AI in Insurance: Automating Claims & Underwriting with Insight
- Introduction: Why AI is Reshaping Insurance Claims and Underwriting
- Introduction: Why AI is Reshaping Insurance Claims and Underwriting
- Traditional Challenges: Complexity, Delays, and Errors
- The Transformative Power of AI in Claims and Underwriting
- Balancing Promise with Ethical and Operational Realities
- Setting the Stage for a Nuanced Exploration
- Foundations of AI in Insurance: Core Technologies and Methodologies
- Foundations of AI in Insurance: Core Technologies and Methodologies
- Machine Learning: The Analytical Backbone
- Natural Language Processing, OCR, and Intelligent Document Processing: Taming Unstructured Data
- Robotic Process Automation and Cognitive Automation: Replicating Human Cognition at Scale
- Integration of Core Technologies: How These Systems Work Together
- Why These Foundations Matter Beyond Efficiency
- Automating Claims Processing: Technical Specifications and Performance Metrics
- Automating Claims Processing: Technical Specifications and Performance Metrics
- AI-Driven Claims Automation Workflows: From Intake to Payment Authorization
- System Architectures: Integrating AI with Legacy Insurance Platforms
- Performance Metrics: Quantifying AI’s Impact on Claims Automation
- Transforming Underwriting with AI: Enhancing Risk Assessment and Decision-Making
- Transforming Underwriting with AI: Enhancing Risk Assessment and Decision-Making
- AI-Driven Risk Modeling and Predictive Analytics: A New Paradigm
- Technical Foundations: From Feature Engineering to Explainability
- Navigating Challenges: Transparency, Bias, and Compliance
- The Road Ahead: Balancing Innovation and Prudence
- Comparative Analysis: Traditional vs AI-Driven Claims and Underwriting Processes
- Comparative Analysis: Traditional vs AI-Driven Claims and Underwriting Processes
- Efficiency Gains: Compressing Weeks into Days
- Accuracy and Fraud Prevention: Elevating Precision and Proactivity
- Customer Experience: Combining Personalization with Speed
- Operational Challenges: Integration, Training, and Managing Risks
- Separating Hype from Reality: Where AI Delivers and Where Humans Remain Essential
- Key Takeaways
- Practical Implications and Societal Impact: Ethics, Employment, and Customer Experience
- Practical Implications and Societal Impact: Ethics, Employment, and Customer Experience
- Ethical Considerations: Privacy, Fairness, and Transparency
- Workforce Dynamics: Job Displacement and Human-AI Collaboration
- Customer Experience: Faster Settlements, Chatbots, and the Trust Equation
- Key Takeaways
- Future Trends and Challenges: The Road Ahead for AI in Insurance Automation
- Future Trends and Challenges: The Road Ahead for AI in Insurance Automation
- Emerging AI Technologies Shaping Insurance Automation
- Technical Challenges: Navigating Complexity and Risk
- Scalability and Innovation: Unlocking New Business Models
- A Balanced Outlook: Innovation with Ethical Vigilance

Introduction: Why AI is Reshaping Insurance Claims and Underwriting
Introduction: Why AI is Reshaping Insurance Claims and Underwriting
Why has insurance—an industry long regarded as stodgy and risk-averse—become a focal point for artificial intelligence innovation? The answer lies in the intersection of increasing operational pressures and leaps in technological capabilities, compelling insurers to fundamentally rethink claims and underwriting processes.
Traditional Challenges: Complexity, Delays, and Errors
Historically, insurance claims and underwriting have been encumbered by complexity and inefficiency. Underwriters, for example, spend an average of three hours daily on manual data entry, while peer reviews can delay decisions by up to 10 days. These bottlenecks are more than inconvenient; they directly erode profitability and customer satisfaction.
Claims processing faces similar hurdles. Approximately 15–25% of medical claims are initially denied or rejected, with 65% of those never resubmitted, resulting in billions of dollars in lost revenue annually.
Beyond operational inefficiencies, insurers grapple with a rapidly evolving risk landscape—from geopolitical instability to climate volatility—that traditional models inadequately capture. Legacy IT infrastructures, challenging cloud migrations, and constrained budgets further impede modernization. Coupled with an industry culture cautious toward innovation, these factors have slowed progress even as customer demands for speed, accuracy, and transparency intensify.
The Transformative Power of AI in Claims and Underwriting
AI-driven automation is no longer a distant prospect but a present-day force revolutionizing core insurance functions. McKinsey forecasts that by 2030, up to 50% of claims activities will be automated, potentially reducing resolution costs by as much as 75%.
Technologies such as natural language processing (NLP), machine learning (ML), and computer vision empower insurers to analyze vast quantities of unstructured data—from health records to satellite images—with accuracy and speed unattainable by humans alone.
The impact extends beyond efficiency. AI enhances risk assessment precision, enabling underwriters to progress from retrospective evaluations to real-time, data-driven insights. Advanced predictive analytics and data lakehouses facilitate the integration of emerging risks—such as cyber threats and climate impacts—into underwriting decisions with unprecedented granularity.
Balancing Promise with Ethical and Operational Realities
While AI offers transformative potential, its adoption is accompanied by significant operational and ethical considerations. Integrating AI into entrenched legacy systems remains a substantial technical challenge, and upskilling the workforce is critical; surveys reveal that 63% of senior insurance managers identify digital skills shortages as a serious concern.
Ethical issues around bias, transparency, and fairness demand attention. AI-powered claims and underwriting decisions risk unintended discrimination or erroneous denials if not carefully governed, jeopardizing customer trust and inviting regulatory scrutiny. Consequently, insurers are investing not only in AI technology but also in robust governance frameworks that emphasize compliance, responsible data use, and explainability.
Setting the Stage for a Nuanced Exploration
This article will explore how InsurTech leverages AI to revolutionize insurance claims and underwriting—accelerating processing times, enhancing accuracy, and enabling personalized customer experiences. It will also examine the complex trade-offs between innovation, ethics, and operational readiness.
Our evidence-based approach aims to cut through hype, providing nuanced insights into both the transformative opportunities and the challenges insurers face in embracing AI throughout 2025 and beyond.
Aspect | Details |
---|---|
Manual Data Entry by Underwriters | Average 3 hours daily |
Peer Review Delays | Up to 10 days |
Medical Claims Initially Denied | 15–25% |
Denied Claims Never Resubmitted | 65% |
Projected Automation of Claims by 2030 | Up to 50% |
Potential Reduction in Resolution Costs | Up to 75% |
Senior Managers Reporting Digital Skills Shortages | 63% |
Foundations of AI in Insurance: Core Technologies and Methodologies
Foundations of AI in Insurance: Core Technologies and Methodologies
What does it take to automate the labyrinthine processes of insurance claims and underwriting? At the heart of this transformation lies a suite of AI technologies that are not only reshaping workflows but also redefining how insurers assess risk, detect fraud, and enhance customer experience. Let’s unpack the foundational AI technologies powering this seismic shift.
Machine Learning: The Analytical Backbone
Machine learning (ML) is often described as the engine beneath AI’s hood, and in insurance, it drives real-time data analysis at unprecedented scales. Insurers manage mountains of data—from demographics and health records to claims history and telematics. The challenge has always been making sense of this avalanche quickly and accurately.
-
Supervised learning algorithms train on labeled datasets, enabling precise tasks like fraud detection or claims classification. For example, supervised ML models analyze past claims to flag suspicious patterns, helping to reduce the $80 billion annual loss from fraud that industry analyses report.
-
Unsupervised learning uncovers hidden structures in unlabeled data, such as segmenting customers for personalized marketing or detecting anomalies in claims that might indicate fraud or errors.
-
Deep learning, a subset of ML, employs neural networks that mimic human brain functions to interpret complex, unstructured data like images or audio. This is especially valuable for processing damage photos in claims or assessing underwriting risk from satellite imagery.
McKinsey projects that by 2025, roughly 25% of insurance processes will be automated using these techniques. This enables faster claims settlement, optimized pricing, and improved risk assessment. For instance, machine learning models predicting Customer Lifetime Value (CLV) allow insurers to tailor offers and proactively manage customer churn.
Natural Language Processing, OCR, and Intelligent Document Processing: Taming Unstructured Data
Insurance workflows are notoriously paperwork-heavy—policy documents, claims forms, emails, and more. Much of this data is unstructured, challenging traditional systems to parse and analyze efficiently.
-
Natural Language Processing (NLP) converts human language into machine-readable data. It powers chatbots that understand customer queries, virtual assistants that recommend personalized policies, and automated systems that detect compliance risks by analyzing regulatory texts. NLP also extracts insights from customer feedback and identifies fraud through subtle linguistic cues.
-
Optical Character Recognition (OCR) transforms scanned documents, images, and PDFs into editable text. Progressive Insurance, for example, leveraged OCR to speed up claims processing, reducing manual data entry errors and accelerating resolution times.
-
Intelligent Document Processing (IDP) combines OCR, NLP, and machine learning to handle complex documents with minimal human intervention. Unlike traditional rule-based systems, IDP learns from data patterns, adapts to new document formats, and manages both structured and unstructured inputs. Platforms like EY Fabric Document Intelligence integrate these capabilities, enabling insurers to automatically extract and interpret data from 70% of incoming documents, thereby freeing agents to focus on nuanced customer interactions.
Think of IDP as a highly skilled assistant who not only reads your paperwork but also understands context, flags inconsistencies, and learns from corrections—a human-in-the-loop model balancing efficiency with accuracy.
Robotic Process Automation and Cognitive Automation: Replicating Human Cognition at Scale
Robotic Process Automation (RPA) is transforming insurance operations by automating repetitive, rule-based tasks once performed by humans. Imagine software bots tirelessly handling data entry, cross-referencing databases, and triggering workflows without breaks or errors.
-
By 2025, RPA is expected to automate up to 60% of back-office insurance processes, delivering time savings as high as 66%. This includes underwriting data collection, claims verification, and regulatory compliance checks.
-
When combined with AI capabilities like ML and NLP, RPA evolves into cognitive automation—bots capable of interpreting semi-structured data, making decisions, and escalating complex cases for human review.
EY Fabric Document Intelligence exemplifies this integration, combining RPA, NLP, and ML to process insurance claims end to end. This platform automates document extraction, fraud detection, and data validation within legacy systems, enabling insurers to reduce manual workloads while improving accuracy and regulatory compliance.
Integration of Core Technologies: How These Systems Work Together
The transformative power of AI in insurance emerges when these technologies converge:
-
Machine learning models analyze incoming claim data for risk scoring and fraud detection.
-
NLP and OCR convert unstructured documents and customer communications into actionable data.
-
Intelligent Document Processing orchestrates the data flow, learning from exceptions and continuously improving.
-
RPA bots automate repetitive steps, escalating nuanced or high-risk cases to human experts.
This layered, hybrid approach enables insurers to achieve straight-through processing for simpler claims, while dedicating human expertise to complex decisions. The outcome is faster claims resolution, reduced operational costs, and enhanced customer experience.
Why These Foundations Matter Beyond Efficiency
While the technical sophistication of these AI-driven foundations is impressive, they also raise important ethical and operational considerations. As AI assumes more decision-making roles, insurers must ensure models are explainable, free from bias, and compliant with evolving regulations to maintain trust.
Automating claims and underwriting is not solely about speed or cost reduction—it’s about enabling smarter, fairer, and more personalized insurance experiences. The foundations laid today will determine the industry’s ability to adapt to emerging challenges such as climate risk, cyber threats, and shifting customer expectations.
In summary, the core AI technologies—machine learning, natural language processing, optical character recognition, intelligent document processing, and robotic process automation—are collaboratively revolutionizing insurance. They unlock insights from vast, complex datasets and replicate human cognitive tasks at scale, paving the way for a more agile, accurate, and customer-centric insurance industry.
AI Technology | Description | Key Use Cases in Insurance | Examples / Impact |
---|---|---|---|
Machine Learning (ML) | Analyzes large datasets to identify patterns and make predictions using algorithms like supervised, unsupervised, and deep learning. | Fraud detection, claims classification, customer segmentation, pricing optimization, risk assessment. | Reduces $80B annual fraud losses; predicts Customer Lifetime Value; automates ~25% of processes by 2025. |
Natural Language Processing (NLP) | Converts human language into machine-readable data to interpret and generate text or speech. | Chatbots, virtual assistants, compliance risk detection, customer feedback analysis, fraud detection via linguistic cues. | Enables chatbots for customer queries; detects compliance risks; extracts insights from feedback. |
Optical Character Recognition (OCR) | Transforms scanned documents and images into editable, searchable text. | Digitizing policy documents, claims forms, speeding up data entry and processing. | Used by Progressive Insurance to reduce manual data entry errors and accelerate claims resolution. |
Intelligent Document Processing (IDP) | Combines OCR, NLP, and ML to automatically extract and interpret data from complex documents with minimal human input. | Automates document handling, adapts to new formats, manages structured and unstructured data. | EY Fabric Document Intelligence extracts data from 70% of incoming documents, freeing agents for complex tasks. |
Robotic Process Automation (RPA) | Automates repetitive, rule-based tasks through software bots. | Data entry, underwriting data collection, claims verification, regulatory compliance checks. | Expected to automate up to 60% of back-office processes by 2025; saves up to 66% time. |
Cognitive Automation | Enhances RPA with AI to interpret semi-structured data, make decisions, and escalate complex cases. | End-to-end claims processing, fraud detection, data validation, workflow automation. | Integration in platforms like EY Fabric Document Intelligence improves accuracy and compliance. |
Automating Claims Processing: Technical Specifications and Performance Metrics
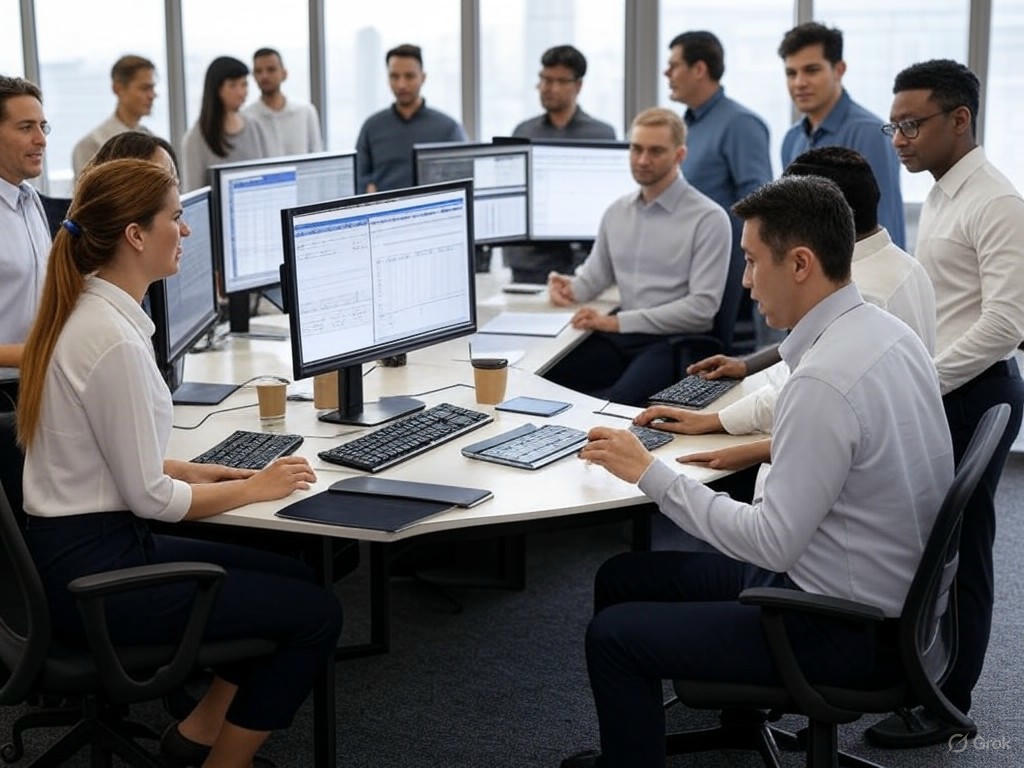
Automating Claims Processing: Technical Specifications and Performance Metrics
Building an AI-driven claims processing system that meaningfully enhances efficiency, accuracy, and fraud prevention requires more than buzzwords. It demands carefully architected workflows, robust system integration, and rigorous measurement of performance outcomes. InsurTech innovators are reshaping claims operations end-to-end—from intake through payment authorization—while bridging legacy platforms and delivering concrete business value.
AI-Driven Claims Automation Workflows: From Intake to Payment Authorization
Claims processing is the operational core where insurer commitments meet customer realities. Modern AI systems orchestrate a series of interdependent stages, each leveraging specialized technologies:
-
Intake: Automated digital forms combined with natural language processing (NLP) capture claim details across multiple channels, supplanting manual paperwork and email exchanges. Platforms like FlowForma enforce validation rules during intake and issue real-time notifications, reducing manual oversight and rework.
-
Validation: AI-powered document processing integrates optical character recognition (OCR) with large language models (LLMs) to interpret unstructured claims documents. For example, V7 Go couples generative AI with OCR and LLM orchestration to achieve approximately 70% accuracy in document interpretation, addressing a major bottleneck in claims automation.
-
Adjudication: Auto-adjudication engines apply configurable business rules supplemented by machine learning models to assess coverage eligibility and calculate payouts. In healthcare claims—where up to 80% of premiums may be spent on processing—tools like QuickCap 7 streamline adjudication, enabling near real-time decisions with minimal human intervention.
-
Fraud Detection: Insurance fraud costs the U.S. industry over $300 billion annually, with about 10% of property and casualty claims suspected fraudulent. Machine learning models analyze multimodal data—including text, images, and audio—to detect anomalies and suspicious patterns. Sprout.ai’s AI platform exemplifies this approach, reducing claims operations costs by 48% while enhancing fraud detection capabilities.
-
Payment Authorization: Post-adjudication, claims trigger payment workflows increasingly leveraging robotic process automation (RPA) to accelerate settlements. These automated systems ensure compliance, maintain auditability, and minimize transactional errors.
Despite these advances, handling exceptions and complex judgment calls remains a challenge for AI. Human adjusters continue to manage nuanced cases, with AI shifting their focus from routine data tasks to higher-value decision-making.
System Architectures: Integrating AI with Legacy Insurance Platforms
Legacy insurance systems, often decades old, were not designed for seamless AI integration, posing technical and organizational hurdles. Successful AI-driven claims processing depends on thoughtful architectural design:
-
Data Pipelines: AI models require consistent, high-quality data streams. Modern architectures employ middleware solutions and data lakes to unify disparate data sources—policy records, claims history, telematics, IoT sensor inputs, and external databases. This unified data infrastructure is critical for enabling real-time processing and advanced predictive analytics.
-
Modular AI Components: Insurers favor modular, task-specific AI models over monolithic solutions. Gartner projects that by 2027, the adoption of these smaller, targeted AI models will triple that of general-purpose large language models, reflecting a preference for precision and reliability in claims workflows.
-
Real-Time Processing and Edge Computing: Integration of IoT devices—such as connected vehicles and smart home sensors—feeds real-time data into AI models for dynamic claim evaluations and fraud detection. Zurich Insurance, for example, employs AI-driven models across more than 160 use cases, leveraging edge computing to identify inefficiencies and fraudulent activity in real time.
-
Human-in-the-Loop Systems: Despite the push for automation, human oversight remains essential. AI systems incorporate feedback loops allowing models to learn continuously and adapt, thereby improving accuracy and reducing false positive rates over time.
A practical illustration is the Nordic insurer’s deployment, which integrates AI modules within legacy claims management platforms. This approach accelerates claim triaging and enables real-time fraud analytics without necessitating a costly overhaul of existing infrastructure.
Performance Metrics: Quantifying AI’s Impact on Claims Automation
Measuring the tangible benefits of AI in claims processing requires clear, relevant metrics:
-
Accuracy Rates: Advanced AI-driven document interpretation platforms like V7 Go now attain around 70% accuracy by combining OCR with generative AI. Fraud detection models that analyze multimodal data deliver high precision, significantly lowering false positives.
-
Claim Resolution Time: Automation technologies have cut claim turnaround times by over 30%. Robotic process automation processes claims up to 75% faster than manual workflows. Sprout.ai’s platform, for instance, reduces claims operations costs by nearly half and enables settlements within hours, a stark contrast to traditional multi-week cycles.
-
Error Rate Improvements: Automated validation and adjudication reduce human errors in data entry and rule application. This improvement enhances regulatory compliance and minimizes costly disputes or claim rejections.
-
Cost Savings: McKinsey estimates that robotic process automation in claims management can yield a 200% return on investment annually. Sprout.ai reports average savings of £21 per claim, reflecting operational efficiencies and fraud reduction combined.
-
Customer Experience: Faster, more transparent claims handling enhances customer satisfaction. Surveys indicate that 59% of UK policyholders are willing to engage AI-powered systems for quicker claims processing, reflecting growing consumer trust in AI-driven services.
These metrics are not theoretical but grounded in real-world deployments. Sprout.ai’s AI platform demonstrates how combining fraud detection with claims automation drives measurable cost savings and operational agility. Similarly, the Nordic insurer’s incremental AI integration within legacy systems has achieved faster claim triage and improved fraud identification, balancing efficiency gains with risk mitigation.
Integrating AI into claims processing is now a strategic imperative rather than a distant vision. Yet, success requires deliberate architectural choices, carefully designed workflows, and rigorous performance tracking. By harmonizing automation with human expertise and leveraging data intelligently, insurers can transform claims from a cost center into a competitive advantage—delivering speed, accuracy, and trust in equal measure.
Stage | Technology / Platform | Key Features / Metrics |
---|---|---|
Intake | Automated digital forms, NLP, FlowForma | Multi-channel claim detail capture, validation rules, real-time notifications, reduced manual oversight |
Validation | OCR, Large Language Models (LLMs), V7 Go | Interprets unstructured documents, ~70% accuracy, addresses automation bottlenecks |
Adjudication | Auto-adjudication engines, machine learning, QuickCap 7 | Configurable business rules, near real-time decisions, minimal human intervention |
Fraud Detection | Machine learning on multimodal data, Sprout.ai | Detects anomalies in text, images, audio; reduces claims costs by 48% |
Payment Authorization | Robotic Process Automation (RPA) | Accelerates settlements, ensures compliance and auditability, minimizes errors |
System Architecture Aspect | Implementation / Example | Benefits |
---|---|---|
Data Pipelines | Middleware, data lakes unifying policy, claims, telematics, IoT, external data | Consistent high-quality data, supports real-time processing and predictive analytics |
Modular AI Components | Task-specific AI models (vs. monolithic LLMs) | Greater precision and reliability; Gartner projects triple adoption by 2027 |
Real-Time Processing & Edge Computing | IoT sensors, connected vehicles, Zurich Insurance (160+ use cases) | Dynamic claim evaluations, real-time fraud detection, efficiency improvements |
Human-in-the-Loop Systems | Feedback loops for continuous learning and adaptation | Improves accuracy, reduces false positives, maintains essential human oversight |
Legacy System Integration | Nordic insurer AI modules integrated into legacy platforms | Accelerates triage, enables real-time fraud analytics without costly overhaul |
Metric | Details / Examples | Impact |
---|---|---|
Accuracy Rates | V7 Go ~70% document interpretation accuracy; high precision fraud detection | Reduces errors and false positives |
Claim Resolution Time | Automation cuts turnaround by over 30%; RPA is 75% faster; Sprout.ai enables settlements within hours | Speeds claims processing significantly vs. traditional multi-week cycles |
Error Rate Improvements | Automated validation and adjudication reduce human errors | Enhances regulatory compliance and reduces disputes/claim rejections |
Cost Savings | McKinsey: 200% ROI from RPA; Sprout.ai saves £21 per claim on average | Operational efficiency and fraud reduction yield significant savings |
Customer Experience | 59% UK policyholders willing to engage AI-powered claims processing | Improves satisfaction through faster, transparent handling |
Transforming Underwriting with AI: Enhancing Risk Assessment and Decision-Making
Transforming Underwriting with AI: Enhancing Risk Assessment and Decision-Making
What if underwriting could evolve from a largely manual, time-consuming activity to a streamlined, data-driven process delivering greater precision, speed, and fairness? This scenario is rapidly becoming reality. The insurance industry is experiencing a profound transformation driven by AI technologies that are redefining risk assessment and policy issuance.
AI-Driven Risk Modeling and Predictive Analytics: A New Paradigm
Traditional underwriting often relied on incomplete or static data, limiting risk assessment to a fixed snapshot in time. Today, AI empowers insurers to ingest and analyze a far more diverse and dynamic data ecosystem. Internet of Things (IoT) devices and telematics exemplify this shift. Embedded in vehicles, homes, and wearables, these devices continuously stream data on driving behavior, environmental conditions, and health metrics, turning risk assessment into a real-time, personalized process.
For example, telematics data capturing acceleration, braking, and speed patterns allows insurers to identify risky driving behaviors and reward safer drivers with customized premiums. Similarly, smart sensors in homes monitor fire hazards or water leaks, enabling proactive risk mitigation before claims arise. This integration of IoT and AI is revolutionizing underwriting accuracy and pricing algorithms, shifting insurers from reactive payers to proactive risk managers.
Historical claims data remains foundational but is now enriched by machine learning models that detect subtle correlations and emerging trends. These models analyze vast datasets—often spanning millions of records—to forecast loss probabilities with unprecedented granularity. The outcome is more personalized policies that accurately reflect individual risk profiles rather than relying on broad segment averages.
Yet, incorporating such heterogeneous data sources poses challenges. Legacy IT systems frequently struggle with data incompatibility, and robust security protocols are essential given the sensitive nature of the information. Insurers that successfully navigate these obstacles gain a competitive advantage through faster, more accurate underwriting that reduces operational costs and enhances customer satisfaction.
Technical Foundations: From Feature Engineering to Explainability
The effectiveness of AI in underwriting rests on rigorous technical methodologies. Feature engineering—the process of selecting, transforming, and crafting predictive variables—is critical. Domain expertise guides the identification of relevant features such as driving patterns, health indicators, or property characteristics, which feed into predictive models.
Underwriting models often combine traditional actuarial techniques with advanced machine learning algorithms, including deep learning and ensemble methods. For instance, Poisson regression remains valuable for modeling count data, especially when combined with regularization or Bayesian methods to avoid overfitting.
However, accuracy alone is insufficient. Trust is paramount in insurance, and AI models must be explainable, traceable, and auditable. Explainable AI (XAI) frameworks provide transparency by revealing which features influenced specific underwriting decisions. For example, a system might indicate that a driver’s frequent hard braking significantly increased their premium calculation.
Model validation is an ongoing, iterative process involving thorough testing against diverse datasets to ensure consistency, fairness, and robustness. Continuous Integration/Continuous Deployment (CI/CD) workflows adapted from software development facilitate regular updates and improvements while maintaining strict role-based access controls to safeguard data integrity.
Human expertise remains indispensable. AI systems act as augmented underwriters rather than replacements—human reviewers handle exceptions and provide feedback that continuously refines models, enhancing reliability and domain understanding.
Navigating Challenges: Transparency, Bias, and Compliance
While AI offers powerful capabilities, it also amplifies longstanding challenges related to fairness and regulatory compliance. Training data often encodes societal biases—such as those related to gender or socioeconomic status—that, if unchecked, can lead to unfair underwriting decisions.
Mitigating bias requires a comprehensive, multi-layered approach:
- Data interrogation to identify and cleanse biased inputs early in the pipeline.
- Fairness constraints incorporated into model training to minimize disparate impacts proactively.
- Statistical audits assessing bias across key variables aligned with the insurer’s fairness philosophy.
- Explainability tools that illuminate model reasoning, enabling auditors and regulators to scrutinize decisions effectively.
Regulators are increasingly active in this space. In the U.S., over twenty states have issued AI governance bulletins emphasizing transparency, risk management, and the necessity of auditable AI processes. Insurers must comply with these evolving regulations while safeguarding consumer trust by demonstrating ethical AI deployment.
Data privacy and security are equally critical. The surge in IoT data collection heightens cyber risks, requiring insurers to implement strong encryption, access controls, and selective data-sharing agreements to prevent unauthorized use.
Consumer perception also plays a vital role. Although many customers recognize AI’s potential to expedite underwriting and claims, skepticism persists regarding reliability and fairness. Clear communication about AI’s role and safeguards is essential to bridge this trust gap.
The Road Ahead: Balancing Innovation and Prudence
AI-powered underwriting is not a panacea but a transformative tool that, when applied judiciously, enhances risk assessment, operational efficiency, and customer experience. Early adopters who combine advanced analytics with robust governance frameworks and ethical safeguards are positioned to lead the industry.
This transformation requires not only technological investment but also cultural change—upskilling underwriters, fostering cross-disciplinary collaboration, and embracing continuous model monitoring. In an industry where trust is the foundation, AI integration must prioritize accountability and fairness alongside speed and precision.
The future of underwriting will be a hybrid model: AI’s computational power complementing human judgment, together crafting smarter, fairer insurance products capable of addressing the complex risks of the digital age.
Aspect | Description | Examples/Notes |
---|---|---|
AI-Driven Risk Modeling | Utilizes diverse, dynamic data sources for real-time, personalized risk assessment. | IoT devices, telematics data on driving behavior, smart home sensors. |
Predictive Analytics | Machine learning models analyze vast data to forecast loss probabilities with high granularity. | Models detect subtle correlations beyond traditional actuarial averages. |
Technical Foundations | Feature engineering, machine learning algorithms (e.g. deep learning, Poisson regression), and explainable AI frameworks. | Explainability reveals feature impact; models are continuously validated and updated. |
Challenges | Addressing data incompatibility, security, bias, transparency, and regulatory compliance. | Strong encryption, fairness constraints, statistical audits, and regulatory adherence. |
Human-AI Collaboration | AI augments human underwriters; humans handle exceptions and provide feedback. | Ensures reliability, domain expertise, and continuous model improvement. |
Regulatory Environment | Governance emphasizing transparency, risk management, and auditable AI processes. | U.S. states’ AI governance bulletins and evolving compliance requirements. |
Consumer Perception | Mixed views on AI reliability and fairness; communication is key to building trust. | Clear explanation of AI role and safeguards reduces skepticism. |
Future Outlook | Hybrid underwriting models combining AI and human judgment for smarter, fairer insurance. | Requires upskilling, cultural change, and ongoing governance frameworks. |
Comparative Analysis: Traditional vs AI-Driven Claims and Underwriting Processes
Comparative Analysis: Traditional vs AI-Driven Claims and Underwriting Processes
When centuries-old insurance practices meet today’s AI-driven automation, the transformation is both profound and nuanced. The contrast between legacy manual workflows and AI-augmented processes extends beyond mere speed improvements—it encompasses accuracy, customer experience, and fraud prevention. However, this evolution is not about wholesale replacement but rather about amplifying human expertise through intelligent automation.
Efficiency Gains: Compressing Weeks into Days
Traditional claims and underwriting workflows heavily depend on manual data entry, paperwork, and human judgment. Underwriters typically spend around three hours daily on manual entry, and peer reviews can delay final decisions by up to ten days. Such bottlenecks often stretch claim processing times to weeks or even months, contributing to customer dissatisfaction and revenue loss.
According to Deloitte projections cited by Ricoh USA, by 2025, 60% of claims will be triaged with automation, signaling a seismic shift in operational tempo. AI’s capability to process vast volumes of structured and unstructured data—ranging from electronic health records to satellite imagery—enables insurers to drastically reduce turnaround times.
Case studies highlight insurers cutting claim resolution from weeks to a few days by leveraging AI-powered data extraction and risk assessment tools. For example, SmartDev (2025) reports significant acceleration in claims processing through AI integration. ENTER’s layered AI platform showcases how intelligent document processing (IDP), combined with robotic process automation (RPA), expedites reimbursements while enhancing compliance.
However, these efficiency gains are not guaranteed out of the box. Many insurers face integration complexity, as legacy IT systems often silo data, slowing AI adoption (Aufait Technologies). Modular, cloud-based architectures provide a scalable path forward, enabling insurers to embed AI capabilities incrementally without costly infrastructure overhauls.
Accuracy and Fraud Prevention: Elevating Precision and Proactivity
Manual underwriting and claims evaluation are prone to human error and inconsistencies, which can lead to costly mistakes or overlooked risks. AI-driven workflows enhance precision by applying machine learning (ML) models to detect anomalous patterns indicative of fraud or misstatements.
Fraud detection is one of AI’s clearest value propositions. Sprout.ai’s platform, for instance, combines fraud analytics with claims automation to reduce operational costs by 48%, saving approximately £21 per claim while enhancing detection accuracy. ENTER’s Enterprise Denial AI identifies suspicious claims early and supports appeal strategies to recover underpaid claims, bolstering financial resilience.
Modern AI systems merge rule-based engines with ML algorithms to analyze claims in real time, flagging potential fraud before payouts occur (Vonage, 2025). This proactive approach not only curtails financial losses but also allows human investigators to focus on complex or ambiguous cases, enhancing overall fraud management effectiveness.
Yet AI accuracy depends heavily on data quality and transparency. IBM cautions that poor data or biased algorithms can degrade actuarial models, potentially leading to unfair underwriting decisions or missed emerging risks. To mitigate this, explainable AI (XAI) frameworks and continuous model audits are essential to uphold trust and comply with regulators such as NAIC and The American College of Financial Services.
Customer Experience: Combining Personalization with Speed
Customer expectations in insurance are evolving rapidly, driven by experiences in consumer technology demanding fast, personalized, and transparent service. AI enables insurers to aggregate and unify diverse data streams—from telematics devices to social media footprints—to tailor policies and claims processes to individual customer profiles (SmartDev; Vonage).
AI chatbots and virtual agents now handle up to 95% of routine customer interactions in some firms, providing 24/7 support and real-time claim status updates (Wisedocs.ai). This “Netflix-level personalization” model frees human agents to focus on complex inquiries requiring empathy and nuanced judgment.
Nevertheless, human oversight remains indispensable. AI lacks the social intuition to interpret subtle emotional cues or handle sensitive conversations, areas where experienced agents excel. As Stan Bowers from Spear Technologies emphasizes, adopting a “human in the loop” approach blends AI’s data-processing power with human domain expertise, ensuring customers feel valued and understood rather than managed by machines.
Operational Challenges: Integration, Training, and Managing Risks
Despite AI’s promise, insurers face significant hurdles in deploying AI at scale. Legacy IT environments often resist seamless integration, necessitating substantial modernization and customization efforts (FBSPL). Embedding AI tools into existing workflows can disrupt operations if not carefully orchestrated.
Staff retraining is a critical component of successful AI adoption. Insurance professionals—including underwriters and claims adjusters—need to develop AI literacy to collaborate effectively with automated systems. Leading managing general agents (MGAs) invest heavily in cross-departmental AI education to build capabilities and foster organizational trust (FBSPL).
Error handling in AI production environments demands vigilance. While AI reduces routine errors, it introduces new risks such as algorithmic bias, operational drift, or system failures, which require robust governance and human intervention. Regulatory bodies increasingly expect insurers to implement explainability, fairness, and audit mechanisms around AI decision-making (The American College of Financial Services; NAIC).
Separating Hype from Reality: Where AI Delivers and Where Humans Remain Essential
The hype around AI sometimes overstates its current capabilities, risking unrealistic expectations. Not every underwriting decision or claim evaluation can be fully automated today. Nurix AI’s 2025 assessment underscores that while AI demonstrates clear value in fraud detection and claims automation, full transformation is still a work in progress, constrained by data challenges, regulatory complexity, and trust issues.
Charles Skamser highlights that the most effective AI applications function as contextually aware digital assistants, augmenting rather than replacing human judgment. Hybrid intelligence systems that combine AI’s computational strengths with human insight produce superior outcomes, especially in complex or high-stakes scenarios.
Moreover, AI’s strategic value extends beyond operational efficiency to competitive differentiation. Early adopters who successfully navigate ethical, privacy, and regulatory challenges position themselves as industry leaders, offering more personalized and agile insurance products (SmartDev; Forbes).
Key Takeaways
- AI significantly accelerates claims processing and underwriting, often compressing turnaround times from weeks to days.
- Fraud detection benefits immensely from AI’s real-time pattern recognition, though data quality and model transparency are critical.
- Customer experience improves through AI-powered personalization and automation, but human empathy and judgment remain vital.
- Operational challenges include legacy system integration, staff retraining, and managing AI-related risks.
- The reality of AI in insurance is nuanced: it excels as an augmentation tool and strategic enabler rather than a wholesale replacement for human expertise.
In summary, AI is reshaping insurance workflows with impressive results, yet it is not a magic wand. Successful insurers will be those who balance technological enthusiasm with thoughtful governance, integrating AI-driven automation into workflows that retain human oversight where it counts most.
Aspect | Traditional Processes | AI-Driven Processes |
---|---|---|
Efficiency | Manual data entry (~3 hours daily), peer reviews delaying decisions up to 10 days, claims processing taking weeks or months | Automation triages 60% of claims (projected by 2025), data extraction and risk assessment reduce claim resolution to days, modular cloud architectures facilitate integration |
Accuracy & Fraud Prevention | Prone to human error, inconsistent underwriting, slower fraud detection | Machine learning detects anomalies, fraud analytics reduce costs by 48%, real-time fraud flagging, explainable AI frameworks ensure transparency |
Customer Experience | Slower, less personalized service relying heavily on human agents | AI chatbots handle up to 95% routine interactions, personalized policies using diverse data streams, human agents focus on complex cases with emotional nuance |
Operational Challenges | Legacy IT systems cause data silos, manual workflows resist automation | Integration complexity with legacy systems, need for staff AI literacy and retraining, governance for bias and errors, continuous audits |
Human Role | Central in all processes, full manual judgment | AI as digital assistant augmenting human expertise, human oversight essential for sensitive and complex decisions |
Risk Factors | Human errors, slow fraud detection, inconsistent decisions | Algorithmic bias, operational drift, system failures, data quality issues |
Strategic Value | Established methods, slower adaptation | Competitive differentiation through agile, personalized products, ethical and regulatory compliance as differentiators |
Practical Implications and Societal Impact: Ethics, Employment, and Customer Experience
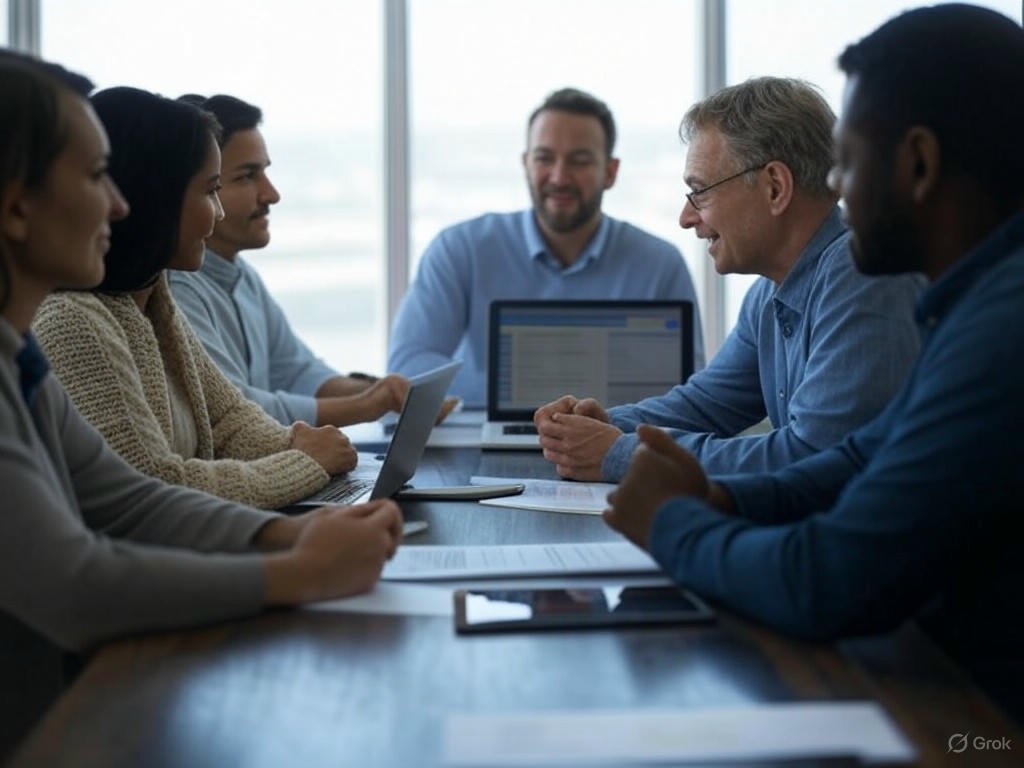
Practical Implications and Societal Impact: Ethics, Employment, and Customer Experience
AI is no longer just optimizing insurance processes; it is reshaping how insurers operate and engage with customers. While automation in underwriting and claims processing has boosted efficiency and accuracy, it also presents complex challenges around fairness, workforce transformation, and customer trust.
Ethical Considerations: Privacy, Fairness, and Transparency
Insurance companies manage vast and sensitive datasets, including health records, financial histories, telematics data, and social media footprints. AI leverages this data to enhance underwriting precision and fraud detection but simultaneously raises pressing ethical concerns.
-
Data privacy remains paramount. Despite 76% of U.S. insurers adopting generative AI in some form (Insurance Thought Leadership, 2025), concerns around data security and privacy continue to limit broader AI deployment. Insurers must implement robust governance frameworks that balance cutting-edge AI capabilities with rigorous privacy protections aligned with regulations like GDPR and CCPA.
-
Fairness is inherently complex. Unlike simple equal treatment, actuarial fairness requires premiums to reflect actual risk. Yet, opaque AI algorithms risk embedding unintended biases, provoking regulatory and consumer pushback. The National Association of Mutual Insurance Companies (NAMIC) cautions that enforcing fairness detached from risk-based pricing could distort markets and harm consumers (Browne Jacobson, 2025).
-
Transparency fosters trust. Customers increasingly demand clarity on how AI-driven decisions occur. However, transparency often lags behind AI adoption. The Federal Trade Commission (FTC) has intensified enforcement against misleading AI claims, signaling that insurers must clearly communicate AI’s role and limitations (Lathrop GPM, 2025). Chris Daniel of Sapiens underscores this, stating, “Trust is the cornerstone” — emphasizing the need to explain AI decision logic and embed empathy even within automated interactions (Insurance Edge, 2025).
Successfully navigating these ethical dimensions requires a balanced approach: harnessing AI for operational advantage while safeguarding consumer rights and societal norms. Without vigilant oversight and open dialogue, AI risks becoming a double-edged sword in insurance.
Workforce Dynamics: Job Displacement and Human-AI Collaboration
The impact of AI on the insurance workforce is profound but nuanced, involving both displacement and role evolution.
-
Routine tasks will be automated. McKinsey projects that up to 50% of claims activities could be automated by 2030 (Wisedocs, 2025). AI excels at processing voluminous documents, detecting fraud patterns, and applying underwriting rules with efficiency and consistency unattainable by humans.
-
Human expertise will shift toward judgment and empathy. Despite AI’s analytical strengths, it cannot replicate human intuition, empathy, or nuanced social cues critical in complex claims and customer interactions (Vonage, 2025). The future workforce will emphasize human-AI collaboration, where AI systems handle data-intensive tasks and humans focus on decision-making, empathy, and relationship management.
-
Reskilling and governance are essential. Insurers need to invest in upskilling employees to work alongside AI tools and establish governance structures that ensure responsible AI use (Fenwick, 2025). Rather than replacement, AI acts as an augmentation—empowering employees with AI copilots that surface insights, flag anomalies, and tailor workflows to individual expertise (Alltius.ai, 2025).
As the industry experiences a generational workforce turnover, insurers that embrace hybrid human-AI models stand to enhance operational efficiency while maintaining the trusted human touch essential for customer confidence.
Customer Experience: Faster Settlements, Chatbots, and the Trust Equation
AI’s integration into insurance has transformed customer interactions, delivering speed and personalization but also raising concerns about depersonalization.
-
Speed and personalization redefine service. AI-powered claims processing can slash turnaround times from weeks to days or even hours in some cases (SmartDev, 2025). Fraud detection algorithms reduce costly errors, while generative AI enables tailored policy recommendations by analyzing vast, heterogeneous datasets (Vonage, 2025).
-
AI chatbots serve as the new front line. Virtual assistants like GEICO’s “Kate” and Zurich’s AI-driven chatbots provide 24/7 support, instantly addressing queries and streamlining processes such as First Notice of Loss (FNOL) (Nurix AI, 2025). The global market for insurance chatbots is projected to reach $1.25 billion by 2025, reflecting their rapid adoption (Chatbase, 2025).
-
Risks of depersonalization persist. While customers appreciate convenience, they also seek to feel genuinely heard and understood. Overreliance on AI can make interactions feel transactional or opaque, potentially eroding trust. Industry experts like Bridgenext emphasize the critical need to balance digital efficiency with authentic human connection to remain competitive in 2025 and beyond.
-
Regulatory frameworks are evolving. Guidelines such as the NAIC’s Model Bulletin and multiple state-level AI governance bulletins now emphasize fairness, transparency, and consumer protection in AI use (Fenwick, 2025). Insurers face the challenge of ensuring AI-enabled customer service not only meets technical standards but also aligns with societal expectations for accountability and empathy.
In sum, AI is reshaping the customer journey by accelerating processes and expanding access, but insurers must carefully design experiences that preserve empathy and transparency. The future will favor those who use AI to enable meaningful human engagement rather than replace it.
Key Takeaways
-
Ethical AI deployment in insurance requires stringent privacy safeguards, sophisticated fairness frameworks consistent with actuarial principles, and transparent communication to build and maintain trust.
-
The insurance workforce is undergoing transformation, not wholesale replacement, with AI automating routine tasks while humans emphasize empathy, creativity, and complex judgment through collaborative models.
-
Customer experience is enhanced by rapid claims processing and AI chatbots, but insurers must vigilantly balance efficiency with personalization to avoid alienating customers and undermining trust.
-
Regulatory oversight is intensifying globally, mandating responsible AI governance; insurers must proactively embed ethical frameworks and compliance into their AI strategies.
The insurance industry stands at a pivotal crossroads: technological advances in AI promise significant operational and customer experience gains, but realizing this potential demands thoughtful integration that harmonizes innovation with human values, ethical rigor, and customer-centric design. Only through this balanced approach can AI become a genuine force for good in insurance.
Aspect | Details | Implications |
---|---|---|
Ethical Considerations |
|
|
Workforce Dynamics |
|
|
Customer Experience |
|
|
Regulatory Oversight |
|
|
Future Trends and Challenges: The Road Ahead for AI in Insurance Automation
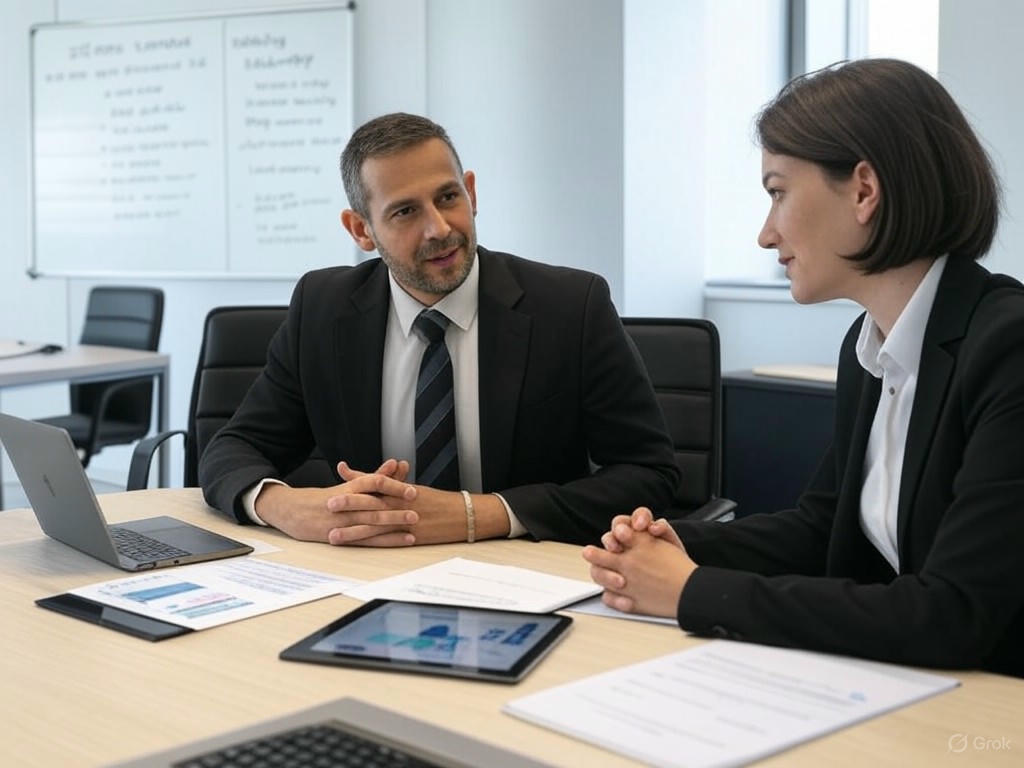
Future Trends and Challenges: The Road Ahead for AI in Insurance Automation
What lies ahead for AI-driven automation in insurance? With over 15 years of experience in AI system design, I see an industry on the cusp of profound transformation—yet facing intricate challenges. The coming era will be shaped not only by smarter AI algorithms but also by insurers’ ability to manage technical risks, ethical responsibilities, and evolving regulations, all while scaling innovation to meet practical business needs.
Emerging AI Technologies Shaping Insurance Automation
A defining shift is the growing adoption of large language models (LLMs). These sophisticated models go beyond chatbots: when fine-tuned for insurance workflows, LLMs can digitize end-to-end risk processes—from customer onboarding to claims settlement—at near-zero marginal cost. Experts like Richard Hartley emphasize how LLMs unlock value by processing heterogeneous data formats that previously bottlenecked automation.
Complementing LLMs are smaller language models (SLMs), specialized for tasks such as querying policy details or claim statuses. These models enhance accuracy and reliability across underwriting and claims management. Additionally, generative AI is playing a critical role in producing synthetic data for risk assessment and claims simulations, boosting underwriting precision and fraud detection effectiveness.
Beyond language models, federated learning (FL) is emerging as a vital privacy-preserving approach. FL enables insurers to collaboratively train AI models across organizations without sharing raw data—crucial in an industry where data privacy and security are paramount. By transmitting only model updates and keeping data local, insurers can build robust predictive models that comply with consumer privacy expectations and regulatory mandates.
Simultaneously, Internet of Things (IoT) integration is accelerating real-time underwriting capabilities. Telematics devices monitoring driving behavior and smart sensors assessing property conditions allow insurers to dynamically price risk and automate claims processing. The IoT insurance market exemplifies this growth, expanding from $31.5 billion in 2022 to a projected $686.9 billion by 2032, at a compound annual growth rate (CAGR) of 36.4%.
Technical Challenges: Navigating Complexity and Risk
Despite these advances, significant technical hurdles remain. Ensuring model robustness is a perennial concern; AI systems must maintain reliable performance across diverse and evolving data environments. Adversarial AI—where attackers manipulate inputs to deceive models—is no longer theoretical. Financial services, including insurance, face increasing risks from such attacks, necessitating proactive defense measures such as AI Red Teams that rigorously test system vulnerabilities.
Legacy IT systems present integration challenges. Data incompatibility, security concerns, and complex architectures complicate AI deployment, requiring modular, cloud-based solutions and strong governance frameworks. Furthermore, regulatory landscapes are rapidly evolving. For example, over twenty U.S. states have issued AI governance bulletins, and frameworks like the Wisconsin OCI bulletin mandate transparency, risk management, and bias mitigation throughout the AI lifecycle.
Algorithmic fairness is non-negotiable. Insurers must prevent AI decisions from unfairly disadvantaging protected groups or causing adverse consumer outcomes. This requires continuous bias analysis, explainability, and human-in-the-loop oversight to detect and correct errors or unintended consequences. Globally, regulatory efforts like the European Union’s AI Act emphasize data governance and transparency, demanding agility from insurers operating across jurisdictions.
Scalability and Innovation: Unlocking New Business Models
Looking forward, AI’s scalability will redefine insurance products and services. Automated underwriting already enables straight-through processing for up to 90% of applications, with leading systems delivering quotes in under four minutes. This rapid decision-making liberates resources to focus on complex cases and enhance customer engagement.
Parametric insurance illustrates AI-enabled innovation. These products pay out automatically based on predefined triggers, such as weather events, meeting growing climate risk demands. The parametric insurance market is expected to reach $51.3 billion by 2034. AI-driven predictive analytics and IoT data streams facilitate faster, more transparent risk evaluation and claim settlements.
Embedded insurance—where coverage is seamlessly integrated into product purchases or services—is another emerging trend, projected to comprise 15% of global gross written premiums soon. AI-powered personalization and automation underpin these offerings, aligning insurance more closely with customer lifestyles and preferences.
However, scaling AI capabilities must be balanced with ethical vigilance. While AI can expand coverage and improve operational efficiency, risks remain around exacerbating inequalities or infringing on privacy if safeguards are insufficient. Human oversight remains indispensable—not only as a compliance mechanism but as a strategic partner interpreting AI insights, managing exceptions, and maintaining customer trust.
A Balanced Outlook: Innovation with Ethical Vigilance
AI automation in insurance is a powerful tool—but not a panacea. Success hinges on thoughtful system design, robust governance, and continuous learning. Early adopters that combine technical excellence with strong ethical frameworks will gain a competitive advantage.
Innovation is accelerating rapidly: 76% of U.S. insurers have adopted generative AI in some capacity, and 90% of finance firms report revenue gains from these initiatives. Yet, premature or ungoverned adoption risks pitfalls such as model hallucinations, data poisoning, regulatory non-compliance, and erosion of consumer trust.
The path forward demands:
- Transparent AI governance programs that embed risk identification and mitigation mechanisms.
- Multidisciplinary collaboration involving IT, business, compliance, and ethics experts.
- Continuous human-in-the-loop oversight to ensure fairness, accountability, and adaptability.
- Investment in privacy-preserving technologies like federated learning to safeguard sensitive data.
- Active monitoring and defense against adversarial threats to enhance model robustness.
In sum, the future of AI in insurance automation is both promising and complex. It is a journey of harnessing transformative technology to deliver smarter, fairer, and more responsive insurance ecosystems—while keeping the human impact at the forefront. Insurers who master this balance will lead the industry into its next frontier.
Category | Details | Key Points / Metrics |
---|---|---|
Emerging AI Technologies |
|
|
Technical Challenges |
|
|
Scalability & Innovation |
|
|
Balanced Outlook & Recommendations |
|
|