Through Personalization and Tactical Intelligence
- Predicting and Preventing Injuries with AI-Enabled Athlete Management
- Revolutionizing Fan Engagement: Immersive, Personalized, and Ethical
- Balancing Innovation with Responsibility
- Challenges, Limitations, and Ethical Considerations in AI-Driven Sports Analytics
- Challenges, Limitations, and Ethical Considerations in AI-Driven Sports Analytics
- Data Biases: The Invisible Fouls in AI Performance Analytics
- Athlete Data Privacy and Consent: Guarding the Playing Field of Personal Information
- Regulatory and Governance Frameworks: Balancing Innovation with Fairness and Transparency
- The Human Element: Why AI Should Augment, Not Replace, Human Judgment
- Future Directions: Emerging Trends and the Evolving Role of AI in Sports
- Future Directions: Emerging Trends and the Evolving Role of AI in Sports
- Integrating Multimodal Data: The Next Frontier in Performance Analytics
- Real-Time Adaptive Coaching and Immersive Technologies: Coaching 2.0
- Frontier Technologies: Explainable AI and Federated Learning in Sports Analytics
- Broader Impacts: Competitive Balance, Athlete Health, and the Sports Ecosystem
- A Note of Caution: Navigating Uncertainties
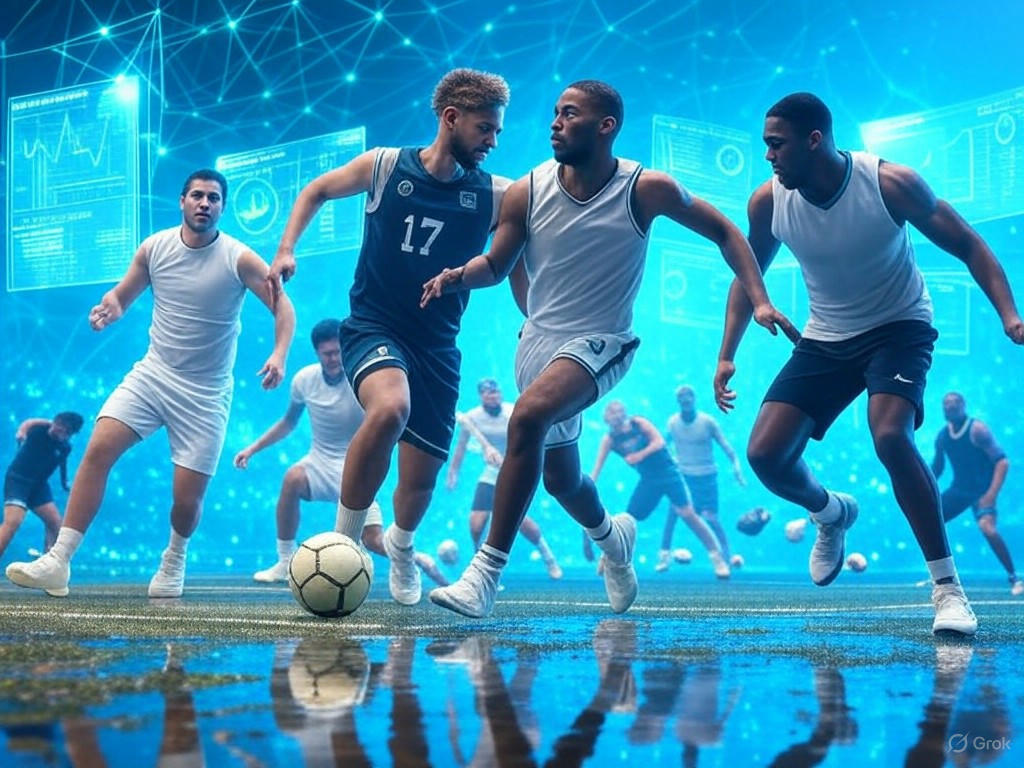
Introduction: Why AI is a Game-Changer in Sports Analytics and Coaching
Introduction: Why AI is a Game-Changer in Sports Analytics and Coaching
What if the subtle nuances that distinguish a good athlete from a great one could be decoded, quantified, and enhanced in real time? Thanks to artificial intelligence (AI), this is no longer a hypothetical scenario but an emerging reality. With over 15 years of experience architecting AI systems, I have witnessed firsthand how AI integration is reshaping sports analytics and coaching—impacting not only elite professionals but athletes across all levels.
Transforming Player Performance and Coaching Strategies
Traditional scouting and player development have historically depended on subjective observations, intuition, and limited datasets. AI disrupts this model by leveraging vast volumes of data—from wearable sensors to extensive video footage—and applying advanced machine learning algorithms to uncover patterns invisible to the human eye.
For example, AI-powered computer vision technologies analyze every movement on the field, offering coaches precise insights into player positioning, decision-making, and fatigue levels. This enables data-driven tactical adjustments and personalized feedback that were previously unattainable in real time.
Beyond elite sports, AI democratizes talent identification, especially in developing regions where financial or geographic barriers limited access to high-level training. Research from Loughborough University highlights how AI-driven scouting objectively evaluates performance metrics, surfacing hidden talents and fostering a more inclusive and meritocratic sports ecosystem.
Coaching strategies themselves are evolving rapidly. AI systems simulate countless tactical scenarios, identify opponents’ weaknesses, and optimize training schedules tailored to each athlete’s physiological and psychological profile. Personalized training regimens powered by AI not only boost performance but also extend athletes’ careers by minimizing risks associated with overtraining.
Injury Prevention: A New Frontier in Athlete Care
Injury prevention stands as one of AI’s most compelling contributions to sports. Wearable devices equipped with AI monitor an athlete’s biomechanics in real time, detecting subtle deviations in movement that may signal impending injury before symptoms appear.
These systems integrate data from motion capture, physiological sensors, and historical injury records to predict and prevent injuries with greater accuracy than traditional methods. For instance, the NFL’s Digital Athlete program continuously monitors player health metrics, dynamically adjusting workloads to reduce injury rates.
Similarly, predictive analytics platforms ingest multi-source data—while respecting data privacy and ownership—to deliver actionable insights for coaches and medical teams. The sports injury prediction market is projected to grow significantly in North America and Asia-Pacific, reflecting robust confidence in AI’s preventive capabilities.
Furthermore, integration of virtual reality (VR) and augmented reality (AR) into rehabilitation is accelerating. These immersive technologies create safe and effective recovery environments, enabling athletes to regain function with enhanced engagement and precision.
Despite these advances, challenges remain around data privacy, ethical use, and the reliability of AI predictions. Addressing these requires rigorous governance frameworks and ongoing collaboration between technologists, medical professionals, and sports organizations.
Revolutionizing Fan Engagement and Ethical Considerations
AI’s transformative impact extends beyond the playing field to redefine how fans engage with sports. Personalized content delivery, real-time statistics, automated highlight reels, and immersive AR overlays are turning passive spectators into active participants.
According to the 2025 Stats Perform report, over 700 sports executives emphasize AI’s pivotal role in crafting customized fan journeys that boost engagement and unlock new revenue streams. AI-powered chatbots and virtual assistants provide instant responses to ticketing inquiries and merchandise questions, enhancing operational efficiency.
However, this evolution raises critical ethical questions. The collection and analysis of personal and biometric data necessitate stringent safeguards to protect athlete privacy and autonomy. The rise of AI-generated content—such as deepfakes and automated commentary—introduces concerns about authenticity, transparency, and the potential erosion of human judgment in sports entertainment.
Regulatory frameworks are striving to keep pace with these technological innovations. Forward-thinking organizations must balance enthusiasm for AI’s possibilities with responsibility, ensuring that AI complements human expertise and operates within clear ethical boundaries.
A Balanced Perspective on AI’s Role in Sports
The sports industry stands at a technological crossroads. AI offers unprecedented capabilities—from enhancing athletic performance and reducing injuries to enriching fan experiences. Yet it simultaneously challenges long-standing norms around privacy, fairness, and the human essence of competition.
This article takes a critical yet optimistic approach, unpacking the technical advances alongside ethical and societal implications. Through concrete case studies and current trends, we aim to provide a nuanced understanding of how AI is truly a game-changer—and what it means for the future of sports.
Aspect | AI Application | Benefits | Examples/Notes |
---|---|---|---|
Player Performance | Computer vision, machine learning | Precise insights on positioning, decision-making, fatigue; personalized feedback; data-driven tactics | Analysis of movements on field; real-time adjustments |
Talent Identification | AI-driven scouting | Objective evaluation; discovers hidden talent; democratizes access | Research by Loughborough University; focus on developing regions |
Coaching Strategies | Simulation of tactical scenarios; training optimization | Tailored training schedules; career longevity; risk reduction | Physiological and psychological profiling |
Injury Prevention | Wearable AI devices; predictive analytics | Early detection of injury risks; workload adjustment; reduced injury rates | NFL Digital Athlete program; motion capture and sensor data integration |
Rehabilitation | Virtual Reality (VR) and Augmented Reality (AR) | Safe recovery environments; enhanced engagement and precision | Accelerates rehabilitation process |
Fan Engagement | Personalized content; AI chatbots; AR overlays | Customized experiences; operational efficiency; increased revenue streams | 2025 Stats Perform report; over 700 sports executives involved |
Ethical Considerations | Data privacy safeguards; governance frameworks | Protects athlete privacy; ensures transparency; maintains human judgment | Concerns over biometric data and AI-generated content |
Foundations of AI in Sports: Core Technologies and Data Infrastructure
Foundations of AI in Sports: Core Technologies and Data Infrastructure
What drives the transformative wave in sports analytics today? It is the synergy of advanced AI technologies—machine learning, computer vision, predictive modeling, and natural language processing—intertwined with diverse, high-quality data streams. A firm grasp of these foundational elements is essential to understand how AI reshapes athlete performance, coaching strategies, and fan engagement.
Core AI Technologies Shaping Sports Analytics
Machine learning (ML) forms the backbone of sports analytics, empowering systems to uncover intricate patterns within vast datasets. For example, IPL 2025 teams employ decision tree algorithms to simulate match scenarios and cluster analysis to categorize players by style, optimizing strategic matchups. This shift moves coaching from intuition-driven methods to data-driven insights, revealing nuances invisible to the naked eye.
Computer vision has emerged as a game-changer by enabling machines to interpret visual data from multi-angle video feeds. AI-powered systems can track player movements, ball trajectories, and referee decisions in real time with remarkable precision. This technology is extensively applied in bat-and-ball sports such as cricket and baseball, as well as fitness monitoring exercises like squats and lunges. A notable application is the measurement of “pressing intensity” in soccer—calculating how quickly defenders reach attackers to regain possession—providing coaches with actionable defensive metrics.
Predictive modeling further elevates sports strategy by analyzing historical and live data to forecast player performance, injury risks, and game outcomes. This is no longer theoretical: teams like the Toronto Raptors operate “analytics war rooms” that deliver in-game predictions, guiding tactical decisions dynamically.
Natural language processing (NLP) adds a less visible but equally impactful layer. Beyond numeric and visual data, NLP algorithms summarize live match events, generate highlight reels, and script commentaries. The IPL’s use of NLP to produce instant match summaries exemplifies AI’s role in enriching coaching insights and fan experiences.
Critical Data Sources: The Fuel for AI in Sports
Sophisticated AI models rely on robust, varied data streams. Sports organizations harness multiple sources, including:
-
Wearable sensors: Devices monitoring physiological metrics such as heart rate, muscle activity, and movement patterns. Innovations range from skin patches with microneedles to dry electrodes embedded in headphones, offering granular insights into athlete health. The wearable sensor market is projected to grow steadily at around 5% CAGR through 2035.
-
Video feeds: High-definition cameras capture live action from multiple angles. Computer vision techniques process these feeds to extract spatial and temporal data, including player positioning, ball speed, and team formation dynamics.
-
Internet of Things (IoT) devices: Connected equipment like smart footwear and apparel generate real-time performance data, bridging physical and digital domains. IoT empowers coaches with contextual insights formerly inaccessible, enhancing training and in-game decisions.
-
Historical performance databases: Comprehensive repositories such as the Ergast Formula One and MLB historical scores provide decades-spanning context for predictive models and benchmarking. These datasets refine AI’s analytical precision by embedding sports history.
Integrating these data types establishes a powerful ecosystem but introduces unique challenges.
Navigating Data Quality, Preprocessing, and Integration Challenges
Sports data is inherently heterogeneous—originating from diverse sensors, video systems, and databases with varying formats and update frequencies. Effective AI analytics demand rigorous preprocessing to ensure data quality and consistency.
Key challenges include:
-
Data inconsistencies and noise: Wearables may experience signal dropouts; video feeds can suffer occlusions or lighting variations. Filtering and calibration are vital to maintain reliable accuracy.
-
Synchronizing multimodal data: Aligning timestamped sensor readings with video and IoT data requires precise synchronization to prevent analytical errors.
-
Cross-platform integration: Combining real-time streaming data with large historical datasets necessitates robust infrastructure and scalable cloud solutions.
-
Bias and representativeness: AI model efficacy hinges on training data quality. For instance, performance metrics derived from male athletes may not generalize to female athletes without adequate adjustment, underscoring the need for inclusive datasets.
Data preprocessing pipelines often include feature extraction, normalization, and dimensionality reduction techniques such as Principal Component Analysis (PCA) to distill complex datasets while preserving critical information. Clustering algorithms further identify behavioral or tactical patterns, like grouping teams by defensive aggressiveness or play styles.
Key Performance Metrics and Evaluation Criteria in AI Models
Success in sports AI transcends raw accuracy; evaluation metrics must align with the practical imperatives of coaches, athletes, and organizations. Common criteria include:
-
Predictive accuracy: The model’s capability to forecast outcomes such as player performance or injury likelihood. Soccer’s expected goals (xG) metric exemplifies quantifying scoring chance quality, serving as a benchmark for offensive effectiveness.
-
Real-time responsiveness: Insights must be delivered with minimal latency to influence in-game decisions, demanding efficient algorithms and optimized data pipelines.
-
Interpretability: Coaches favor transparent, understandable insights over black-box predictions. Metrics like defensive win percentage or interception value must be clearly communicated to inform tactical adjustments.
-
Robustness and generalization: AI models should maintain reliable performance across teams, leagues, and conditions, avoiding overfitting to niche datasets.
-
Integration with human expertise: Despite AI’s power, seasoned coaches’ intuition remains irreplaceable. Effective AI systems augment rather than replace human judgment.
The evolution of sports analytics often follows a four-stage maturity model:
-
Descriptive: What happened?
-
Diagnostic: Why did it happen?
-
Predictive: What will happen?
-
Prescriptive: How can the desired outcome be achieved?
Leading organizations are actively advancing through these stages, leveraging AI not only for analysis but to proactively shape training and game strategies.
In summary, the foundation of AI in sports analytics is a complex fusion of cutting-edge machine learning, computer vision, predictive modeling, and NLP technologies, fueled by rich, multi-source data. Overcoming challenges in data quality and integration requires thoughtful engineering and domain expertise.
As these technologies mature, their impact will deepen—enhancing athletic performance, optimizing coaching decisions, and revolutionizing fan engagement. Yet, the human element remains central. AI tools must be designed to complement, not supplant, the nuanced expertise that defines elite sports coaching and management.
AI Technology | Description | Applications in Sports | Examples |
---|---|---|---|
Machine Learning (ML) | Empowers systems to uncover intricate patterns within vast datasets. | Simulating match scenarios, categorizing players by style, optimizing strategic matchups. | IPL 2025 teams using decision tree algorithms and cluster analysis. |
Computer Vision | Enables machines to interpret visual data from multi-angle video feeds. | Tracking player movements, ball trajectories, referee decisions in real time. | Measuring “pressing intensity” in soccer, fitness monitoring exercises. |
Predictive Modeling | Analyzing historical and live data to forecast player performance, injury risks, and game outcomes. | In-game predictions guiding tactical decisions. | Toronto Raptors’ “analytics war rooms”. |
Natural Language Processing (NLP) | Summarizes live match events, generates highlight reels, scripts commentaries. | Producing instant match summaries and enriching fan experiences. | IPL’s use of NLP for match summaries. |
Data Source | Description | Examples/Applications | Market Trends |
---|---|---|---|
Wearable Sensors | Devices monitoring physiological metrics like heart rate, muscle activity, and movement. | Skin patches with microneedles, dry electrodes in headphones. | Projected 5% CAGR growth through 2035. |
Video Feeds | High-definition cameras capturing live action from multiple angles. | Extracting spatial/temporal data: player positioning, ball speed, team formations. | Used in computer vision applications. |
Internet of Things (IoT) Devices | Connected equipment generating real-time performance data. | Smart footwear and apparel providing contextual insights. | Enhances training and in-game decisions. |
Historical Performance Databases | Comprehensive repositories providing decades-spanning sports data. | Ergast Formula One, MLB historical scores. | Refines AI analytical precision. |
Challenge | Description | Impact | Solutions/Techniques |
---|---|---|---|
Data Inconsistencies and Noise | Signal dropouts in wearables, occlusions or lighting variations in video feeds. | Reduces accuracy of AI analytics. | Filtering, calibration. |
Synchronizing Multimodal Data | Aligning timestamped sensor, video, and IoT data. | Prevents analytical errors. | Precise synchronization techniques. |
Cross-platform Integration | Combining real-time streaming data with large historical datasets. | Requires robust infrastructure. | Scalable cloud solutions. |
Bias and Representativeness | Training data may lack diversity, e.g., male-centric performance metrics. | Limits model generalization. | Inclusive datasets and adjustments. |
Metric/Evaluation Criterion | Description | Importance | Examples |
---|---|---|---|
Predictive Accuracy | Model’s ability to forecast outcomes like player performance or injury risk. | Benchmark for effectiveness. | Soccer’s expected goals (xG) metric. |
Real-time Responsiveness | Delivering insights with minimal latency. | Influences in-game decisions. | Efficient algorithms and data pipelines. |
Interpretability | Transparency and understandability of AI insights. | Facilitates tactical adjustments by coaches. | Defensive win percentage, interception value. |
Robustness and Generalization | Maintaining reliable performance across teams and conditions. | Avoids overfitting. | Applicable across leagues and datasets. |
Integration with Human Expertise | Augmenting rather than replacing human judgment. | Combines AI power with coaching intuition. | Effective AI-human collaboration. |
Stage | Description |
---|---|
Descriptive | What happened? |
Diagnostic | Why did it happen? |
Predictive | What will happen? |
Prescriptive | How can the desired outcome be achieved? |
Technical Deep Dive: Machine Learning Models and Algorithms Driving Performance Insights
Technical Deep Dive: Machine Learning Models and Algorithms Driving Performance Insights
What powers the AI-driven performance insights that are reshaping modern sports? Let’s unpack the machine learning techniques and workflows that teams, medical staff, and analysts rely on to transform raw data into actionable intelligence.
Supervised Learning: Injury Prediction and Performance Forecasting
Supervised learning forms the backbone of many sports analytics applications, particularly injury prediction. Models are trained on labeled datasets—incorporating player biometrics, workload statistics, and historical injury records—to identify risk factors signaling potential injuries.
For instance, research indicates that tree-based models often outperform other algorithms in predicting injuries in football (soccer) and rugby union, achieving around 70% accuracy. Interestingly, classical methods like logistic regression sometimes outperform complex machine learning models, highlighting that increased complexity does not always equate to better predictions.
In practice, injury risk models ingest diverse inputs such as GPS tracking data, heart rate variability, and biomechanical markers collected via wearable sensors. These models can detect athletes at heightened risk for conditions like medial stress syndrome or soft tissue injuries, enabling timely, preventative interventions.
However, challenges persist. Data quality can be inconsistent, and overfitting—where models capture noise rather than meaningful patterns—is a common risk. Regularization techniques such as L1 (lasso) and L2 (ridge) penalize model complexity, helping ensure predictions generalize effectively across different players and seasons.
Reinforcement Learning: Strategy Optimization on the Field
How does AI dynamically optimize game strategies in real time? Reinforcement learning (RL) offers a compelling answer. Unlike supervised learning, RL models learn through interaction with an environment—such as a simulated match—receiving feedback via rewards (e.g., points scored, defensive stops).
Recent studies showcase RL’s prowess in sports video tracking, achieving accuracies exceeding 99%. Beyond tracking, RL frameworks simulate countless tactical scenarios to refine formations, player positioning, and play calls, learning strategies that maximize favorable outcomes.
A concrete example is Liverpool FC’s post-Klopp strategic hiring and tactical refinement, which reportedly leveraged data-driven models potentially incorporating RL elements. This shift from intuition-based coaching to algorithmically informed decision-making marks a fundamental transformation in competitive sports.
Unsupervised Learning: Discovering Hidden Patterns
Not all valuable insights come from labeled data. Unsupervised learning techniques—such as clustering and dimensionality reduction—uncover latent structures and patterns without predefined outcomes.
In sports, this translates to grouping players by playing style or physical attributes, identifying emergent team behaviors, or segmenting opponents’ tactical patterns. The Indian Premier League (IPL), for example, employs cluster analysis to optimize player matchups and strategic depth.
Unsupervised methods excel in exploratory analysis, aiding scouts in uncovering undervalued talent and helping coaches recognize novel strategies that might otherwise go unnoticed.
Model Training Workflows: From Data to Deployment
Building effective machine learning models in sports is a complex process involving multiple stages:
-
Data Collection & Preprocessing
Aggregating sensor data, video annotations, and historical statistics. High-quality labeled data is critical for precise training and evaluation. -
Feature Engineering
Selecting and transforming variables that best represent player conditions and game contexts. -
Model Selection
Choosing algorithms suited to the task—for example, random forests for injury prediction or deep RL for strategy optimization. -
Hyperparameter Tuning
Optimizing model parameters such as tree depth or learning rate. Traditional methods include grid search (exhaustive but computationally heavy) and random search (more efficient but less systematic). Advanced frameworks like Optuna leverage probabilistic models and pruning strategies to accelerate tuning and conserve resources. -
Regularization
Applying L1 or L2 penalties to prevent overfitting, especially vital when handling high-dimensional, noisy sports data. -
Validation & Testing
Employing cross-validation and holdout datasets to ensure robust performance on unseen data. -
Real-Time Integration
Deploying models to process streaming data from wearables and video feeds, delivering instant feedback to coaches and medical teams.
This pipeline demands substantial computational resources and domain expertise. For example, Liverpool FC integrates AI platforms that automatically generate player highlights and track development, feeding into recruitment and tactical decisions. Balancing model complexity with latency is critical—real-time analytics require fast, reliable processing without compromising accuracy.
Real-Time Data Processing: The Final Frontier
By 2025, the convergence of wearable sensors, computer vision, and cloud computing forms a powerful ecosystem delivering real-time insights. Systems like KINEXON PERFORM’s GPS and LPS tracking have passed FIFA’s stringent Electronic Performance and Tracking System tests, ensuring precision and reliability.
These technologies provide granular workload metrics—such as acceleration, deceleration, and heart rate zones—directly to coaching staff. AI models then analyze this continuous data stream, flagging abnormal patterns signaling fatigue or injury risk.
Nonetheless, real-time systems face challenges including latency, data consistency, and noise handling. Synchronizing multiple data streams seamlessly is essential. Moreover, protecting athlete privacy while leveraging sensitive biometric data remains a paramount ethical concern.
Concrete Example: Liverpool FC’s AI-Driven Recruitment and Performance Systems
Liverpool FC exemplifies advanced AI integration in sports operations. Following the Klopp era, management employed algorithm-informed processes for managerial appointments and player recruitment. This approach incorporated advanced performance indicators and predictive analytics to assess player fit and potential.
Furthermore, Liverpool’s partnership with AI-driven video analysis companies enables automatic highlight generation and progress tracking. This accelerates player development across their global academies, reducing manual scouting efforts and introducing objective, data-backed evaluations.
Balancing Hype with Reality
While AI’s potential in sports is immense, it is vital to maintain pragmatic expectations. Injury prediction models with approximately 70% accuracy leave considerable room for improvement; misclassifications can carry serious consequences. Reinforcement learning models require carefully designed reward functions to avoid unintended tactical behaviors.
Transparency in AI decision-making and continuous model updates are essential for sustained success. The synergy between human expertise and AI insights is paramount—AI should augment, not replace, the nuanced judgment of coaches, trainers, and medical professionals.
In summary, machine learning in sports orchestrates supervised prediction, reinforcement-driven strategy optimization, and unsupervised pattern discovery. Combined with rigorous training workflows and real-time data integration, these technologies are setting new standards for performance insights. They are reshaping how athletes train, compete, and recover, yet fully realizing AI’s promise demands ongoing innovation, ethical vigilance, and a clear-eyed awareness of its limitations.
Machine Learning Technique | Applications in Sports | Key Features | Examples / Notes |
---|---|---|---|
Supervised Learning | Injury Prediction, Performance Forecasting |
|
|
Reinforcement Learning | Real-time Strategy Optimization, Tactical Simulation |
|
|
Unsupervised Learning | Pattern Discovery, Player Clustering, Opponent Analysis |
|
|
Model Training Workflows | End-to-End ML Model Development |
|
|
Real-Time Data Processing | Live Performance Monitoring and Feedback |
|
|
AI Tools and Platforms: Evaluating Leading Solutions in Sports Analytics
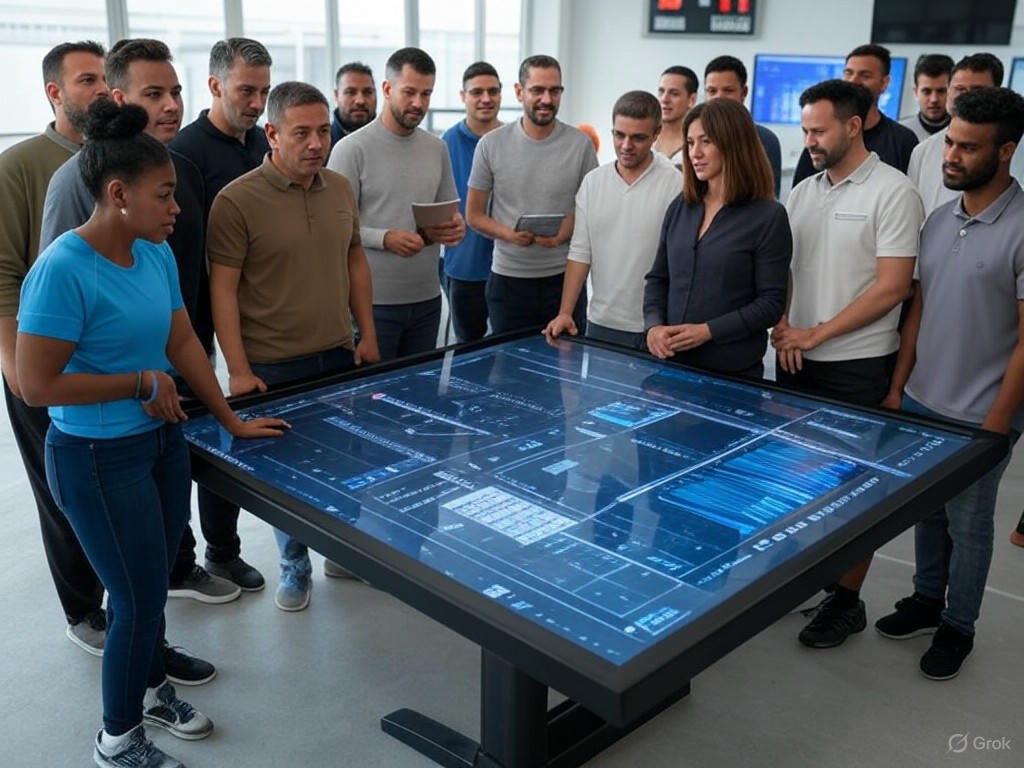
AI Tools and Platforms: Evaluating Leading Solutions in Sports Analytics
The landscape of AI-driven sports analytics platforms is vast and varied, with each solution carving out a unique position based on its technical capabilities, user experience, and integration potential. To navigate this complexity, we’ll analyze leading platforms—Hudl Sportscode, Coach’s Eye, Dartfish, Nacsport, and Krossover—highlighting their core functionalities, technical specifications, and real-time integration features relevant to performance analytics and coaching insights.
Technical Specifications and Core Functionalities
Hudl Sportscode stands out as a highly customizable powerhouse tailored for elite teams with complex tactical demands. The 2025 version introduces faster scripting workflows and enhanced multi-angle IP video capture, crucial for teams managing multiple camera feeds simultaneously. Its ability to handle diverse video sources—from local AVFoundation captures to IP streams—supports granular video analysis.
Key features include:
- Exclusive link toggling for precise clip control
- Advanced labeling and tagging for nuanced play annotation
- Telestration tools for detailed visual breakdowns
- Extended timeline trimming and matrix playback for dissecting complex tactics
These capabilities empower coaches to annotate and review gameplay with exceptional precision, aligning with the needs of top-tier teams in sports like football, rugby, and basketball.
Coach’s Eye targets accessibility and rapid feedback with a mobile-first approach. Its intuitive interface excels in quick video capture and annotation, making it ideal for individual athletes and smaller coaching staffs who prioritize ease of use over extensive customization. While it lacks the depth of Hudl’s workflow integrations, Coach’s Eye serves well for post-session visual reviews but offers limited real-time data integration.
Dartfish brings decades of innovation with its pioneering SimulCam™ technology and AI-enhanced video analysis. It integrates computer vision and machine learning to provide detailed biomechanical insights, especially valued in individual sports such as cycling and tennis. The platform supports private video hosting, collaborative tagging, and live tracking with data overlays to facilitate near real-time performance evaluation. Dartfish’s modular offerings, like myDartfish Tennis, scale from grassroots to elite levels but often require specialized knowledge to unlock full potential.
Nacsport offers a versatile video analysis suite emphasizing tagging, drawing, and reporting tools. Recent 9.x releases introduced native Mac support and expanded device compatibility, broadening its user base. Its integration with external data streams like InStat sports videos enriches analytics. Nacsport Hub, their online collaboration platform, functions as a private social network, enabling teams to share videos, add commentary, and track metrics collectively. These features support real-time collaboration and tactical adjustments, although advanced functionality may demand significant setup and training.
Krossover simplifies video breakdown and statistical analysis, targeting amateur to mid-tier professional teams. Its strength lies in automating manual tagging processes, significantly reducing analyst workload. While it offers less customization compared to Hudl or Dartfish, Krossover’s streamlined approach suits resource-constrained teams seeking efficient post-game analysis without the complexity of deep tactical tools.
Integration Capabilities and Real-Time Feedback
Real-time feedback is now essential for modern coaching, shifting how in-game decisions and training adjustments occur. Platforms that ingest, process, and present data seamlessly during live sessions provide a strategic edge.
-
Hudl Sportscode enhances its capture capabilities with API support, enabling integration into broader team workflows. Coaches can synchronize video with sensor data, accelerating the generation of actionable insights. Improved video latency and live sharing features allow coaches, analysts, and players to access key moments simultaneously during games or practices.
-
Nacsport leverages its integration with InStat and the collaborative Hub platform to support real-time clip comparison and live metric tracking. This immediacy facilitates tactical changes mid-game, critical in fast-paced sports where split-second decisions matter.
-
Dartfish employs AI-powered computer vision in its live software to provide dynamic in-game analysis. Its real-time player and puck tracking—common in broadcast and betting contexts—demonstrates the convergence of media and coaching analytics. Coaches in tennis and cycling utilize Dartfish’s tagging panels and online reports to deliver near-instant feedback during competition intervals.
-
Coach’s Eye, despite its strengths in mobile video capture and annotation, focuses primarily on post-session review. Its limited real-time data integration may constrain teams seeking immediate tactical adjustments on the field.
-
Krossover emphasizes rapid post-game breakdown and integrates data sources to accelerate clip generation and statistical summaries, enabling faster turnaround times for coaches without live feedback capabilities.
Benchmarking Accuracy, Scalability, and User Adoption
Selecting the right AI sports analytics tool hinges on balancing accuracy, scalability, and usability within the coaching ecosystem.
-
Hudl Sportscode enjoys widespread adoption among elite teams due to its accuracy and scalability. It supports complex workflows with deep customization but presents a steep learning curve, often necessitating dedicated analysts or tech-savvy coaching staff for maximal benefit.
-
Nacsport is gaining traction through its versatility and cross-platform availability. The native Mac support and collaborative Hub platform encourage broader team engagement and shared analytics, although advanced features require investment in setup and training.
-
Dartfish excels in biomechanical and motion analysis precision, particularly for technical individual sports. Its modular design allows scalability from academies to elite competition but often requires specialized expertise, limiting casual user accessibility.
-
Coach’s Eye prioritizes usability, making it popular among smaller teams or individual athletes seeking rapid visual feedback without extensive analytics. Its simplicity promotes higher adoption where resources or technical support are limited.
-
Krossover appeals to teams seeking to automate tedious tagging and statistical tasks. While it reduces manual effort, its relative simplicity may not suffice for organizations demanding highly tactical or data-intensive analytics.
Key Takeaways
-
Hudl Sportscode: Ideal for teams requiring a highly customizable, scalable platform with deep workflow integration—perfect for complex, multi-angle tactical sports.
-
Nacsport: Balances versatility with collaboration and emerging real-time features, suitable for teams emphasizing shared analytics and multi-device accessibility.
-
Dartfish: Specializes in AI-driven biomechanical and motion analysis with advanced tools tailored for individual sports and technical coaching.
-
Coach’s Eye: Focuses on ease of use and rapid video feedback, best suited for smaller teams or athletes needing quick post-session insights.
-
Krossover: Automates manual tagging and statistical summaries, serving teams that prefer streamlined video breakdowns without heavy customization.
In conclusion, the choice among these platforms depends on your sport’s tactical complexity, team size, and coaching staff’s technical appetite. No single solution fits all scenarios; understanding each tool’s strengths and limitations enables sports organizations to harness AI’s transformative potential effectively. As AI technologies mature, expect these platforms to deepen integration, enhance real-time feedback, and democratize advanced analytics beyond elite circles.
Platform | Core Functionalities | Technical Specifications | Real-Time Integration | Target Users | Strengths | Limitations |
---|---|---|---|---|---|---|
Hudl Sportscode | Customizable workflows, multi-angle IP video capture, exclusive link toggling, advanced labeling/tagging, telestration, extended timeline trimming, matrix playback | 2025 version with faster scripting, supports local AVFoundation and IP streams, API support for data sync | API integration, live sharing, improved video latency, sensor data synchronization | Elite teams with complex tactical demands | Highly customizable, scalable, deep workflow integration, precise annotation | Steep learning curve, requires dedicated analysts or tech-savvy staff |
Coach’s Eye | Mobile-first video capture, quick annotation, rapid visual feedback | Intuitive interface optimized for mobile; limited depth in workflow integration | Minimal real-time data integration; focused on post-session review | Individual athletes, smaller coaching staffs | Ease of use, fast post-session insights | Limited real-time feedback, less customization |
Dartfish | AI-enhanced video analysis, SimulCam™, biomechanical insights, collaborative tagging, private video hosting | Computer vision, machine learning integration, modular offerings like myDartfish Tennis | AI-powered live analysis, player and puck tracking, near real-time feedback during competition intervals | Individual sports (cycling, tennis), technical coaching | Advanced biomechanical analysis, scalable from grassroots to elite | Requires specialized expertise, less accessible for casual users |
Nacsport | Video tagging, drawing, reporting tools, native Mac support, multi-device compatibility | Integration with external data streams (InStat), Nacsport Hub online collaboration platform | Real-time clip comparison, live metric tracking, collaborative online platform | Teams emphasizing shared analytics and collaboration | Versatile, cross-platform, supports real-time collaboration | Advanced features require setup and training |
Krossover | Automated video breakdown, statistical analysis, manual tagging automation | Streamlined approach targeting resource-constrained teams | Rapid post-game clip generation and statistics summary; no live feedback | Amateur to mid-tier professional teams | Reduces analyst workload, simple and efficient | Less customization, limited tactical analytics |
Practical Applications: Enhancing Coaching, Injury Prevention, and Fan Engagement
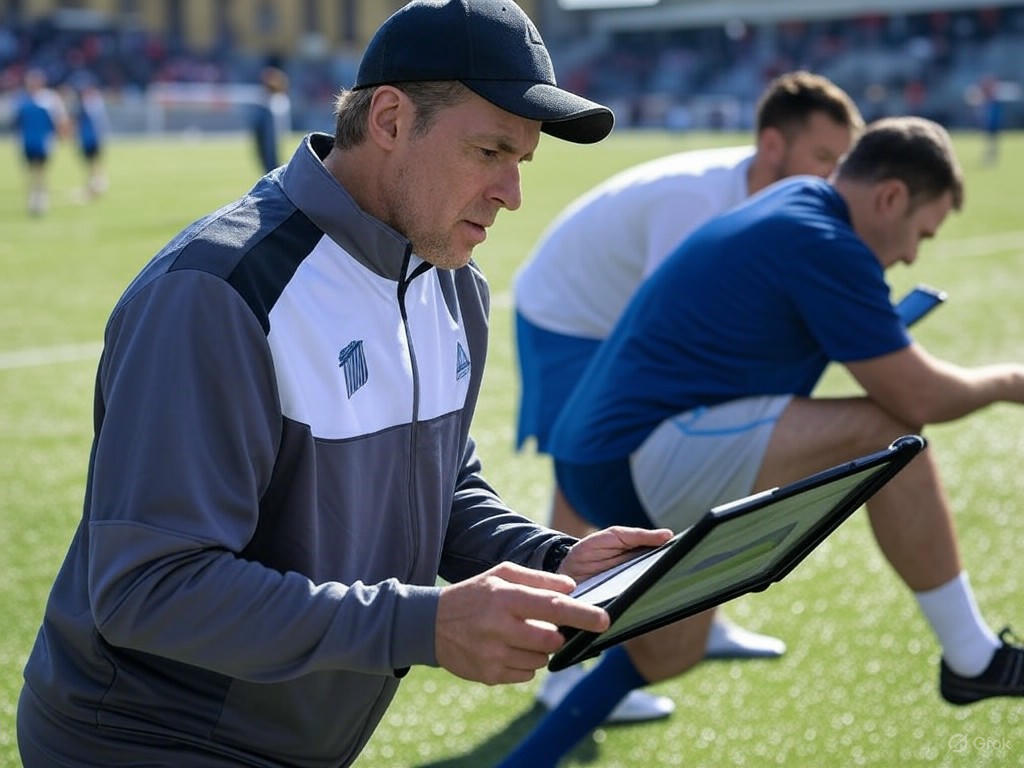
Practical Applications: Enhancing Coaching, Injury Prevention, and Fan Engagement
How exactly is AI reshaping the sports world beyond the buzzwords? Let’s explore concrete ways artificial intelligence is transforming coaching, injury prevention, and the fan experience—three pillars where data-driven insights meet real-world impact.
Smarter Coaching Through Personalization and Tactical Intelligence
Imagine a coaching assistant who never sleeps, continuously learning and adapting to each athlete’s unique needs. That’s the promise AI delivers today. Platforms like Athletica.ai streamline personalized training by syncing with popular devices such as Garmin and Strava, enabling athletes to dynamically adjust workouts based on real-time data. This level of personalization is no longer a luxury but an expectation—especially in endurance sports like running, cycling, and triathlon, where AI-generated training plans demonstrate higher trust and acceptance compared to traditional human-designed programs.
But personalization is just the starting point. Modern AI-powered coaching software is evolving into tactical decision support systems. In football (soccer), for example, AI tools integrate advanced analytics to optimize team strategies, monitor player fitness, and provide real-time insights on game dynamics. These systems simulate alternative plays using game theory algorithms—such as Monte Carlo Tree Search and Alpha-Beta pruning—to suggest optimal moves in fast-paced, complex scenarios.
Moreover, AI’s fusion with augmented reality (AR) and virtual reality (VR) is creating immersive training environments that deepen tactical understanding and enhance skill execution. This multi-layered approach transforms coaching from intuition-driven art into a data-driven science, while still respecting the irreplaceable value of human expertise and judgment.
Predicting and Preventing Injuries with AI-Enabled Athlete Management
Injury prevention has long been a holy grail in sports science. Today, AI revolutionizes this field by consolidating data from wearables, smart apparel, and IoT-connected devices into comprehensive athlete management systems. These platforms monitor not only performance metrics but also subtle biomechanical patterns and player interactions.
A striking example is a machine learning model developed at the University of Delaware, which predicts post-concussion musculoskeletal injury risk with 95% accuracy. This predictive capability enables medical teams to tailor rehabilitation protocols and significantly reduce subsequent injury likelihood.
AI-powered virtual assistants now also guide athletes through rehabilitation exercises remotely, ensuring adherence and optimizing recovery timelines even without continuous physical therapist supervision. These assistants leverage movement pattern recognition and workload monitoring to flag early signs of overtraining or injury risk.
By integrating physiological data, sleep quality, hydration levels, and mental well-being indicators, AI creates a multidimensional picture of athlete health. As of 2025, AI-driven injury prevention is an active practice adopted by elite teams worldwide—particularly in demanding sports like soccer, basketball, and American football, where physical strain is substantial.
Revolutionizing Fan Engagement: Immersive, Personalized, and Ethical
Sports fans increasingly demand personalized, immersive experiences that deepen their connection to teams and players. AI is rising to meet this expectation with a suite of innovative tools.
One prominent application is AI-powered chatbots and virtual assistants that provide instant, personalized responses to fan inquiries—covering ticketing, merchandise, and real-time game statistics. These systems enhance operational efficiency while creating a more engaging, interactive fan environment.
Augmented reality (AR) represents another frontier where AI’s impact is profound. Projected to become a $97 billion market by 2028, AR experiences enable fans—whether in stadiums or at home—to access enriched content such as player stats overlays, immersive replays, and virtual meet-and-greets without distracting from live action. Location-based AR campaigns further encourage year-round venue visits, transforming fan interaction within sports ecosystems.
Personalization extends deeply into content delivery. AI algorithms analyze extensive fan data to tailor highlights, news, and promotions to individual preferences. This targeted approach not only increases fan loyalty but also unlocks new revenue streams for sports organizations.
However, these advances come with significant ethical and privacy challenges. The extensive collection and use of personal data demand transparent policies and strict adherence to emerging regulations such as the European Union’s GDPR and U.S. privacy laws. Additionally, concerns around AI-generated content—including deepfakes—raise issues of intellectual property, authenticity, and trust. Sports entities must carefully navigate this landscape to sustain fan confidence while innovating.
Balancing Innovation with Responsibility
Across coaching, injury prevention, and fan engagement, AI’s practical applications are profound and rapidly expanding. Yet, as an AI architect familiar with both opportunities and pitfalls, I caution against uncritical adoption. The effectiveness of AI hinges on high-quality data, interdisciplinary collaboration, and rigorous ethical oversight.
The sports industry stands at a crossroads where AI can become a powerful multiplier of human skill and connection—or a source of unintended consequences if privacy, fairness, and transparency are neglected. The coming years will be pivotal in establishing standards that maximize AI’s benefits while safeguarding the integrity of sport and the rights of athletes and fans alike.
In summary, AI is no longer a distant concept in sports; it is an active player reshaping how athletes train, recover, and compete, while redefining fan experiences through personalization and immersion. Unlocking AI’s full potential depends on thoughtful integration, transparency, and continuous critical evaluation—principles every stakeholder must embrace in this fast-evolving arena.
Application Area | AI Technologies & Methods | Key Benefits | Examples & Notes |
---|---|---|---|
Coaching Enhancement |
Personalized training platforms (Athletica.ai), integration with Garmin and Strava, Tactical decision support using game theory algorithms (Monte Carlo Tree Search, Alpha-Beta pruning), Augmented Reality (AR) & Virtual Reality (VR) |
Personalized workouts with real-time adjustment, Optimized team strategies and player fitness monitoring, Immersive training environments enhancing tactical skills |
Higher trust in AI-generated training for endurance sports; Real-time game insights in football (soccer); Transformation of coaching into data-driven science while preserving human judgment |
Injury Prevention & Athlete Management |
Data consolidation from wearables, smart apparel, IoT devices, Machine learning predictive models, AI-powered virtual rehabilitation assistants, Multidimensional health monitoring (physiological, sleep, hydration, mental well-being) |
High-accuracy injury risk prediction (e.g., 95% accuracy for post-concussion injuries), Tailored rehabilitation and optimized recovery, Early detection of overtraining and injury risk, Adoption by elite teams in high-strain sports |
University of Delaware model for musculoskeletal injury prediction; Remote guidance for rehabilitation exercises; Active use in soccer, basketball, American football as of 2025 |
Fan Engagement |
AI-powered chatbots and virtual assistants, Augmented Reality (AR) experiences, AI algorithms for personalized content delivery |
Instant personalized responses and interactive fan environments, Immersive AR content (player stats overlays, replays, virtual meet-and-greets), Tailored highlights, news, and promotions increasing loyalty and revenue |
$97 billion projected AR market by 2028; Location-based AR campaigns for venue visits; Ethical/privacy challenges addressed by GDPR, U.S. laws and content authenticity concerns |
Challenges, Limitations, and Ethical Considerations in AI-Driven Sports Analytics
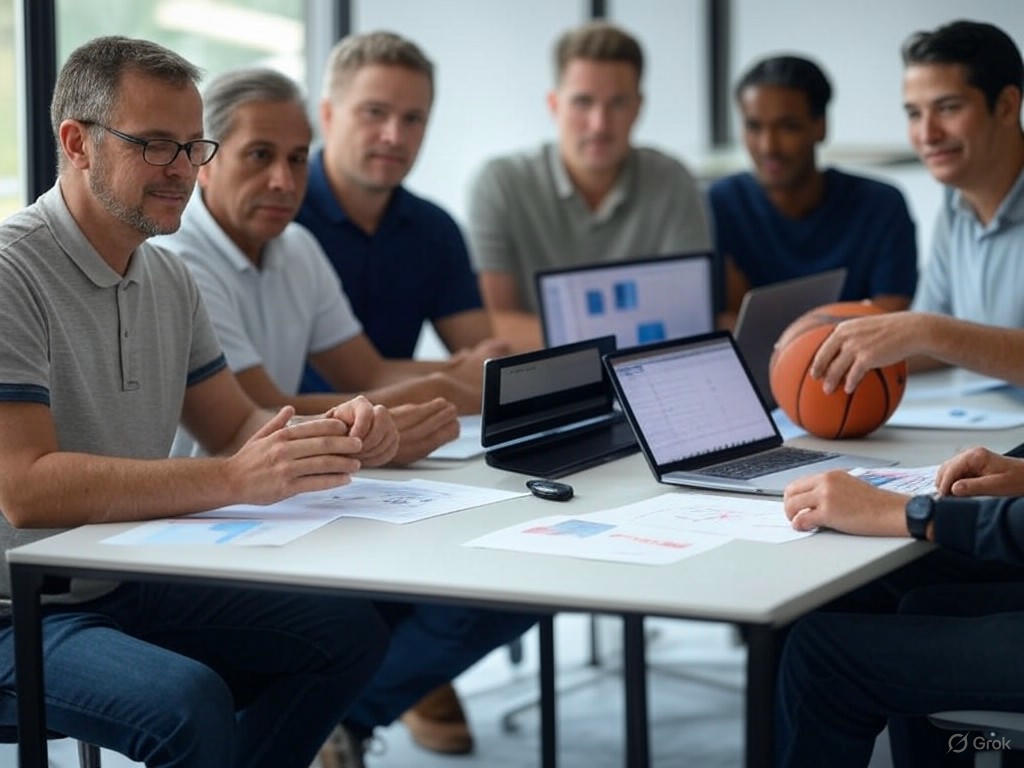
Challenges, Limitations, and Ethical Considerations in AI-Driven Sports Analytics
Artificial intelligence is indeed a transformative force in sports analytics, yet it faces significant challenges when confronted with the complexities of human data and ethical responsibilities. While AI’s potential is vast—from injury prevention to tactical insights—its deployment demands careful scrutiny of both technical and moral dimensions.
Data Biases: The Invisible Fouls in AI Performance Analytics
Data bias remains one of the most subtle yet damaging obstacles undermining AI’s effectiveness in sports. As Shripal Shah of Georgetown University points out, biases often lie hidden within training datasets, skewing AI outputs and potentially perpetuating inequities rather than correcting them.
For example, if historical performance data underrepresents certain demographics—whether by gender, ethnicity, or league—AI models trained on such data may fail to fairly assess or identify talent from those groups. This risk is especially acute in computer vision applications used for athlete identification and rule violation detection. Models trained predominantly on data from one league or demographic can misclassify or overlook others, creating real-world disadvantages in scouting, officiating, and player evaluation.
Moreover, the increasing complexity of AI systems contributes to their “black box” nature. When coaches and athletes receive recommendations without transparent explanations, trust can erode quickly, especially in high-stakes environments. Over-reliance on AI outputs without complementing them with human expertise risks ignoring contextual subtleties that data alone cannot capture. As emphasized in the introduction, AI should serve as a powerful tool augmenting—not replacing—seasoned human intuition.
Athlete Data Privacy and Consent: Guarding the Playing Field of Personal Information
The vast data appetite of AI systems raises critical concerns about athlete privacy and informed consent. Modern athlete management platforms collect extensive personal, physiological, and performance data, offering unprecedented opportunities for personalized training and injury prevention but simultaneously exposing sensitive information to misuse.
Legal frameworks such as the European Union’s GDPR and the U.S. HIPAA set rigorous standards for handling such data, yet many sports organizations lag behind these requirements. Collegiate athletics further complicate matters with evolving NIL (Name, Image, and Likeness) laws, raising questions about data ownership, sharing rights, and commercial exploitation of biometric and performance data.
Informed consent processes in sports are often ill-equipped to address AI’s pervasive, continuous data collection. Athletes must be fully aware of what data is collected, how it’s used, and who accesses it. Experts in sports psychology advocate for more dynamic, context-sensitive consent frameworks that reflect the realities of ongoing AI monitoring.
Cybersecurity threats compound privacy risks. AI systems storing and analyzing athlete data are tempting targets for cyberattacks, which could expose health vulnerabilities or proprietary training insights. Such breaches can affect contracts, sponsorships, and competitive advantage. Given the sophistication of AI-driven analytics, current security protocols must evolve rapidly to safeguard athlete information effectively.
Regulatory and Governance Frameworks: Balancing Innovation with Fairness and Transparency
The regulatory landscape for AI in sports remains fragmented and in flux, struggling to keep pace with rapid technological advances. Gaps persist around data privacy, intellectual property, and fairness, demanding proactive governance.
The European Union’s AI Act exemplifies pioneering legislation, emphasizing safety, transparency, and accountability. It seeks to ensure AI systems deployed in high-stakes domains—including sports—are trustworthy and mitigate bias, thereby protecting athlete careers and public confidence.
Industry voices, such as Zak Mohammed from Databloom Partners, stress that governance models must embed fairness and transparency at their core. While AI enables hyper-personalized experiences, irresponsible or opaque use risks eroding credibility and stakeholder trust.
Emerging AI governance tools provide real-time monitoring for data drift, bias detection, and compliance tracking. For sports organizations, adopting such frameworks transcends legal compliance; it is essential for preserving competitive integrity and public trust.
However, unresolved questions remain, including:
- How should intellectual property and data ownership be handled when AI models train on proprietary sports data?
- What ethical standards should govern predictive analytics in betting and officiating contexts?
- How can regulators balance encouraging innovation with upholding athletes’ rights and fairness?
Addressing these issues requires collaboration among policymakers, technologists, and sports stakeholders to develop clear, adaptable frameworks.
The Human Element: Why AI Should Augment, Not Replace, Human Judgment
Despite AI’s prowess in analyzing vast datasets and detecting patterns beyond human capability, it cannot replicate the nuanced judgment, ethical reasoning, and contextual understanding that coaches, trainers, and athletes bring to the game.
For instance, AI might flag a player as fatigued based on biometric signals, but a coach may perceive subtle behavioral cues or motivational factors that elude sensors. Similarly, AI-generated tactical suggestions must be integrated with considerations of team chemistry, morale, and psychological readiness—elements not easily quantified.
The optimal approach is one of augmentation, where AI insights complement human expertise rather than dictate decisions. This balance preserves the “soul of the game,” ensuring technology enhances rather than diminishes the human spirit and strategic depth intrinsic to sports.
In conclusion, while AI in sports analytics holds transformative promise, realizing its full potential demands vigilant attention to data biases, athlete privacy, and robust governance. Ethical deployment grounded in transparency, fairness, and human collaboration is essential. Only by confronting these challenges head-on can the sports community harness AI’s power responsibly, preserving the integrity and human values at the heart of competition.
Category | Challenges / Issues | Details | Examples / Notes |
---|---|---|---|
Data Biases | Hidden biases in training data | Biases skew AI outputs, perpetuating inequities | Underrepresentation by gender, ethnicity, league; misclassification in computer vision applications |
Data Biases | Lack of transparency (“black box” problem) | Coaches/athletes receive recommendations without explanations, risking trust erosion | Over-reliance risks ignoring contextual subtleties |
Athlete Data Privacy and Consent | Extensive personal data collection | Risks of misuse of sensitive physiological and performance data | Personal, biometric, and performance data collected by AI platforms |
Athlete Data Privacy and Consent | Compliance with legal frameworks | Many organizations lag behind GDPR, HIPAA standards | Complications due to NIL laws affecting data ownership and sharing |
Athlete Data Privacy and Consent | Informed consent inadequacies | Consent processes often not suited for ongoing AI data collection | Need for dynamic, context-sensitive consent frameworks |
Athlete Data Privacy and Consent | Cybersecurity threats | Risk of data breaches exposing health or proprietary info | Potential impact on contracts, sponsorships, competitive advantage |
Regulatory and Governance Frameworks | Fragmented and evolving regulations | Gaps in data privacy, IP, fairness; need for proactive governance | EU AI Act as pioneering legislation |
Regulatory and Governance Frameworks | Need for fairness and transparency | Governance models must embed these principles to maintain trust | Real-time monitoring tools for bias detection and compliance |
Regulatory and Governance Frameworks | Unresolved ethical questions | IP rights, predictive analytics ethics, balancing innovation and fairness | Requires collaboration among policymakers, technologists, sports stakeholders |
Human Element | AI cannot replicate nuanced human judgment | Ethical reasoning, contextual understanding, behavioral cues | Coaches integrate AI insights with team chemistry, morale, readiness |
Human Element | Augmentation over replacement | AI should complement human expertise, preserving the spirit of sport | Ensures technology enhances rather than diminishes strategic depth |
Future Directions: Emerging Trends and the Evolving Role of AI in Sports
Future Directions: Emerging Trends and the Evolving Role of AI in Sports
What unfolds when the raw power of multimodal data converges with the agility of real-time AI coaching and immersive technologies? The sports arena is rapidly transcending traditional statistics and isolated metrics, evolving into an integrated, adaptive ecosystem powered by artificial intelligence. Drawing on over 15 years of experience architecting AI systems, I see this convergence reshaping not only how athletes train and compete but also how fans engage with sports on an unprecedented scale.
Integrating Multimodal Data: The Next Frontier in Performance Analytics
Historically, sports performance analytics has depended on discrete data streams—such as GPS tracking or isolated video analysis. The future, however, lies in synthesizing diverse data types simultaneously to create holistic athlete profiles. Imagine merging high-resolution video feeds with biometric sensors, environmental conditions, and even molecular data like metabolomics to unlock deeper insights.
Key examples include:
-
Wearable sensors equipped with accelerometers, pressure sensors, and bending detectors feed real-time motion data into Artificial Synaptic Neural Networks (ASNNs). These networks adapt efficiently to new data with minimal power consumption, achieving injury risk detection accuracies exceeding 92%. This is critical for wearable tech used during intense training sessions.
-
The Philadelphia 76ers reported a 31% reduction in load-related injuries after implementing AI-based monitoring that integrates wearable biometric data with game footage.
-
In Major League Baseball, AI-driven pitch design algorithms analyzing biomechanics alongside environmental factors such as wind and humidity have increased pitcher velocity by an average of 2.3 mph.
-
The integration of omics sciences adds yet another rich layer. By combining internal physiological metrics—like metabolic profiles and genetic markers—with external workload data captured via GPS and wearables, AI models can tailor training and recovery protocols with unprecedented precision. This fusion transforms “big data” into “smart data,” revealing subtle, often invisible signals that are critical to performance and health.
This multimodal approach moves sports analytics beyond isolated measurements, enabling dynamic, context-aware insights that can adapt as new data streams emerge.
Real-Time Adaptive Coaching and Immersive Technologies: Coaching 2.0
Coaching is evolving from reactive post-session analysis to proactive, real-time adaptive guidance powered by AI. Modern coaching systems leverage continuous data streams and machine learning to provide dynamic feedback during practice and competition.
Notable advancements include:
-
Virtual Reality (VR) training has matured beyond novelty. The NFL’s Washington Commanders credit VR for accelerating their quarterback’s rookie success by simulating high-pressure game scenarios that adjust difficulty based on the player’s performance. VR compresses time and increases repetition, enabling faster development of cognitive skills such as decision-making and situational awareness.
-
AI-powered virtual coaching overlays technique corrections visually, allowing athletes to self-correct instantly. Major leagues—including the NFL, NBA, and Premier League football clubs—have invested heavily in VR infrastructure, reporting measurable gains in both cognitive and physical performance.
-
Augmented Reality (AR) is revolutionizing fan engagement by layering real-time statistics, 360-degree replays, and interactive visualizations directly into the spectator experience. The PGA Tour’s 2025 initiatives include AI-driven course setup tools and shared reality venues that extend fan interaction beyond the stadium.
-
On the broadcast front, AI automates highlight generation and personalizes content delivery based on viewer preferences, transforming passive viewing into immersive, participatory experiences.
This fusion of AI and immersive technologies is redefining the entire sports ecosystem—from training methodologies to fan engagement and media consumption.
Frontier Technologies: Explainable AI and Federated Learning in Sports Analytics
As AI models become increasingly complex, explainability is no longer optional. Coaches and athletes must understand why an AI system makes specific recommendations to build trust and effectively apply these insights.
-
Emerging explainable AI (XAI) frameworks, employing techniques such as knockoff methods, Shapley values, and LIME, are illuminating the inner workings of machine learning models in sports. These approaches provide transparent, actionable explanations rather than opaque, black-box predictions.
-
Federated learning offers a promising solution to balance competitive advantage with data privacy concerns. Instead of centralizing sensitive biometric and performance data, federated learning enables decentralized model training where teams keep their data local and share only aggregated model updates. This approach safeguards proprietary information while building robust AI systems benefitting from a broader knowledge base.
-
The NBA, for example, could leverage federated learning to improve injury prediction models without compromising confidential team data.
This privacy-preserving collaborative paradigm aligns with increasing scrutiny over athlete data privacy and regulatory compliance, fostering an ecosystem where innovation and confidentiality coexist.
Broader Impacts: Competitive Balance, Athlete Health, and the Sports Ecosystem
AI’s influence will reverberate across competitive balance, athlete health, and the broader sports landscape.
-
Competitive Balance: AI-driven analytics and training tools may initially widen the gap between well-funded teams able to invest in cutting-edge technology and those with fewer resources. However, democratization efforts—such as cloud-based platforms and open APIs—hold the potential to level the playing field over time.
-
Athlete Health: AI models predicting post-concussion injury risk with accuracies up to 95% exemplify how technology can enhance athlete wellbeing. By detecting subtle biomechanical compensations and early warning signs invisible to the naked eye, AI enables earlier interventions and personalized recovery plans, potentially extending careers and improving quality of life.
-
Sports Ecosystem: AI-driven fan engagement technologies are transforming how sports are consumed, monetized, and experienced. Immersive broadcasts, targeted content, and interactive experiences forge deeper emotional connections. Yet, these advances raise ethical questions regarding data privacy, psychological effects, and equitable access that the industry must proactively address.
A Note of Caution: Navigating Uncertainties
While the trajectory for AI in sports is exhilarating, it is also marked by significant uncertainties.
-
Model Robustness: Ensuring AI models perform reliably across diverse populations and contexts remains an open challenge.
-
Ethical Use: Responsible handling of sensitive data and transparency in AI decision-making processes are essential to maintain trust.
-
Overreliance Risks: Excessive dependence on AI may risk sidelining human intuition and creativity pivotal to sports.
The most successful AI implementations will augment—not replace—human expertise, empowering coaches, athletes, and fans with deeper insights while preserving the human elements that define sports.
In summary, the coming years will witness AI systems architected not merely for raw predictive power but for seamless integration of multimodal data, real-time adaptability, immersive fan experiences, and transparent, privacy-preserving analytics. This evolving landscape offers a tantalizing glimpse of a sports future that is smarter, safer, and more engaging—provided we navigate its challenges with equal measures of innovation and integrity.
Category | Application / Example | Details / Impact |
---|---|---|
Integrating Multimodal Data | Wearable Sensors with ASNNs | Real-time motion data with injury risk detection accuracy over 92%; efficient, low-power adaptation during intense training |
Philadelphia 76ers AI Monitoring | 31% reduction in load-related injuries using wearable biometric data combined with game footage | |
MLB AI Pitch Design | Pitch velocity increased by 2.3 mph via biomechanical and environmental analysis | |
Omics Integration | Combines metabolic profiles, genetic markers with workload data for tailored training and recovery | |
Real-Time Adaptive Coaching & Immersive Technologies | Washington Commanders VR Training | Accelerated rookie QB success by simulating adjustable high-pressure scenarios |
AI-Powered Virtual Coaching | Visual technique corrections enabling instant athlete self-correction; adopted by NFL, NBA, Premier League | |
PGA Tour 2025 Initiatives | AI-driven course setup and shared reality venues enhancing fan interaction beyond stadium | |
Broadcast AI Automation | Automated highlight generation and personalized content transforming viewer experience | |
Frontier Technologies | Explainable AI (XAI) | Techniques like Shapley values and LIME provide transparent, actionable model explanations |
Federated Learning | Decentralized model training preserving data privacy; example use in NBA injury prediction | |
Privacy & Collaboration | Balances innovation with confidentiality amid increasing data privacy scrutiny | |
Broader Impacts | Competitive Balance | Initial tech disparity vs. democratization efforts via cloud platforms and open APIs |
Athlete Health | AI predicts post-concussion injury risk with up to 95% accuracy enabling early interventions | |
Sports Ecosystem | Immersive fan engagement raises ethical considerations around privacy and equitable access | |
A Note of Caution | Model Robustness | Need for reliable performance across diverse populations and contexts |
Ethical Use | Responsible data handling and transparency essential for trust | |
Overreliance Risks | AI to augment, not replace human intuition and creativity in sports |