AI in Marketing: Personalization, Targeting & Analytics Demystified
- Introduction: Why AI is Redefining Personalization and Targeting in Modern Marketing
- Introduction: Why AI is Redefining Personalization and Targeting in Modern Marketing
- The Rising Imperative of Personalized Marketing Amid Digital Transformation
- Economic Drivers and Evolving Consumer Behaviors Fueling AI Adoption
- Defining the Scope: Personalization, Analytics, and Customer Targeting with AI
- Balancing Enthusiasm with Ethical Considerations
- Foundations of AI-Driven Personalization: Core Technologies and Data Inputs
- Foundations of AI-Driven Personalization: Core Technologies and Data Inputs
- Core AI Techniques Powering Personalization
- Types of Data: Zero-, First-, and Third-Party
- Data Integration: Building Unified Customer Profiles
- Why These Foundations Matter
- AI-Powered Customer Targeting: Algorithms, Segmentation, and Dynamic Content Delivery
- AI-Powered Customer Targeting: Algorithms, Segmentation, and Dynamic Content Delivery
- Advanced Customer Segmentation: Beyond Age and Location
- Dynamic Content Generation: The Rise of Generative AI in Marketing
- Feedback Loops and Continuous Learning: Refining Targeting Precision
- In Summary: The AI-Powered Targeting Ecosystem
- Analytics in AI Marketing: Measuring Effectiveness and Driving Optimization
- Analytics in AI Marketing: Measuring Effectiveness and Driving Optimization
- Advanced Attribution Models: Beyond the Last Click
- Real-Time Performance Tracking and Predictive Insights
- Integrating AI Analytics into Marketing Decision Engines
- Balancing AI’s Promise with Practical Realities
- Final Thoughts
- Technical and Ethical Challenges in AI Marketing Personalization
- Technical and Ethical Challenges in AI Marketing Personalization
- Navigating Data Privacy and Transparency
- The Risk of Algorithmic Bias and Manipulation
- Technical Challenges: Integration, Data Quality, and Multi-Channel Personalization
- Balancing Act: Ethics, Trust, and Technical Excellence
- Comparative Insights: Traditional Marketing vs. AI-Enhanced Personalization Approaches
- Comparative Insights: Traditional Marketing vs. AI-Enhanced Personalization Approaches
- Scale and Speed: AI’s Unmatched Advantage
- Depth of Customization: Moving Beyond Demographics
- Case Studies: Retail, Media, and E-Commerce in Action
- Limitations and Complementary Roles of Traditional Marketing
- Strategic Takeaways
- Future Trajectories: Emerging AI Innovations and Their Impact on Marketing Personalization
- Future Trajectories: Emerging AI Innovations and Their Impact on Marketing Personalization
- Foundation Models and Real-Time Adaptive Personalization
- Cross-Device Identity Resolution: The Key to Seamless Personalization
- Evolving Consumer Expectations and Regulatory Realities
- Human-AI Collaboration: The Next Frontier in Marketing Personalization
- Key Takeaways
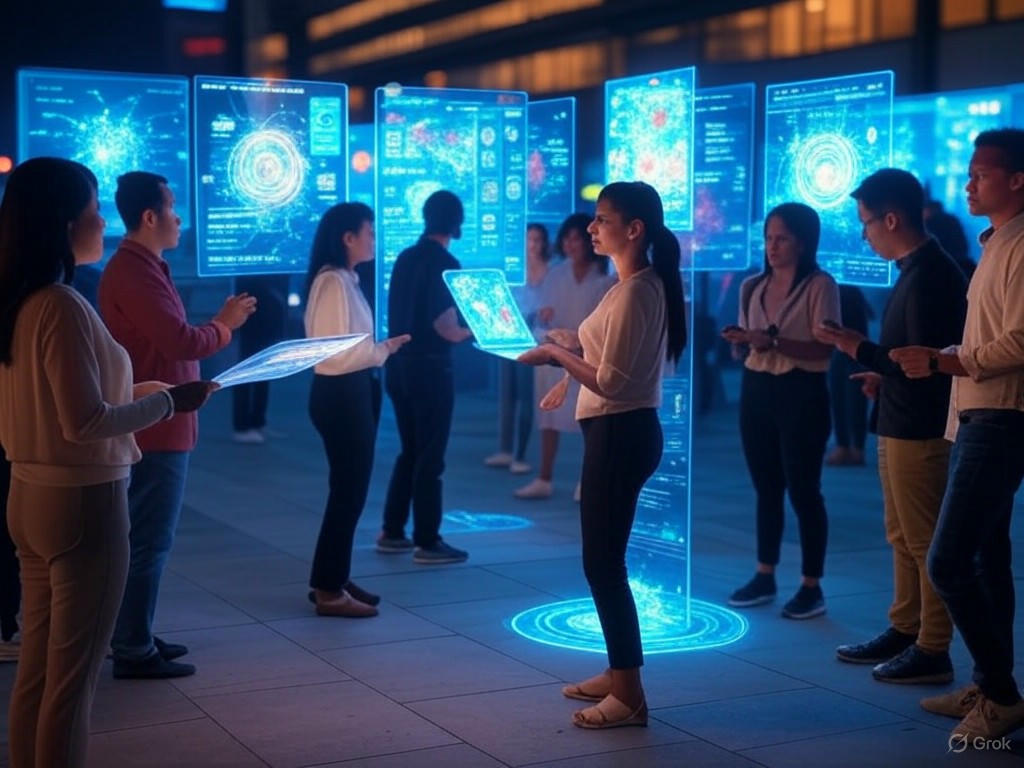
Introduction: Why AI is Redefining Personalization and Targeting in Modern Marketing
Introduction: Why AI is Redefining Personalization and Targeting in Modern Marketing
Imagine your favorite online store not only remembering your last purchase but anticipating exactly what you want next—crafting messages and offers that feel tailor-made just for you. This is no longer a futuristic vision but a rapidly unfolding reality powered by AI-driven personalization and targeting. As digital transformation accelerates, AI has become the backbone reshaping how brands connect with consumers, with profound implications spanning strategy, economics, and ethics.
The Rising Imperative of Personalized Marketing Amid Digital Transformation
By 2025, personalization has shifted from a competitive advantage to a baseline expectation. McKinsey reports that 65% of customers identify targeted promotions as a key driver of their purchase decisions, underscoring the critical role of delivering relevant offers at the right moment.
Generative AI is revolutionizing content creation, transforming manual, time-consuming workflows into agile, scalable processes. Marketers now deploy AI to generate copy, visuals, and even videos tailored dynamically to diverse audience segments. This capability not only accelerates campaign deployment but also magnifies impact through real-time, hyper-personalized outreach.
Simultaneously, the digital transformation wave is redefining how marketing success is measured. Integrated AI-powered platforms provide granular insights into campaign performance by audience segments, creative variants, and channels. This comprehensive visibility empowers leadership to maximize return on investment and refine strategies with agility.
Yet, personalization transcends technology alone. McKinsey emphasizes that success requires “seamlessly integrated platforms backed by well-trained teams.” Without this alignment, even the most advanced AI models can underperform or misfire.
Economic Drivers and Evolving Consumer Behaviors Fueling AI Adoption
Why are organizations accelerating investments in AI-powered marketing? The answer encompasses both significant economic upside and evolving consumer expectations.
The economic potential is staggering. McKinsey estimates generative AI alone could add between $2.6 trillion and $4.4 trillion in value across industries, with marketing and sales poised to capture substantial revenue growth. Adoption is widespread and accelerating—72% of organizations deploy AI in at least one business function, with marketing and sales adoption rates doubling year over year. For marketers, AI is rapidly becoming indispensable for maintaining relevance and competitive edge.
Consumer behaviors are evolving in tandem. Buyers increasingly demand personal, timely interactions that resonate with their unique preferences. In B2B contexts, 58% of marketers incorporate customer stories or testimonials into advertising, while personalized video content provides a notable advantage. For B2C brands, hyper-personalization and seamless omnichannel experiences are now standard expectations.
However, challenges remain—while 78% of businesses regard first-party data as their most valuable personalization resource, only 37% of customers trust companies with their personal data. This trust gap highlights the critical need for transparency, robust data governance, and privacy-centric AI practices.
Defining the Scope: Personalization, Analytics, and Customer Targeting with AI
AI’s transformative impact in modern marketing spans several interconnected domains:
-
Personalization at Scale: By analyzing vast datasets—including browsing behavior, purchase history, and engagement patterns—AI crafts individualized experiences in real time across channels. Generative AI further enhances this by producing customized content such as ad copy, images, and videos that deeply resonate with targeted subgroups.
-
Advanced Analytics and Predictive Models: AI-powered predictive analytics forecast customer behaviors, promotion responsiveness, and engagement likelihood. These dynamic, continuously learning models enable brands to deliver the right message to the right individual at the optimal moment, moving beyond static segmentation toward agile, data-driven decision engines.
-
Customer Targeting Without Cookies: As privacy regulations tighten and third-party cookies fade, AI-driven cookieless targeting techniques analyze behavioral patterns—like preferred communication channels and purchasing trends—while respecting privacy. These approaches offer a compliant path to effective audience segmentation and targeting.
Despite these advances, marketers must temper enthusiasm with realism. AI’s effectiveness depends on the quality and representativeness of training data. Risks such as algorithmic bias, data privacy challenges, and “hallucinated” or misleading content persist. Ethical AI practices—including diverse datasets, human-in-the-loop validation, and transparent communication—are essential for sustainable success.
Balancing Enthusiasm with Ethical Considerations
The promise of AI-driven marketing is compelling but demands responsible stewardship. Key ethical considerations include:
-
Algorithmic Bias: AI systems trained on unrepresentative or skewed data can perpetuate biases, resulting in unfair targeting or exclusion that undermines brand trust and invites regulatory scrutiny.
-
Data Privacy: With only about a third of customers trusting companies to handle their personal data responsibly, transparent data policies and stringent security measures are non-negotiable.
-
Human Oversight: AI excels at automation and scale but cannot replace human judgment. Ongoing human involvement is vital to interpret AI outputs, catch errors, and preserve creativity and empathy.
-
Consumer Awareness: Brands should disclose when AI shapes marketing communications to foster trust and avoid perceptions of manipulation.
In sum, AI is evolving from a tactical tool to a strategic imperative in marketing—one that must be managed thoughtfully. Organizations that master this balance—harnessing AI’s power while upholding ethical principles—will unlock unprecedented growth and forge deeper, more authentic customer connections.
In the chapters ahead, we will explore how AI concretely transforms personalization, analytics, and customer targeting. Through practical examples, limitations analysis, and guidance on navigating complex ethical and technical landscapes, we’ll uncover where data meets empathy and technology meets trust—the frontier defining marketing’s future.
Topic | Details |
---|---|
Customer Expectation by 2025 | Personalization as a baseline expectation; 65% of customers driven by targeted promotions (McKinsey) |
Generative AI Impact | Transforms content creation (copy, visuals, video), accelerates campaigns, enables hyper-personalized outreach |
AI-powered Analytics | Granular insights by audience, creative variants, and channels; improves ROI and strategy refinement |
Economic Impact | Generative AI could add $2.6T-$4.4T in value; 72% organizations deploy AI; marketing/sales adoption doubling annually |
Consumer Behavior | Demand for personal, timely interactions; 58% B2B marketers use testimonials; hyper-personalization standard in B2C |
Data Trust | 78% businesses value first-party data; only 37% customers trust companies with personal data |
AI Domains in Marketing | Personalization at scale, advanced analytics/predictive models, cookieless customer targeting |
Ethical Considerations | Algorithmic bias, data privacy, human oversight, consumer awareness and transparency |
Foundations of AI-Driven Personalization: Core Technologies and Data Inputs
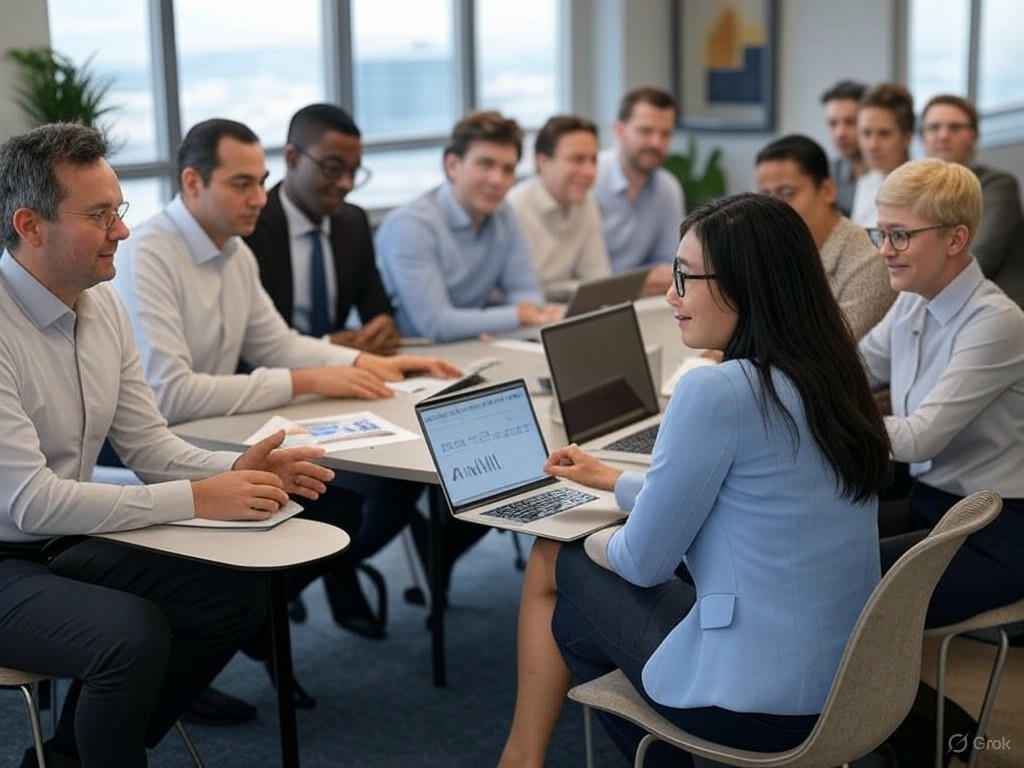
Foundations of AI-Driven Personalization: Core Technologies and Data Inputs
What enables AI to craft marketing experiences so finely tuned they almost feel intuitive? At its essence, AI-driven personalization relies on a combination of advanced techniques—machine learning (ML), natural language processing (NLP), generative AI, and predictive analytics—that analyze vast volumes of data to anticipate individual customer needs and preferences in real time.
Core AI Techniques Powering Personalization
Machine learning (ML) serves as the foundation for personalization’s adaptability. By examining historical customer behaviors, ML algorithms identify which content, offers, or products resonate with each individual and automate decisions to deliver precisely tailored experiences. This approach surpasses traditional segmentation by uncovering subtle, evolving patterns in customer preferences with every interaction. For example, Indian brands like Nykaa and Dunzo leverage ML to create user experiences that dynamically respond to behavior—not just static demographics or broad segments.
Natural language processing (NLP) adds a vital dimension by enabling systems to understand and interpret human language from diverse sources such as customer reviews, chat transcripts, and social media posts. Breakthroughs in transformer architectures, like GPT-4, have transformed NLP’s capacity to grasp nuance and context. This empowers chatbots and virtual assistants to engage customers with natural, context-aware conversations—enhancing customer service and surfacing insights that inform more personalized marketing strategies.
Generative AI, a rapidly emerging technology, accelerates personalized content creation. It enables marketers to produce tailored blog posts, social media messages, and ad copy at scale, moving beyond generic messaging. Generative AI crafts unique, contextually relevant content that can be updated in real time, responding fluidly to shifting customer moods and preferences. Platforms such as Jasper and Brandwell exemplify how generative AI tools help marketers generate persuasive, brand-aligned content efficiently.
Predictive analytics integrates these capabilities to forecast customer behavior before it happens. Think of it as a marketing crystal ball—predicting which products a customer might be interested in next or the optimal moment to present an offer. Tools like Progress Sitefinity and Adobe’s AI-powered Customer AI employ predictive models that shift marketing from reactive to anticipatory, enabling brands to “predict and profit” rather than play catch-up.
Types of Data: Zero-, First-, and Third-Party
The sophistication of AI personalization depends heavily on the quality and diversity of data it processes. Understanding the distinctions between zero-, first-, and third-party data is crucial.
-
Zero-party data is the most accurate and privacy-respecting form. It includes information customers deliberately and proactively share with brands—such as preferences, purchase intentions, or personal contexts. Since this data comes directly from the customer, it avoids many privacy pitfalls and supports transparent, respectful personalization. Imagine it as a customer handing you a map to their tastes and desires.
-
First-party data is collected through direct brand interactions, including website visits, app usage, purchases, surveys, and support contacts. This data is rich and reliable, reflecting actual behaviors and touchpoints. With tightening privacy regulations limiting third-party data use, 78% of businesses now regard first-party data as their most valuable personalization asset.
-
Third-party data aggregates information from external sources and is often used for broader targeting. However, it is generally less precise and increasingly restricted by privacy laws, making it less reliable for nuanced personalization.
Brands that seamlessly integrate zero- and first-party data can develop nuanced, consent-based customer understanding, enabling personalization that feels intuitive rather than intrusive.
Data Integration: Building Unified Customer Profiles
Picture trying to understand a customer’s preferences by piecing together fragmented snapshots from various sources—social media channels, email campaigns, in-store visits, and support calls—each telling only part of the story. Without integration, personalization remains siloed and inconsistent.
Data integration merges these disparate data streams into a unified, comprehensive customer profile—a “single source of truth.” This requires advanced techniques such as ETL (Extract, Transform, Load) processes, middleware solutions, and modern data warehousing. The objective is to harmonize diverse data formats and channels in real time, enabling a coherent 360-degree view of each customer.
With unified profiles, marketers can employ advanced concepts like propensity scoring—a statistical technique that assigns each customer a likelihood score for responding to specific offers or content. Think of it as a heat map highlighting where marketing efforts will yield the greatest impact. For instance, a propensity score might reveal that a young professional is highly responsive to short, festive-themed promotions but less engaged by detailed financial breakdowns, while an older customer prefers the opposite. This insight enables AI to tailor messages with surgical precision.
Real-time decisioning extends this capability by allowing systems to instantly interpret customer data and context, then autonomously select and deliver the optimal message or offer. Imagine a digital concierge who knows your preferences, mood, and timing to engage you at just the right moment. Leading platforms like Hightouch and OfferFit employ continuous learning to optimize these interactions across channels, scaling personalized experiences beyond human capacity.
Why These Foundations Matter
The interplay of cutting-edge AI techniques, rich and privacy-conscious data, and seamless integration forms the bedrock of effective personalization. When executed thoughtfully, this transforms marketing from broad, scattershot efforts into finely tuned dialogues that respect customer intent, anticipate needs, and deliver genuine value.
Yet, the complexity of these systems also demands vigilance. Ethical considerations—such as avoiding bias in AI models, ensuring transparency, and safeguarding data privacy—are not optional add-ons but foundational responsibilities. The future of AI-driven personalization is not just about being smarter and faster; it must be ethical and respectful to earn and maintain customer trust.
Looking ahead, brands that master these foundational technologies and data strategies will not only outperform competitors but also reshape customer expectations for personalized experiences. As AI continues to evolve, so too must our commitment to deploying it wisely and responsibly.
Category | Description | Examples / Tools |
---|---|---|
Machine Learning (ML) | Analyzes historical customer behaviors to identify patterns and automate personalized experiences beyond traditional segmentation. | Nykaa, Dunzo |
Natural Language Processing (NLP) | Enables understanding of human language from reviews, chats, and social media; powers context-aware conversations and insights. | GPT-4, Chatbots, Virtual Assistants |
Generative AI | Creates tailored content at scale, adapting in real time to customer moods and preferences. | Jasper, Brandwell |
Predictive Analytics | Forecasts customer behavior to enable anticipatory marketing rather than reactive strategies. | Progress Sitefinity, Adobe Customer AI |
Zero-Party Data | Data customers proactively share, such as preferences and intentions; privacy-respecting and highly accurate. | Direct customer inputs, surveys |
First-Party Data | Collected from direct brand interactions like website visits, purchases, and support; rich and reliable. | Website analytics, app usage data |
Third-Party Data | Aggregated from external sources; less precise and increasingly limited by privacy laws. | External data providers |
Data Integration | Combines diverse data streams into a unified customer profile for consistent personalization across channels. | ETL processes, Middleware, Data Warehousing |
Propensity Scoring | Assigns likelihood scores to customers for responsiveness to offers or content, guiding targeted marketing. | Statistical models, Heat maps |
Real-Time Decisioning | Instantly interprets customer data to autonomously deliver optimal messages or offers. | Hightouch, OfferFit |
AI-Powered Customer Targeting: Algorithms, Segmentation, and Dynamic Content Delivery
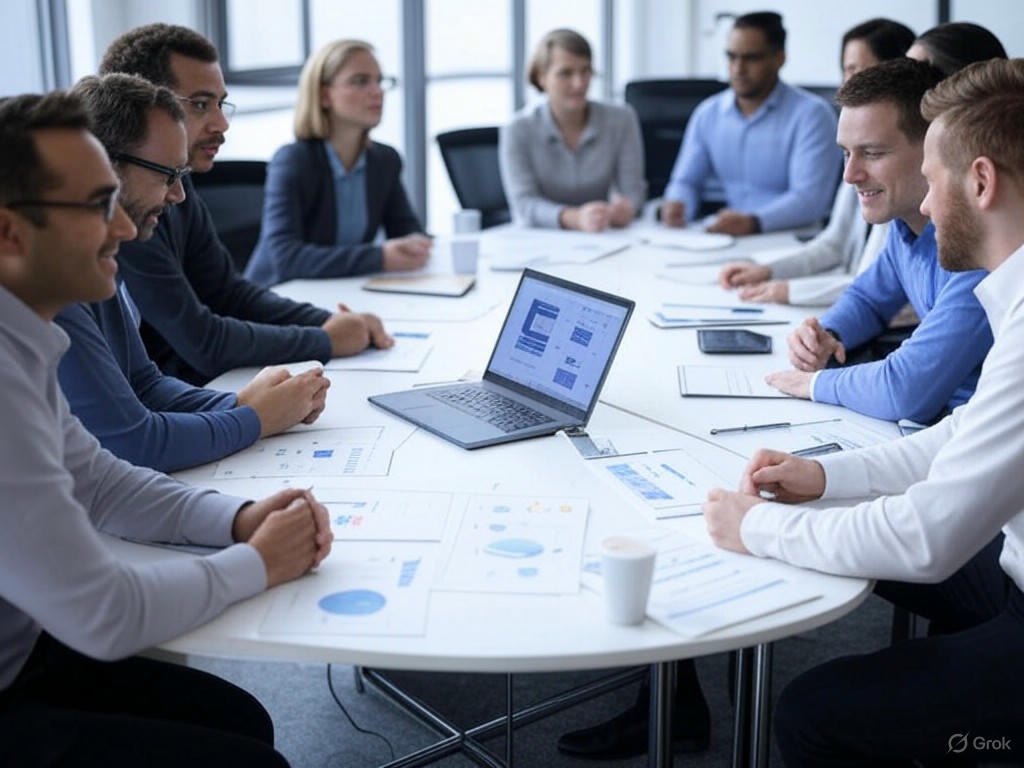
AI-Powered Customer Targeting: Algorithms, Segmentation, and Dynamic Content Delivery
How do marketers move beyond traditional demographics to truly understand and anticipate customer needs? The answer increasingly lies in AI’s ability to analyze behavior, preferences, and predictive signals—uncovering insights that were once inaccessible. This shift represents more than a technological upgrade; it’s a fundamental transformation in how brands engage customers with precision and empathy.
Advanced Customer Segmentation: Beyond Age and Location
Conventional segmentation—grouping audiences by age, gender, or location—has long been a blunt instrument. Today, AI algorithms delve into far richer datasets, leveraging behavioral patterns and predictive analytics to create micro-segments that mirror genuine customer intent and lifestyles.
By analyzing browsing histories, purchase behavior, engagement metrics, and social media interactions, AI systems identify who is likely to become a loyal customer, who might churn, or who will respond to specific promotions. This segmentation is dynamically refined as customer behaviors evolve, ensuring marketing messages stay relevant and impactful.
Key AI-driven segmentation capabilities include:
- Behavioral Segmentation: Monitoring actions such as time spent on product pages, interaction frequency, and click-through rates to develop nuanced profiles.
- Preference Analysis: Combining explicit data (e.g., survey responses or app settings) with implicit signals (e.g., content consumption patterns).
- Predictive Signals: Applying machine learning to forecast future behaviors like purchase propensity or churn risk, enabling proactive engagement.
Marketers leveraging AI-powered platforms report more meaningful outcomes. A 2025 EventX study highlights AI’s ability to “learn and adapt from user behavior,” helping brands deliver campaigns that “feel right” by closely aligning with customer intent. The result? Higher engagement, increased loyalty, and optimized return on investment.
Dynamic Content Generation: The Rise of Generative AI in Marketing
While segmentation determines who to reach, dynamic content generation shapes what to say—and how to say it. Generative AI has matured into a powerful enabler, transforming marketers into content creators capable of producing personalized, compelling messages at scale.
For an e-commerce campaign, for example, generative AI can produce multiple variations of copy, images, and offers tailored to distinct audience segments. Examples include:
- Automated copywriting tools like Jasper and Brandwell that generate persuasive ad copy in diverse tones—from playful to formal—optimized per segment.
- AI-powered creative asset generators producing image or video variations by adjusting colors, layouts, and messaging to resonate with subgroups.
- Personalized promotional offers dynamically adjusted based on predicted customer preferences and buying behaviors, boosting conversion potential.
The impact is tangible: JPMorgan Chase reported a 450% increase in clicks on AI-personalized ad copy, while Farfetch saw a 7% rise in email open rates deploying AI-driven personalized content. These successes exemplify a broader trend where generative AI accelerates creativity and precision simultaneously.
Yet, this power demands responsibility. Ethical considerations around authenticity and transparency are critical—especially as the boundary between human and machine-generated content continues to blur.
Feedback Loops and Continuous Learning: Refining Targeting Precision
What sets effective AI marketing systems apart is their capacity for continuous learning—closing the loop between campaign results and model refinement.
In practice, AI-driven campaigns monitor real-time engagement metrics such as clicks, conversions, and dwell time. This feedback informs ongoing model adjustments, recalibrating segment definitions, fine-tuning offers, and optimizing content delivery dynamically.
Benefits of this continuous learning approach include:
- Adaptive Targeting: Models evolve with shifting customer behaviors, maintaining relevance in fast-changing markets.
- Improved ROI: Iterative refinement focuses marketing spend on what truly resonates.
- Transparency and Trust: Techniques in explainable AI (XAI)—such as LIME and SHAP—help marketers and consumers understand targeting decisions, mitigating bias and privacy concerns.
For instance, The New York Times employs AI to schedule and distribute articles based on reader behavior, ensuring content reaches the right audience at the optimal moment. This dynamic personalization significantly boosts engagement and loyalty.
Privacy-preserving techniques like federated learning—where data remains on user devices and only model updates are shared—enhance user privacy while enabling model improvements. This approach is increasingly vital amid tightening data regulations.
In Summary: The AI-Powered Targeting Ecosystem
Together, advanced segmentation, generative content creation, and continuous learning form a synergistic ecosystem redefining customer targeting. Marketing is no longer about broad volume or guesswork; it’s about precision crafted through data insight, creativity, and iterative refinement.
For marketers, integrating AI-driven targeting tools is no longer optional—it’s essential for competitiveness and relevance. Success hinges on balancing technological innovation with ethical practices, transparency, and respect for consumer privacy.
Embracing this balanced approach enables brands to craft marketing experiences that feel personalized, timely, and genuinely human—even when powered by advanced AI systems.
Aspect | Description | Examples / Benefits |
---|---|---|
Advanced Customer Segmentation | AI uses behavioral patterns, preferences, and predictive signals to create micro-segments beyond basic demographics. |
|
Dynamic Content Generation | Generative AI creates personalized copy, images, and offers tailored to segments. |
|
Feedback Loops and Continuous Learning | AI systems use real-time engagement data to refine targeting models continuously. |
|
Analytics in AI Marketing: Measuring Effectiveness and Driving Optimization
Analytics in AI Marketing: Measuring Effectiveness and Driving Optimization
In today’s fragmented customer journeys, how can marketers accurately identify which channels and touchpoints truly drive conversions? Traditional attribution methods—like last-click or first-touch—often oversimplify a complex sequence of interactions, overlooking the subtle impacts of multiple engagements. AI-powered analytics are transforming this landscape, enabling much more precise and agile measurement of marketing effectiveness.
Advanced Attribution Models: Beyond the Last Click
Think of trying to assign credit for a touchdown in football by only looking at the final pass—you’d miss the blockers’ efforts, the running back’s dash, and strategic plays that set the stage. Similarly, AI-driven multi-touch attribution models assess all customer interactions leading up to a conversion, distributing credit across channels based on their actual influence.
AI enriches these models by:
- Aggregating massive datasets from both online (ad clicks, web visits) and offline (in-store visits, phone calls) touchpoints.
- Learning dynamically which interactions truly impact conversion, moving beyond static, rule-based approaches.
- Providing real-time attribution, empowering marketers to react promptly to shifting trends and channel performance.
Despite 76% of marketers still struggling with channel credit assignment, AI is demystifying multi-touch attribution for organizations of all sizes. Tools like Wicked Reports and Adinton combine AI with marketing mix modeling (MMM) and incrementality testing to deliver a comprehensive view of campaign effectiveness that extends beyond digital channels.
The bottom line? There’s no one-size-fits-all attribution model. However, AI’s adaptability and continuous refinement capabilities enable smarter budget allocation, minimize waste, and amplify ROI.
Real-Time Performance Tracking and Predictive Insights
Attribution explains what happened. Real-time tracking and predictive analytics reveal what’s next.
Modern AI systems continuously monitor campaigns, delivering critical metrics such as:
- Return on Ad Spend (ROAS): AI isolates the most revenue-efficient ads and creatives, often adjusting bids and placements autonomously.
- Conversion Uplift: By benchmarking AI-driven personalized campaigns against control groups, marketers quantify the incremental lift generated.
- Customer Lifetime Value (CLV): AI forecasts future customer value with fine granularity, surpassing reliance on historical averages.
Machine learning models analyze purchase history, demographics, and behavioral data to predict customers likely to churn, re-engage, or become high-value advocates. These forward-looking insights allow marketers to tailor retention efforts and allocate resources for maximum long-term impact.
Recent reports highlight that 65% of businesses have improved SEO outcomes through AI, and 75% of companies leveraging AI in marketing are pivoting towards strategic, predictive applications. This aligns with AI’s ability to synthesize complex datasets into actionable insights faster and more accurately than traditional analysis.
Integrating AI Analytics into Marketing Decision Engines
How do marketers translate sophisticated analytics into smarter, on-the-fly decisions? The key lies in embedding AI insights seamlessly into marketing decision engines that govern budget allocation, campaign automation, and creative optimization.
Leading AI platforms analyze ongoing performance data to:
- Identify underperforming creatives and low-ROI channels, automatically pausing or adjusting spend.
- Detect audience oversaturation, preventing wasted impressions and reducing customer fatigue.
- Recommend budget reallocations toward high-impact segments and channels based on predictive modeling.
This creates a continuous feedback loop where AI learns from outcomes to optimize marketing mix and media spend. As digital marketing expert Sara Vicioso observes, success in 2025 “is no longer about who can outspend the competition but about adapting quickly and making smarter decisions.”
Platforms like Salesforce Customer 360 and DataRobot unify customer data and provide AI-driven journey orchestration, enabling hyper-personalized experiences at scale. Automation tools such as AdCreative.ai generate optimized creatives informed by past successes, closing the loop between data insights and execution.
Balancing AI’s Promise with Practical Realities
While AI markedly enhances marketing analytics, it’s crucial to acknowledge its boundaries. Even the most advanced attribution models cannot fully account for offline influences like word-of-mouth referrals or trade show interactions. Predictive models require ongoing updates to stay aligned with evolving consumer behaviors and market dynamics.
Transparency and explainability remain challenges. Marketers must ensure AI-driven decisions comply with ethical standards and regulatory frameworks—particularly around data privacy—to maintain consumer trust.
Final Thoughts
AI-powered analytics are not merely improving measurement—they are revolutionizing how marketers optimize every marketing dollar. By deploying advanced attribution models, leveraging real-time performance data, and integrating predictive insights into agile decision engines, brands unlock unprecedented efficiency and personalization.
The future of marketing analytics hinges not on more data, but on smarter data—transformed into timely, actionable intelligence that empowers marketers to cultivate deeper customer relationships and sustainable growth.
Aspect | Description | Examples/Tools | Benefits |
---|---|---|---|
Advanced Attribution Models | AI-driven multi-touch attribution assessing all customer interactions to distribute credit across channels. | Wicked Reports, Adinton | Precise channel credit assignment, smarter budget allocation, ROI amplification. |
Real-Time Performance Tracking & Predictive Insights | Continuous monitoring of campaigns with predictive analytics forecasting future trends and customer behavior. | AI systems analyzing ROAS, Conversion Uplift, CLV | Optimized ad spend, quantified incremental lift, improved customer retention strategies. |
Integrating AI Analytics into Decision Engines | Embedding AI insights into marketing automation for budget allocation, campaign adjustment, and creative optimization. | Salesforce Customer 360, DataRobot, AdCreative.ai | Automatic spend adjustment, prevention of audience oversaturation, continuous campaign optimization. |
Balancing AI’s Promise with Practical Realities | Recognizing limitations such as offline influence gaps and the need for ongoing model updates. | Ethical and regulatory compliance considerations | Maintaining consumer trust, transparency, and data privacy adherence. |
Technical and Ethical Challenges in AI Marketing Personalization
Technical and Ethical Challenges in AI Marketing Personalization
How can marketers harness the power of hyper-personalization while navigating the rising demands for privacy, fairness, and trust? The balance between leveraging rich consumer data and respecting individual rights is no longer hypothetical—it is actively reshaping marketing strategies and the global regulatory environment.
Navigating Data Privacy and Transparency
The landscape of data privacy is evolving rapidly under the influence of robust regulations such as the EU’s GDPR, California’s CCPA, and emerging laws like the New Hampshire Privacy Act and Minnesota Consumer Data Protection Act. These frameworks have fundamentally changed how marketers collect, manage, and utilize data.
One major shift is the move away from heavy reliance on third-party data toward privacy-centric, first-party data strategies. This transition is not just about compliance—it has become a competitive differentiator, as brands that prioritize transparent, respectful data practices build stronger customer trust.
For example, Apple’s App Tracking Transparency framework empowers users to control data sharing, setting a high standard for privacy integration. According to Cisco’s 2025 Data Privacy Benchmark Study, 82% of consumers are more likely to engage with brands that clearly communicate how their data is used, and 79% are willing to share data if they trust it is handled responsibly.
AI itself plays a dual role in this space. On one hand, it enhances compliance by automating consent management, auditing data use, and dynamically adapting to privacy preferences. AI-powered cookie consent managers, for instance, scan websites for tracking technologies, block non-essential cookies until explicit user consent is given, and provide granular control—helping businesses meet stringent regulatory requirements without sacrificing personalization capabilities.
On the other hand, AI introduces new risks if not properly governed. Think of AI as a “brilliant but judgment-lacking intern” that requires human oversight. Transparency about AI’s role in personalization is critical. Brands that openly disclose AI involvement and explain decision-making processes foster user confidence. Explainable AI (XAI) frameworks, such as those leveraging techniques like LIME and SHAP, are emerging as best practices to provide transparency into algorithmic operations, addressing expectations for fairness and control.
The Risk of Algorithmic Bias and Manipulation
Algorithmic bias is a pressing and tangible challenge in AI marketing personalization. AI models trained on incomplete or skewed datasets can inadvertently reinforce stereotypes or unfairly target specific demographics, resulting in negative customer experiences and reputational harm.
For instance, studies have revealed AI systems misclassifying individuals based on gender or ethnicity—such as associating women disproportionately with homemaking or linking Black men unfairly with criminality. These biases undermine trust and may expose brands to legal liabilities.
The personal care brand Dove has publicly pledged not to use generative AI in their advertising, highlighting concerns about perpetuating harmful stereotypes through AI-generated content.
Mitigating these risks requires proactive governance, including:
- Establishing comprehensive ethical guidelines and AI governance frameworks.
- Conducting regular audits and impact assessments to detect and correct bias.
- Utilizing diverse, representative training datasets alongside inclusive development teams to reduce blind spots.
- Avoiding over-personalization that narrows content diversity and alienates segments of the audience.
Consumer sentiment reflects these concerns: 63% of customers express worry about bias and discrimination in AI-driven algorithms. Ignoring these issues risks eroding loyalty and engagement.
Technical Challenges: Integration, Data Quality, and Multi-Channel Personalization
Behind the scenes, integrating AI tools into existing marketing ecosystems presents significant technical complexity. Organizations often grapple with data interoperability, ensuring AI systems seamlessly communicate with CRM platforms, content management systems, and analytics tools.
A foundational requirement for effective AI-driven personalization is clean, high-quality data. Gartner notes that the majority of AI initiatives falter due to poor data quality—issues such as inaccuracies, incompleteness, or stale information can lead AI models to generate irrelevant recommendations or flawed customer insights. Conversely, high-quality data can improve AI insight accuracy by up to 80%, directly enhancing campaign ROI and customer satisfaction.
Multi-channel personalization further complicates the picture. Customers interact with brands across myriad touchpoints—email, social media, mobile apps, websites, and offline channels—creating fragmented and diverse data streams. Delivering a seamless, consistent personalized experience demands:
- Centralized data integration platforms that unify customer profiles into a single source of truth.
- Automated workflows to maintain message consistency and reduce manual errors.
- Analytics tools that monitor channel performance and inform real-time budget allocation.
Brands mastering multichannel personalization report up to a 60% increase in digital revenue and a 33% uplift attributed to consistent messaging across platforms.
Balancing Act: Ethics, Trust, and Technical Excellence
The convergence of ethical imperatives and technical challenges defines the frontier of AI marketing personalization. Ethical AI—centered on transparency, fairness, and respect for privacy—is not merely regulatory obligation but a strategic asset that drives customer loyalty and brand reputation.
Simultaneously, marketing teams must commit to robust data governance, maintain pristine data foundations, and ensure seamless integration of AI tools with existing systems. This balance is essential to fully unlock AI’s potential without compromising customer trust.
Operationalizing this balance involves:
- Engaging compliance, legal, and ethical experts throughout AI deployment.
- Implementing explainable AI tools to demystify personalization decisions.
- Continuously monitoring AI outputs for bias, inaccuracies, and operational drift.
- Embedding privacy-centric design principles from the ground up.
Successful brands treat AI not as a magic wand but as a powerful, nuanced tool requiring careful stewardship—combining human judgment, ethical rigor, and technical precision to deliver authentic, personalized experiences that customers can trust.
Category | Challenge | Details | Examples/Statistics | Mitigation Strategies |
---|---|---|---|---|
Data Privacy & Transparency | Regulatory Compliance | Adapting to GDPR, CCPA, and emerging privacy laws affecting data collection and usage. | Apple’s App Tracking Transparency; 82% consumers engage more with transparent brands (Cisco 2025 study). | Shift to first-party data; transparent data practices; AI-powered consent management tools. |
Consumer Trust | Building trust through clear communication about data use. | 79% willing to share data if handled responsibly. | Open disclosure of AI role; explainable AI frameworks (LIME, SHAP). | |
AI Role | Dual role of AI in compliance and risk introduction. | Automates consent management but needs human oversight to avoid opaque decision-making. | Use Explainable AI (XAI); human supervision. | |
Transparency | Need for transparency about AI personalization processes. | Consumers expect fairness and control over algorithms. | Implement Explainable AI; disclose AI involvement. | |
Privacy Integration | Embedding privacy into marketing tech stack. | Privacy as competitive differentiator. | Privacy-centric design principles; AI cookie consent managers. | |
Algorithmic Bias & Manipulation | Bias in AI Models | Skewed or incomplete training data reinforcing stereotypes. | Misclassification examples: gender roles, racial profiling. | Diverse data; inclusive teams; regular audits. |
Reputational Risks | Harm from biased or discriminatory AI outputs. | Dove avoids generative AI to prevent stereotypes. | Ethical guidelines; AI governance frameworks. | |
Consumer Concern | Worries about bias and fairness. | 63% customers worry about bias in AI algorithms. | Impact assessments; bias detection and correction. | |
Over-Personalization | Risk of narrowing content diversity and alienating users. | May reduce engagement and loyalty. | Balanced personalization strategies. | |
Legal Liabilities | Potential legal issues from discriminatory AI. | Brands must avoid unfair targeting or profiling. | Compliance with laws; transparent AI practices. | |
Technical Challenges | Integration Complexity | Connecting AI tools with CRM, CMS, analytics. | Requires seamless interoperability. | Centralized data platforms; automated workflows. |
Data Quality | Need for clean, accurate, complete data. | Poor data leads to irrelevant recommendations. | Data governance; ongoing data cleansing. | |
Multi-Channel Personalization | Fragmented customer touchpoints complicate personalization. | Channels: email, social media, apps, websites, offline. | Unified profiles; consistent messaging; real-time analytics. | |
Performance Impact | Effectiveness tied to data and integration quality. | High-quality data improves AI accuracy by up to 80%. | Invest in data quality and integration tech. | |
Ethics, Trust & Excellence | Ethical AI | Transparency, fairness, privacy as strategic assets. | Enhances brand reputation and loyalty. | Ethical guidelines; transparent AI use. |
Governance | Need for compliance and ethical oversight. | Involvement of legal and ethical experts. | Continuous monitoring; explainability tools. | |
Operational Balance | Combining human judgment with AI capabilities. | AI is a nuanced tool, not a magic wand. | Embed privacy-centric design; human oversight. | |
Customer Trust | Critical for adoption and engagement. | Trust depends on fairness and privacy respect. | Open communication; bias mitigation; transparent personalization. |
Comparative Insights: Traditional Marketing vs. AI-Enhanced Personalization Approaches
Comparative Insights: Traditional Marketing vs. AI-Enhanced Personalization Approaches
How do AI-driven personalization strategies truly stack up against traditional marketing methods? The answer lies in a nuanced blend of scale, speed, and customization depth—dimensions where AI often redefines the playing field without rendering classical approaches obsolete.
Scale and Speed: AI’s Unmatched Advantage
Traditional marketing has long excelled at broad reach—think TV spots, print ads, and billboards designed for mass audiences. These methods, however, inherently lack the agility and granularity demanded by today’s consumers.
In contrast, AI-powered marketing systems analyze millions of data points in real time, enabling hyper-personalization at scale. For instance, Zalando leverages generative AI to rapidly produce fresh visual content across 25 European markets, responding swiftly to fleeting fashion trends. Such responsiveness is virtually impossible with traditional creative pipelines, which are slower and resource-intensive.
Similarly, platforms like Tavus employ AI to generate thousands of personalized videos simultaneously—each tailored automatically to individual customer data without manual input. This exemplifies how AI transforms marketing from one-to-many broadcasts into one-to-one dialogues, all at massive scale.
Statistics underscore this shift:
- 75% of companies using AI in marketing report reallocating resources toward strategic activities due to automation.
- 65% observe improved SEO outcomes after integrating AI tools.
- The AI marketing sector is projected to grow at a 26.7% CAGR through 2034.
These efficiency and effectiveness gains highlight AI’s role as a catalyst for marketing evolution.
Depth of Customization: Moving Beyond Demographics
Traditional segmentation—based on age, gender, or location—only scratches the surface of personalization. AI dives deeper by synthesizing behavioral patterns, purchase history, engagement channels, and even emerging preferences predicted through machine learning.
Retailers using AI-driven personalization report 10% to 25% increases in return on ad spend (ROAS). This is no accident. By fusing zero-, first-, and third-party data into holistic, real-time customer profiles, brands tailor communications with unprecedented precision.
For example, Shopify’s AI tools enable merchants to segment customers using everyday language queries, simplifying complex analytics and enhancing targeting accuracy. Netflix and Amazon’s AI-powered recommendation engines further demonstrate deep personalization by anticipating user preferences, fostering long-term engagement.
However, AI personalization thrives on vast, high-quality datasets and robust technical infrastructure—a luxury not all businesses possess. Smaller companies or those with limited data maturity may still find traditional analytics and marketing intuition valuable, especially when combined with AI insights in a hybrid approach.
Case Studies: Retail, Media, and E-Commerce in Action
Retail
In retail, the transformation is tangible. Shark Ninja integrates AI-powered user-generated content (UGC) to showcase authentic customer experiences, enhancing social proof and engagement. This dynamic, consumer-centric storytelling contrasts with traditional static advertising, illustrating a shift toward authenticity and responsiveness.
Media
Media companies are adapting rapidly. Deloitte reports that social video platforms have surpassed traditional cable and satellite TV subscriptions, particularly among younger audiences. AI algorithms curate personalized content feeds that traditional media cannot match, fostering stronger parasocial connections with creators—an engagement level impossible through mass-market TV ads.
E-Commerce
E-commerce exemplifies AI’s impact vividly. Sephora employs AI-driven beauty recommendations and virtual fitting rooms to personalize shopping journeys, reducing product returns and boosting conversion rates. AI also enables dynamic pricing adjustments based on demand and customer behavior, a flexibility unattainable with traditional pricing strategies.
Limitations and Complementary Roles of Traditional Marketing
Despite AI’s transformative capabilities, it is not a panacea. Over-personalization can risk alienating customers or diluting brand identity by creating inconsistent experiences. As CMSWire advises, the 1:1 marketing ideal should guide rather than dictate strategy.
Privacy and ethical considerations further constrain AI use. With only about 50% of consumers trusting companies to secure their personal data, transparency and responsible AI practices are paramount. Complex regulatory environments demand marketers balance personalization with robust privacy protections.
Traditional marketing retains strengths in brand awareness, credibility, and reaching demographics less engaged online or resistant to digital targeting. For example, sectors like automotive and real estate continue to benefit from billboards and TV ads to build trust and local presence.
The most effective marketing strategies in 2025 integrate AI and traditional methods, leveraging each where they excel. Community marketing—fostering genuine relationships through collaborative and user-generated content—complements AI’s data-driven precision by enhancing engagement and loyalty at lower acquisition costs.
Strategic Takeaways
- AI excels in delivering hyper-personalized experiences rapidly and at scale, driving measurable improvements in ROI, customer engagement, and operational efficiency.
- Traditional marketing remains valuable for brand building, broad awareness, and segments less accessible via digital channels, especially where emotional resonance and trust are paramount.
- Hybrid approaches that combine AI’s analytical power with human creativity and traditional media’s reach create the most balanced and effective marketing ecosystems.
- Ethical AI use and data privacy considerations must underpin personalization efforts to maintain consumer trust and comply with evolving regulations.
In sum, AI-driven personalization represents an evolutionary leap rather than a wholesale replacement of traditional marketing. When thoughtfully integrated, it amplifies the strengths of classical methods, empowering marketers to harness precision and scale without sacrificing human connection and brand integrity. The future belongs to those who master this synergy.
Aspect | Traditional Marketing | AI-Enhanced Personalization |
---|---|---|
Scale and Speed | Broad reach via TV, print, billboards; slower, resource-intensive creative pipeline | Real-time analysis of millions of data points; hyper-personalization at massive scale; rapid content generation (e.g., Zalando, Tavus) |
Customization Depth | Segmentation by demographics (age, gender, location); limited personalization | Deep personalization using behavioral data, purchase history, engagement channels; real-time customer profiles (e.g., Shopify, Netflix, Amazon) |
Examples in Retail | Static advertising, broad messaging | AI-powered user-generated content enhancing authenticity and engagement (e.g., Shark Ninja) |
Examples in Media | Mass-market TV ads, cable/satellite dominance | Personalized content feeds via AI; stronger parasocial connections; social video platforms surpass traditional TV (Deloitte) |
Examples in E-Commerce | Standard pricing and generic recommendations | AI-driven beauty recommendations, virtual fitting rooms, dynamic pricing (e.g., Sephora) |
Limitations | Less adaptable, slower response; limited granularity | Requires large quality datasets and infrastructure; risk of over-personalization; privacy and ethical concerns |
Strengths | Brand awareness, credibility, trust; effective for demographics less engaged online | Hyper-personalized experiences; operational efficiency; improved ROI and engagement |
Strategic Role | Brand building, broad awareness, emotional resonance | Data-driven precision, rapid scalability, complex targeting |
Integration Approach | Valuable in hybrid strategies combining creativity and reach | Complements traditional methods to create balanced marketing ecosystems |
Future Trajectories: Emerging AI Innovations and Their Impact on Marketing Personalization
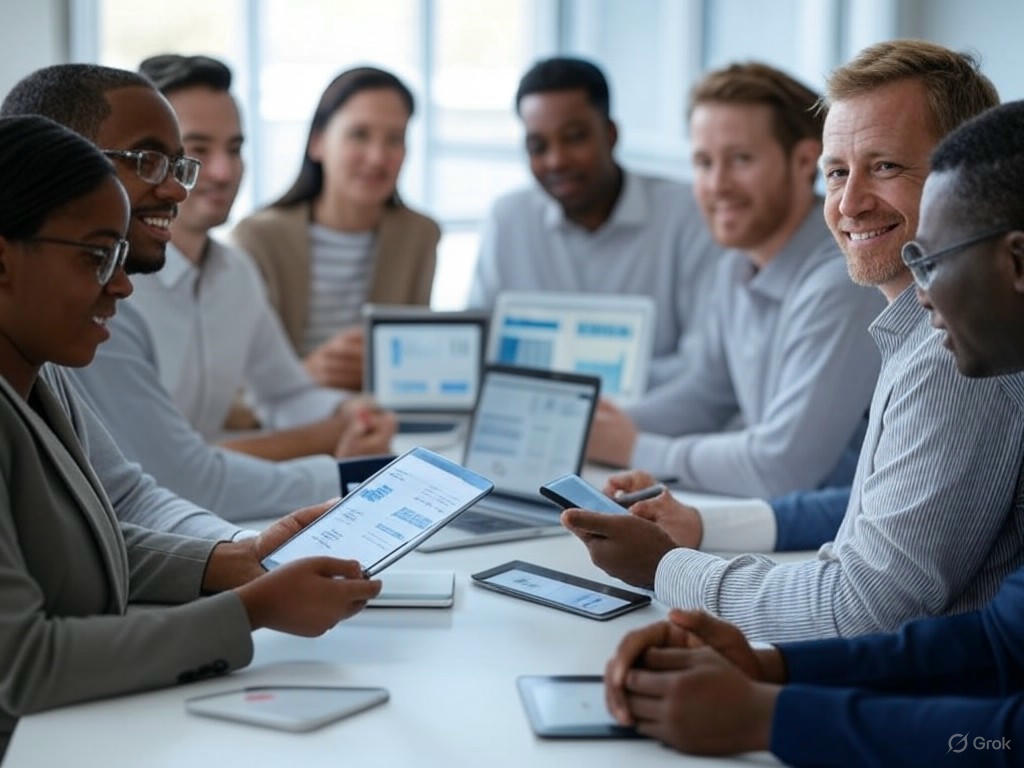
Future Trajectories: Emerging AI Innovations and Their Impact on Marketing Personalization
Imagine a world where marketing anticipates your needs in real time, seamlessly across all your devices, while fully honoring your privacy and values. This vision is no longer a distant dream but the accelerating trajectory of AI-driven marketing personalization. Navigating this evolving landscape demands a clear understanding of emerging AI technologies, shifting consumer expectations, and robust ethical frameworks that ensure personalization remains both powerful and principled.
Foundation Models and Real-Time Adaptive Personalization
At the forefront of this transformation are foundation models—large, versatile AI frameworks trained on vast datasets capable of generalizing across multiple tasks and domains. These models underpin real-time adaptive personalization, empowering marketers to deliver hyper-relevant content and offers at unmatched speed and scale.
Platforms like Zeta leverage AI not merely for customer segmentation but to deeply understand intent, enabling brands to:
- Optimize marketing spend by focusing on the most promising prospects
- Enhance engagement through messaging that resonates personally
- Foster loyalty by anticipating individual preferences and needs
- Predict and proactively reduce customer churn
According to McKinsey, generative AI accelerates content creation, producing finely tuned messages tailored to subgroups beyond traditional demographic categories. As marketing teams integrate foundation models, they establish continuous feedback loops where AI learns from customer interactions, constantly refining personalization.
A tangible example is Wix’s launch of an AI-powered adaptive content tool. It utilizes real-time session data to dynamically generate content aligned with visitor behavior within a single browsing session. This on-the-fly adaptation revolutionizes web personalization, driving engagement and conversions in ways static content cannot.
Cross-Device Identity Resolution: The Key to Seamless Personalization
A significant challenge in personalization is creating a unified customer profile from interactions occurring across multiple devices, channels, and offline touchpoints. Without this comprehensive view, personalization risks feeling disjointed or irrelevant.
Advancements in identity resolution technologies—employing probabilistic and deterministic matching—are addressing this challenge by merging disparate data points into unified profiles. Research by Spiceworks and Data Axle highlights that effective identity resolution enables marketers to:
- Deliver consistent personalized experiences across mobile, desktop, in-store, and call center touchpoints
- Optimize advertising spend by preventing redundant or conflicting messaging
- Detect fraud and anomalies by linking activity across channels
Customer Data Platforms (CDPs) like Klaviyo, combined with composable data architectures, empower marketers to unify and activate customer data in real time. Despite this, only 17% of marketers report high CDP utilization, underscoring integration complexities.
As data volume grows, fragmented customer views cause missed signals and lost opportunities. Unified data platforms emerge as essential solutions, breaking down silos to enable smarter, privacy-conscious, AI-driven decision-making that delivers seamless customer experiences.
Evolving Consumer Expectations and Regulatory Realities
Today’s consumers expect more than generic offers—they demand authenticity, relevance, and respect for their privacy. Research shows that 65% of customers identify targeted promotions as a primary purchase driver, yet they simultaneously seek transparency and control over their data.
On the regulatory front, the landscape is tightening. U.S. state laws such as California’s Privacy Protection Agency regulations and Texas’s HB 1709 for “high-risk AI systems” impose new requirements for transparency, accountability, and fairness. Organizations must navigate a complex patchwork of rules mandating:
- Clear disclosures about AI’s use of personal data
- Rigorous audits to detect and mitigate bias in AI-driven marketing
- Human oversight to validate AI outputs and intervene when necessary
This regulatory momentum aligns with ethical AI principles critical for maintaining consumer trust. Ethical AI design transcends compliance—it requires a commitment to fairness, avoidance of manipulative tactics, and ensuring AI complements rather than replaces human creativity and judgment.
Human-AI Collaboration: The Next Frontier in Marketing Personalization
Despite rapid AI advancements, human expertise remains indispensable. The future of marketing personalization is not AI versus humans, but humans empowered by AI. AI automates routine workflows and complex data analysis, liberating marketers to focus on strategic storytelling, ethical decision-making, and brand building.
Leading organizations are establishing “AI-powered innovation centers” within marketing teams that blend data science, creative strategy, and domain expertise. This collaborative approach ensures AI tools deliver measurable ROI while upholding brand values and consumer sensibilities.
Moreover, as highlighted by McKinsey and Workday, human skills such as collaborative problem-solving and ethical oversight will grow alongside AI capabilities. Marketers who master this synergy will unlock personalization’s full potential—delivering experiences that feel both individually crafted and authentically human.
Key Takeaways
- Foundation models and generative AI enable real-time, intent-driven personalization at scale, fundamentally changing customer engagement.
- Cross-device identity resolution is crucial for unified customer profiles, though effective integration remains a challenge.
- Consumer demand for personalized experiences coexists with heightened expectations for privacy and transparency, reinforced by evolving regulations.
- Ethical AI frameworks and human-AI collaboration are strategic imperatives essential for sustainable, trustworthy marketing success.
Looking forward, brands that thrive will be those who harness AI’s power thoughtfully—melding cutting-edge technology with deep respect for the human experience. The future of personalization lies not just in smarter algorithms but in smarter, more conscientious marketing.
Emerging AI Innovation | Impact on Marketing Personalization | Examples/Notes |
---|---|---|
Foundation Models & Real-Time Adaptive Personalization | Enables hyper-relevant content and offers at speed and scale; deep understanding of intent; continuous feedback loops refine personalization | Zeta platform for intent understanding; Wix AI-powered adaptive content tool for dynamic session-based personalization |
Cross-Device Identity Resolution | Creates unified customer profiles across devices/channels; enables consistent experiences; optimizes ad spend; detects fraud | Probabilistic and deterministic matching; CDPs like Klaviyo; only 17% high CDP utilization due to integration challenges |
Evolving Consumer Expectations & Regulatory Realities | Demand for authenticity, relevance, privacy; need for transparency, control; compliance with state laws on AI and data use | 65% customers driven by targeted promotions; California Privacy Protection Agency; Texas HB 1709; requirements for AI disclosure, audits, human oversight |
Human-AI Collaboration | Combines AI automation with human creativity and ethical oversight; enables strategic storytelling and brand building | AI-powered innovation centers blending data science and creative strategy; emphasis on collaborative problem-solving and ethical marketing |