AI in Manufacturing: Automation, Quality Control & Predictive Maintenance
- Introduction: Why AI is a Game Changer for Modern Manufacturing
- Introduction: Why AI is a Game Changer for Modern Manufacturing
- The Transformative Impact of AI Amid Rising Complexity and Competition
- Broad Applications: Automation, Quality Control, and Predictive Maintenance
- Balancing Promise with Practical and Ethical Challenges
- Key Questions Ahead
- Foundations of AI in Manufacturing: Core Technologies and Architectures
- Foundations of AI in Manufacturing: Core Technologies and Architectures
- Core AI Technologies: Machine Learning, Deep Learning, Neural Networks, and Generative AI
- Sensor Integration, Data Acquisition, and Edge Computing Architectures
- Conceptual Frameworks: Digital Twins, Self-Organizing Systems, and Human-AI Collaboration
- Key Takeaways
- AI-Driven Automation: From Repetitive Tasks to Lights-Out Factories
- AI-Driven Automation: From Repetitive Tasks to Lights-Out Factories
- From Assisted Robotics to Autonomous Operations
- Generative AI: A Game-Changer for Setup and Flexibility
- Technical Challenges: Integration, Reliability, and Workforce Transition
- Final Thoughts
- Enhancing Quality Control through AI: Precision, Anomaly Detection, and Real-Time Feedback
- Enhancing Quality Control through AI: Precision, Anomaly Detection, and Real-Time Feedback
- AI-Driven Computer Vision: From Detection to Prevention
- Predictive Analytics and Acoustic Monitoring: Detecting Trouble Before It Strikes
- Closed-Loop Feedback Systems: From Insight to Instant Action
- Performance Metrics and Benchmarks: Gatekeepers of Industrial Adoption
- Final Thoughts
- Predictive Maintenance: Reducing Downtime with AI-Based Prognostics
- Predictive Maintenance: Reducing Downtime with AI-Based Prognostics
- How AI Anticipates Equipment Health: Core Techniques in Predictive Maintenance
- Real-World Impact: Successful AI Predictive Maintenance Deployments
- Overcoming Barriers: Challenges in Implementing AI Predictive Maintenance
- The Road Ahead: Strategic Value and Cautions in AI Predictive Maintenance
- Comparative Analysis: AI Adoption Across Manufacturing Sectors and Scale
- Comparative Analysis: AI Adoption Across Manufacturing Sectors and Scale
- AI Maturity Across Key Manufacturing Sectors
- SMEs vs. Multinationals: Diverging Paths to AI Gains
- Barriers to Adoption: Skill Shortages, Cybersecurity, and Investment Risks
- Best Practices and Lessons Learned
- Future Outlook: Emerging Trends, Ethical Considerations, and Societal Impacts
- Future Outlook: Emerging Trends, Ethical Considerations, and Societal Impacts
- Emerging AI Innovations: From Augmented Reality to Digital Twins and Conversational Interfaces
- Ethical Considerations: Workforce Displacement, Data Privacy, and AI Transparency
- Societal Impacts: Sustainability Gains and Strategic Pathways Forward
- Strategic Recommendations for Manufacturers Navigating AI’s Complex Landscape
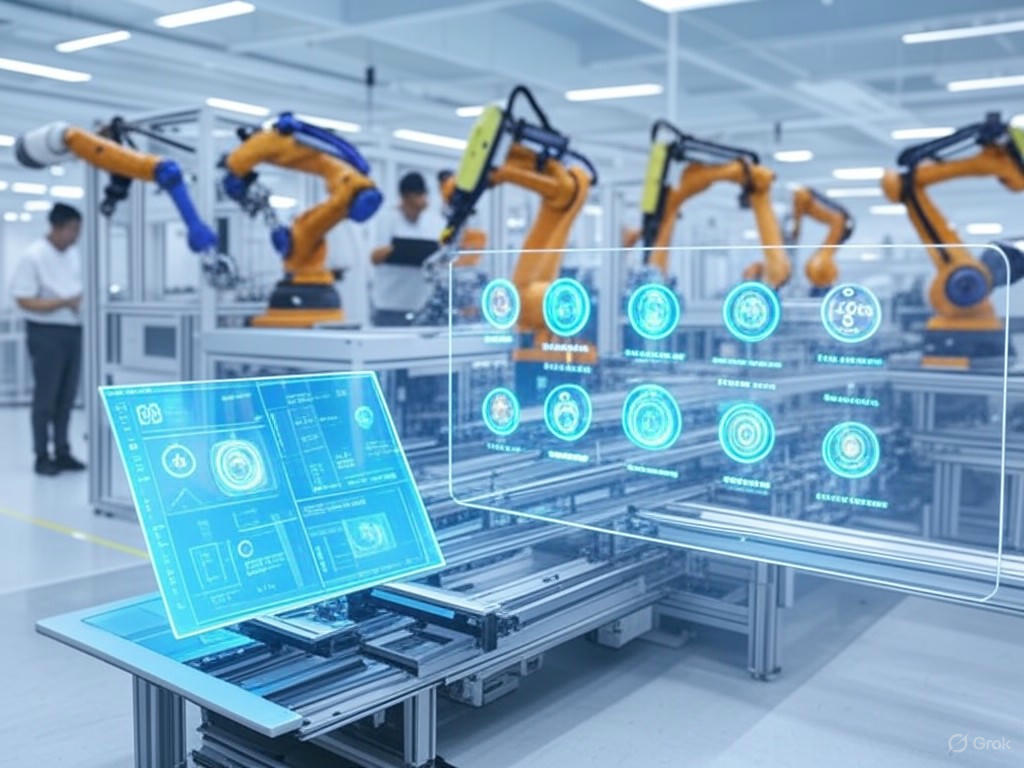
Introduction: Why AI is a Game Changer for Modern Manufacturing
Introduction: Why AI is a Game Changer for Modern Manufacturing
What if you could predict a machine failure before it halts your entire production line? Or instantly detect microscopic defects invisible to the human eye, ensuring every product meets flawless quality standards? This is no longer a futuristic vision—artificial intelligence (AI) is rapidly transforming manufacturing in ways previously unimaginable.
The Transformative Impact of AI Amid Rising Complexity and Competition
Manufacturing today faces unprecedented complexity: global supply chains stretched thin, rising costs, and intense competition demanding faster innovation and superior quality. According to PwC’s 2025 AI Business Predictions, AI is becoming a critical driver of global manufacturing competitiveness. Companies that leverage AI to optimize operations are positioned to lead in this challenging landscape.
However, achieving AI maturity remains a significant hurdle. McKinsey’s research reveals that while 92% of manufacturers plan to increase AI investments over the next three years, only 1% consider themselves mature in AI deployment. This discrepancy highlights both the immense promise and the practical challenges of AI adoption—realizing AI’s full potential requires strategic focus and capability development.
Deloitte’s 2025 Manufacturing Industry Outlook further underscores this dynamic. Despite economic headwinds such as rising interest rates and supply chain disruptions like the Panama Canal drought, manufacturers continue to invest heavily in digital cores and AI-driven initiatives. More than half (55%) of industrial manufacturers are already leveraging generative AI tools, with over 40% planning to increase investments in AI and machine learning. These commitments reflect a growing belief that AI not only boosts efficiency but also enhances agility and resilience in uncertain environments.
Broad Applications: Automation, Quality Control, and Predictive Maintenance
AI’s influence extends across several critical manufacturing domains:
-
Automation: AI-powered industrial robots and collaborative robots (cobots) are evolving beyond repetitive tasks. Equipped with intelligent sensors, they adapt in real time to complex factory environments and collaborate safely alongside human workers. For instance, Nissan’s AI-integrated robots have revolutionized vehicle assembly, improving both precision and throughput.
-
Quality Control: AI-driven visual inspection systems detect defects at scales beyond human perception, enabling manufacturers like Foxconn to maintain high-quality output efficiently. These systems employ deep learning to analyze sensor data and identify subtle anomalies that traditional inspection methods might overlook.
-
Predictive Maintenance: One of AI’s most impactful applications is predictive maintenance. By continuously monitoring equipment health through extensive sensor networks, AI can forecast failures days or weeks in advance. This capability reduces downtime by up to 50% and cuts maintenance costs by 25%, according to BizTech Magazine. Siemens and Boeing exemplify this approach, using AI analytics to preemptively address machinery issues, ensuring smoother operations and substantial cost savings.
These examples illustrate a broader industry shift toward smart factories, where AI, IoT, and edge computing converge to create dynamic, self-optimizing production environments.
Balancing Promise with Practical and Ethical Challenges
Despite AI’s compelling benefits, adoption is complex. Deloitte’s 2025 Smart Manufacturing Survey reveals that manufacturers face strategic risks, talent shortages, and cybersecurity challenges as they integrate AI. While more than half have established dedicated smart manufacturing teams, only about a third engage external partners for change management support. This indicates that AI adoption is not merely a technical upgrade but a cultural and organizational transformation.
Ethical considerations introduce additional complexity. Algorithmic bias, data privacy, and transparency directly impact trust and workforce inclusion on the factory floor. Biased AI models can inadvertently perpetuate inequities in workforce management or quality assessments. Ensuring fairness demands diverse, representative datasets and continuous human oversight.
Cybersecurity risks also escalate as factories become increasingly connected. AI systems managing sensitive operational data are attractive targets for malicious actors, potentially compromising safety and intellectual property. Manufacturers must prioritize robust security frameworks alongside AI deployment to safeguard their operations.
Key Questions Ahead
As AI’s role in manufacturing deepens, several critical questions emerge:
- How can manufacturers measure and maximize return on AI investments amid competing priorities?
- What governance frameworks ensure AI systems remain transparent, fair, and accountable in high-stakes industrial environments?
- How will workforce skills evolve alongside increasingly autonomous and intelligent machines?
- Can AI contribute to sustainability goals without exacerbating energy consumption, especially given the growing demand for AI-driven data centers?
Addressing these questions requires a balanced approach. Enthusiasm for AI’s capabilities must be tempered with rigorous evaluation and ethical vigilance. Only then can AI fulfill its promise as a true game changer—driving innovation, efficiency, and resilience in modern manufacturing while preserving human values and societal trust.
Aspect | Details |
---|---|
AI Adoption Statistics | 92% of manufacturers plan to increase AI investments; only 1% consider themselves mature in AI deployment (McKinsey) |
Current AI Usage | 55% of industrial manufacturers use generative AI tools; 40% plan to increase AI/ML investments (Deloitte) |
Applications of AI | Automation, Quality Control, Predictive Maintenance |
Automation Example | Nissan’s AI-integrated robots improving vehicle assembly precision and throughput |
Quality Control Example | Foxconn’s AI-driven visual inspection systems detecting microscopic defects |
Predictive Maintenance Impact | Reduces downtime by up to 50% and maintenance costs by 25% (BizTech Magazine); examples include Siemens and Boeing |
Challenges | Strategic risks, talent shortages, cybersecurity challenges, ethical issues like bias and privacy (Deloitte) |
Ethical Concerns | Algorithmic bias, data privacy, transparency, need for diverse datasets and human oversight |
Cybersecurity Risks | Increased vulnerability due to connected AI systems managing sensitive operational data |
Key Questions | Measuring AI ROI, governance for transparency and fairness, workforce skill evolution, AI sustainability impact |
Foundations of AI in Manufacturing: Core Technologies and Architectures
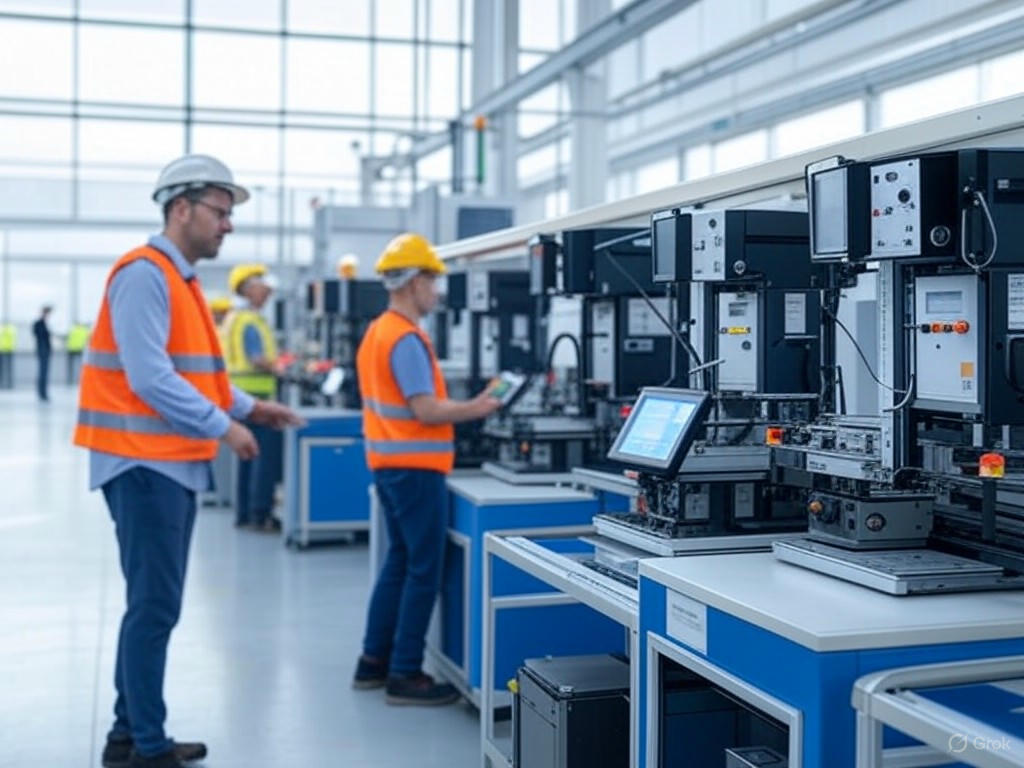
Foundations of AI in Manufacturing: Core Technologies and Architectures
The smart factory of today—and tomorrow—is powered by a sophisticated blend of AI technologies, sensor networks, and computing architectures designed to deliver real-time insights and autonomous actions. To fully understand this transformation, we begin by exploring the foundational AI technologies that are reshaping manufacturing operations.
Core AI Technologies: Machine Learning, Deep Learning, Neural Networks, and Generative AI
At the heart of AI in manufacturing lies machine learning (ML), enabling systems to learn from data and improve autonomously. A prime example is predictive maintenance, where ML algorithms analyze sensor data to detect subtle anomalies before equipment failure occurs. This predictive capability significantly reduces costly downtime and prolongs asset life. For instance, Siemens integrates generative AI into its predictive maintenance workflows, automating early defect detection and optimizing maintenance scheduling.
Beyond maintenance, ML enhances quality control by processing visual data from production lines to identify defects in real time. This task increasingly leverages deep learning neural networks, which emulate human pattern recognition but execute at greater scale and speed. Such networks are ideal for high-throughput inspection scenarios, enabling manufacturers like Foxconn to maintain stringent quality standards.
Generative AI is emerging rapidly as a transformative force. Beyond generating novel product designs—as BMW does for optimized automotive components—generative AI dynamically adjusts manufacturing parameters like temperature and pressure to optimize quality on the fly. This co-creative approach accelerates prototyping and customization, positioning AI as an active collaborator with human engineers rather than a mere tool.
Robotics, too, are evolving. AI-equipped robots transition from static automatons to perceptive agents capable of sensing their surroundings and adapting actions in real time. Autonomous guided vehicles (AGVs) and robotic arms now incorporate ML models to navigate complex factory floors and manipulate parts with precision, enhancing flexibility while reducing manual intervention.
Together, these AI technologies are forging a living, learning manufacturing ecosystem that drives efficiency, quality, and innovation.
Sensor Integration, Data Acquisition, and Edge Computing Architectures
AI’s effectiveness hinges on the quality and timeliness of the data it ingests, starting at the sensor level. Manufacturing sensors act as the factory’s “eyes and ears,” capturing critical parameters such as pressure, temperature, vibration, and imagery. The pressure sensor market, projected to reach $17 to $20 billion by 2025, underscores their pivotal role.
However, integrating a diverse array of sensors—spanning both legacy machinery and modern equipment—into seamless data streams presents challenges. Brownfield deployments retrofit sensors onto existing infrastructure, confronting a mosaic of protocols and standards. Addressing this requires robust industrial data acquisition systems that can collect, filter, and preprocess sensor data in real time.
This is where edge computing becomes essential. By processing data closer to its source—directly on the factory floor—edge computing reduces latency and bandwidth usage. Instead of transmitting raw data continuously to centralized cloud servers, edge nodes perform preliminary analysis, anomaly detection, and immediate decision-making locally. This architecture is vital for latency-sensitive operations such as robotic control and emergency shutdowns.
Edge computing is rapidly maturing and is expected to rival cloud platforms in agility and flexibility by 2025. The deployment of private 5G networks enhances this ecosystem by providing reliable, high-speed connectivity that enables seamless integration between edge nodes and cloud infrastructure.
An illustrative analogy is to envision the factory as a network of smart neighborhoods (edges), each empowered to make local decisions independently, yet connected to a central city center (cloud) for broader coordination and strategic planning.
Conceptual Frameworks: Digital Twins, Self-Organizing Systems, and Human-AI Collaboration
The concept of the digital twin embodies the convergence of physical and virtual manufacturing worlds. A digital twin is a high-fidelity, near-real-time digital replica of physical assets or processes. Analogous to a sophisticated flight simulator, it allows manufacturers to simulate, monitor, and predict system behavior without interrupting actual operations. NASA’s Apollo missions pioneered this concept decades ago, and today, companies like BMW employ digital twins to simulate factory workflows, optimize production, and preempt bottlenecks.
Digital twins vary in complexity and purpose—from prototypes to live operational models and aggregated simulations—serving distinct decision-making layers. Their effectiveness depends on balancing complexity: overly simplistic twins offer limited insight, while excessively detailed ones risk overwhelming users with data.
Building on this, self-organizing systems represent a paradigm where multiple autonomous agents coordinate actions without centralized control. Imagine a swarm of robots on a factory floor dynamically adapting to changes and collaborating efficiently, akin to natural systems such as ant colonies. This emergent behavior offers resilience, scalability, and adaptability in manufacturing operations.
Yet, technology alone is insufficient. Data quality is paramount—garbage in, garbage out remains a guiding principle. Interoperability standards like the Unified Namespace facilitate seamless communication across disparate systems, breaking down silos that otherwise fragment data and hinder AI effectiveness.
Equally crucial are human-AI collaboration frameworks. AI excels in specialized, well-defined tasks but depends on human expertise to provide context, interpret ambiguous situations, and set ethical boundaries. Frameworks such as SmythOS emphasize trust, transparent communication, and adaptive learning between human operators and AI agents.
This collaborative model transforms operators into orchestrators who supervise AI systems, focusing on strategic and high-level decisions. The future manufacturing workforce will not be replaced by AI but empowered to work smarter and innovate faster.
Key Takeaways
-
Machine learning, deep learning, and generative AI are foundational to manufacturing’s AI transformation, enabling predictive maintenance, real-time quality control, and dynamic process optimization.
-
Effective sensor integration and edge computing architectures are critical, ensuring real-time data acquisition and decision-making while overcoming challenges of legacy equipment and data fragmentation.
-
Digital twins offer virtual mirrors of factory assets, supporting simulation and proactive optimization, while self-organizing systems introduce scalable, autonomous coordination akin to natural swarms.
-
High data quality, interoperability standards, and robust human-AI collaboration frameworks are essential to unlock AI’s full potential responsibly and effectively in manufacturing.
These foundational technologies and architectures form the gears and circuits powering the smart factories of the future. Their successful deployment demands careful design, ethical oversight, and ongoing partnership between humans and machines.
Category | Technology/Concept | Description | Examples/Applications |
---|---|---|---|
Core AI Technologies | Machine Learning (ML) | Enables systems to learn from data and improve autonomously; used for predictive maintenance and quality control. | Siemens uses ML for predictive maintenance; Foxconn uses ML for defect detection in quality control. |
Deep Learning Neural Networks | Emulates human pattern recognition at scale and speed; ideal for high-throughput inspection. | Used by Foxconn for real-time defect identification in production lines. | |
Generative AI | Generates novel designs and dynamically optimizes manufacturing parameters like temperature and pressure. | BMW uses generative AI for automotive component design and process optimization. | |
AI-Equipped Robotics | Robots with perception and adaptation abilities, incorporating ML for navigation and manipulation. | Autonomous guided vehicles (AGVs) and robotic arms with ML models for precision and flexibility. | |
Living, Learning Ecosystem | Integration of AI technologies creating adaptive, efficient, and innovative manufacturing environments. | General concept of smart factories powered by AI technologies. | |
Sensor Integration & Edge Computing | Sensor Networks | Capture critical parameters like pressure, temperature, vibration, and imagery for real-time data. | Pressure sensor market projected at $17-20 billion by 2025. |
Industrial Data Acquisition Systems | Collect, filter, and preprocess sensor data from legacy and modern equipment in real time. | Used in brownfield deployments to integrate diverse sensors and protocols. | |
Edge Computing | Processes data near the source to reduce latency and bandwidth usage, enabling immediate decisions. | Vital for latency-sensitive operations like robotic control and emergency shutdowns. | |
Private 5G Networks | Provide reliable, high-speed connectivity for seamless integration between edge and cloud. | Enhances agility and flexibility of edge-cloud manufacturing ecosystems. | |
Conceptual Frameworks | Digital Twins | High-fidelity, near-real-time digital replicas of physical assets or processes for simulation and optimization. | BMW uses digital twins to simulate factory workflows and optimize production. |
Self-Organizing Systems | Multiple autonomous agents coordinate without centralized control for scalable, resilient manufacturing. | Swarm-like robot collaboration analogous to ant colonies. | |
Interoperability Standards | Standards like Unified Namespace enable seamless communication across disparate systems. | Break down data silos to improve AI effectiveness. | |
Human-AI Collaboration Frameworks | Frameworks emphasizing trust, transparency, and adaptive learning between humans and AI agents. | SmythOS promotes transparent communication and collaboration. | |
Data Quality Importance | Ensuring high-quality data input to avoid poor AI outcomes (“garbage in, garbage out”). | Fundamental principle for AI success in manufacturing. |
AI-Driven Automation: From Repetitive Tasks to Lights-Out Factories
AI-Driven Automation: From Repetitive Tasks to Lights-Out Factories
How far can AI push automation in manufacturing before human hands become optional? The path from assisted robotics to fully autonomous “lights-out” factories is advancing rapidly, fueled by breakthroughs in perception, planning, and generative AI. This shift not only transforms physical tasks but also integrates cognitive decision-making, creating new opportunities and challenges that demand a balanced perspective beyond the hype.
From Assisted Robotics to Autonomous Operations
Traditionally, robotics in manufacturing performed repetitive, physically demanding tasks like welding or palletizing, following rigid, preprogrammed motions. Today’s AI-powered robots are far more adaptive and intelligent. Advances in sensor fusion, machine reasoning, and simulation techniques—including sim-to-real transfer and human-to-robot training—enable robots to perceive their environment with rich 3D detail and autonomously plan complex task sequences.
For instance, adaptive robots can interpret natural language commands and adjust their operations dynamically, blurring the line between mechanical execution and cognitive processing. At Automate 2025, companies such as Universal Robots and Mobile Industrial Robots demonstrated collaborative robots (cobots) that actively learn from their surroundings and human partners. These cobots provide unprecedented flexibility on production lines, eliminating the need for extensive manual reprogramming and enabling rapid adaptation to changing manufacturing demands.
The ultimate vision is the realization of “lights-out” factories—facilities that operate with minimal or no human intervention. These factories run continuously, controlled by integrated AI systems that manage robotic fleets and optimize processes in real time through advanced data analytics and machine learning. Siemens exemplifies this with its Tecnomatix Plant Simulation and Opcenter Execution systems, which digitally simulate, control, and refine entire production ecosystems before executing physical actions on the factory floor.
Generative AI: A Game-Changer for Setup and Flexibility
Beyond robotics, generative AI is revolutionizing how manufacturers reduce setup times and achieve flexible production. According to Deloitte’s 2025 Manufacturing Industry Outlook, 87% of manufacturers have initiated generative AI pilots, with half prioritizing full implementation within two years.
Generative AI excels in pattern recognition and content creation, enabling it to propose optimized machine configurations and work instructions that might otherwise be overlooked. It also powers immersive learning environments, translating complex operational knowledge into interactive simulations that accelerate workforce training and democratize expertise across the shop floor.
Additionally, generative AI dynamically adapts manufacturing plans in response to real-time events such as supply chain disruptions or design changes. This capability is critical as manufacturers increasingly face demand for customization without compromising efficiency or quality.
Technical Challenges: Integration, Reliability, and Workforce Transition
Despite these advances, integrating AI-driven automation into manufacturing environments remains complex. Systems integration is often the critical bottleneck, as legacy equipment and disparate vendor solutions must be orchestrated into a cohesive and reliable whole. Organizations like the ARM Institute and companies such as Advantech advocate for modular, edge-computing architectures to bridge this gap, but widespread adoption is still evolving.
Reliability and safety are paramount concerns for fully autonomous operations. Fail-safe mechanisms must be in place to handle unexpected anomalies without human oversight. High-frequency data capture platforms—such as MachineMetrics, which collects 1,000 data points per second—enable predictive and prescriptive maintenance, but interpreting and acting on this vast data in real time requires robust AI models continuously validated against operational realities.
Workforce transition presents one of the most sensitive challenges. Automation reshapes labor rather than simply replacing it. Research from McKinsey and Innopharma Education indicates that while AI will automate routine tasks, it simultaneously creates millions of new roles focused on AI supervision, data analysis, and human-machine collaboration. Addressing this shift demands strategic investment in reskilling and ethical stewardship to ensure AI amplifies human potential on the factory floor.
Final Thoughts
The evolution from assisted robotics to fully autonomous lights-out factories is more than a technological revolution—it is a profound transformation of industrial practices and workforce dynamics. AI-driven automation delivers unprecedented productivity and flexibility, but successful adoption requires careful navigation of integration complexities, system reliability, and human factors.
Manufacturers that excel will treat AI not as a silver bullet but as a continuously evolving partner—one that necessitates ongoing calibration, transparency, and respect for indispensable human expertise. As we advance the frontier of automation, the critical question evolves from “Can we automate?” to “How do we automate responsibly and sustainably?” The answers will shape manufacturing’s future for decades to come.
Aspect | Description | Examples / Key Points |
---|---|---|
From Assisted Robotics to Autonomous Operations | Transition from repetitive, preprogrammed robotic tasks to AI-driven adaptive and intelligent robots capable of perception and autonomous planning. | Universal Robots and Mobile Industrial Robots cobots; Siemens Tecnomatix Plant Simulation and Opcenter Execution systems; lights-out factories. |
Generative AI in Manufacturing | Use of generative AI to reduce setup times, optimize machine configurations, create work instructions, and adapt plans dynamically. | 87% manufacturers piloting generative AI (Deloitte 2025); immersive learning environments; real-time adaptation to supply chain and design changes. |
Technical Challenges | Integration of legacy and new systems, ensuring reliability and safety, managing workforce transitions. | Modular edge-computing architectures (ARM Institute, Advantech); fail-safe mechanisms; MachineMetrics data capture; reskilling and ethical stewardship (McKinsey, Innopharma Education). |
Final Thoughts | AI-driven automation requires balanced integration of technology and human expertise for sustainable manufacturing transformation. | Continuous calibration, transparency, respect for human roles; shift from “Can we automate?” to “How do we automate responsibly?” |
Enhancing Quality Control through AI: Precision, Anomaly Detection, and Real-Time Feedback
Enhancing Quality Control through AI: Precision, Anomaly Detection, and Real-Time Feedback
Imagine a manufacturing line capable of detecting microscopic defects invisible to the naked eye, instantly alerting operators, and autonomously adjusting processes to prevent costly waste and rework. Thanks to AI-driven quality control systems, this is no longer a futuristic concept but a present-day reality. As manufacturing complexity intensifies and profit margins tighten, AI technologies—especially computer vision and predictive analytics—are becoming indispensable for achieving precision and operational efficiency.
AI-Driven Computer Vision: From Detection to Prevention
Traditional quality control often operates reactively, identifying defects after they occur. AI transforms this approach by enabling real-time defect detection coupled with immediate process intervention.
Modern machine vision systems powered by deep learning function as electronic inspectors scanning thousands of products per minute. These systems detect surface flaws, misalignments, and irregularities at resolutions beyond human capability. For instance, Nanotronics has led the field for over a decade, and in 2025, its AI-enhanced optical inspection equipment flags microscopic anomalies as items move along the line, significantly reducing defects and waste .
The impact is tangible: automated visual inspection systems have demonstrated up to a 30% reduction in defect rates and dramatically shortened inspection times—often achieving full return on investment within two years . This efficiency boost is a compelling advantage for production managers.
Beyond speed, AI-powered pattern recognition algorithms classify and segment complex defect types, enabling nuanced quality decisions that transcend simple pass/fail judgments. Companies like Averroes.ai and Orbotech illustrate how AI integration enhances yield optimization and defect classification even in challenging manufacturing environments .
Further advancements come from 3D vision systems. Photoneo’s automated inspection cells incorporate precise metrology into real-time feedback loops, allowing manufacturers to measure dents, deformations, and dimensional accuracy with unprecedented fidelity .
Predictive Analytics and Acoustic Monitoring: Detecting Trouble Before It Strikes
Predictive maintenance (PdM) is vital for minimizing unplanned downtime and extending equipment lifespan. Imagine machinery that warns operators before failure occurs.
AI integrated with sensor data—including vibrations and acoustic signatures—enables early anomaly detection. Acoustic monitoring uses deep learning models, such as convolutional neural networks (CNNs) and long short-term memory (LSTM) networks, to analyze sound patterns emitted by machinery. These models detect subtle shifts signaling impending mechanical failures like bearing wear or rotor imbalances.
Applications span industries from additive manufacturing to aerospace, where predictive analytics have reduced downtime and enhanced safety .
The PdM market is projected to grow from $10.6 billion in 2024 to nearly $48 billion by 2029, underscoring its strategic importance in manufacturing sustainability (Source 0, Predictive Analytics).
Platforms like IBM Maximo exemplify integrated solutions combining AI, IoT, and edge computing to deliver real-time equipment health monitoring. Maintenance teams using IBM Maximo have reported up to a 70% reduction in breakdowns and a 25% decrease in maintenance costs .
Closed-Loop Feedback Systems: From Insight to Instant Action
Identification of defects and anomalies is only part of the solution. The true breakthrough is when AI systems autonomously initiate corrective actions.
Closed-loop quality control systems merge AI-driven analytics with manufacturing execution systems, enabling real-time adjustments of production parameters. For example, a vision inspection system deployed in a North American toilet paper plant detected a persistent production issue and immediately triggered process modifications, delivering an immediate return on investment (Source 0, Closed Loop).
These systems leverage continuous data streams and adaptive learning to detect process drift early, reducing scrap and rework. Anna Olsson of Intelecy highlights that “real-time optimization and closed-loop AI” critically depend on reliable data access and trust—challenges that are increasingly surmountable thanks to improved industrial data infrastructures (Source 1, Closed Loop).
In sectors with razor-thin quality tolerances—such as semiconductor fabrication and medical device manufacturing—AI-driven closed-loop systems are becoming essential for yield optimization and competitive differentiation (Source 2, Closed Loop).
Performance Metrics and Benchmarks: Gatekeepers of Industrial Adoption
Industrial adoption of AI quality control hinges on rigorous performance validation. Key metrics include:
- Detection Accuracy: The true positive rate of defect identification balanced against false positives.
- Processing Speed: Capability for real-time or near-real-time analysis to match production line velocity.
- Defect Rate Reduction: Quantifiable improvements in product yield and waste reduction.
- Return on Investment (ROI): Cost savings relative to implementation and operational expenses, often realized within two years.
- Integration Ease: Compatibility with existing production lines, control systems, and workflow processes.
Leading AI vision systems report accuracy surpassing human inspectors, supported by extensive validation on large and diverse datasets (Source 0, AI Vision).
For predictive maintenance, evaluation focuses on early fault detection rates and minimizing false positives, typically assessed using confusion matrices and corroborated by real-world case studies demonstrating significant downtime reductions (Source 6, Acoustic Monitoring).
Final Thoughts
AI’s role in manufacturing quality control is transitioning from promising concept to practical, high-impact application. From microscopic defect detection via AI-powered computer vision to acoustic diagnostics and closed-loop systems reacting instantly, AI reshapes quality assurance with unprecedented precision and agility.
Yet challenges persist. Data quality, integration complexity, and trust in AI-driven decisions remain critical hurdles. Addressing these demands robust data infrastructures, transparent algorithms, and human oversight.
Manufacturers investing thoughtfully in AI-driven quality control position themselves to reduce costs, minimize waste, and enhance resilience—key advantages as they navigate the demands of tomorrow’s market. The question is no longer if AI will transform quality assurance, but how quickly organizations can leverage its power to gain a competitive edge.
Aspect | Description | Examples / Metrics | Sources |
---|---|---|---|
AI-Driven Computer Vision | Real-time defect detection and immediate process intervention using deep learning-powered machine vision systems. | 30% reduction in defect rates; faster inspection times; classification of complex defects; 3D vision for dimensional accuracy. | Nanotronics (2025), Averroes.ai, Orbotech, Photoneo ([0], [5], [8], [9]) |
Predictive Analytics & Acoustic Monitoring | Use of AI on sensor data (vibration, acoustic) to detect early anomalies and predict equipment failure. | 70% reduction in breakdowns; 25% decrease in maintenance costs; PdM market growth from $10.6B to $48B (2024-2029). | ResearchGate (Additive Manufacturing), IBM Maximo, Market projections ([7], [11], [0]) |
Closed-Loop Feedback Systems | AI-driven analytics combined with manufacturing execution systems enabling autonomous real-time process adjustments to reduce defects and scrap. | Immediate ROI from process modifications; critical in semiconductor and medical device sectors. | QualityMag, Intelecy, Averroes.ai ([0], [1], [2]) |
Performance Metrics | Key benchmarks for AI quality control adoption. | – Detection Accuracy – Processing Speed – Defect Rate Reduction – Return on Investment (ROI) – Integration Ease | Nanotronics, MathWorks, Case Studies ([0], [6]) |
Predictive Maintenance: Reducing Downtime with AI-Based Prognostics
Predictive Maintenance: Reducing Downtime with AI-Based Prognostics
Imagine having the ability to anticipate exactly when a machine component will fail—before it disrupts production. This capability is at the core of AI-driven predictive maintenance, an increasingly indispensable technology in modern manufacturing. By continuously analyzing streams of sensor data, AI models forecast equipment failures and estimate the remaining useful life (RUL) of components, transforming maintenance from a reactive necessity into a strategic advantage.
How AI Anticipates Equipment Health: Core Techniques in Predictive Maintenance
Predictive maintenance hinges on interpreting complex time-series data collected from sensors embedded within machinery. These sensors capture vibrations, temperature, pressure, and other critical parameters, producing temporal patterns that often elude traditional statistical analysis.
Key AI techniques powering predictive maintenance include:
-
Time-Series Analysis: Advanced algorithms, such as transformer-based neural networks, excel at modeling long-range dependencies in sensor data. They detect subtle, gradual shifts signaling wear or impending faults well before failure.
-
Anomaly Detection: Machine learning models continuously monitor operational data to identify deviations from established “normal” behavior. Unlike static threshold alarms, AI adapts to evolving conditions and uncovers nuanced anomalies that might otherwise go unnoticed.
-
Prognostic Health Management (PHM): PHM models integrate live sensor readings with historical maintenance records to accurately estimate component RUL. This enables manufacturers to schedule maintenance activities precisely, avoiding unnecessary downtime or premature part replacements.
For example, dynamic time warping algorithms—more flexible than simple Euclidean distance—compare current machine signals against known healthy and faulty patterns. These comparisons generate a health index that trends over time, allowing early detection of deterioration before catastrophic breakdowns occur.
Real-World Impact: Successful AI Predictive Maintenance Deployments
The practical benefits of AI-based predictive maintenance are demonstrated through several industry applications:
-
Tooling and CNC Machines: MachineMetrics offers an AI-powered tool monitoring platform that analyzes centuries’ worth of anonymized machine data without requiring invasive sensors. This system delivers real-time insights into tool life, helping reduce scrap rates and costly downtime on the shop floor.
-
Belts and Gears: In power transmission and bearing sectors, AI models analyze vibration and temperature data to predict failures. This predictive capability optimizes energy efficiency and extends component lifespans, critical for industries like agriculture and mining where belt drives are prevalent.
-
Large-Scale Industrial Plants: Owens Corning integrated IoT sensor data with SAP’s Intelligent Asset Management solutions, achieving $2 million in annual savings by preventing unnecessary repairs and optimizing maintenance schedules.
These examples underscore the broad applicability of AI-driven predictive maintenance—from precision tooling in factories to large industrial facilities—delivering measurable reductions in downtime and improved asset longevity.
Overcoming Barriers: Challenges in Implementing AI Predictive Maintenance
Despite proven successes, deploying AI for predictive maintenance entails several challenges:
-
Data Scarcity and Quality: Reliable AI models require abundant, high-quality labeled data. Many manufacturers struggle with limited failure records or inconsistent sensor coverage, complicating model training and accuracy.
-
Model Generalizability: AI models trained on specific equipment or environments may underperform when deployed in different plants due to variations in machine types, operational conditions, and sensor configurations. Techniques like uncertainty quantification and transfer learning are essential to enhance robustness across diverse contexts.
-
Cost-Benefit Tradeoffs: For small and medium-sized manufacturers, the initial investment in AI infrastructure, sensor installation, and workforce upskilling can be significant. However, industry reports indicate that companies embracing AI predictive maintenance often realize a return on investment within 1.5 to 2.5 years by reducing emergency repairs and labor costs.
-
Workforce Integration: For predictive insights to be actionable, AI solutions must be designed with operators and maintenance teams in mind. Building early buy-in, demonstrating clear ROI, and ensuring model transparency foster trust and successful adoption.
The Road Ahead: Strategic Value and Cautions in AI Predictive Maintenance
AI-powered predictive maintenance is more than a tool for preventing equipment failures—it is a foundation for sustainability, operational agility, and smarter decision-making. Industry data shows that such approaches can reduce maintenance costs by up to 25%, cut breakdowns by 70%, and slash downtime by half.
Nevertheless, it is important to temper expectations with realism. AI models are powerful aids, not infallible oracles. Their effectiveness depends on continuous access to high-quality data, ongoing model validation, and seamless integration into broader operational workflows. Additionally, advancements in sensor technology, edge computing, and IoT connectivity require scalable and adaptable AI architectures to fully harness their potential.
Manufacturers who strategically invest in AI-driven predictive maintenance today position themselves not only for immediate cost savings but also for enhanced resilience against supply chain disruptions, labor shortages, and tightening sustainability regulations. The future of manufacturing belongs to those who predict challenges proactively rather than reactively.
Category | Details |
---|---|
Core AI Techniques |
|
Examples of AI Algorithms | Dynamic time warping for comparing current signals against healthy and faulty patterns, generating a health index over time. |
Real-World Applications |
|
Implementation Challenges |
|
Strategic Value |
|
Comparative Analysis: AI Adoption Across Manufacturing Sectors and Scale
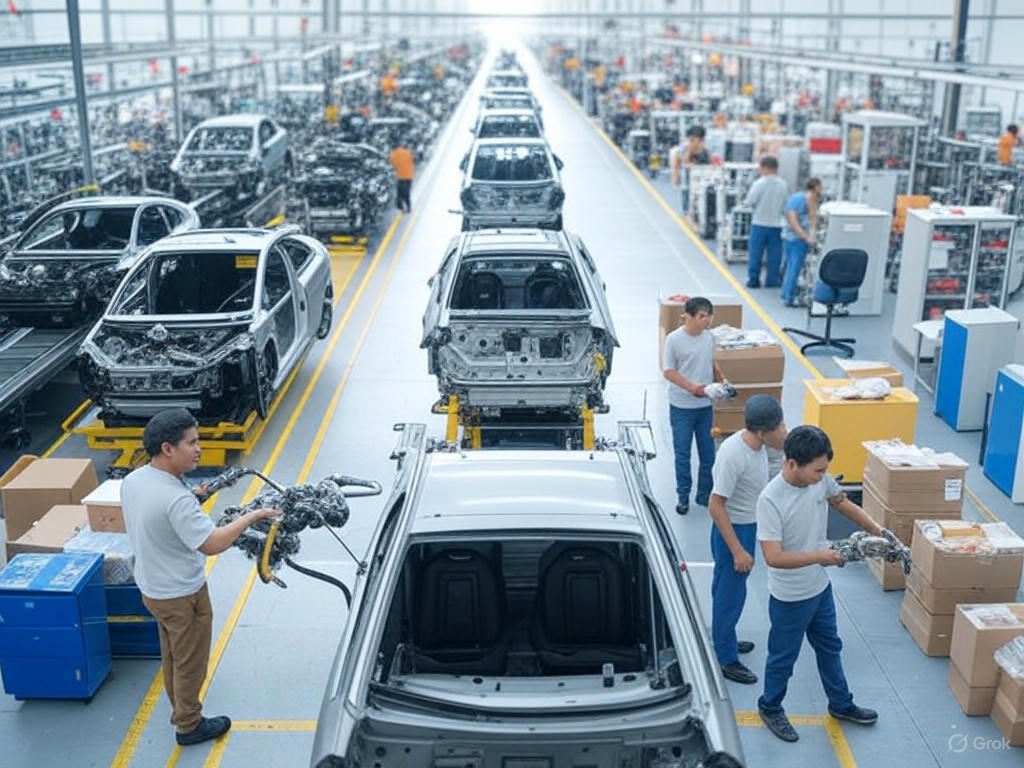
Comparative Analysis: AI Adoption Across Manufacturing Sectors and Scale
How differently is AI reshaping manufacturing when comparing automotive giants to small consumer goods producers? The penetration and maturity of AI technologies vary widely across sectors and company sizes. This uneven adoption reflects unique operational demands, investment capacities, and workforce readiness.
AI Maturity Across Key Manufacturing Sectors
The automotive industry exemplifies rapid AI advancement. Valued at $4.8 billion in 2024, the AI market in automotive is projected to surge to $186.4 billion by 2034 with a compound annual growth rate of 42.8%. This sector leads in deploying sophisticated AI applications such as image recognition for autonomous driving. These systems interpret complex, real-time environmental data to detect pedestrians, traffic signals, and lane markings with high precision. Tesla’s Gigafactories stand out as near-autonomous manufacturing hubs, where AI-powered robots oversee most production stages, representing a leap far beyond traditional automation.
The semiconductor and electronics sectors prioritize synchronized hardware-software development. Semiconductor sales reached $627 billion in 2024 with a 19% growth rate. AI adoption here focuses on optimizing upstream engineering workflows and implementing flexible reskilling programs to address anticipated talent shortages. In electronics manufacturing, AI-driven computer vision and generative AI enhance quality control and accelerate innovation cycles, aligning with the sector’s demand for precision and speed.
The consumer goods industry is rapidly catching up, particularly in supply chain optimization and personalized production. Recent reports indicate that 41% of consumer goods manufacturers utilize AI to manage supply chain data, improving material availability and reducing waste. This sector emphasizes human-centric AI solutions that balance automation with workforce empowerment, reflecting its diverse operational contexts.
Heavy industry and industrial equipment manufacturers adopt AI more cautiously but with targeted investments. Predictive maintenance to avert costly downtime is a key focus, alongside AI integration in quality control and digital twins that simulate and optimize operations. Challenges include upgrading legacy equipment and implementing specialized cybersecurity measures to protect increasingly connected systems.
SMEs vs. Multinationals: Diverging Paths to AI Gains
The divide in AI adoption between small and medium-sized enterprises (SMEs) and multinational corporations (MNCs) is significant but nuanced. SMEs account for over half of the U.S. workforce yet operate at roughly 47% of the productivity level of larger firms. Nevertheless, AI offers SMEs a powerful lever for productivity gains; those adopting AI report average operational efficiency improvements of 32.7%. Targeted AI applications such as inventory management and workflow automation enable SMEs to compete more effectively despite limited resources.
Multinationals leverage their scale to invest heavily in cutting-edge AI technologies and maintain centralized teams dedicated to smart manufacturing initiatives. Deloitte’s 2025 survey reveals that 52% of large manufacturers have established specialized AI groups, compared to fewer SMEs that often face resource constraints.
Benchmarking key performance indicators shows that MNCs typically achieve more substantial quality improvements and cost savings by integrating AI across multiple plants and supply chains. Conversely, SMEs often realize faster returns by focusing on specific AI use cases like predictive maintenance or customer demand forecasting. These targeted applications impact the bottom line without requiring extensive infrastructure overhauls.
Barriers to Adoption: Skill Shortages, Cybersecurity, and Investment Risks
Despite AI’s transformative potential, several barriers hinder widespread adoption, especially among smaller manufacturers. Contrary to common assumptions, employee resistance is generally low, with workers ready and willing to embrace AI. The primary bottleneck lies in leadership hesitation and a lack of strategic direction. Only about 1% of companies consider themselves mature in AI adoption, underscoring the gap between pilot projects and scaled implementations.
Skill shortages present a critical challenge. The demand for AI talent in manufacturing grows annually by 74%, yet many firms struggle to recruit or develop necessary expertise. While upskilling and reskilling programs are vital, SMEs often lack sufficient resources to implement them effectively.
Cybersecurity concerns add complexity. Industrial control systems and operational technology environments are prime targets for sophisticated cyberattacks such as ransomware and supply chain intrusions. The convergence of IT and OT expands the attack surface, necessitating robust, AI-enhanced defenses. Best practices include adopting zero trust architectures, identity-based segmentation, and continuous monitoring powered by AI to detect threats in real time.
Investment risk perception also limits adoption. Manufacturers face uncertainty regarding return on investment timelines and integration complexities. Many adopt a cautious approach, prioritizing use cases with high ROI and establishing strong digital foundations before scaling AI efforts. Collaborations with external partners for expertise and change management have proven effective, particularly for organizations lacking internal AI maturity.
Best Practices and Lessons Learned
From leaders in automotive manufacturing to agile SMEs, several best practices have emerged:
-
Prioritize targeted, high-impact AI use cases. Focus on projects delivering measurable efficiency or quality improvements to build momentum and justify further investment.
-
Establish cross-functional teams. Combine technical experts with operations and business leaders to align AI initiatives with strategic objectives.
-
Invest continuously in workforce development. Ongoing training and clear communication about job roles help alleviate workforce anxiety and foster human-AI collaboration.
-
Strengthen cybersecurity early. Integrate AI-driven threat detection and response mechanisms alongside traditional defenses to mitigate risks from increased connectivity.
-
Leverage external expertise. Partnering with consultants or technology providers can accelerate AI adoption and aid in managing organizational change.
In summary, AI adoption in manufacturing is uneven, shaped by sector-specific requirements, company scale, and organizational readiness. However, the trajectory is clear: manufacturers that strategically embrace AI, proactively address barriers, and invest in people and security frameworks will unlock significant productivity, quality, and cost advantages in the coming years.
Aspect | Automotive Industry | Semiconductor & Electronics | Consumer Goods | Heavy Industry & Equipment | SMEs | Multinationals (MNCs) |
---|---|---|---|---|---|---|
AI Market Value (2024) | $4.8 billion | Part of $627 billion semiconductor sales | Not specified | Not specified | Not specified | Not specified |
Projected AI Market Growth | $186.4 billion by 2034 (42.8% CAGR) | 19% growth rate in semiconductor sales | Increasing adoption, 41% use AI in supply chain | More cautious adoption, targeted investment | Operational efficiency improvement: 32.7% | 52% have specialized AI groups |
Key AI Applications | Image recognition for autonomous driving, near-autonomous factories | Optimizing engineering workflows, flexible reskilling, computer vision, generative AI | Supply chain optimization, personalized production | Predictive maintenance, quality control, digital twins | Inventory management, workflow automation, predictive maintenance, demand forecasting | Integrated AI across plants and supply chains, centralized smart manufacturing teams |
Operational Focus | High precision, real-time data interpretation | Precision and speed in production cycles | Human-centric AI balancing automation and workforce empowerment | Legacy equipment upgrades, cybersecurity | Targeted use cases, faster ROI | Large scale integration, cost savings, quality improvement |
Challenges | Complex systems integration | Talent shortages, workflow optimization | Diverse operational contexts | Legacy upgrades, cybersecurity | Resource constraints, skill shortages | Investment scale, complexity |
Cybersecurity | Not specifically highlighted | Not specifically highlighted | Not specifically highlighted | Specialized cybersecurity measures essential | Limited resources for security | Strong cybersecurity frameworks |
Workforce Readiness | Advanced automation, AI-robot collaboration | Flexible reskilling programs | Workforce empowerment emphasis | Workforce challenges due to legacy systems | Upskilling difficult but critical | Continuous workforce development |
AI Adoption Maturity | Leading sector, near-autonomous factories | Growing adoption with engineering focus | Rapidly catching up | Cautious but targeted | Lower maturity, targeted gains | Higher maturity, specialized teams |
Future Outlook: Emerging Trends, Ethical Considerations, and Societal Impacts
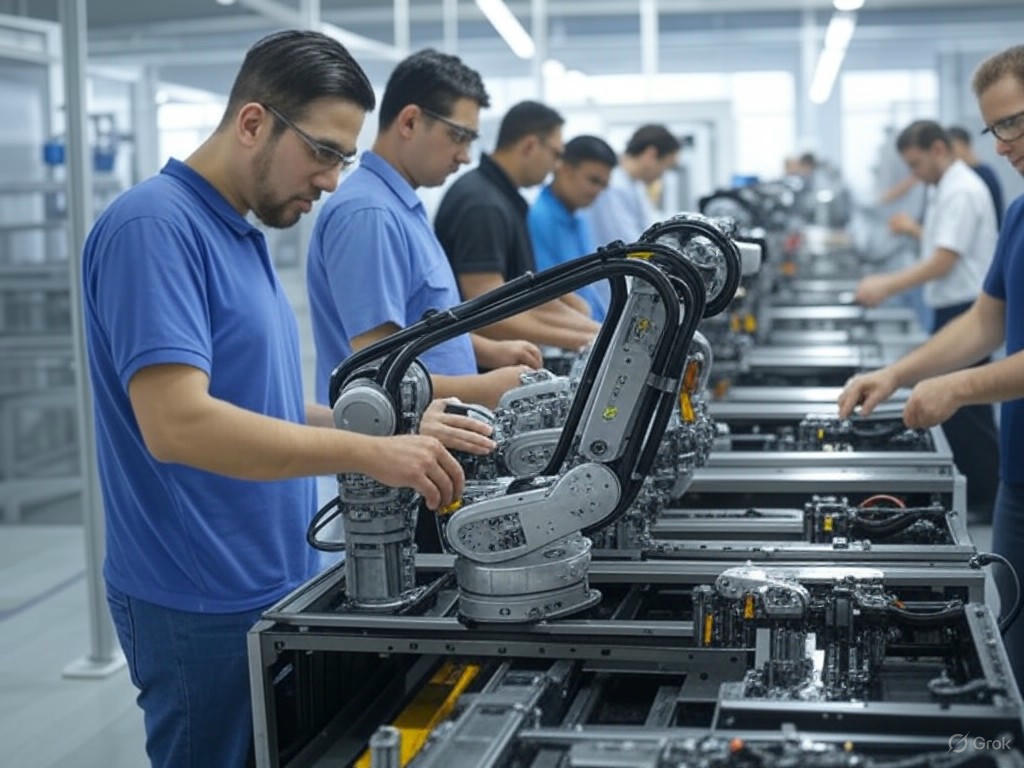
Future Outlook: Emerging Trends, Ethical Considerations, and Societal Impacts
What will manufacturing look like when AI evolves from a mere tool to a seamless collaborator? As we approach 2025 and beyond, emerging AI innovations are set to reshape factory floors, quality assurance, and maintenance practices fundamentally. Yet, these advances bring ethical complexities and societal implications that demand thoughtful attention.
Emerging AI Innovations: From Augmented Reality to Digital Twins and Conversational Interfaces
Augmented reality (AR) is transitioning from novelty to a core productivity enhancer in manufacturing. By overlaying digital information onto physical components, AR empowers workers to perform assembly, maintenance, and training tasks with higher precision and fewer errors. For instance, Jabil Circuit reported a 10% increase in production yield through AR-powered interactive work instructions. Similarly, Samsung combines 3D vision scanning with AR overlays to meet the stringent quality demands of LCD panel production, exemplifying how digital augmentation enhances both efficiency and quality control.
The scale of AR adoption is impressive: ABI Research forecasts 27 million AR smart glasses shipments for manufacturing by 2025, while mobile AR users worldwide are projected to exceed 1.19 billion by 2028. The rollout of 5G networks will further reduce latency, enabling real-time, hands-free AR experiences essential for complex manufacturing environments.
Complementing AR, digital twins are advancing from static models to dynamic, AI-driven simulations that closely mirror real-world factory conditions. Valued at $6.9 billion in 2022, the digital twin market is expected to surge to $73.5 billion by 2027. Siemens, partnering with NVIDIA Omniverse, leads in photorealistic digital twin visualizations integrated into product lifecycle management systems, enabling unprecedented factory planning and process optimization.
Conversational AI is also poised for transformative impact on human-machine interaction on factory floors. Next-generation conversational agents will handle complex queries, offer instant troubleshooting, and facilitate seamless workflows by understanding context, sentiment, and multimodal inputs such as text, voice, and gestures. Market forecasts anticipate conversational AI platforms will grow into a $49.9 billion industry by 2030. Low-code and no-code platforms will democratize access, empowering manufacturers of all sizes to harness these interfaces.
Together, these technologies signal a shift toward more intuitive, immersive, and data-rich manufacturing ecosystems. However, alongside this innovation surge, ethical and societal dimensions must be carefully considered.
Ethical Considerations: Workforce Displacement, Data Privacy, and AI Transparency
The prospect of workforce displacement is a critical ethical concern in manufacturing’s AI future. Studies estimate up to 800 million jobs globally could be affected by AI and automation by 2030. While AI-driven efficiency gains are undeniable, responsible deployment requires proactive measures such as investing in reskilling, continuous education, and inclusive economic policies. Manufacturers carry the responsibility to balance technological progress with social impact, ensuring displaced workers transition to new roles rather than face obsolescence.
Data privacy represents another pressing challenge. AI systems in manufacturing process sensitive operational and employee data, making them attractive targets for cyberattacks. Security leaders project that by 2025, 93% will face daily AI-driven attacks. Additionally, opaque data practices, especially involving biometric or behavioral information, undermine trust. Adhering to privacy-by-design principles and compliance with regulations like GDPR and CCPA is essential for sustainable AI adoption.
Transparency and explainability are equally vital. Black-box AI models risk eroding accountability and user trust, especially when AI decisions affect operational safety or workforce management. Emerging tools such as IBM Watsonx’s transparency suite and Explainable AI (XAI) methodologies are gaining prominence. By 2025, explainability will be a defining feature of successful AI-powered manufacturing systems, enabling stakeholders to understand, audit, and trust AI recommendations.
Societal Impacts: Sustainability Gains and Strategic Pathways Forward
AI’s potential to advance sustainability in manufacturing is among its most promising societal contributions. When including logistics, manufacturing accounts for nearly 60% of human-induced carbon dioxide emissions. AI-powered analytics can identify inefficiencies and optimize energy use, waste reduction, and supply chain emissions in real time.
For example, AI-driven closed-loop manufacturing systems supported by 5G and cloud technologies enable virtual factories to run diagnostics remotely, reducing physical resource consumption and travel-related emissions. The World Economic Forum emphasizes AI’s role in bridging environmental, social, and governance (ESG) disclosures with actionable strategies, positioning sustainability as a core business objective rather than a mere compliance requirement.
Nonetheless, sustainability gains are not automatic; they require intentional governance and cross-sector collaboration. Ethical frameworks must integrate environmental justice principles to avoid exacerbating inequalities during the transition to greener manufacturing models.
Strategic Recommendations for Manufacturers Navigating AI’s Complex Landscape
Given these multifaceted dimensions, manufacturers should adopt a strategic and responsible approach to AI implementation:
-
Prioritize Ethical AI Deployment: Invest in workforce reskilling initiatives and maintain transparent communication with employees about AI-driven transformations. Establish AI governance policies addressing bias, fairness, and accountability.
-
Embed Privacy and Security by Design: Implement robust cybersecurity tailored to AI systems. Ensure strict compliance with data protection laws such as GDPR and CCPA, and maintain transparent data handling to foster trust.
-
Leverage Explainability Tools: Adopt Explainable AI frameworks and transparency tools to illuminate AI decision-making processes, supporting regulatory compliance and stakeholder confidence.
-
Integrate Sustainability Metrics: Utilize AI to systematically monitor and reduce carbon footprints. Collaborate with supply chain partners to extend sustainability benefits throughout the value chain.
-
Embrace Emerging Interfaces: Explore AR, digital twins, and conversational AI as complementary tools that augment human expertise rather than replace it.
-
Foster Cross-Industry Collaboration: Engage with startups, technology providers, regulators, and academia to co-shape AI’s evolution in manufacturing responsibly.
In conclusion, AI’s trajectory in manufacturing transcends automation and cost-cutting. It embodies a complex interplay of innovation, ethics, and societal responsibility. As manufacturers stand at this transformative threshold, the imperative is clear: harness AI’s full potential with vigilance, empathy, and strategic foresight. Only through such stewardship can AI become a true partner in building a sustainable, equitable industrial future.
Category | Details | Examples / Data |
---|---|---|
Emerging AI Innovations | Augmented Reality (AR), Digital Twins, Conversational AI for enhanced productivity, quality control, and interaction | – Jabil Circuit: 10% production yield increase via AR – Samsung: 3D vision + AR overlays for LCD panel quality – AR smart glasses: 27 million shipments by 2025 (ABI Research) – Digital twin market: $6.9B (2022) to $73.5B (2027) – Conversational AI market: $49.9B by 2030 |
Ethical Considerations | Workforce displacement, data privacy, AI transparency and explainability | – Up to 800 million jobs affected by 2030 – 93% of organizations face daily AI-driven cyberattacks by 2025 – Compliance with GDPR, CCPA – IBM Watsonx transparency suite and Explainable AI (XAI) tools emerging |
Societal Impacts | Sustainability gains, environmental and social governance (ESG), ethical governance | – Manufacturing accounts for ~60% of human-induced CO₂ emissions – AI enables energy optimization, waste reduction, supply chain emission management – AI-driven closed-loop manufacturing reduces physical resource use – Environmental justice integration needed for equitable transition |
Strategic Recommendations | Ethical AI deployment, privacy/security by design, explainability, sustainability metrics, emerging interfaces, collaboration | – Invest in reskilling and inclusive policies – Robust cybersecurity and transparent data handling – Adopt Explainable AI tools – Monitor and reduce carbon footprints – Use AR, digital twins, conversational AI to augment expertise – Collaborate with startups, regulators, academia |