AI in Real Estate: Smart Homes and Property Analysis Unveiled
- Introduction: The Convergence of AI and Real Estate – Opportunities and Challenges
- Introduction: The Convergence of AI and Real Estate – Opportunities and Challenges
- The Technological Landscape: Beyond Automation
- Dual Vectors of Transformation: Property Analysis and Smart Homes
- Beyond Capabilities: Ethical Considerations and Real-World Impact
- The Evolving Role of Generative AI Alongside Analytical AI
- Strategic Imperatives for Industry Leaders
- Technical Foundations and Specifications of AI in Real Estate
- Technical Foundations and Specifications of AI in Real Estate
- Machine Learning and Generative AI in Property Analysis
- Computer Vision: Unlocking Visual Intelligence for Property and Smart Homes
- IoT Integration and Hardware-Software Synergy in Smart Homes
- Balancing Innovation with Real-World Constraints
- Performance Metrics and Evaluation of AI Applications in Property Analysis
- Performance Metrics and Evaluation of AI Applications in Property Analysis
- Accuracy in Property Valuation and Predictive Analytics
- Computer Vision: Precision and Robustness in Real Estate Imaging
- Generative AI: Customization and Visualization Capabilities
- Navigating Data Quality and Bias in AI Models
- Key Takeaways
- Smart Home Technology: Design, User Experience, and Innovation
- Smart Home Technology: Design, User Experience, and Innovation
- Architecture and Design Principles Tailored for Real Estate
- User Experience: Adaptability, Privacy, and Interoperability
- Innovative Features: Anomaly Detection, Energy Optimization, and Personalization
- Comparative Analysis: Traditional Real Estate Practices vs. AI-Enhanced Approaches
- Comparative Analysis: Traditional Real Estate Practices vs. AI-Enhanced Approaches
- Efficiency, Error Reduction, and Scalability: From Manual to Machine
- Benchmarking AI Platforms Against Traditional Appraisal and Marketing Methods
- Integration Challenges and Adoption Curves
- Impact on Decision-Making and Stakeholder Roles
- Final Thoughts: Navigating the Transition
- Strengths, Limitations, and Ethical Considerations of AI in Real Estate
- Strengths, Limitations, and Ethical Considerations of AI in Real Estate
- Technical Strengths: Data-Driven Insights and Automation Efficiencies
- Limitations: Interpretability, Privacy, and Bias
- Ethical Considerations: Transparency, Fairness, and Regulatory Compliance
- The Uncertainty of AI Predictions and Unintended Consequences
- Balancing Enthusiasm with Prudence
- Conclusion and Strategic Recommendations for Real Estate Stakeholders
- Conclusion and Strategic Recommendations for Real Estate Stakeholders
- AI’s Transformative Potential and Current Maturity
- Practical Recommendations for Real Estate Firms
- Continuous Evaluation and Adaptability in a Dynamic Environment
- Future Research Directions and Societal Implications

Introduction: The Convergence of AI and Real Estate – Opportunities and Challenges
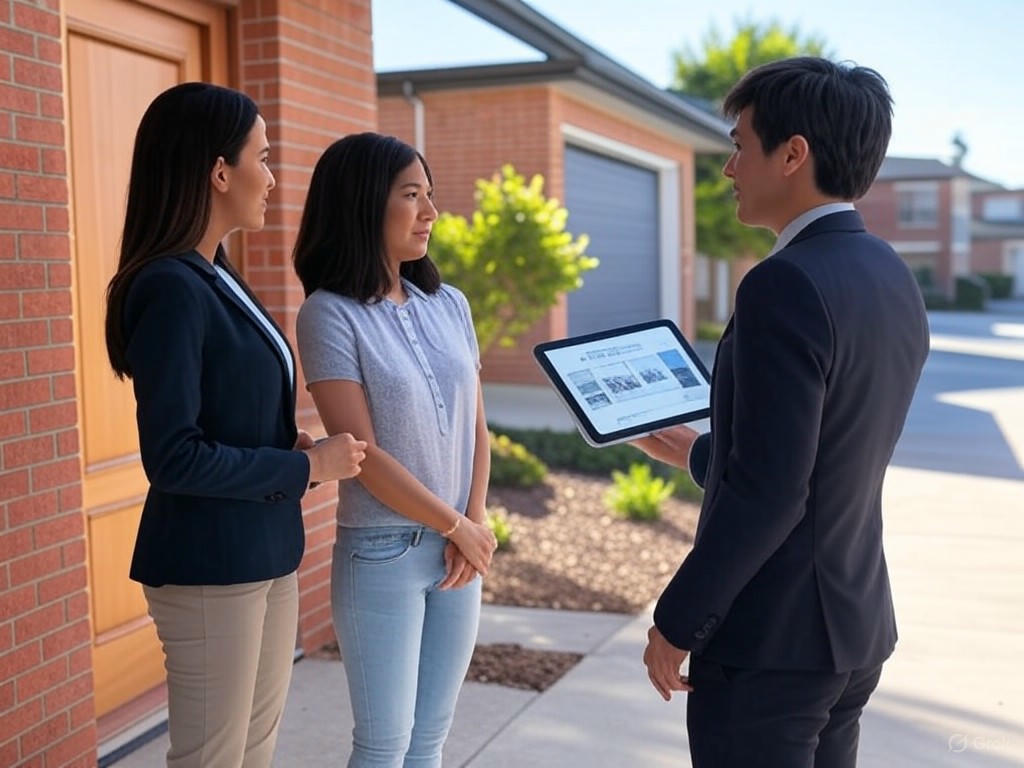
Introduction: The Convergence of AI and Real Estate – Opportunities and Challenges
What happens when one of the world’s most traditional industries intersects with one of today’s most disruptive technologies? The real estate sector, long viewed as slow to evolve, is now experiencing a profound transformation through the integration of artificial intelligence (AI). This shift goes well beyond surface-level hype; it is fundamentally reshaping how properties are analyzed, managed, and experienced. The impact spans economic, social, and ethical dimensions, marking a new era for the industry.
The Technological Landscape: Beyond Automation
AI in real estate is not just about automating repetitive tasks—it is redefining critical processes. Take property analysis, for example: sophisticated AI systems now process vast datasets encompassing market trends, demographics, economic indicators, and satellite imagery to deliver predictive insights that outperform traditional appraisal methods. Brainvire’s 2025 AI trends report highlights how generative AI can anticipate emerging property hotspots well before they enter mainstream awareness, enabling investors to strategically position themselves ahead of market shifts.
At the same time, AI-powered smart home technology combined with Internet of Things (IoT) devices is revolutionizing property management and occupant experience. The smart home market is projected to exceed $190 billion by 2025, with PCMag emphasizing a surge in AI-enabled devices designed to optimize energy efficiency, security, and user comfort. Imagine homes where heating systems adjust dynamically based on occupancy patterns or security cameras detect anomalies in real time. These advancements not only enhance property value but also prompt vital discussions on data privacy and user consent.
Dual Vectors of Transformation: Property Analysis and Smart Homes
AI-driven innovation in real estate converges primarily along two vectors:
-
Property Analysis: Leveraging AI-driven big data analytics and generative AI models, real estate professionals now achieve unprecedented accuracy in market segmentation, risk assessment, and property valuation. Generative AI tools synthesize economic data, local consumer behavior, and historical market trends to produce nuanced investment forecasts. Rentastic’s industry insights suggest that real estate businesses adopting these AI tools can realize net income increases exceeding 10%.
-
Smart Home Technology: On the operational front, AI-integrated smart devices are transforming properties into adaptive ecosystems rather than static assets. Technologies such as predictive maintenance, energy consumption analytics, and personalized environmental controls are becoming expected features in contemporary real estate portfolios. This evolution also redefines property management roles, requiring expertise in integrating and overseeing complex AI-driven systems.
Beyond Capabilities: Ethical Considerations and Real-World Impact
While the technical promise of AI is significant, ethical considerations remain paramount. Questions about who benefits from AI advances are critical. JLL’s research highlights concerns that AI-driven disparities may widen, with smaller market players struggling to keep pace against AI-enabled incumbents, potentially exacerbating inequalities.
Data governance is another pressing challenge. AI models rely on extensive, often personal and sensitive, datasets, raising privacy and consent concerns. Rentastic stresses the imperative for clear data management roles and transparency to establish responsible AI adoption frameworks.
Generative AI introduces additional uncertainties. Its probabilistic predictions depend heavily on data quality and model design. Overreliance on AI-generated forecasts without human oversight risks costly misjudgments. As Forbes contributor Bruce Weinstein observes, balancing AI’s transformative potential with ethical challenges requires careful navigation.
The Evolving Role of Generative AI Alongside Analytical AI
A key development is the rise of generative AI complementing traditional analytical AI. Generative AI models now synthesize narratives, virtual tours, and complex simulations, enhancing property marketing and understanding. DigitalDefynd highlights how generative AI enables immersive 3D virtual tours, reducing the need for physical visits and accelerating transactions while broadening market access.
Concurrently, analytical AI sharpens decision-making frameworks through tasks like lease agreement error detection and predictive maintenance scheduling. The synergy between generative and analytical AI demands strategic foresight from real estate leaders. Fragmented adoption risks inefficiency, whereas cohesive AI strategies can transform entire business models.
Strategic Imperatives for Industry Leaders
Facing these transformative pressures, real estate executives must make critical strategic choices. EY’s analysis of generative AI in commercial real estate urges organizations to develop comprehensive AI strategies that encompass careful use case selection, ethical frameworks, and process re-engineering.
The potential benefits are substantial: McKinsey estimates AI could generate between $110 billion and $180 billion in value for the real estate industry by 2030. Achieving this requires more than technology adoption—it entails cultural shifts, investment in data quality, and vigilant management of unintended consequences.
In summary, the convergence of AI and real estate is a current reality, not a distant prospect. The twin engines of AI-powered property analysis and smart home technologies are driving a new era filled with opportunity and complexity. Successfully navigating this landscape calls for a combination of technical expertise, ethical mindfulness, and strategic clarity—qualities that will define the leaders shaping real estate’s AI-powered future.
Aspect | Details |
---|---|
Industry Impact | Transformation through AI integration in property analysis, management, and experience |
Technological Focus | Beyond automation: predictive insights, AI-driven big data analytics, generative AI, smart home IoT devices |
Property Analysis | Market segmentation, risk assessment, valuation with generative AI; net income increases over 10% |
Smart Home Technology | Adaptive ecosystems with predictive maintenance, energy analytics, personalized controls; market projected >$190B by 2025 |
Ethical Considerations | Concerns on disparities, data privacy, consent, and responsible AI governance |
Generative AI Role | Enables 3D virtual tours, narrative synthesis, marketing enhancement |
Analytical AI Role | Lease error detection, predictive maintenance scheduling, decision support |
Strategic Imperatives | Comprehensive AI strategies, ethical frameworks, cultural shifts, data quality investment |
Economic Potential | Estimated AI value generation of $110B-$180B by 2030 |
Technical Foundations and Specifications of AI in Real Estate
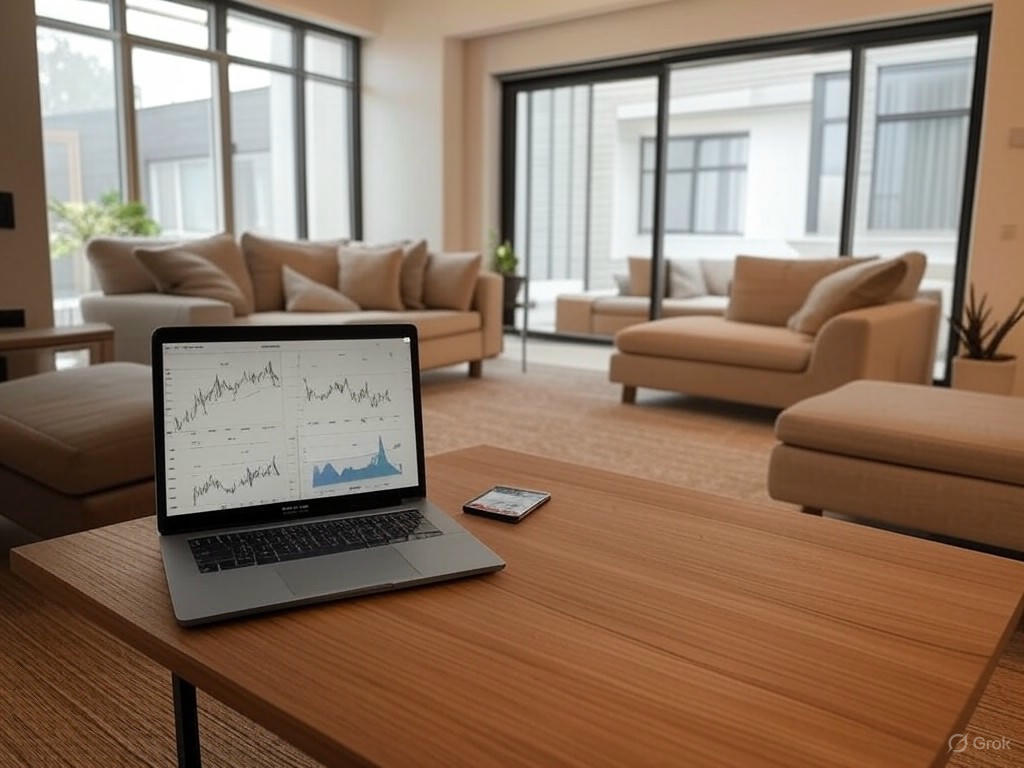
Technical Foundations and Specifications of AI in Real Estate
Understanding what powers today’s AI-driven property analysis and smart home innovations requires delving into a sophisticated ecosystem of machine learning models, advanced computer vision, and tightly integrated IoT systems. These components collectively reshape real estate workflows and occupant experiences with unprecedented precision and adaptability.
Machine Learning and Generative AI in Property Analysis
Property valuation and market trend prediction have evolved well beyond traditional heuristics. At the core of modern AI in real estate are transformer-based generative AI models—architectures akin to large language models but fine-tuned specifically on real estate datasets. These models analyze vast data sources, including historical sales, property features, neighborhood demographics, and economic indicators, to deliver nuanced insights.
Current predictive models incorporate hundreds of features, ranging from straightforward metrics like the number of bedrooms and square footage to subtler factors such as proximity to public transit or school quality. Training datasets often span millions of property records, exemplified by the California housing dataset, which includes over 1,400 samples enriched with census and geospatial data.
Classical machine learning models—such as Support Vector Regression (SVR) with radial basis function kernels and Random Forest algorithms—remain foundational due to their capacity to capture nonlinear relationships and reduce overfitting. These outperform simpler linear regression models in valuation accuracy.
Generative AI extends beyond numeric prediction. It now contributes to creating realistic property descriptions, dynamic market reports, and even architectural design options. Central to these advances is prompt engineering, the art of crafting precise input queries that guide generative models toward relevant, high-quality outputs. In real estate contexts, prompts can instruct AI to generate investment risk assessments or simulate renovation impacts on property value.
Domain-specific fine-tuning has enhanced prompt effectiveness by embedding industry jargon and localized market nuances. This specificity elevates the relevance and practical utility of AI-generated content, supporting stakeholders in making informed decisions.
Computer Vision: Unlocking Visual Intelligence for Property and Smart Homes
AI’s transition from numeric data to visual intelligence marks a pivotal advancement for buyers, sellers, and investors. Computer vision systems analyze property images and videos with remarkable accuracy, extracting detailed information about condition, quality, and architectural style.
A compelling example comes from Restb.ai’s analysis of over 1,200 property appraisals, which uncovered a hidden $27 billion risk stemming from inconsistent condition assessments. Their AI-powered computer vision algorithms automatically parse images to detect discrepancies, helping ensure valuations more accurately reflect a property’s true state.
In smart home environments, computer vision is increasingly deployed at the edge—processing data locally on devices to minimize latency and protect privacy. Vision sensors such as SensoPart’s VISOR integrate multiple detectors with onboard AI, enabling real-time object recognition and anomaly detection.
Smart cameras have matured from passive surveillance tools into intelligent assistants. They can recognize faces, package deliveries, or suspicious activity, often responding within sub-second latencies.
Key technical specifications of these vision-enabled devices include:
- Resolution: Ranging from 1080p to 4K, with AI models optimized for inference efficiency on embedded hardware.
- Processing: Edge AI chips perform inferencing directly on-device, reducing cloud dependency and enabling immediate alerts.
- Connectivity: Low-latency mesh networking protocols like Z-Wave and Thread ensure robust communication among devices.
Beyond individual cameras, AI video analytics platforms now support complex features such as multi-camera tracking, license plate recognition, and behavior prediction. These capabilities are increasingly integrated into residential and commercial properties to enhance security and operational intelligence.
IoT Integration and Hardware-Software Synergy in Smart Homes
The Internet of Things (IoT) serves as the backbone that connects smart devices and AI models into cohesive, interoperable ecosystems. By 2025, global IoT device counts are projected to exceed 40 billion, generating nearly 80 zettabytes of data annually—a volume demanding standardized protocols and rigorous security frameworks.
The Matter standard—endorsed by major players like Amazon, Apple, and Google—addresses interoperability challenges by enabling devices from diverse manufacturers to communicate seamlessly over Wi-Fi, Thread, and Bluetooth Low Energy. This unification promises a user experience free from fragmented apps and complex setups, fostering broader adoption.
Real-time processing is further empowered by 5G networks, which reduce latency and boost automation efficiency. Wellness-oriented IoT devices that monitor air quality, sleep patterns, or emotional well-being exemplify how AI-powered IoT extends beyond convenience into health and lifestyle domains.
Smart home architectures typically comprise layered components:
- Sensors and Actuators: Devices capturing environmental data—motion, temperature, light—and executing commands such as locking doors or adjusting lighting.
- Edge AI Processors: Local inference engines that enable responsiveness and enhance privacy.
- Cloud Platforms: Centralized systems for data aggregation, model retraining, and cross-device orchestration.
- User Interfaces: Mobile applications, voice assistants (e.g., Alexa, Google Assistant), and smart displays offering intuitive control.
Prompt engineering also plays a growing role in smart home customization. Generative AI can create personalized automation scripts or optimize energy usage patterns based on user habits and external data like weather forecasts. As no-code platforms advance, homeowners and real estate professionals gain the ability to tailor AI interactions without deep technical expertise.
Balancing Innovation with Real-World Constraints
Despite impressive technical capabilities, challenges persist in deploying AI-driven real estate solutions. Data quality remains a fundamental bottleneck—many property datasets are incomplete, inconsistent, or biased, which can skew model predictions and exacerbate inequalities.
Privacy concerns loom large, particularly regarding video analytics and pervasive IoT data collection. Transparent policies, user consent mechanisms, and secure architectures are essential to maintaining trust.
Hardware limitations such as sensor battery life and network reliability also affect system performance. Moreover, even with standards like Matter, achieving true interoperability and scalability across a fragmented device market requires continuous coordination.
Nonetheless, these obstacles are surmountable through thoughtful design, regulation, and collaboration. The prospective benefits are compelling: AI foundations enable faster, smarter, and more accurate property analysis, while smart homes evolve into adaptive living environments responsive to occupants’ needs.
In summary, the fusion of transformer-based generative AI, sophisticated computer vision, and interoperable IoT ecosystems forms the technical backbone of AI’s transformative impact on real estate. A detailed understanding of these components equips stakeholders to harness AI’s full potential responsibly, balancing innovation with ethical and operational realities.
Component | Details / Specifications |
---|---|
Machine Learning Models | Transformer-based generative AI fine-tuned on real estate datasets; classical models include Support Vector Regression with RBF kernels and Random Forests |
Features Used in Prediction | Number of bedrooms, square footage, proximity to public transit, school quality, neighborhood demographics, economic indicators |
Training Data | Millions of property records; e.g., California housing dataset with 1,400+ samples plus census and geospatial data |
Generative AI Applications | Property descriptions, market reports, architectural design options, investment risk assessments, renovation impact simulations |
Computer Vision Examples | Restb.ai analysis of 1,200+ property appraisals revealing $27B risk; SensoPart VISOR vision sensors with onboard AI |
Vision-Enabled Device Specifications | Resolution: 1080p to 4K; Processing: Edge AI chips for on-device inference; Connectivity: Z-Wave, Thread mesh networks |
Advanced Vision Features | Multi-camera tracking, license plate recognition, behavior prediction |
IoT Device Projections | 40+ billion devices by 2025; 80 zettabytes data generated annually |
IoT Standards | Matter standard supporting Wi-Fi, Thread, Bluetooth Low Energy for interoperability |
Smart Home Architecture Layers | Sensors and actuators; Edge AI processors; Cloud platforms; User interfaces (mobile apps, voice assistants, smart displays) |
Network Technology | 5G for low latency and automation efficiency |
Privacy and Security Considerations | Data quality challenges, user consent, secure architectures, battery life, network reliability |
Performance Metrics and Evaluation of AI Applications in Property Analysis
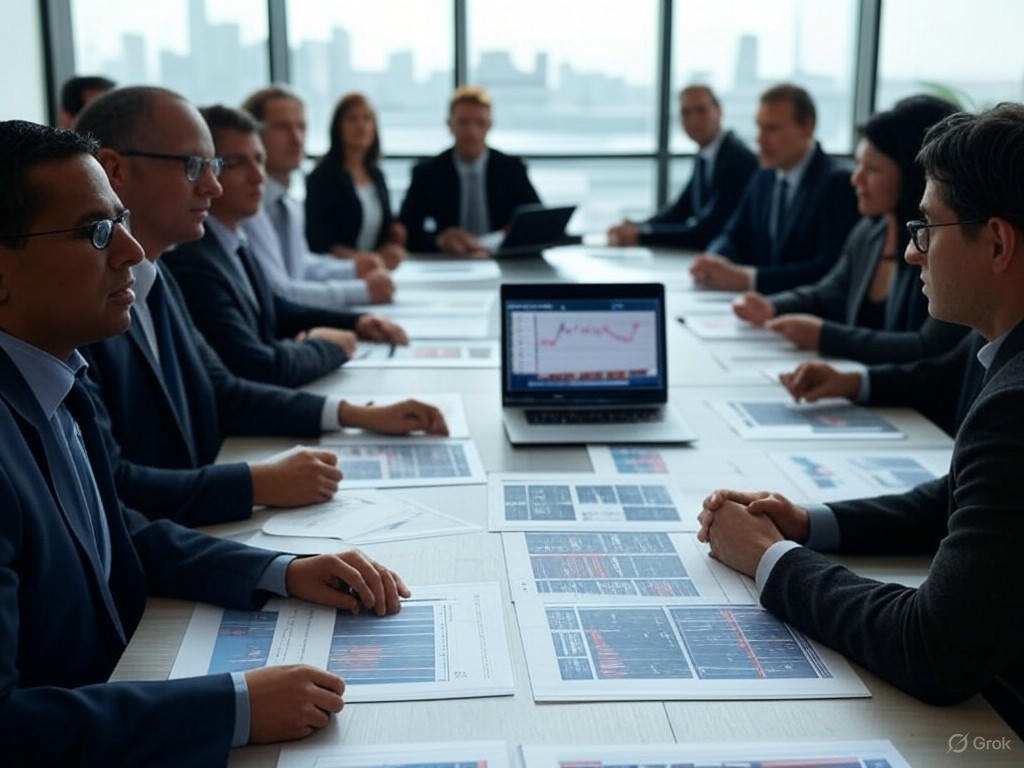
Performance Metrics and Evaluation of AI Applications in Property Analysis
Measuring the success of AI in the traditionally human-centric domain of property valuation and market forecasting requires a rigorous, data-driven approach. Quantitative metrics are essential, but so is a cautious perspective on their inherent limitations.
Accuracy in Property Valuation and Predictive Analytics
The cornerstone metrics for evaluating AI performance in property valuation are Mean Absolute Error (MAE) and Mean Absolute Percentage Error (MAPE). MAE offers a straightforward average of absolute prediction errors, providing practical resilience against market anomalies that can skew other metrics. This makes it especially suited to real estate, where unique property characteristics and local fluctuations are common.
Industry research highlights significant advances in AI-driven Automated Valuation Models (AVMs). For example, artificial neural networks (ANNs) deployed in markets like Surabaya, Indonesia, have consistently outperformed traditional regression models, achieving notably lower MAE values across various property types. This demonstrates AI’s strength in capturing complex, nonlinear relationships in property data that classical hedonic models often overlook.
Further, predictive analytics powered by machine learning contributes to operational gains. According to Number Analytics, firms leveraging AI for early detection of market shifts and property-specific risks report a 5-7% increase in operating incomes. These improvements, however, depend heavily on robust data quality, thoughtful variable selection, and the integration of alternative data sources—ranging from social media signals to transportation developments—to enrich model inputs.
Model latency—the delay between data input and prediction output—is critical for applications demanding real-time responsiveness, such as dynamic pricing and investment decisions. Advances in AI infrastructure, including the use of edge computing and optimized inference engines, have significantly reduced latency. This enables more timely price adjustments that better align with current market conditions.
Computer Vision: Precision and Robustness in Real Estate Imaging
Visual data is increasingly pivotal in real estate, and AI-powered computer vision is unlocking new capabilities in image analysis. Leading solutions now deliver high image recognition precision, accurately identifying property conditions, amenities, and subtle quality distinctions from photographs.
A compelling example is Restb.ai’s analysis of over 1,200 property appraisals, which uncovered $27 billion in hidden financial risks due to inconsistent condition and quality assessments—risks often missed in traditional appraisals. Restb.ai’s AI algorithms automatically extract scalable, actionable insights from images, showcasing computer vision’s transformative role in risk mitigation.
Precision, however, is only part of the equation. Robustness against data variability remains a significant challenge. Real estate images vary widely—in lighting, angles, resolution, and staging quality—requiring AI models to generalize effectively across these inconsistencies. While current models manage common variability well, edge cases and poor-quality images can still cause errors or biases. This underscores the importance of diverse training datasets and continuous model validation.
Moreover, privacy and ethical considerations add complexity. As computer vision technologies become more pervasive in monitoring properties and tenants, organizations must navigate strict data protection laws and ethical frameworks to ensure transparency, fairness, and user trust.
Generative AI: Customization and Visualization Capabilities
Generative AI is emerging as a powerful tool for property customization and visualization, reshaping how buyers and investors engage with real estate listings. AI-generated virtual staging, tailored floor plans, and immersive walkthroughs allow stakeholders to visualize spaces far beyond static images.
For instance, Zillow’s Zestimate integrates AI-generated valuation scores directly into listings, enhancing buyer confidence. Tools like Collov automate virtual staging and personalized floor plan creation, while AI-powered virtual assistants deliver customized customer interactions. These capabilities accelerate decision-making and deepen buyer engagement.
Nonetheless, generative AI has its constraints. Output quality heavily depends on the diversity and relevance of training data. Overly generic or unrealistic visualizations risk misleading buyers. Additionally, customization engines must carefully balance creative flexibility with adherence to architectural standards and regulatory constraints—a complex technical and ethical challenge.
Navigating Data Quality and Bias in AI Models
The foundation of effective AI in real estate is high-quality data. Property data is sourced from a heterogeneous mix—public records, MLS databases, sensor readings, and social media—each varying in accuracy and completeness.
Bias embedded in training datasets poses a silent but serious threat. Historical data reflecting systemic inequalities or market distortions can cause AI models to perpetuate these biases, impacting price predictions, tenant screening, and lead generation. For example, regions with less digital footprint or lower-quality images may receive unfairly suboptimal valuations or recommendations.
Addressing these issues requires robust testing frameworks and continuous monitoring. The industry’s gradual adoption of standardized evaluation protocols—including fairness audits and scenario-based testing—is encouraging but still nascent within real estate.
Key Takeaways
-
AI property valuation models demonstrate measurable accuracy gains, with reductions in MAE and enhanced predictive analytics supporting smarter investment decisions. However, the complexity of real-world markets demands ongoing validation and refinement.
-
Computer vision significantly enhances appraisal precision and risk detection, but must overcome challenges related to image variability, privacy, and ethical considerations to ensure reliable, fair outcomes.
-
Generative AI enriches property customization and visualization, improving buyer experience and engagement, yet requires careful calibration to prevent misleading or noncompliant outputs.
-
Data quality and bias remain critical challenges, necessitating transparent, ethical AI development practices and rigorous testing to uphold fairness, accuracy, and trust.
In conclusion, AI is reshaping property analysis and smart home technologies by combining technical sophistication with ethical stewardship. Its true impact depends on integrating rigorous performance evaluation, diverse data sources, and responsible governance—ensuring AI serves as a transformative partner rather than a blind oracle in real estate.
AI Application Area | Performance Metrics / Outcomes | Challenges / Considerations |
---|---|---|
Property Valuation and Predictive Analytics | Mean Absolute Error (MAE), Mean Absolute Percentage Error (MAPE); 5-7% increase in operating incomes reported; lower MAE values vs traditional models | Data quality, variable selection, alternative data integration; ongoing model validation; market complexity |
Computer Vision in Real Estate Imaging | High image recognition precision; discovery of $27 billion hidden financial risks via image analysis | Image variability (lighting, angles, resolution, staging); robustness to poor-quality images; privacy and ethical concerns |
Generative AI for Customization and Visualization | AI-generated virtual staging, tailored floor plans, immersive walkthroughs; enhanced buyer confidence (e.g., Zillow Zestimate) | Output quality dependent on training data diversity; risk of misleading or unrealistic visuals; balancing creativity with architectural and regulatory standards |
Data Quality and Bias | Use of heterogeneous data sources (public records, MLS, sensors, social media); emerging standardized evaluation protocols | Bias perpetuation from historical data; fairness audits and scenario testing still nascent; transparency and ethical AI development needed |
Smart Home Technology: Design, User Experience, and Innovation
Smart Home Technology: Design, User Experience, and Innovation
What happens when a smart home evolves from a collection of isolated gadgets into a truly intelligent ecosystem? The answer lies in the architecture and design principles driving AI-powered smart homes today—principles that prioritize adaptability, privacy, and seamless integration within real estate environments.
Architecture and Design Principles Tailored for Real Estate
By 2025, the global smart home market is projected to reach $135 billion, with over 500 million connected households expected by 2028. This surge is propelled by AI’s capability to make home systems more intuitive and responsive, alongside the widespread rollout of 5G networks enabling real-time, low-latency communication among devices.
In real estate, this shift transforms homes from static assets into dynamic environments that anticipate occupant needs and adjust automatically. Forward-thinking designers and architects are integrating AI early in the home planning process. Platforms like Aalaya empower homeowners to visualize and customize smart technologies through interactive 3D models, ensuring automation is embedded from the outset rather than retrofitted.
This integration extends well beyond convenience. Energy efficiency and occupant wellness are now central design considerations, reflecting growing concerns about climate change and health. The smart home of 2025 is less about standalone devices and more about an orchestrated network: wellness monitors that track air quality and sleep, smart offices facilitating remote work, and intelligent systems learning and adapting to family routines.
Think of this architecture as analogous to a well-trained AI model—layers of sensors, processors, and communication protocols working harmoniously to deliver a living experience that is personalized, efficient, and secure.
User Experience: Adaptability, Privacy, and Interoperability
The ultimate measure of AI-powered smart home success is the user experience. Systems must operate effortlessly, adapting dynamically to occupants’ lifestyles without requiring constant manual input. This adaptability is enabled by AI models that learn behavior patterns—such as wake-up times, preferred evening temperatures, and room occupancy schedules.
Privacy remains a paramount concern. Smart homes collect vast amounts of sensitive data, including movement patterns and energy usage, which must be safeguarded against unauthorized access. Leading privacy practices now include:
- Customizable privacy settings that adjust data sharing based on time of day or room.
- Network segmentation to isolate smart home devices from other household networks.
- End-to-end encryption securing data in transit and at rest.
From a user perspective, these controls need to be transparent and straightforward. Complex security measures should not compromise usability. Much like tuning hyperparameters in an AI model for optimal performance, privacy settings require careful calibration to balance convenience with protection.
Interoperability poses another significant challenge. The smart home market is highly fragmented, with hundreds of device types and brands that often do not communicate seamlessly. The vision is a cohesive ecosystem in which devices work harmoniously regardless of manufacturer. Achieving this demands open standards and active industry collaboration, such as the Matter standard endorsed by Amazon, Apple, and Google.
Until this vision is realized, homeowners may juggle multiple apps or hubs, reminiscent of early AI systems that required bespoke integrations for each new capability.
Innovative Features: Anomaly Detection, Energy Optimization, and Personalization
Among the most compelling innovations in AI-powered smart homes are real-time anomaly detection systems. These function like sentinel AI agents, continuously monitoring for unusual events—unauthorized entry, sudden water leaks, or abnormal appliance behavior. Advances in edge AI enable data processing locally on devices, reducing latency and enhancing privacy.
A promising approach combines complementary machine learning models, such as Isolation Forest algorithms and Long Short-Term Memory (LSTM) autoencoders, to detect anomalies accurately and efficiently. This mirrors frontier AI models that blend different neural architectures to balance precision and computational cost. Immediate anomaly detection helps prevent costly damage or security breaches, providing peace of mind well beyond traditional alarm systems.
Energy optimization algorithms represent another vital innovation. With rising energy costs and environmental concerns, smart homes increasingly manage consumption proactively. Techniques like Model Predictive Control and Reinforcement Learning optimize heating, cooling, and appliance usage by predicting occupant behavior and integrating renewable energy sources.
Imagine the smart home as a miniature city grid with its own demand-response system. AI learns when to draw power, store energy, or reduce consumption—much like an advanced AI balancing competing objectives under constraints. The result is a home that lowers its environmental footprint and reduces utility bills without sacrificing occupant comfort.
Personalized environment adjustments form the final layer in this AI symphony. From temperature and lighting shifts calibrated to mood or activity, to smart textiles and bedding that regulate temperature and improve sleep quality, AI tailors living spaces intimately to each resident. This level of customization echoes advances in frontier AI models that dynamically adapt outputs to user preferences, blurring the boundary between technology and lifestyle.
In summary, AI-powered smart homes in real estate are evolving into truly intelligent environments: architected with foresight, designed for seamless user interaction, and enriched with innovative features that enhance security, efficiency, and personalization. Nonetheless, addressing privacy concerns and overcoming interoperability challenges remain critical. As with any emerging AI frontier, progress requires a careful balance—between enthusiasm and skepticism, innovation and responsibility. This balance defines the promise and puzzle of smart home technology today.
Category | Details |
---|---|
Market Projection | Global smart home market projected to reach $135 billion by 2025; over 500 million connected households expected by 2028 |
Design Principles | Adaptability, privacy, seamless integration, energy efficiency, occupant wellness |
Architecture Features | Integrated AI, 5G-enabled real-time communication, interactive 3D customization platforms (e.g., Aalaya) |
User Experience | Dynamic adaptation via AI behavioral learning, customizable privacy settings, network segmentation, end-to-end encryption |
Privacy Practices | Time or room-based data sharing controls, device network isolation, encrypted data transmission and storage |
Interoperability Challenges | Fragmented device ecosystems; solution via open standards like Matter supported by Amazon, Apple, Google |
Innovative Features | Anomaly detection (Isolation Forest, LSTM autoencoders), energy optimization (Model Predictive Control, Reinforcement Learning), personalized environment adjustments |
Anomaly Detection | Real-time local edge AI processing for security and damage prevention |
Energy Optimization | Predictive energy management integrating renewable sources, demand-response balancing |
Personalization | Temperature, lighting, smart textiles, and bedding tailored to user preferences and activities |
Comparative Analysis: Traditional Real Estate Practices vs. AI-Enhanced Approaches
Comparative Analysis: Traditional Real Estate Practices vs. AI-Enhanced Approaches
What happens when the longstanding reliance on gut instinct and manual spreadsheets gives way to AI algorithms analyzing millions of data points in seconds? The real estate industry is in the midst of this transformative shift. For decades, property analysis and management depended heavily on human expertise, paper documentation, and localized knowledge. Today, AI-driven workflows are not just accelerating these processes—they are fundamentally redefining how value is assessed, decisions are made, and properties are managed.
Efficiency, Error Reduction, and Scalability: From Manual to Machine
Traditional property analysis typically requires appraisers to visit sites, compare recent sales, and manually adjust valuations based on neighborhood nuances. While grounded in experience, this approach is time-consuming and susceptible to subjective bias. For example, appraisers may overlook subtle market shifts or emerging risks such as climate vulnerabilities, which are increasingly critical to accurate property valuations (MSCI, 2025).
AI-powered models like Zillow’s Zestimate and Redfin’s appraisal tools have changed the landscape. These platforms process vast datasets—including historical sales, neighborhood trends, and even social behavior metrics—to generate property valuations with median error rates as low as 1.99% on active listings (The Close, 2025). Achieving this level of precision with traditional methods would require substantial manpower and time.
Beyond accuracy, AI delivers dramatic improvements in speed and scalability. Whereas manual analyses can take days or weeks, AI systems process thousands of properties in real time. This agility enables investors and property managers to swiftly respond to market dynamics, optimizing portfolio allocation and mitigating risk. According to Number Analytics, AI users identify investment opportunities nearly three times faster than those relying solely on conventional analysis.
Moreover, AI reduces human errors common in data entry, oversight, or fatigue. Automated workflows flag anomalies, predict maintenance needs through sensor data, and streamline routine tasks such as tenant communication and rent collection (SoftKraft, 2025). These reductions in error translate directly to cost savings and higher tenant satisfaction.
Benchmarking AI Platforms Against Traditional Appraisal and Marketing Methods
Comparing Zillow’s Zestimate with traditional appraisals highlights the balance between data-driven speed and nuanced human judgment. Zillow aggregates data from multiple sources, applying machine learning to estimate property values. However, its accuracy depends heavily on data quality and completeness. In markets with sparse listing details or hyper-local factors—such as Rhode Island’s micro-markets—traditional agents maintain an advantage (WhatsUpNewp, 2025).
Redfin’s AI-enhanced appraisal tools leverage direct MLS access and proprietary matchmaking algorithms, delivering comparable median error rates of 1.99% on active listings (The Close, 2025). However, Redfin’s model requires agents within its brokerage network, limiting accessibility compared to Zillow’s more open platform.
Marketing has also been revolutionized by AI. Platforms now automatically enhance property photos, generate immersive virtual tours using AR/VR technologies, and personalize buyer recommendations through natural language processing. These innovations create engaging, tailored experiences that go far beyond static photos and traditional open houses. The impact is measurable: listings with AI-enhanced imagery sell faster and command higher prices (API4AI, 2025).
Still, these advances come with trade-offs. Traditional marketing heavily depends on agent-client relationships, local market expertise, and trust—intangibles that AI cannot fully replicate. The key challenge lies in integrating AI tools without diminishing these essential human elements.
Integration Challenges and Adoption Curves
Despite clear benefits, adopting AI in real estate is not a plug-and-play endeavor. Many firms face difficulties integrating AI into existing systems due to legacy technology silos and inadequate data infrastructure (V7 Labs, 2025). Furthermore, the AI hype cycle often prompts organizations to pilot multiple tools without a coherent strategy, leading to fragmented workflows and limited return on investment (JLL, 2025).
Successful AI adoption demands a cultural shift, emphasizing data literacy and redefining professional roles. Rather than replacing experts, AI reshapes their work. Agents and appraisers increasingly act as interpreters of AI-generated insights, focusing on strategic decision-making while machines manage data processing and routine operations (Number Analytics, 2025).
Adoption rates also vary among stakeholders:
- Investors gain from accelerated, data-backed asset selection.
- Agents leverage AI for lead scoring, personalized marketing, and dynamic pricing.
- Property managers utilize AI for tenant matching, predictive maintenance, and operational automation.
Smaller firms and independent agents may encounter barriers due to costs and technical expertise gaps. As the World Economic Forum emphasizes, democratizing AI knowledge is essential for equitable, widespread adoption.
Impact on Decision-Making and Stakeholder Roles
AI’s influence extends beyond efficiency, fundamentally transforming decision-making processes. Traditional decisions often rely on intuition and experience. AI introduces predictive analytics that uncover market trends, migration patterns, and climate risks often missed by human analysis (MSCI, 2025; Leni.co).
For instance, AI can dynamically adjust property prices based on real-time market signals—a capability impossible with conventional static appraisals (Brainvire, 2025). Tenant matching algorithms improve occupancy rates and reduce turnover by analyzing behavioral and financial data (Rapid Innovation, 2025).
This shift requires new competencies for real estate professionals, including data interpretation, ethical AI use, and transparent communication about algorithmic decisions. Maintaining trust hinges on explainability, especially as AI models grow more complex and opaque.
Final Thoughts: Navigating the Transition
The contrast between legacy real estate practices and AI-enhanced workflows is pronounced. AI offers:
- Dramatic efficiency gains,
- Reduced errors,
- Scalability across portfolios,
- Enhanced marketing impact,
- And data-driven decision-making.
However, realizing these benefits requires thoughtful integration that balances machine intelligence with human judgment. As with any frontier technology, it is vital to temper expectations with awareness of AI’s limitations—biases in training data, privacy concerns, and the necessity of ongoing oversight.
For stakeholders willing to invest in infrastructure, skills, and ethical frameworks, AI provides a pathway to a more responsive, transparent, and efficient real estate ecosystem. Those relying solely on traditional methods risk falling behind in a market increasingly shaped by data and automation.
In sum, the AI revolution in real estate is not about replacing expertise but amplifying it—transforming scattered data into actionable insights and repetitive tasks into strategic opportunities.
Aspect | Traditional Real Estate Practices | AI-Enhanced Approaches |
---|---|---|
Property Analysis Method | Manual site visits, comparison of recent sales, subjective neighborhood adjustments | AI algorithms processing millions of data points including sales, trends, social metrics |
Accuracy | Subject to human bias and oversight, may miss emerging risks like climate vulnerabilities | Median error rates as low as 1.99% on active listings (Zillow, Redfin) |
Speed & Scalability | Days or weeks for analysis, limited by manpower | Real-time processing of thousands of properties, investment opportunities identified nearly 3x faster |
Error Reduction | Prone to data entry mistakes, oversight, fatigue | Automated anomaly detection, predictive maintenance, error minimization |
Marketing | Static photos, open houses, reliant on agent-client trust and local expertise | AI-enhanced photos, AR/VR virtual tours, personalized recommendations; faster sales, higher prices |
Platform Accessibility | Available to all agents, dependent on agent expertise | Zillow: open platform; Redfin: limited to brokerage network agents |
Integration Challenges | Established workflows, legacy systems | Requires data literacy, cultural shift, overcoming legacy silos, coherent AI strategy needed |
Stakeholder Benefits | Investors, agents, property managers rely on experience and manual processes | Investors: accelerated asset selection; Agents: lead scoring, dynamic pricing; Property Managers: tenant matching, automation |
Decision-Making | Based on intuition and experience | Predictive analytics for market trends, dynamic pricing, tenant matching algorithms |
Human Role | Expert judgment and manual data processing | Interpreters of AI insights, strategic decision-makers, ethical AI use and communication |
Limitations & Considerations | Bias from human error, limited data processing capacity | Bias in training data, privacy concerns, need for ongoing oversight and explainability |
Strengths, Limitations, and Ethical Considerations of AI in Real Estate
Strengths, Limitations, and Ethical Considerations of AI in Real Estate
What makes AI a true game-changer in real estate, and where do challenges arise? The promise of AI is clear: smarter property analysis, automation of repetitive tasks, and more personalized tenant and homeowner experiences. However, beneath these benefits lie technical, ethical, and regulatory complexities that require careful examination.
Technical Strengths: Data-Driven Insights and Automation Efficiencies
AI’s primary advantage in real estate is its capacity to analyze vast and diverse datasets rapidly and with precision. According to Rentastic and other industry analysts, AI adoption can increase net income by over 10% for real estate businesses by 2025. McKinsey projects that AI and data analytics could help the industry grow beyond $1 billion by 2032.
In property valuation, AI models integrate historical sales data, market trends, economic indicators, and even social media sentiment to generate dynamic pricing and investment recommendations. Unlike traditional appraisal methods, which rely heavily on manual comparisons and local intuition, AI uncovers subtle market signals invisible to human analysts.
Automation further accelerates real estate operations. AI-powered CRMs streamline customer interactions and lead management, while augmented reality–enhanced virtual tours improve client engagement and shorten sales cycles. Tenant screening powered by AI can instantly verify backgrounds, detect potential fraud, and forecast rental payment risks, transforming a once labor-intensive process into an efficient, data-driven workflow.
Smart home technology exemplifies AI’s role beyond analysis. Devices like Roborock’s AI-driven vacuum cleaners and LG’s moisture-sensing dryers optimize home maintenance and energy consumption by learning user habits and environmental conditions. These AI-enabled appliances improve comfort, reduce utility costs, and contribute to sustainability goals embraced by 63% of new homes.
Limitations: Interpretability, Privacy, and Bias
Despite these gains, significant limitations temper AI’s impact. One critical issue is model interpretability. Many AI systems operate as “black boxes,” producing recommendations without transparent reasoning. This opacity undermines trust and complicates accountability, especially for high-stakes decisions such as tenant screening or investment analysis.
Data privacy concerns are equally pressing. AI’s reliance on large datasets often involves sensitive personal and financial information. Legal frameworks like the California AI Transparency Act mandate disclosures and bias audits, but real estate companies must implement robust governance to protect data and comply with evolving regulations. Frost Brown Todd and Snappt emphasize the complexity of navigating these requirements.
Bias in AI models is a documented problem with tangible consequences. Studies in Sun Belt states reveal that tenant screening algorithms disproportionately disadvantage Black and Latinx applicants, perpetuating existing housing inequalities. Without rigorous oversight, AI risks reinforcing systemic disparities rather than mitigating them.
Ethical Considerations: Transparency, Fairness, and Regulatory Compliance
The intersection of technical challenges and ethical imperatives demands deliberate action. Transparency is essential: tenants, buyers, and investors must understand how AI influences decisions impacting their lives. For instance, California’s AI labeling laws require clear disclosures when AI-generated content affects consumer interactions, setting important precedents for fairness.
Fairness requires inclusive data practices, ongoing bias audits, and human oversight to align AI systems with legal standards such as the Fair Housing Act. Landlords relying solely on AI for tenant screening face legal risks unless ethical guidelines and supervisory mechanisms are integrated.
The regulatory environment is rapidly evolving but remains fragmented. As reported by Berkeley Research Group, fewer than half of companies feel confident in their AI compliance. This highlights the imperative for real estate firms to invest in internal safeguards, staff training, and collaborative governance frameworks to navigate complex legal landscapes effectively.
The Uncertainty of AI Predictions and Unintended Consequences
AI’s predictive abilities offer significant value but come with inherent uncertainty. Real estate markets are influenced by unpredictable factors—geopolitical events, economic shocks, and social trends—that AI models may not fully capture. JLL underscores the challenges of forecasting, indicating that AI-generated predictions should be approached with caution.
Unintended consequences also emerge from automation and AI-driven decision-making. For example, overreliance on AI tenant screening can speed approvals but may unjustly exclude qualified renters if underlying data or algorithms are flawed. Similarly, automation may streamline transactions but risks deskilling human agents and eroding personalized client relationships.
Balancing Enthusiasm with Prudence
The transformative potential of AI in real estate is vast—enhancing operational efficiency, enabling smarter investments, and enriching smart home ecosystems. Yet, this revolution is not without pitfalls. Industry leaders must champion transparency, demand explainability, safeguard privacy, and actively mitigate bias.
Only through this balanced approach can AI’s promise be realized responsibly, ensuring technology serves communities and individuals as well as business objectives. The path forward is clear: embrace AI’s strengths while rigorously addressing its limitations and ethical dimensions to harness its power with both confidence and conscience.
Category | Details |
---|---|
Technical Strengths |
|
Limitations |
|
Ethical Considerations |
|
Uncertainty & Unintended Consequences |
|
Balanced Approach |
|
Conclusion and Strategic Recommendations for Real Estate Stakeholders
Conclusion and Strategic Recommendations for Real Estate Stakeholders
What does artificial intelligence truly offer the real estate industry today, and how can firms harness its power responsibly? After a thorough examination of AI’s role in property analysis and smart home technology, it is evident that AI is no longer a distant vision but a tangible force reshaping real estate. This transformation presents remarkable opportunities alongside complex challenges that require careful navigation.
AI’s Transformative Potential and Current Maturity
By 2025, AI’s influence in real estate spans a broad spectrum of applications with growing depth. Predictive analytics refine property valuations in real time, while AI-powered virtual assistants streamline tenant interactions, enabling smarter and faster decisions. For instance, AI tools now identify off-market deals and foreclosure opportunities, providing investors with competitive advantages unattainable through manual methods alone.
However, AI maturity is uneven across domains. Dynamic pricing engines and automated valuation models (AVMs) have become established tools, with platforms like Zillow’s Zestimate and Redfin achieving median error rates as low as 1.99% on active listings. In contrast, integrating AI into smart home ecosystems remains in early stages of sophistication. Devices such as Moen’s Flo smart water sensors and AI-enhanced smart locks are gaining adoption but still face hurdles related to user acceptance and interoperability.
The real estate sector is beginning to see measurable financial benefits. Industry forecasts, including those from McKinsey Global Institute, estimate AI could generate between $110 billion and $180 billion in value for real estate by 2030, with net incomes potentially increasing by over 10%. Yet, these gains come alongside growing pains—from data privacy concerns to the technical challenges of integrating AI with legacy infrastructures.
Practical Recommendations for Real Estate Firms
To remain competitive and ethically responsible in this AI-infused landscape, real estate firms must establish a strategic, data-centric roadmap. Key priorities include:
-
Data Governance & Quality: Implement clear accountability for data integrity, privacy, and regulatory compliance. High-quality, curated data is the foundation for reliable AI outputs and trustworthy insights, as emphasized by Rentastic and Frost Brown Todd.
-
Master Prompt Engineering: As generative AI tools become embedded in customer relationship management (CRM) platforms and tenant engagement solutions, proficiency in prompt engineering unlocks more precise and actionable results. Structured prompts—such as specifying AI roles or contexts—enhance relevance and minimize errors.
-
Responsible AI Governance: Develop comprehensive frameworks balancing innovation with ethical oversight. Define AI principles, establish continuous monitoring, and ensure transparency. Gartner forecasts that by 2026, half of governments will enforce responsible AI mandates, making proactive governance a strategic imperative.
-
Foster Cross-Disciplinary Collaboration: Successful AI adoption requires partnership among technology providers, real estate professionals, and policymakers. Collaboration helps address technical, ethical, and legal challenges holistically rather than in isolated silos.
Continuous Evaluation and Adaptability in a Dynamic Environment
AI capabilities and regulatory landscapes evolve rapidly, often unpredictably. Real estate organizations must embed continuous evaluation and adaptability into their AI strategies to keep pace:
-
Monitor Regulatory Changes: Stay abreast of updates in lending requirements, zoning laws, data privacy regulations such as the California AI Transparency Act, and emerging AI compliance standards.
-
Implement Feedback Loops: Regularly assess AI system performance, bias, and unintended consequences. Vigilance prevents costly errors and builds stakeholder trust.
-
Invest in Workforce Upskilling: Prepare for AI-augmented roles by developing data literacy and AI governance skills. With 40% of the real estate workforce nearing retirement, cultivating new talent fluent in AI technologies is vital.
Future Research Directions and Societal Implications
Looking forward, several areas warrant focused research to unlock AI’s potential while safeguarding societal interests:
-
Interoperability in Smart Homes: Investigate how AI systems across diverse devices and manufacturers can create seamless, secure user experiences without compromising privacy. The Matter standard and federated learning architectures are promising avenues.
-
AI Explainability and Trust: Develop explainable AI (XAI) models that transparently articulate decision-making processes. This is critical for regulatory compliance and fostering client confidence, as seen in emerging ethical AI frameworks.
-
Environmental Impact: Address sustainability concerns arising from AI’s computational demands, especially in data centers. Innovations in energy-efficient hardware and green technologies must be prioritized.
-
Social Equity: Ensure AI systems do not exacerbate housing inequalities or discrimination. Rigorous bias mitigation, ethical frameworks, and adherence to standards like the Fair Housing Act are essential.
In summary, AI in real estate is a double-edged sword—offering unprecedented analytical power and operational efficiency, yet requiring thoughtful stewardship. Stakeholders who combine technical expertise with ethical foresight, anchored by robust data governance and adaptive frameworks, will lead this transformation responsibly.
The journey is complex, but with deliberate strategy and continuous learning, AI’s promise can be realized in ways that benefit businesses, consumers, and society alike.
Category | Key Points | Details / Examples |
---|---|---|
AI’s Transformative Potential and Current Maturity | Applications & maturity levels | Predictive analytics for valuations; AI virtual assistants; Dynamic pricing engines and AVMs like Zillow Zestimate (1.99% median error); Early-stage smart home AI (Moen’s Flo sensors, AI smart locks) |
Financial Impact | Forecasted value and challenges | $110B-$180B value by 2030; net incomes +10%; challenges include data privacy, legacy system integration |
Data Governance & Quality | Data integrity and compliance | Clear accountability; regulatory compliance; high-quality curated data (Rentastic, Frost Brown Todd) |
Master Prompt Engineering | Effective use of generative AI | Embedding in CRM and tenant engagement; structured prompts for precision and relevance |
Responsible AI Governance | Ethical frameworks and oversight | AI principles; continuous monitoring; transparency; Gartner predicts half governments enforce mandates by 2026 |
Cross-Disciplinary Collaboration | Partnerships for holistic adoption | Technology providers, real estate pros, policymakers addressing technical, ethical, legal challenges |
Continuous Evaluation and Adaptability | Monitoring and flexibility | Track regulatory changes (e.g., California AI Transparency Act); feedback loops for bias and errors; workforce upskilling for AI roles |
Future Research Directions | Key focus areas | Smart home interoperability (Matter standard); AI explainability (XAI); environmental sustainability; social equity and bias mitigation |