AI in Media & Entertainment: Transforming Creation and Analytics
- Introduction: Why AI Is a Game-Changer in Media and Entertainment
- Introduction: Why AI Is a Game-Changer in Media and Entertainment
- The Dual Engine: Innovation Meets Ethical Complexity
- Revolutionizing Creative Workflows: Amplifier, Not Replacement
- Redefining Distribution and Audience Engagement
- Navigating Ethical Challenges in a Rapidly Evolving Landscape
- Looking Ahead: Balancing Enthusiasm with Caution
- Foundations of AI Technologies in Media Content Creation
- Foundations of AI Technologies in Media Content Creation
- Generative Models: GANs and Transformers
- Neural Creativity and Procedural Generation Techniques
- Limitations: Bias, Hallucination, and Computational Demands
- Wrapping Up the Technical Foundations
- AI-Driven Audience Analytics: Algorithms, Data, and Insights
- AI-Driven Audience Analytics: Algorithms, Data, and Insights
- Data Sources: Beyond Traditional Viewership Metrics
- Machine Learning Models: Personalization, Segmentation, and Feedback Loops
- Case Study: Netflix’s Recommendation Engine
- Challenges: Navigating Privacy, Transparency, and Interpretability
- Balancing Innovation with Responsibility
- Redefining Creative Collaboration: Human-AI Synergy and Authorship
- Redefining Creative Collaboration: Human-AI Synergy and Authorship
- From Linear to Iterative: How AI Transforms Creative Workflows
- Evolving Authorship and Intellectual Property in the AI Era
- Case Studies: Ethical and Practical Dimensions of Shared Creative Agency
- Key Takeaways
- Operational and Business Impacts: Efficiency, Risk, and Industry Adoption
- Operational and Business Impacts: Efficiency, Risk, and Industry Adoption
- Streamlining Media Operations: From Contracts to Talent and Copyright
- Productivity Gains vs. Liability Risks: What Industry Surveys Reveal
- Adoption Patterns: Major Studios vs. Indie Creators
- AI SaaS Tools: Seamless Integration into Media Workflows
- Balancing Optimism with Caution
- Comparative Analysis of AI Content Creation Tools and Platforms
- Comparative Analysis of AI Content Creation Tools and Platforms
- Generative AI Models: Balancing Output Quality, Customization, and Scalability
- Image and Video Processing APIs: Performance and Workflow Compatibility
- AI-Powered Audience Analytics Suites: Data Accuracy, Real-Time Insights, and Integration
- Wrapping Up: Choosing the Right Tool for the Job
- Future Trajectories: Emerging Trends, Ethical Frontiers, and Societal Implications
- Future Trajectories: Emerging Trends, Ethical Frontiers, and Societal Implications
- Virtual Beings as Autonomous Content Creators
- Federated Learning: Privacy-Preserving AI in Media
- Progress Toward Artificial General Intelligence (AGI) in Entertainment
- Ethical Frontiers: Authenticity, Inclusivity, and Data Governance
- Societal Implications: Employment, Cultural Production, and Audience Interaction
- Navigating Uncertainty with Evidence-Based Strategies
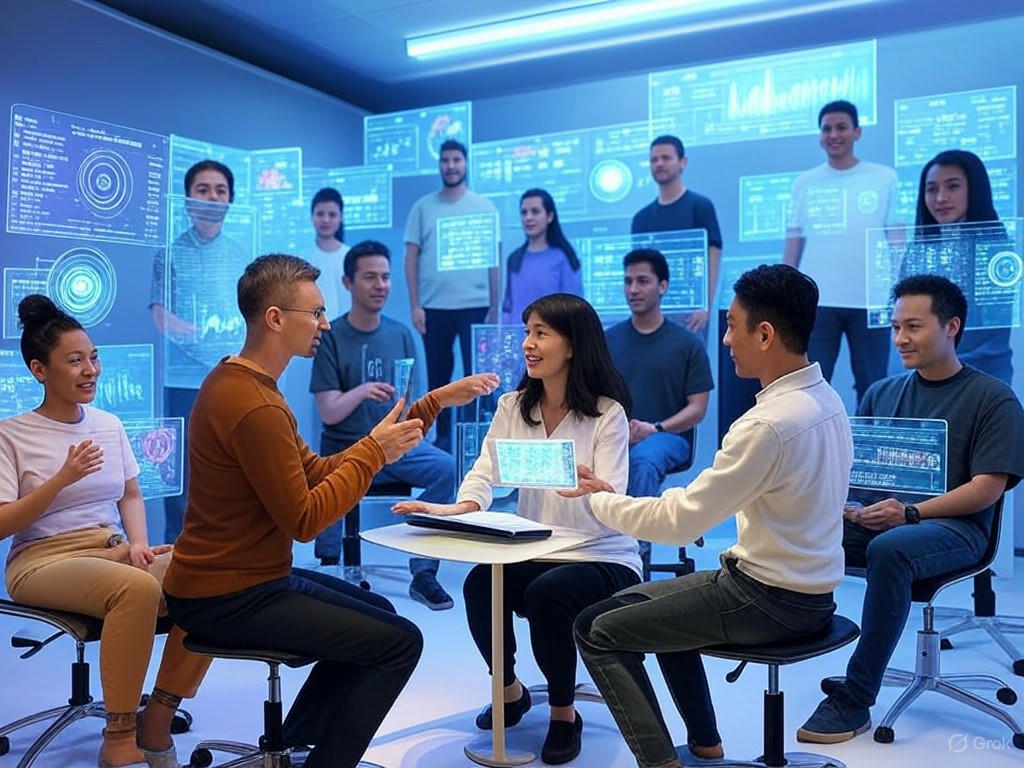
Introduction: Why AI Is a Game-Changer in Media and Entertainment
Introduction: Why AI Is a Game-Changer in Media and Entertainment
Imagine your favorite movie or playlist not only crafted by human creativity but co-created with intelligent systems that learn and adapt to your preferences in real time. By 2025, artificial intelligence has evolved beyond novelty to become a fundamental force reshaping media and entertainment—from igniting the creative spark in content generation to delivering granular, data-driven audience analytics.
The Dual Engine: Innovation Meets Ethical Complexity
AI’s transformative impact in media operates across two tightly linked domains: content creation and audience insight.
-
Content Creation: Generative AI tools like Leonardo and ElevenLabs’ Suno empower creators to produce text, images, music, and video at unprecedented speed and personalization. These platforms enable artists and marketers to generate B-roll footage, background music, and even virtual avatars starring in videos tailored for niche audiences.
-
Ethical Complexities: This surge in AI-generated content raises pressing questions about intellectual property, authenticity, and data privacy. High-profile legal cases involving AI-generated replicas of public figures highlight the urgent need for clear frameworks balancing creativity with rights protection. As Pablo Rivas, Ph.D., from Baylor University’s Center on Responsible Artificial Intelligence and Governance, emphasizes, AI development entails not only technical but also moral and spiritual dimensions that demand thoughtful stewardship.
Revolutionizing Creative Workflows: Amplifier, Not Replacement
Rather than replacing human creativity, AI acts as an amplifier within creative workflows. Content creator Charles Ross notes that professionals thriving today are those who harness AI to enhance their creative agency.
Key AI tools include:
- ChatGPT: Simulates reader reactions to drafts, helping writers refine content.
- Notion AI: Assists in evolving ideas through iterative brainstorming.
- Midjourney: Generates aesthetically compelling artwork and instant scene mock-ups using advanced GANs.
These tools accelerate iteration, broaden creative directions, and reduce manual workloads. However, AI currently excels primarily at short-form content and concept generation. High-quality, long-form storytelling still relies on human nuance and judgment.
Redefining Distribution and Audience Engagement
AI’s influence extends into content distribution and consumption:
-
Predictive Analytics and Cookieless Targeting: Marketers leverage AI to analyze behavioral patterns—such as shopping habits and preferred communication channels—without relying on traditional cookies, aligning with evolving privacy standards.
-
Streaming Services and Broadcasters: AI-powered tools optimize ad placement and tailor content recommendations, driving a 12% year-over-year growth in connected TV ad spend.
-
Niche Content Providers: Smaller players can now compete by identifying underserved markets and customizing offerings, leveling the playing field against tech giants and streaming behemoths.
-
AI-Driven Virtual Influencers: On platforms like Instagram and TikTok, AI-generated digital personas engage audiences 24/7 with dynamic AR filters, helping brands maintain relevance in crowded digital spaces.
Navigating Ethical Challenges in a Rapidly Evolving Landscape
The rapid adoption of AI demands robust ethical safeguards. Industry discourse increasingly focuses on:
- Bias in AI Algorithms: Biased training data can perpetuate stereotypes or marginalize groups.
- Data Privacy: Ensuring compliance with regulations such as CCPA and GDPR is critical.
- Transparency: Explainable AI (XAI) frameworks help demystify AI decision-making and build user trust.
Regulatory responses include U.S. laws criminalizing malicious use of synthetic media and ongoing debates at copyright offices worldwide regarding intellectual property protections for AI-generated works. Collaborative efforts among technologists, policymakers, and creatives are vital to establish standards that safeguard fairness, privacy, and artistic integrity.
Looking Ahead: Balancing Enthusiasm with Caution
AI is not a magic solution to all challenges in media and entertainment. Its integration requires careful calibration—embracing AI’s efficiency and personalization benefits while critically addressing risks and limitations.
As this article explores specific applications and implications, remember that the true game-changer is not AI alone but the thoughtful stewardship with which the industry wields it. AI is reshaping media into a dynamic ecosystem where technology and human creativity coexist, with the next frontier defined by ethical frameworks and strategic vision guiding AI deployment.
Aspect | Description |
---|---|
Content Creation Tools | Leonardo, ElevenLabs’ Suno – Generate text, images, music, video; ChatGPT, Notion AI, Midjourney – Enhance creative workflows |
Ethical Complexities | Intellectual property concerns, authenticity, data privacy, legal cases on AI replicas, moral stewardship |
Creative Workflow Role | AI as amplifier, not replacement; accelerates iteration; excels in short-form and concept generation |
Distribution & Engagement | Predictive analytics, cookieless targeting, AI-optimized ad placement, niche content customization, AI-driven virtual influencers |
Ethical Challenges | Bias in algorithms, data privacy compliance (CCPA, GDPR), transparency via explainable AI, regulatory frameworks |
Future Outlook | Balanced integration of AI benefits with risk management; emphasis on ethical frameworks and strategic stewardship |
Foundations of AI Technologies in Media Content Creation
Foundations of AI Technologies in Media Content Creation
What powers the AI tools reshaping media content today? At their core, many of these advances rely heavily on generative models—especially Generative Adversarial Networks (GANs) and transformer architectures—that have evolved dramatically over the past decade. A clear understanding of these foundational technologies reveals both their vast creative potential and their intrinsic limitations.
Generative Models: GANs and Transformers
Generative Adversarial Networks (GANs) are a compelling neural architecture consisting of two competing networks: the generator and the discriminator. The generator creates new data samples—such as images, audio, or video—while the discriminator evaluates their authenticity. This adversarial training process refines the generator’s output, enabling GANs to produce photorealistic images and complex visual effects that often appear indistinguishable from real-world content.
For instance, tools like Midjourney utilize GANs to generate deepfake-quality images used in marketing, gaming, and entertainment. Advanced variants like CycleGANs perform image translation without paired examples, enabling style transfers and domain adaptation. However, GAN training is notoriously unstable and requires careful hyperparameter tuning, coupled with massive datasets, to achieve convergence.
In contrast, transformer models—originally developed for natural language processing—have become the backbone of AI’s ability to generate coherent text, scripts, and even music. These models employ self-attention mechanisms, which weigh the relevance of all parts of an input sequence to generate each output token. This mechanism allows transformers to capture long-range dependencies effectively.
Large language models like GPT and multimodal models such as DALL·E extend this capability, producing text, images, and combinations thereof with impressive fluency and coherence. Transformers excel in creative applications like AI-assisted scriptwriting, generating dialogue, plot outlines, or complete scenes based on prompts. Their training typically involves unsupervised learning on vast corpora of text or paired image-text datasets, demanding enormous computational resources and requiring diligent bias management.
Neural Creativity and Procedural Generation Techniques
How do AI systems transcend mere replication and approach genuine creativity? In media, this often manifests through neural creativity and procedural generation techniques that enable dynamic, adaptive content creation responsive to user inputs or environmental factors.
In scriptwriting, generative AI models analyze narrative structures and character archetypes to produce drafts that human writers can refine. This collaborative approach boosts productivity—studies indicate teams using AI generate up to 68% more content while reducing production time by approximately 45%. Yet, AI serves as a creative partner rather than an autonomous author; human oversight remains vital to infuse emotional depth and cultural context.
Music composition has advanced through procedural generation powered by evolutionary algorithms, rule-based systems, and increasingly, neural networks trained on extensive musical datasets. These AI models generate melodies, harmonies, and rhythms that adapt in real time—especially in video games, where soundtracks shift dynamically according to player actions or narrative developments. Middleware platforms like Wwise and FMOD facilitate seamless integration of AI-generated music into game engines, supporting dynamic scoring.
Visual effects (VFX) production benefits from AI’s ability to automate repetitive tasks such as rotoscoping and simulate complex phenomena like smoke, fire, or fluids. GANs and deep learning models generate synthetic textures, enhance resolution, and animate characters from minimal inputs. This accelerates production timelines and reduces costs significantly, addressing bottlenecks common in traditional workflows.
Limitations: Bias, Hallucination, and Computational Demands
A balanced technical foundation requires acknowledging AI’s critical limitations. Bias is a persistent challenge: GANs and transformers trained on skewed datasets often reproduce and amplify stereotypes in generated images or text. For example, image generation platforms have been observed defaulting to stereotypical gender or occupational roles unless deliberately corrected.
Another significant issue is hallucination, where AI confidently produces false or nonsensical information. This problem is especially acute in scriptwriting and content generation, where fabricated facts can undermine trust, necessitating rigorous human fact-checking. Some OpenAI models reportedly hallucinate over 50% of the time on specific benchmarks, underscoring the need for cautious deployment.
Moreover, the computational cost of training and deploying these models is substantial. Large-scale transformer training demands petaflops of processing power and datasets comprising hundreds of thousands to millions of examples. The financial and environmental costs are considerable, often limiting accessibility to well-resourced organizations and raising sustainability concerns.
Wrapping Up the Technical Foundations
From the adversarial interplay of GANs to the attention-driven dynamics of transformers, the AI technologies underpinning media content creation are complex yet incredibly powerful. They enable unprecedented creative workflows—automating routine tasks, generating novel content, and augmenting human creativity.
However, these technologies are not magical solutions. They require vast data, meticulous tuning, and critical human oversight to mitigate bias, hallucination, and resource constraints. As AI continues to evolve, understanding its architectures, training paradigms, and limitations is essential for creators and consumers alike.
Only with this clarity can the media and entertainment industry responsibly harness AI’s transformative potential, balancing innovation with ethical and practical considerations.
AI Technology | Description | Applications in Media | Challenges |
---|---|---|---|
Generative Adversarial Networks (GANs) | Two competing networks (generator and discriminator) creating and evaluating data samples to produce photorealistic images and complex visual effects. | Deepfake-quality images, style transfer, domain adaptation, visual effects automation, synthetic textures, character animation. | Unstable training, requires massive datasets and hyperparameter tuning, bias in generated content. |
Transformer Models | Self-attention mechanisms weigh relevance in input sequences for coherent text, scripts, music, and multimodal content generation. | AI-assisted scriptwriting, dialogue & plot generation, large language models (GPT), multimodal models (DALL·E). | High computational resources needed, bias, hallucination, requires large-scale datasets. |
Neural Creativity & Procedural Generation | AI models dynamically create content responsive to inputs and environment using neural networks, evolutionary algorithms, and rule-based systems. | Scriptwriting drafts, collaborative writing, adaptive music composition in games, dynamic scoring, automated VFX tasks. | Human oversight needed for emotional and cultural context, integration complexity. |
Limitations | Bias, hallucination, and computational demands inherent in AI models. | Bias amplification in images/text, false or nonsensical content production, high financial and environmental costs. | Requires careful dataset curation, fact-checking, and resource-intensive training. |
AI-Driven Audience Analytics: Algorithms, Data, and Insights
AI-Driven Audience Analytics: Algorithms, Data, and Insights
What fuels the remarkable accuracy of Netflix’s recommendation engine or the pinpoint-targeted ads that follow you across social media? At the core of these capabilities is a sophisticated system of AI-driven audience analytics—an intricate fusion of extensive data ingestion, advanced machine learning models, and continuous real-time feedback loops.
Beneath these seemingly magical insights lies a complex ecosystem of diverse data sources, algorithmic strategies, and ethical considerations that merit close examination.
Data Sources: Beyond Traditional Viewership Metrics
Today’s audience analytics go far beyond simple viewership counts. Streaming platforms and media companies collect and analyze a broad spectrum of data points to build a comprehensive understanding of their audiences:
- Viewership metrics: Fundamental quantitative data includes time spent watching, pause and rewind behaviors, completion rates, device types, and geographic locations.
- Social media signals: Platforms like TikTok, Instagram, and Twitter offer a rich, real-time pulse of audience sentiment through likes, shares, comments, and trending hashtags. For instance, TikTok users spend an average of 47.3 minutes daily on the platform, making it a valuable source for trend and sentiment analysis.
- Engagement benchmarks: Metrics such as comment frequency, shares, and impressions capture active audience participation, signaling deeper emotional or intellectual connections beyond passive consumption.
- Demographic and psychographic data: Insights into age groups, interests, and behavioral tendencies enable nuanced segmentation that transcends broad categorical profiling.
By 2025, AI-powered platforms no longer rely solely on retrospective data analysis. Instead, real-time analytics have become standard, allowing for immediate adjustments in marketing campaigns and content recommendations. This dynamic feedback mechanism continuously sharpens audience targeting and engagement strategies.
Machine Learning Models: Personalization, Segmentation, and Feedback Loops
How do these vast and varied data streams translate into hyper-personalized user experiences? The answer lies in machine learning models engineered to segment audiences and tailor content at near real-time speeds.
- Personalization engines: Platforms like Netflix deploy collaborative filtering and deep learning techniques to recommend shows based on both individual preferences and patterns observed in similar users. This hybrid approach captures explicit tastes alongside implicit behavioral signals.
- Segmentation models: AI clusters users into meaningful groups by analyzing complex patterns across multiple dimensions—viewing frequency, genre affinity, time of day, and even emotional sentiment inferred from social media chatter.
- Predictive analytics: Leveraging historical data, these models anticipate content likely to engage specific audience segments, optimizing not only recommendations but also marketing promotions and pricing strategies.
- Real-time feedback loops: Continuous ingestion of user interactions and social responses enable AI systems to update and refine models promptly. This “closed-loop learning” ensures recommendations evolve in tune with shifting audience preferences and trends.
For example, McKinsey highlights generative AI’s role in crafting marketing copy tailored to distinct subgroups, enhancing resonance and conversion rates. Similarly, AI marketing platforms such as Salesforce Einstein GPT synthesize extensive data to deliver highly personalized outreach, showcasing AI’s augmentation of human creativity and decision-making.
Case Study: Netflix’s Recommendation Engine
Netflix is a prime example of AI-driven audience analytics in action. Its recommendation system processes billions of data points daily—from viewing histories and search queries to the time spent browsing.
The engine employs matrix factorization combined with neural networks to generate deeply personalized content suggestions. This approach is credited with driving over 75% of viewer activity on the platform, underscoring its impact.
Moreover, Netflix integrates real-time feedback mechanisms. For instance, if a viewer consistently skips certain recommended content, the algorithm adapts by deprioritizing similar options. This dynamic responsiveness keeps recommendations relevant and aligned with evolving viewer tastes, exemplifying the power of continuous model refinement.
Challenges: Navigating Privacy, Transparency, and Interpretability
Despite impressive technical achievements, deploying AI in audience analytics introduces significant challenges that must be addressed thoughtfully:
- Data privacy and consent: Research reveals a persistent gap between data collection practices and meaningful user consent. Nearly half of tracking technologies continue data harvesting even after opt-out requests, violating privacy norms and risking regulatory sanctions. Cisco’s 2025 Data Privacy Benchmark Study reports that 86% of consumers support privacy legislation, emphasizing the urgent need for ethical data stewardship.
- Data quality and compliance risks: “Dirty data”—data of uncertain or poor quality—can skew model outputs, leading to unreliable insights and flawed business decisions. This poses legal liabilities under tightening regulations such as GDPR and CCPA, making rigorous data governance indispensable.
- Model transparency and trust: Deep learning models powering personalization are often “black boxes,” with decision-making processes opaque to users and stakeholders. Explainable AI (XAI) initiatives seek to illuminate these mechanisms, fostering trust and enabling human oversight. Without transparency, users may distrust AI-driven recommendations, undermining adoption and acceptance.
- Accuracy versus interpretability trade-offs: More complex models typically deliver higher accuracy but sacrifice explainability. In media analytics, striking a balance is crucial. For example, a simple decision tree may be easier to interpret but less precise than a deep neural network. Organizations must align model choices with their priorities—whether maximizing predictive power or ensuring compliance and user trust.
Balancing Innovation with Responsibility
The rapid growth of AI-powered audience analytics is revolutionizing media and entertainment. Hyper-personalized experiences delight consumers while driving business growth and operational efficiency.
However, this progress demands a parallel commitment to ethical stewardship. Transparent data governance, clear mechanisms for user consent, and ongoing attention to fairness and accountability are essential.
As AI systems become deeply embedded across content ecosystems, media companies must engage multidisciplinary teams—ethicists, legal experts, data scientists—to navigate complex ethical, legal, and operational dimensions.
Ultimately, AI-driven audience analytics is as much about upholding human values as it is about refining algorithms. The future belongs to those who harness AI’s power responsibly, maintaining the trust and respect of their audiences while embracing innovation.
Category | Details |
---|---|
Data Sources |
|
Machine Learning Models |
|
Netflix Case Study |
|
Challenges |
|
Balancing Innovation & Responsibility |
|
Redefining Creative Collaboration: Human-AI Synergy and Authorship
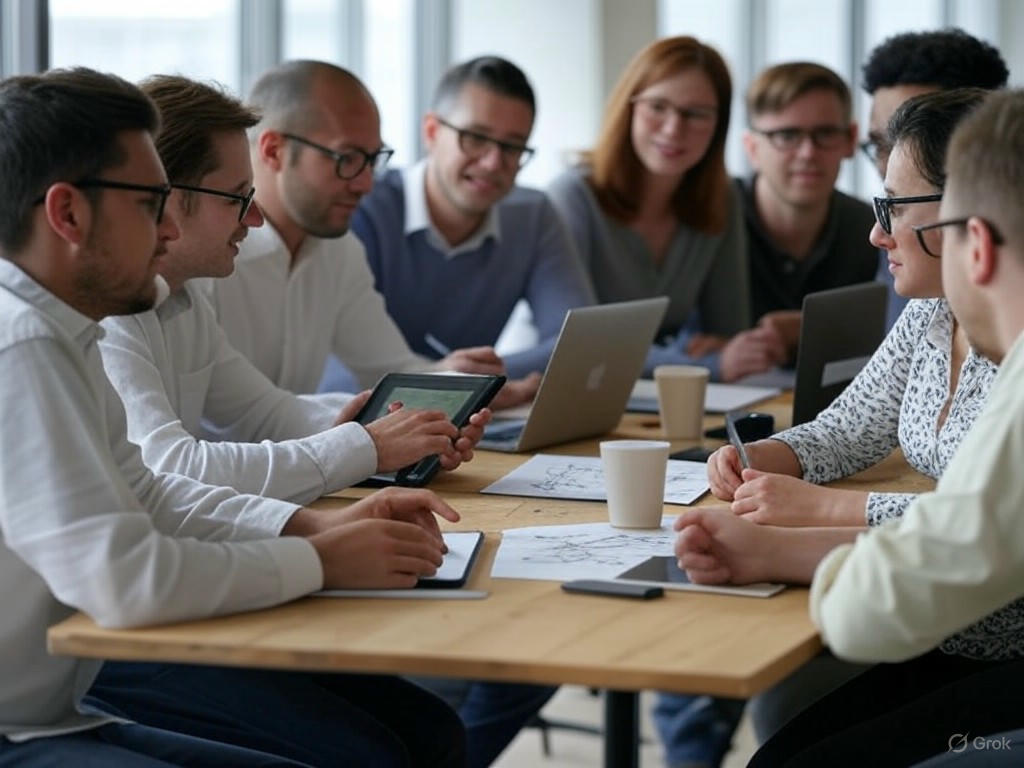
Redefining Creative Collaboration: Human-AI Synergy and Authorship
What unfolds when human ingenuity converges with the relentless computational power of AI? Rather than displacing human creativity, AI in media and entertainment is reshaping the creative process into an iterative, collaborative partnership. This transformation challenges traditional notions of authorship and intellectual property, calling for new frameworks that recognize and balance both human and machine contributions.
From Linear to Iterative: How AI Transforms Creative Workflows
Historically, media content creation has followed a linear trajectory: concept development, production, and distribution. AI disrupts this model by introducing flexibility and speed, enabling creators to explore ideas dynamically rather than sequentially.
Jameson Proctor, a thought leader in advertising, highlights that AI’s greatest potential lies in expanding the field of creative exploration, not just automating routine tasks. By automating labor-intensive activities—such as background generation or drafting initial versions—AI liberates creative teams to act as curators, editors, and ethical stewards.
AI-assisted filmmaking exemplifies this evolution. Platforms like BytePlus ModelArk leverage large language models to rapidly prototype scripts, generate storyboards, and recommend visual elements. This accelerates early-stage ideation, allowing filmmakers to iterate on concepts before investing substantial resources. Meanwhile, AI-generated visuals from tools like DALL·E and Midjourney offer immediate scene mock-ups, giving filmmakers a starting point to refine rather than beginning from scratch.
Disney’s use of AI further illustrates this synergy. Beyond personalizing content on Disney+, AI streamlines production workflows by automating localization and cultural adaptation for global audiences. This reduces traditional bottlenecks in voiceover and translation while preserving creative intent across diverse markets.
However, this shift entails risks. Industry experts caution that AI-driven creativity risks becoming generic or diluted if human oversight diminishes. The creative team’s role remains vital to ensure AI outputs align with brand values and narrative authenticity. AI amplifies but cannot replace the nuanced emotional intelligence and contextual understanding that humans bring to storytelling.
Evolving Authorship and Intellectual Property in the AI Era
The rise of AI-generated content raises pressing questions: who owns the resulting works? Current U.S. Copyright Office guidelines clarify that purely AI-generated works lack copyright protection without significant human creative input. This stance aligns with international trends; for example, the EU’s frameworks require demonstrable human intervention for copyright eligibility.
Yet, the legal terrain is unsettled. High-profile cases such as Getty Images vs. Stability AI and appeals by Emotional Perception AI Ltd in England are shaping critical precedents on liability and ownership. Contrastingly, the Beijing Internet Court’s recent decision to grant copyright to an AI-generated image signals evolving interpretations abroad.
This ambiguity complicates matters for creators and companies. AI models often train on massive datasets scraped from copyrighted works without explicit permission, sparking ethical concerns about the appropriation of creative styles and intellectual property. As commentator Andrew Keen notes, human creativity is transforming—not disappearing—and laws must evolve to ensure fair attribution and compensation.
Policymakers face the challenge of fostering innovation while safeguarding creators’ rights and livelihoods. Some experts advocate new legal instruments, such as federal rights of publicity laws, to address AI’s unique challenges. Transparency regarding AI’s role in content creation and clear guidelines on authorship attribution are essential to navigate this complex landscape.
Case Studies: Ethical and Practical Dimensions of Shared Creative Agency
How does shared creative agency between humans and AI manifest in practice? Consider virtual actors and AI-generated voiceovers, which blur the lines between authentic human performance and synthetic creation. These technologies empower filmmakers and game developers to craft immersive experiences more efficiently.
AI-driven interactive media further expands creative possibilities. Machine learning tailors narratives in real time based on audience engagement, enabling hyper-personalized storytelling. While exciting, these innovations raise ethical questions: who is accountable when AI-generated content misrepresents individuals? How can creators ensure AI respects cultural sensitivities and mitigates bias?
The 2025 NAB Show featured a workshop on ethical AI use in video creation, emphasizing bias awareness, transparency, and accountability. Creators must critically evaluate AI tools, acknowledging their limitations and societal impacts. As AI increasingly influences content, establishing clear authorship and ethical stewardship practices is critical to maintaining trust and integrity.
Academic publishing offers a relevant parallel. Journals are updating policies to define AI’s acceptable role in research authorship, emphasizing that a human must remain “in charge.” This principle applies equally in media: AI is a powerful collaborator, but ultimate creative responsibility and ethical accountability rest with humans.
Key Takeaways
-
AI revolutionizes creative workflows by enabling iterative, collaborative processes that expand creative horizons rather than replace human ingenuity.
-
Current legal frameworks require significant human authorship for AI-assisted works to qualify for copyright, but evolving case law and international variations create ongoing uncertainty.
-
Ethical considerations—including bias mitigation, accountability, and fair attribution—must be integrated into creative workflows to preserve artistic integrity.
-
Case studies from filmmaking, interactive media, and localization illustrate AI’s transformative potential when paired with thoughtful human oversight.
-
The future of creative collaboration depends on transparent, balanced partnerships between humans and AI, supported by evolving legal and ethical frameworks that protect all stakeholders.
As the media and entertainment industry navigates this new frontier, creators and policymakers must embrace both the promise and pitfalls of AI. The goal is clear: technology should amplify human creativity without compromising the values that make art meaningful.
Aspect | Description | Examples / Cases | Key Considerations |
---|---|---|---|
Creative Workflow Transformation | Shift from linear to iterative and collaborative processes enabled by AI | BytePlus ModelArk (script prototyping), DALL·E, Midjourney (visual mock-ups), Disney (localization and adaptation) | AI liberates creators from routine tasks but human oversight critical to maintain authenticity and brand alignment |
Authorship & Intellectual Property | New challenges in defining ownership of AI-generated content | US Copyright Office guidelines, EU legal frameworks, Getty Images vs. Stability AI, Beijing Internet Court ruling | Significant human input required for copyright; evolving legal precedents; ethical concerns over data use and attribution |
Ethical & Practical Dimensions | Balancing human and AI roles in creative agency and accountability | Virtual actors, AI voiceovers, AI-driven interactive media, 2025 NAB Show workshop | Bias mitigation, transparency, accountability, cultural sensitivity, clear authorship responsibility |
Key Takeaways | Summary of AI’s impact and necessary frameworks | Industry-wide implications across media and entertainment sectors | Need for balanced human-AI partnerships, evolving laws, and ethical stewardship to protect creativity and integrity |
Operational and Business Impacts: Efficiency, Risk, and Industry Adoption
Operational and Business Impacts: Efficiency, Risk, and Industry Adoption
Beyond the spotlight on AI-generated scripts and virtual actors, AI is quietly transforming the essential business functions underpinning media and entertainment. Contract negotiations, talent management, and copyright enforcement—once labor-intensive and complex—are now prime areas where AI drives efficiency and strategic advantage.
Streamlining Media Operations: From Contracts to Talent and Copyright
Contract negotiation in major studios typically involves meticulous review of countless clauses, renewals, and risk assessments. AI tools are revolutionizing this process by automating routine contract analysis and flagging potential inconsistencies or risks. This accelerates legal workflows and reduces human error. According to Deloitte’s 2025 industry insights, generative AI increasingly automates and augments contract negotiations, freeing legal teams to focus on strategic decisions rather than paperwork.
In talent management, AI-powered talent intelligence platforms enable studios and agencies to analyze workforce skills, forecast future needs, and build dynamic, skills-based teams. Forbes highlights AI as a cornerstone of HR transformation, strengthening decision-making around talent attraction, engagement, and retention. AI-driven simulations and “what-if” scenarios help studios prepare for fluctuating project demands, a critical capability in an industry marked by unpredictable workforce needs.
Copyright enforcement remains one of the thorniest challenges in media. AI systems now monitor vast amounts of online content to detect unauthorized use or duplication, providing studios with real-time protection against infringement. The U.S. Copyright Office’s 2025 report acknowledges that copyright principles are adapting to AI-generated content, but enforcement is complicated by the evolving legal landscape. Notably, prompt engineering does not confer human authorship, complicating ownership and liability claims.
Productivity Gains vs. Liability Risks: What Industry Surveys Reveal
Industry surveys in 2025 expose a persistent tension between AI-driven efficiency gains and emerging liability risks. McKinsey’s global AI survey shows that while 78% of organizations deploy AI in at least one business function, only 1% view their AI use as mature. Large studios, in particular, face a paradox: 42% of executives cite efficiency and cost reduction as primary benefits, yet they remain cautious about legal liability and intellectual property risks.
Liability concerns often arise from reliance on public or third-party AI models, which may produce unvetted outputs or inadvertently violate copyrights. Deloitte’s analysis underscores big studios’ wariness of potential litigation or reputational damage stemming from AI-related IP risks. While insurance providers are developing AI-specific policies, only 31% of businesses feel confident that their coverage adequately addresses AI risks. This highlights the pressing need for robust risk management frameworks.
Despite these challenges, the business case for AI-driven operational efficiency is strong. PwC and McKinsey report measurable improvements in workflow automation, faster decision-making, and enhanced audience analytics linked to AI adoption. Studios realizing these benefits invest in scaling AI with centralized governance, compliance assurance, and staff upskilling to collaborate effectively with AI agents.
Adoption Patterns: Major Studios vs. Indie Creators
The media and entertainment AI adoption landscape reveals a clear divide between major studios and independent creators. Large studios tend to adopt AI cautiously, integrating generative AI primarily into operations and distribution rather than direct content creation. This conservative approach reflects their scale, legacy systems, and the high stakes involved in IP protection.
Deloitte notes that Hollywood studios favor AI capabilities embedded within established software and SaaS platforms—such as AI-assisted contract management, talent analytics suites, and automated rights monitoring systems. These integrations minimize disruption while enabling incremental yet impactful operational improvements.
In contrast, indie creators embrace AI as a democratizing force. With fewer legacy constraints and lower risk exposure, many are adopting AI content-generation tools to accelerate production and distribution cost-effectively. Newsweek reports that over 65 new AI-centric film studios have launched globally since 2022, with rapid growth continuing through 2025. These creators leverage hybrid AI pipelines that blend human creativity with AI automation to scale storytelling beyond traditional budget limits.
This divergence extends to preferred software tools. Indie creators often rely on accessible AI applications—such as video generators, scriptwriting assistants, and audience segmentation platforms—that require minimal technical expertise. Meanwhile, major studios invest in enterprise-grade SaaS solutions designed for complex workflows, regulatory compliance, and seamless integration with existing infrastructure.
AI SaaS Tools: Seamless Integration into Media Workflows
Successful AI adoption in media hinges on smooth integration into existing operational pipelines. Fortunately, an expanding ecosystem of AI-powered SaaS platforms facilitates this transition. From contract lifecycle management tools utilizing natural language processing to extract key terms, to talent management software predicting workforce needs, these solutions support plug-and-play implementation.
Examples include AI-driven marketing platforms that generate personalized ad variations targeting niche audience segments, as highlighted by Deloitte in streaming video advertising. Real-time AI analytics tools provide actionable audience insights, enabling dynamic programming and promotional decisions. On the copyright front, AI-powered content identification services continuously scan digital landscapes to flag unauthorized uses, proactively safeguarding studio assets.
Smaller studios and creators benefit from subscription-based AI video editors and script generators, lowering barriers to professional-grade production. This modular approach enables organizations to pilot specific functions, evaluate ROI, and scale adoption selectively.
Balancing Optimism with Caution
The media and entertainment industry stands at a critical crossroads. AI offers unprecedented operational efficiencies and creative potential but brings novel risks and ethical considerations. Studios and creators must carefully weigh productivity gains against liabilities, particularly in copyright enforcement and contract management.
The way forward requires not just technology adoption but strategic governance. Embedding AI within legal, talent, and risk management frameworks is essential to harnessing its full potential without compromising creativity, rights, or trust.
Organizations that combine cautious experimentation with robust operational integration will lead the transformation—turning cumbersome media workflows into cutting-edge processes while navigating the inherent uncertainties AI introduces.
Aspect | Details | Sources/Reports |
---|---|---|
Contract Negotiation | AI automates routine contract analysis, flags risks, accelerates legal workflows, reduces human error | Deloitte 2025 industry insights |
Talent Management | AI-powered talent intelligence platforms analyze skills, forecast needs, enable dynamic teams, support HR decisions | Forbes HR transformation insights |
Copyright Enforcement | AI monitors content for unauthorized use, provides real-time infringement protection; challenges with AI authorship and ownership | U.S. Copyright Office 2025 report |
Efficiency Gains | 78% organizations use AI in business functions; 42% of executives cite efficiency and cost reduction benefits | McKinsey global AI survey 2025 |
Liability Risks | Concerns over unvetted AI outputs, IP risks, litigation, reputational damage; only 31% confident in AI risk insurance coverage | Deloitte analysis, industry surveys |
Adoption by Major Studios | Cautious AI use focused on operations and distribution; preference for embedded AI in software/SaaS for contract, talent, rights management | Deloitte industry notes |
Adoption by Indie Creators | Embrace AI content-generation tools for cost-effective production; rapid growth of AI-centric studios; use accessible AI apps | Newsweek reports |
AI SaaS Tools | Platforms for contract lifecycle management, talent forecasting, marketing personalization, real-time analytics, copyright monitoring | Deloitte streaming video advertising insights |
Strategic Governance | Need for embedding AI in legal, talent, risk frameworks to balance efficiency with liabilities and ethical considerations | Industry consensus |
Comparative Analysis of AI Content Creation Tools and Platforms

Comparative Analysis of AI Content Creation Tools and Platforms
What truly sets apart the leading AI content creation tools in 2025? As the media and entertainment landscape embraces AI at an unprecedented scale, evaluating these tools requires a granular look at their core capabilities, real-world performance, and integration nuances.
Generative AI Models: Balancing Output Quality, Customization, and Scalability
Generative AI dominates content creation, with a mix of established leaders and innovative newcomers, each offering unique strengths.
-
ChatGPT (OpenAI): More than a conversational agent, ChatGPT is a versatile content engine. It spans text generation, coding, data visualization, and audio understanding. A key advantage is its customization via Custom GPTs, enabling users to craft tailored bots with specific instructions and data. This adaptability makes it popular across media applications, from scriptwriting to interactive storytelling. However, without precise prompting, its creative output can sometimes feel generic, underscoring the need for skilled user guidance.
-
Claude (Anthropic): Widely regarded for high-quality writing, Claude excels in coherence and nuanced language, making it ideal for editorial content and sophisticated narratives. That said, its deployment remains less widespread than ChatGPT, limiting accessibility for some organizations.
-
Jasper: Known for its user-friendly interface, Jasper specializes in marketing copy and social media content. Its prompt refinement tools help non-technical users navigate creative workflows efficiently, democratizing content generation for marketers.
-
Midjourney and DALL·E 3: These image generation models have become staples for high-quality visual content. Midjourney consistently produces aesthetically compelling images, favored for marketing and entertainment. DALL·E 3’s integration into ChatGPT and Microsoft Copilot democratizes access, offering unlimited free usage that empowers creators at all levels. Adobe Firefly, embedded within Photoshop, provides professional-grade generative features, seamlessly blending AI creativity with industry-standard tools.
-
Runway and Wondershare Filmora: In video content, Runway delivers advanced text-to-video generation and AI-assisted editing, allowing creators to generate unique clips from textual prompts. Wondershare Filmora focuses on streamlined AI video creation workflows, targeting users seeking simplicity. Currently, AI video tools excel mainly in short clips or B-roll footage, with full-length autonomous video production still out of reach.
Scalability varies widely. Cloud-based APIs, such as OpenAI’s models, scale efficiently for enterprise needs, ensuring reliability and ease of integration. Conversely, open-source alternatives offer greater flexibility and cost control but demand substantial infrastructure investment and ongoing maintenance. The choice often hinges on organizational priorities: ease of use and dependability (proprietary cloud services) versus customization and cost efficiency (open-source).
Image and Video Processing APIs: Performance and Workflow Compatibility
With video accounting for an estimated 82% of internet traffic in 2025, AI-powered video processing APIs must deliver both quality and seamless integration into complex workflows.
-
Runway: Beyond generative video, Runway offers powerful features like object recognition, emotion detection, and scene summarization. These capabilities enhance content management and provide creators with AI-driven editing tools that support dynamic video generation.
-
Google Veo 2: This platform impresses with multi-instance processing and sophisticated AI that replicates human motion and generates animations, appealing to animation studios and broadcasters. However, integrating Veo 2 requires significant resources and technical expertise, which can pose barriers.
-
ElevenLabs’ Suno: Specializing in audio and music generation, Suno enables users to create complete songs from lyrics or background scores without lyrics. This is invaluable for media producers who need bespoke soundtracks without the expense or time of traditional composition.
-
AI Video Content Management Systems (VCMS): Enterprise adoption depends on features like auto-tagging, facial recognition, and AI-driven summarization. These enhance content discoverability and personalization but come with challenges in integration complexity and data security.
-
Veed.io and Pictory: These platforms prioritize accessibility, offering intuitive AI video editing tools tailored for small businesses and individual content creators lacking large production teams.
Despite these advancements, AI tools for fully autonomous, polished long-form video production remain aspirational. The nuanced artistry and storytelling finesse still rely heavily on human collaboration.
AI-Powered Audience Analytics Suites: Data Accuracy, Real-Time Insights, and Integration
AI-driven audience analytics have evolved beyond static reports into dynamic, real-time engines shaping content strategies.
-
Proprietary analytics platforms typically offer turnkey solutions with professional support, robust security, and seamless integration into content creation pipelines. This results in faster deployment and reduced technical overhead.
-
Open-source analytics tools provide unmatched flexibility and cost-efficiency, allowing organizations to customize analytics pipelines and experiment with advanced models. However, they often require complex setups and heightened attention to security, especially when handling sensitive media user data.
-
Industry data shows that over 78% of businesses incorporate open-source software in their analytics stacks, evidencing its widespread appeal despite challenges like documentation gaps and maintenance demands.
-
Hybrid approaches like BytePlus combine proprietary technology built on open frameworks to balance rapid innovation with enterprise-grade reliability.
Modern AI analytics suites emphasize:
-
Accuracy: Machine learning models identify viewer preferences, sentiment, and engagement with precision far exceeding traditional metrics.
-
Real-Time Capabilities: These enable creators and marketers to adjust strategies on the fly, enhancing viewer retention and delivering personalized experiences.
-
Compatibility: Deep integration with content creation platforms streamlines workflows, fostering seamless feedback loops between analytics and production teams.
Wrapping Up: Choosing the Right Tool for the Job
Selecting the appropriate AI content creation or analytics platform requires aligning tool capabilities with specific use cases rather than chasing hype.
-
For versatile, text-heavy content with broad language understanding, ChatGPT and Claude remain leading choices.
-
Visual content creators can leverage Midjourney, DALL·E 3, and Adobe Firefly—covering a spectrum from free experimentation to professional-grade outputs.
-
Video creators seeking AI-assisted editing and generation will find Runway and Veed.io valuable allies, although fully autonomous video production is still emerging.
-
On the analytics side, enterprises must balance proprietary ease of deployment against open-source flexibility, carefully weighing security, scalability, and integration needs.
The frontier of AI in media demands critical, informed evaluation beyond buzzwords. By grounding decisions in practical benchmarks—output quality, customization ease, scalability, and integration complexity—media professionals can harness AI’s transformative power while preserving human creativity and ethical responsibility.
Tool / Platform | Core Capabilities | Strengths | Limitations | Use Cases | Scalability & Integration |
---|---|---|---|---|---|
ChatGPT (OpenAI) | Text generation, coding, data visualization, audio understanding; customizable via Custom GPTs | Versatile content engine; broad media applications; customizable bots | Creative output can be generic without skilled prompting | Scriptwriting, interactive storytelling, coding, data tasks | Cloud-based API; scalable; easy integration; proprietary |
Claude (Anthropic) | High-quality text generation, coherent and nuanced language | Excellent for editorial content and sophisticated narratives | Less widespread deployment; limited accessibility | Editorial writing, complex narratives | Proprietary; less accessible; enterprise use limited |
Jasper | Marketing copy and social media content generation; prompt refinement tools | User-friendly interface; democratizes content creation for marketers | Focused mainly on marketing; less versatile for other content types | Marketing copy, social media posts | Cloud-based; scalable; easy for non-technical users |
Midjourney | Image generation | Aesthetically compelling images; favored in marketing and entertainment | Primarily image generation; no text/video integration | Visual content for marketing, entertainment | Cloud-based; scalable |
DALL·E 3 | Image generation integrated with ChatGPT and Microsoft Copilot | Unlimited free usage; democratizes access; versatile | Requires integration; depends on platform support | Visual content creation at all levels | Cloud-based; scalable; integrated in popular platforms |
Adobe Firefly | Generative image features embedded in Photoshop | Professional-grade AI creativity; seamless with industry tools | Requires Adobe ecosystem; professional skills needed | High-end image generation and editing | Integrated; professional workflows; proprietary |
Runway | Text-to-video generation, AI-assisted video editing, object recognition, emotion detection, scene summarization | Advanced video generation and editing tools; AI-powered content management | Best for short clips and B-roll; full-length video still emerging | Video content creation, editing, dynamic video generation | Cloud-based; scalable; enterprise-capable |
Wondershare Filmora | AI video creation workflows | Streamlined, simple video creation for users | Limited to short clips; less advanced AI features than Runway | Simple AI-assisted video creation | Cloud-based; accessible for small teams |
Google Veo 2 | Multi-instance video processing, human motion replication, animation generation | Advanced AI for animation and broadcast studios | Integration requires significant resources and expertise | Animation studios, broadcasters | Complex integration; enterprise-level |
ElevenLabs’ Suno | Audio and music generation from lyrics or background scores | Enables bespoke soundtracks without traditional composition costs | Specialized in audio; not a full video tool | Media producers needing custom soundtracks | Cloud-based; scalable |
Veed.io & Pictory | Intuitive AI video editing tools | Accessible to small businesses and individual creators | Limited advanced AI features; focused on ease of use | Small business and individual content creation | Cloud-based; easy integration |
AI Video Content Management Systems (VCMS) | Auto-tagging, facial recognition, AI-driven summarization | Enhances discoverability and personalization | Integration complexity; data security challenges | Enterprise video content management | Enterprise-grade; complex integration |
Proprietary Audience Analytics Platforms | Turnkey analytics solutions with professional support | Robust security; fast deployment; seamless pipeline integration | Higher cost; less customization | Enterprise content strategy and audience insights | Enterprise-grade; easy deployment |
Open-Source Analytics Tools | Customizable analytics pipelines; advanced model experimentation | Cost-efficient; flexible; widely adopted | Complex setup; documentation gaps; security concerns | Organizations with technical resources and customization needs | Flexible; requires infrastructure and maintenance |
BytePlus (Hybrid) | Proprietary tech built on open frameworks | Balances innovation speed with enterprise reliability | Hybrid complexity | Enterprises seeking balance of customization and reliability | Enterprise-grade; hybrid integration |
Future Trajectories: Emerging Trends, Ethical Frontiers, and Societal Implications

Future Trajectories: Emerging Trends, Ethical Frontiers, and Societal Implications
What happens when the boundaries between human creativity and artificial agency start to blur? In media and entertainment, AI is no longer just a set of tools augmenting human creators. It is ushering in new digital entities that take center stage, reshaping data privacy paradigms, and edging us closer to the possibility of artificial general intelligence (AGI). These advances bring exciting opportunities—but also complex ethical questions and societal shifts that demand our attention.
Virtual Beings as Autonomous Content Creators
By 2025, virtual influencers—AI-generated digital personas crafted through CGI, motion capture, and deep learning—have evolved from novelties into serious cultural and commercial forces. These virtual beings provide brands with unparalleled control over narrative, appearance, and engagement schedules. Unlike human influencers, they don’t require breaks or approvals, enabling continuous, scalable content production across multiple platforms.
For example, some virtual influencers already command large, dedicated fan bases and lucrative brand partnerships, with the market projected to exceed $21 billion by 2024. Looking ahead to 2026, technology is expected to advance so that these digital personas operate with minimal human oversight, potentially dominating sectors like fashion, gaming, and tech marketing.
However, this evolution raises critical concerns about authenticity and audience trust. As virtual influencers grow more lifelike, the line between genuine and artificial interaction blurs, risking deception and reinforcing unrealistic beauty or lifestyle ideals. This dynamic presents a classic double-edged sword: immense creative flexibility balanced against potential manipulation.
Federated Learning: Privacy-Preserving AI in Media
Balancing AI’s power with user privacy is an ongoing challenge. Federated learning (FL) offers a promising path forward by enabling AI models to train locally on distributed devices, sharing only model updates instead of raw sensitive data. This approach aligns well with the media and entertainment industry’s increasing reliance on personalized content and audience analytics that involve sensitive user information.
For instance, FL can improve recommendation algorithms while respecting privacy laws and user consent, addressing critical concerns highlighted in studies like Cisco’s 2025 Data Privacy Benchmark. Yet, federated learning faces hurdles such as security vulnerabilities—including Byzantine attacks where corrupted local models threaten global integrity—high communication overhead, and bias from non-representative local datasets.
The research community is actively developing defenses and optimizations to overcome these challenges. Market forecasts anticipate FL’s global value reaching over $360 million by 2034, with major technology companies like Google, IBM, and NVIDIA investing heavily. Success will depend on integrating federated learning with robust, adaptive data governance frameworks that ensure ethical and effective AI deployment in media.
Progress Toward Artificial General Intelligence (AGI) in Entertainment
AGI—AI capable of performing any intellectual task a human can—looms as a transformative prospect for media and entertainment. Industry leaders, including OpenAI CEO Sam Altman, suggest AGI could emerge within the next few years, potentially revolutionizing content creation, storytelling, and audience engagement.
Imagine an AI collaborator co-writing scripts, composing music, or improvising live performances with human-like creativity and understanding. The possibilities are staggering, but so are the uncertainties. AGI’s arrival would require unprecedented ethical frameworks, regulatory oversight, and societal preparedness.
While excitement is justified, caution is essential. AGI development timelines remain speculative, and potential societal impacts—both positive and disruptive—are not yet fully understood. The entertainment industry must prepare not only technologically but also culturally and legally for this paradigm shift.
Ethical Frontiers: Authenticity, Inclusivity, and Data Governance
As AI-generated content becomes ubiquitous, maintaining authenticity is a core challenge. Deepfakes and synthetic media pose significant risks for misinformation and brand integrity. Combating digital deception demands strong brand reputations, advanced detection tools, and transparent audience communication.
Inclusivity and bias mitigation are equally critical. AI content generators often replicate biases inherent in their training data, leading to stereotypical or exclusionary outputs. For example, image generation platforms frequently default to conventional gender, race, or occupational stereotypes unless deliberately corrected.
Addressing these issues requires diverse AI development teams, enhanced AI literacy across creative industries, and ongoing education. Initiatives such as the AEQUITAS workshops on fairness and bias play a vital role in fostering accountability and inclusivity.
Data governance frameworks must evolve beyond security to manage ethical use of AI-generated insights. Organizations embedding AI into media operations need modular, adaptable governance models capable of handling new data sources and AI tools, while minimizing risks of unintended bias or privacy breaches.
Societal Implications: Employment, Cultural Production, and Audience Interaction
AI’s rapid growth is reshaping the creative workforce. While McKinsey estimates millions of jobs could be disrupted across sectors, many experts emphasize AI as a collaborator that amplifies creative professions rather than replaces them. Journalists and researchers, for instance, leverage AI tools to summarize vast information, freeing time for higher-level analysis and storytelling.
Cultural production is shifting from exclusively human-driven processes to hybrid models where AI-generated content complements human creativity. Social media platforms illustrate this trend vividly: younger generations increasingly form strong connections with both human and virtual digital creators, influencing consumption habits and advertising strategies.
Audience interaction is becoming more dynamic and personalized. AI-powered predictive analytics and virtual agents enable brands to engage users with tailored content in real time, fostering deeper parasocial relationships and innovative monetization models. However, these advances raise concerns about data privacy and the psychological effects of immersive digital experiences.
Navigating Uncertainty with Evidence-Based Strategies
The AI-driven future of media and entertainment is exhilarating but complex. To maximize AI’s positive potential while mitigating risks, stakeholders must pursue evidence-based strategies grounded in rigorous research and transparency.
Key actions include:
- Investing in comprehensive AI ethics education and literacy for creators and consumers alike.
- Prioritizing diversity within AI development teams to reduce systemic biases.
- Implementing adaptive data governance frameworks that evolve with AI technologies.
- Encouraging interdisciplinary collaboration among technologists, ethicists, policymakers, and creatives.
- Monitoring societal impacts attentively to guide responsible innovation.
By balancing technological enthusiasm with critical scrutiny, the media and entertainment industries can harness AI as a force for creative empowerment, cultural enrichment, and social good.
Topic | Description | Key Points | Future Outlook | Challenges & Concerns |
---|---|---|---|---|
Virtual Beings as Autonomous Content Creators | AI-generated digital personas used for branding and content creation | Continuous content production, scalable engagement, market projected > $21B by 2024 | Minimal human oversight by 2026; dominance in fashion, gaming, tech marketing | Authenticity issues, audience trust, risk of deception, unrealistic ideals |
Federated Learning (FL) | Privacy-preserving AI training on distributed devices without sharing raw data | Improves recommendations, respects privacy laws, aligned with personalized content | Market value > $360M by 2034; major investments by Google, IBM, NVIDIA | Security vulnerabilities (Byzantine attacks), communication overhead, data bias |
Artificial General Intelligence (AGI) in Entertainment | AI capable of performing any intellectual task a human can | Potential to revolutionize content creation, storytelling, audience engagement | Possible emergence within next few years; requires new ethical/regulatory frameworks | Speculative timelines, societal impacts uncertain, need cultural and legal prep |
Ethical Frontiers | Issues of authenticity, inclusivity, and data governance in AI-generated content | Risks from deepfakes, bias in AI outputs, need diverse teams and AI literacy | Development of robust detection tools, fairness workshops like AEQUITAS | Combating misinformation, exclusionary stereotypes, evolving governance models |
Societal Implications | Impact on employment, cultural production, and audience interaction | AI as collaborator, hybrid creative models, strong fan engagement with virtual creators | Dynamic personalized audience interaction, new monetization models | Job disruption concerns, data privacy, psychological effects of immersive experiences |
Navigating Uncertainty | Strategies to balance AI benefits and risks in media and entertainment | AI ethics education, diverse teams, adaptive governance, interdisciplinary collaboration | Evidence-based innovation, monitoring societal impacts | Managing biases, privacy risks, ensuring responsible AI use |