AI in Finance: Automating Banking, Investing & Fraud Detection
- Introduction: Why AI Is a Game Changer in Finance
- Introduction: Why AI Is a Game Changer in Finance
- The Transformative Power of AI-Driven Automation
- Balancing Potential with Ethical and Risk Considerations
- Previewing the Frontier: Generative AI, Agentic AI, Data Challenges, and Societal Impacts
- Foundations of AI in Financial Services: Technologies and Architectures
- Foundations of AI in Financial Services: Technologies and Architectures
- Core AI Technologies Powering Financial Automation
- Architectures and Training Paradigms: What Sets AI Apart from Traditional Automation?
- Technical Challenges: Data Quality, Interpretability, and Legacy Integration
- Bringing It All Together: The Road Ahead
- Automating Banking Operations: From Customer Service to Risk and Compliance
- Automating Banking Operations: From Customer Service to Risk and Compliance
- AI-Powered Customer Onboarding and Chatbots: Conversational Interfaces That Convert
- Virtual Regulatory Experts and Generative AI: Revolutionizing Compliance and Detection
- Process Efficiency, Risk Assessment, and the Challenges of Scaling AI
- AI in Investments and Wealth Management: Enhancing Decision-Making and Personalization
- AI in Investments and Wealth Management: Enhancing Decision-Making and Personalization
- Predictive Analytics and Personalized Financial Advice: From Data Deluge to Decision Precision
- Generative AI and Synthetic Data: Simulating the Unseen to Manage the Unknown
- The Human-AI Partnership: Validating Models, Mitigating Bias, and Maintaining Oversight
- Real-World Impact: AI Augmenting Financial Planners and Algorithmic Trading
- Fraud Detection and Cybersecurity: AI’s Double-Edged Sword
- Fraud Detection and Cybersecurity: AI’s Double-Edged Sword
- AI’s Power in Detecting and Preventing Financial Fraud
- The Rising Threat of AI-Enhanced Cyber Attacks
- Defensive Architectures and Continuous Learning Systems
- Strategic Responses and the Path Forward
- Benchmarking AI Solutions in Finance: Performance, Risks, and Ethical Considerations
- Benchmarking AI Solutions in Finance: Performance, Risks, and Ethical Considerations
- Performance Metrics: Accuracy, Latency, Scalability, and Compliance
- Navigating the Risks: Model Opacity, Data Privacy, and Cultural Resistance
- Governance Frameworks: Ethical Guardrails, AI Champions, and Transparent Ecosystems
- Cutting Through the Hype: Realistic Expectations for AI in Finance
- Future Directions: Navigating the AI-Enabled Financial Landscape
- Future Directions: Navigating the AI-Enabled Financial Landscape
- Emerging AI Technologies and Trends Shaping Finance
- Workforce Transformation, Financial Inclusion, and Societal Trust
- Uncertainties and Risks in AI Adoption
- Strategic Recommendations for Responsible AI Adoption
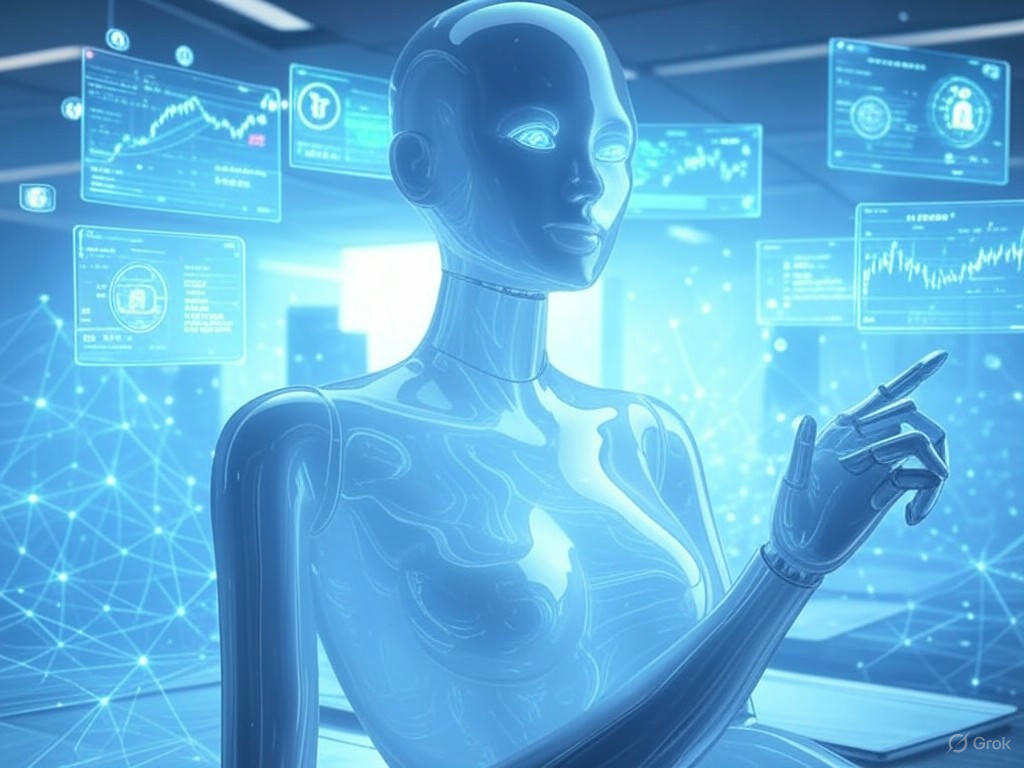
Introduction: Why AI Is a Game Changer in Finance
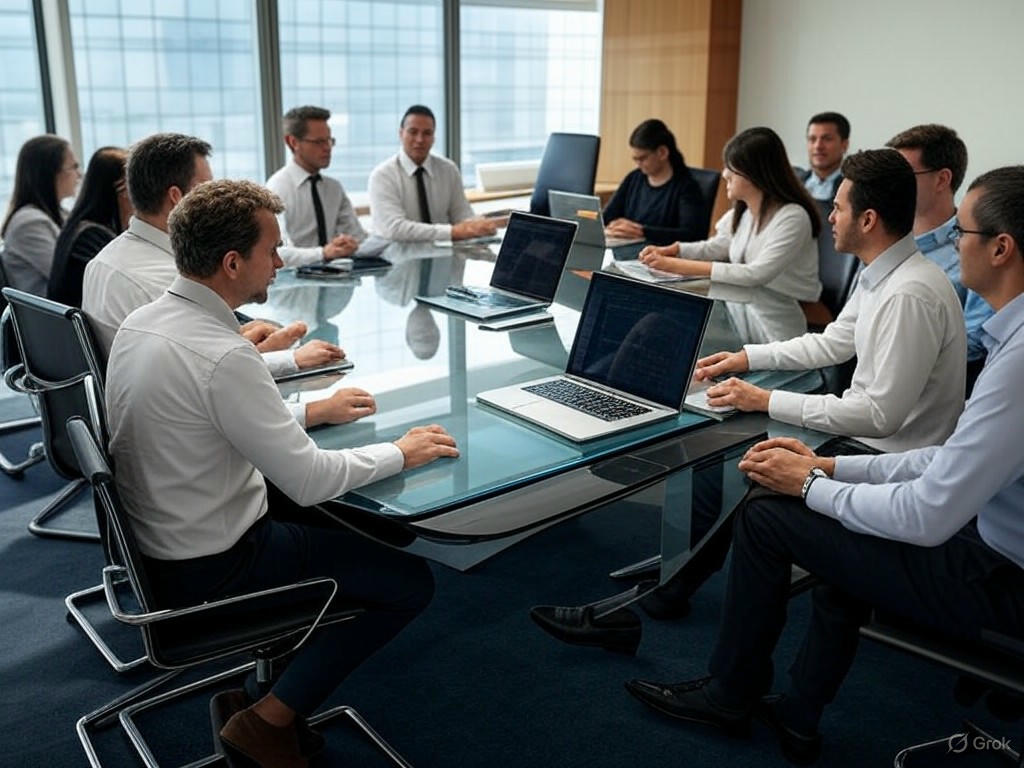
Introduction: Why AI Is a Game Changer in Finance
What does it mean when AI shifts from a niche experiment to the backbone of global finance? As of 2025, artificial intelligence is no longer just a tool for incremental improvement. It is rapidly transforming how banking operations, investment management, and fraud detection are conducted, with profound implications for efficiency, decision-making, and risk management.
The Transformative Power of AI-Driven Automation
The banking sector exemplifies this transformation. By embedding AI deeply into their workflows, banks accelerate routine processes such as loan approvals and account management, often reducing operational costs by hundreds of millions annually. McKinsey predicts that robots will soon perform between 10% and 25% of work in banking operations, ushering in a second wave of automation that transcends simple task execution.
Generative AI (GenAI) is a key catalyst in this evolution. It revolutionizes customer interactions, risk management, and regulatory compliance. For example, GenAI can instantly summarize complex financial documents, freeing bankers to focus on strategic judgment rather than paperwork. In capital markets, AI is reshaping trading and compliance by automating risk assessments and regulatory reporting. Despite these advances, challenges remain—cultural resistance and the delicate balance between innovation costs and returns continue to pose hurdles.
Fraud detection is another domain where AI’s impact is unmistakable. The AI-driven fraud detection market is projected to reach nearly $32 billion by 2029, propelled by AI’s capability to analyze vast datasets in real time and identify suspicious behaviors with unmatched precision. American Express’s deployment of long short-term memory AI models improved fraud detection rates by 6%, illustrating tangible benefits. However, these same AI advances empower cybercriminals to devise increasingly sophisticated attacks, underscoring AI’s dual-edged nature.
Balancing Potential with Ethical and Risk Considerations
AI adoption in finance is not an unchecked race; it is carefully bounded by ethical imperatives and regulatory frameworks. Key questions arise: Where is the line between AI-enabled recommendations and manipulative influence? How do institutions prevent AI systems from perpetuating bias or compromising privacy?
Regulators worldwide are intensifying their focus on AI governance. The EU’s General Data Protection Regulation (GDPR) imposes strict rules on automated decision-making and data privacy, mandating meaningful human oversight of AI outputs. The EU AI Act, enacted in 2024 and effective in 2025, further codifies transparency and accountability requirements for AI systems. AI-generated errors or biased models can cause significant financial and reputational damage if left unchecked.
Industry leaders stress transparency, continuous human intervention, and robust ethical frameworks. As one expert noted, “There is pretty much no compliance without AI” in today’s complex regulatory environment—but that compliance depends fundamentally on responsible AI use. Organizational culture shifts are essential to ensure AI augments human expertise rather than becoming an inscrutable black box.
Previewing the Frontier: Generative AI, Agentic AI, Data Challenges, and Societal Impacts
Looking forward, several key themes will shape the future of AI in finance:
-
Generative AI (GenAI): Beyond data processing, GenAI generates insights, drafts contracts, summarizes due diligence, and customizes communications across stakeholders. Its modular adaptability unlocks new efficiencies in compliance, customer service, and investment management.
-
Agentic AI: Moving past GenAI’s content generation, agentic AI introduces autonomous decision-making abilities. Financial institutions are deploying multiagent systems that coordinate across domains, automating complex workflows with hundreds of specialized AI agents collaborating. This promises to revolutionize credit underwriting, fraud detection, and portfolio management, though it raises governance and trust challenges.
-
Data Challenges: Despite AI’s promise, legacy systems and fragmented data infrastructures remain significant barriers. Finance teams often struggle with data quality, integration, and scaling AI-ready environments. Overcoming these hurdles is crucial to realizing AI’s full potential.
-
Societal and Ethical Impacts: AI’s influence extends beyond operational efficiency, affecting financial inclusion, customer trust, and societal risk. For instance, agentic AI tools could broaden access to financial services for underserved communities but require robust governance to mitigate unintended harms.
In sum, AI is a powerful force reshaping finance’s core functions. This transformation is complex and nuanced—full of opportunity, risk, and responsibility. Success demands balancing technological enthusiasm with thoughtful critique, ensuring AI’s promise translates into tangible, ethical value for institutions and society alike.
Aspect | Description |
---|---|
Banking Automation | AI accelerates routine processes like loan approvals and account management, reducing operational costs by hundreds of millions annually; robots expected to perform 10%-25% of banking operations. |
Generative AI (GenAI) | Revolutionizes customer interactions, risk management, regulatory compliance; summarizes complex documents; automates risk assessments and reporting in capital markets. |
Fraud Detection | AI analyzes vast datasets in real time to identify suspicious behavior; market projected to reach $32 billion by 2029; American Express improved detection rates by 6% using LSTM AI models. |
Ethical & Regulatory Considerations | Focus on transparency, human oversight, bias prevention, and privacy; regulations include EU GDPR and EU AI Act effective 2025; emphasizes responsible AI use and organizational culture shifts. |
Future Themes – Generative AI | Generates insights, drafts contracts, summarizes due diligence, customizes communications; enhances compliance, customer service, and investment management. |
Future Themes – Agentic AI | Enables autonomous decision-making with multiagent systems coordinating complex workflows; impacts credit underwriting, fraud detection, portfolio management; raises governance and trust challenges. |
Future Themes – Data Challenges | Legacy systems and fragmented data infrastructures create barriers; issues with data quality, integration, and AI readiness; overcoming these is crucial. |
Future Themes – Societal & Ethical Impacts | Influences financial inclusion, customer trust, societal risk; agentic AI could expand access to underserved communities but requires strong governance. |
Foundations of AI in Financial Services: Technologies and Architectures
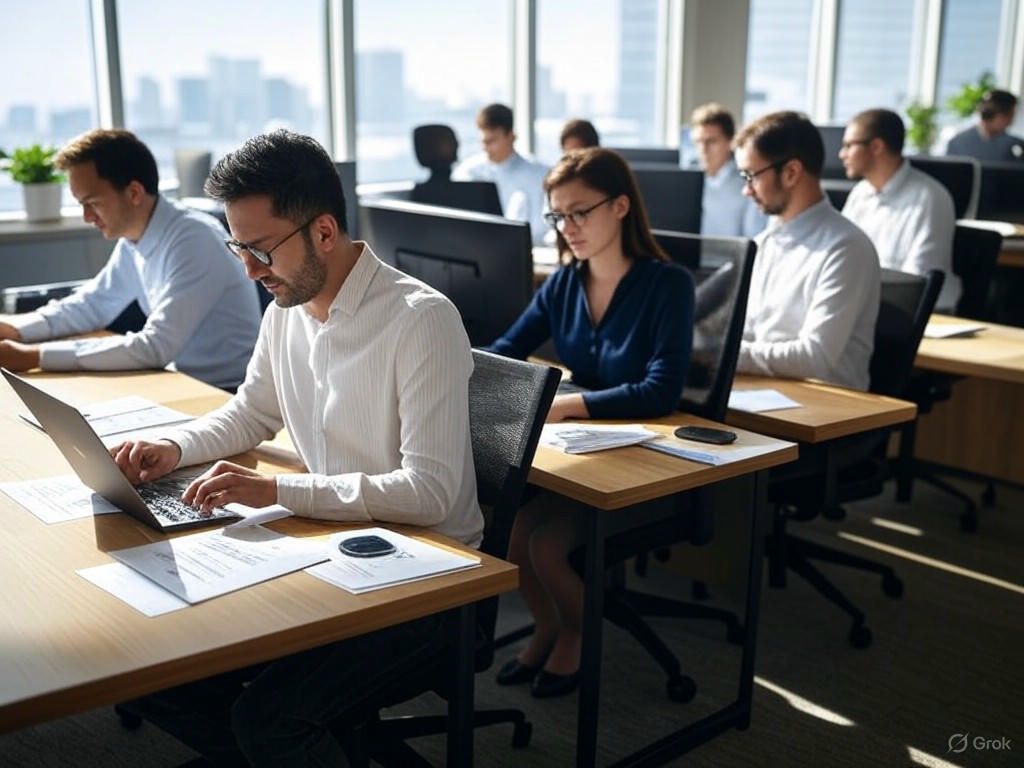
Foundations of AI in Financial Services: Technologies and Architectures
What powers the AI revolution transforming financial services today? To understand this shift, we must look beyond buzzwords and examine the core technologies and architectures driving automation across banking, investments, and fraud detection. These advances are not mere incremental updates to legacy systems; they represent fundamentally new ways to process data, make decisions, and engage customers.
Core AI Technologies Powering Financial Automation
Modern financial automation is built on several distinct yet complementary AI technologies:
-
Machine Learning (ML): Projected to surpass $12 billion in market value by 2032, ML is central to spotting hidden patterns in vast transaction datasets. Its applications include risk management, credit scoring, and regulatory compliance. For instance, Standard Chartered Bank leverages ML-driven transaction monitoring to enhance Anti-Money Laundering (AML) efforts. Meanwhile, Bank of America’s AI assistant, Erica, personalizes customer interactions around the clock, demonstrating ML’s impact on customer experience.
-
Generative AI and Transformers: Generative AI models, particularly transformer-based architectures like GPT, are revolutionizing how banks process and generate language-based content. These models analyze complex financial documents, synthesize realistic customer data for training, and automate regulatory compliance tasks. Citigroup’s use of generative AI to parse over a thousand pages of new U.S. capital rules exemplifies these capabilities. Unlike traditional scripted automation, transformers process entire input sequences simultaneously via self-attention mechanisms, enabling nuanced understanding of context and relationships that earlier models could not achieve.
-
Agentic AI: Going beyond task automation, agentic AI introduces autonomous decision-making. These AI agents perceive their environment, plan, and act independently—much like a seasoned financial advisor adapting to evolving market conditions. The agentic AI market in finance is projected to grow from $0.7 billion in 2024 to over $24 billion by 2034. Use cases range from personalized robo-advisors and dynamic risk assessments to intelligent trading systems. However, this autonomy introduces new governance and transparency challenges that financial institutions must address proactively.
-
Robotic Process Automation (RPA): RPA automates repetitive, rule-based workflows such as data entry, account reconciliation, and standard compliance checks. Think of it as software robots handling routine tasks. While RPA delivers rapid efficiency gains, it lacks learning or adaptability. Combining RPA with AI—known as hyperautomation—enables automation of more complex decisions requiring judgment and adaptation, a necessity for scaling intelligent financial operations.
Architectures and Training Paradigms: What Sets AI Apart from Traditional Automation?
How do modern AI models like transformers and agentic AI differ architecturally and operationally from conventional rule-based systems?
Traditional banking automation is largely rule-based, relying on explicit instructions crafted by domain experts. These systems are predictable and transparent but brittle—they struggle with unstructured data and novel scenarios. For example, a compliance system programmed to flag transactions above a threshold works well within fixed parameters but fails when fraud patterns evolve.
In contrast, AI models learn from data. Transformer architectures, for instance, do not process input sequentially like earlier neural networks. Instead, they employ self-attention mechanisms that weigh the relevance of every part of the input simultaneously. Imagine a financial analyst who can instantly focus on multiple signals—market trends, economic indicators, client sentiment—all at once rather than reading reports line-by-line. This attention mechanism enables models to understand and generate complex financial language, supporting tasks from document analysis to conversational AI.
To visualize self-attention, picture a roundtable discussion where each participant (token) listens to and responds to every other participant, deciding in real time what is most important. Multi-headed attention allows the model to capture diverse relationships simultaneously, enhancing accuracy in financial forecasting or fraud detection.
Agentic AI agents advance this concept by integrating modules for memory, planning, and autonomous action execution. They function like autonomous financial advisors who monitor markets, adjust strategies, and interact with clients without constant human oversight. Unlike rigid automation, these agents learn and adapt continuously but require careful design to ensure interpretability, compliance, and trust.
Technical Challenges: Data Quality, Interpretability, and Legacy Integration
Deploying AI systems in finance is far from plug-and-play. Success hinges on three critical technical pillars:
-
Data Quality and Governance: The adage “garbage in, garbage out” holds especially true. Financial AI systems depend on high-quality, accurate, and well-governed data. Fragmented or low-quality data leads to flawed models and costly errors. Banks often face delays—such as in mortgage approvals—caused by data verification bottlenecks, which can add hours per case. Automated data quality frameworks that validate, cleanse, and monitor data in real time can dramatically improve accuracy and processing speed. KPMG’s 2025 report highlights that 80% of banks see AI as a competitive advantage but emphasize that data governance must be foundational, not an afterthought.
-
Model Interpretability and Explainability: Financial decisions affect lives and markets, making transparency essential. Complex ML models and agentic AI often operate as “black boxes,” complicating regulatory compliance and customer trust. Explainable AI (XAI) techniques aim to make model decisions understandable without sacrificing performance. This transparency is vital in credit lending and fraud detection, where clear explanations build trust and satisfy regulatory scrutiny.
-
Integration with Legacy Banking Systems: Many banks still run COBOL-based mainframes designed for batch processing, not real-time AI workflows. Integrating AI with such legacy infrastructure demands strategic modernization. HSBC’s adoption of AI-driven fraud detection, for example, significantly reduced false positives—but only after layering new AI capabilities atop existing systems. Cloud-native architectures, containerization, and federated learning methods are increasingly essential for scalable AI deployment while safeguarding sensitive customer data during training.
Bringing It All Together: The Road Ahead
Financial institutions that understand the distinctions among these AI technologies and prepare their data and infrastructure accordingly stand to unlock unprecedented operational efficiency and enhanced customer engagement.
- Machine learning forms the backbone for data-driven insights and anomaly detection.
- Generative AI adds sophisticated language understanding and content generation.
- Agentic AI introduces autonomy, enabling faster and more adaptive decisions.
- RPA and hyperautomation handle repetitive tasks and complex workflows in tandem.
This layered AI architecture requires not only technical expertise but also an ethical framework emphasizing transparency, fairness, and regulatory alignment.
In sum, the AI foundation in finance is a complex ecosystem balancing cutting-edge scientific advances with the practical realities of data quality, legacy system integration, and governance. Navigating this landscape thoughtfully is key to harnessing AI’s full promise while managing its inherent risks.
AI Technology | Description | Applications in Finance | Market Projections / Examples | Challenges / Notes |
---|---|---|---|---|
Machine Learning (ML) | Spotting hidden patterns in large transaction data sets using statistical models. | Risk management, credit scoring, regulatory compliance, personalized customer interactions. | Projected >$12 billion market value by 2032; Standard Chartered Bank’s AML transaction monitoring; Bank of America’s AI assistant Erica. | Requires high-quality data; transparency needed for regulatory compliance. |
Generative AI and Transformers | Transformer-based models like GPT for processing/generating language-based financial content. | Document analysis, customer data synthesis, regulatory compliance automation. | Citigroup uses generative AI to parse U.S. capital rules; enables nuanced understanding via self-attention. | Complex architecture; requires large data and computational resources. |
Agentic AI | Autonomous AI agents that perceive, plan, and act independently like financial advisors. | Personalized robo-advisors, dynamic risk assessments, intelligent trading systems. | Projected growth from $0.7 billion (2024) to >$24 billion by 2034. | Governance, transparency, interpretability challenges. |
Robotic Process Automation (RPA) | Software robots automating repetitive, rule-based workflows without learning capacity. | Data entry, account reconciliation, standard compliance checks. | Widely used for rapid efficiency gains; foundation for hyperautomation. | Lacks adaptability; needs combination with AI for complex decisions. |
Technical Challenge | Description | Examples / Notes |
---|---|---|
Data Quality and Governance | High-quality, accurate, well-governed data needed to avoid flawed models and errors. | Mortgage approval delays due to data verification; KPMG: 80% banks see AI as advantage but stress governance. |
Model Interpretability and Explainability | Making complex AI decisions transparent and understandable for trust and compliance. | Essential in credit lending and fraud detection; use of Explainable AI (XAI) techniques. |
Integration with Legacy Banking Systems | Challenges integrating AI with COBOL-based mainframes and batch processing systems. | HSBC reduced fraud false positives by layering AI atop legacy; cloud-native and federated learning methods used. |
Automating Banking Operations: From Customer Service to Risk and Compliance
Automating Banking Operations: From Customer Service to Risk and Compliance
What if the tedious tasks that bog down banking operations—customer onboarding, compliance checks, risk assessments—could be handled seamlessly, accurately, and at scale? Thanks to AI-driven automation, this vision is rapidly becoming a reality, fundamentally reshaping how banks operate from the inside out.
AI-Powered Customer Onboarding and Chatbots: Conversational Interfaces That Convert
Customer onboarding is one of the most friction-heavy points in banking workflows. Over 35% of bank customers defect to competitors due to poor onboarding experiences. Traditional onboarding often involves complex forms, identity verifications, and regulatory checks that frustrate customers and slow operations.
Generative AI and machine learning-powered conversational interfaces are transforming these static forms into dynamic, dialogue-driven experiences. Customers can ask questions and receive instant clarifications, making onboarding more intuitive and personalized. For example, LiveX AI offers an AI Agent that automates routine queries and proactively enhances customer retention by delivering empathetic, tailored support across chat, voice, and email channels.
Banks like LHV and Mashreq have deployed AI assistants—“Uku” and “BankAssist,” respectively—that handle thousands of transactions and inquiries, reducing call center overload by up to 20%. This automation not only improves customer satisfaction but also accelerates account setup, reduces human error in documentation and Know Your Customer (KYC) compliance, and frees human agents for higher-value tasks. Given that up to 20% of inbound calls historically concern onboarding status updates, AI chatbots effectively cut a significant source of inefficiency.
Virtual Regulatory Experts and Generative AI: Revolutionizing Compliance and Detection
Compliance remains an operational minefield for banks. Regulatory requirements are complex, evolving, and non-negotiable. Manual compliance workflows are slow, costly, and prone to oversight.
AI-driven virtual regulatory experts are streamlining regulatory inquiries and risk assessments. For instance, Banco Montepio’s partnership with Devoteam produced an Intelligent Knowledge Management system powered by advanced AI chatbots. These virtual assistants parse dense regulatory texts, answer compliance questions in real-time, and guide personnel through complex rules without the usual delays.
Generative AI further automates the creation and refinement of detection rules for fraud, money laundering, and risk assessment. Citigroup’s use of generative AI to analyze over 1,000 pages of capital regulations exemplifies how AI accelerates regulatory understanding and application. This automation not only speeds compliance but also enhances accuracy by reducing the risk of human misinterpretation.
Fraud detection also benefits from AI’s continuous learning. Platforms like DataDome combine machine learning at the edge with real-time detection to block fraudulent transactions within milliseconds—a critical capability given that reported fraud losses rose by 14% in 2023. However, as AI tools become more sophisticated, banks must remain vigilant against AI-powered fraud techniques employed by adversaries.
Process Efficiency, Risk Assessment, and the Challenges of Scaling AI
AI-driven automation promises faster processes, fewer errors, and improved risk management. For example, AI-powered capital adequacy assessments enable banks to respond swiftly to market fluctuations and regulatory changes without delay.
Yet, scaling AI solutions enterprise-wide presents significant challenges:
-
Data Governance: Robust frameworks are essential to ensure data quality, security, and accessibility. This is no longer a mere compliance checkbox but a strategic enabler. Financial institutions with mature data governance outperform peers by an average of 22% in revenue growth.
-
Regulatory Constraints: The pace of AI innovation often outstrips regulatory comprehension. Banks face uncertainty about which AI applications require scrutiny and how to maintain transparency. The EU’s GDPR imposes strict rules on automated decision-making, requiring AI workflows to avoid bias and discrimination.
-
Talent Shortages: A critical shortage of AI and generative AI experts limits widespread adoption and effective scaling.
-
Infrastructure Modernization: Legacy banking systems, often COBOL-based mainframes designed for batch processing, impede real-time AI integration. Modernizing infrastructure toward modular, cloud-enabled architectures is vital. IBM’s advances in hybrid AI platforms exemplify efforts to enable seamless AI scaling across fragmented enterprise environments.
-
Risk Management: AI systems introduce new risks, including inaccuracies, cybersecurity vulnerabilities, and intellectual property concerns. Leading consultancies recommend phased, carefully monitored AI deployments balanced with ethical considerations and continuous oversight, as emphasized in frameworks like NIST’s AI Risk Management Framework.
Despite these hurdles, successful navigation yields substantial rewards. Forrester predicts that by 2025, digital banking experiences will be more humanlike, connected, and empowering—powered by conversational AI interfaces that bring autonomous finance closer to everyday reality.
In summary, AI-driven automation is redefining banking operations—from customer onboarding to compliance and risk assessment. Unlocking its full potential requires balancing technological innovation with rigorous governance, regulatory alignment, and ethical stewardship. Only then can banks transform operational efficiency into a sustainable competitive advantage, delivering smarter, faster, and more trustworthy financial services.
Aspect | AI Application | Benefits | Examples |
---|---|---|---|
Customer Onboarding & Chatbots | Generative AI-powered conversational interfaces | Improves onboarding experience, reduces call center overload by up to 20%, accelerates account setup, reduces human error, enhances customer retention | LiveX AI Agent, LHV’s “Uku”, Mashreq’s “BankAssist” |
Compliance & Regulatory Expertise | AI-driven virtual regulatory experts, generative AI for rule creation | Streamlines regulatory inquiries, real-time compliance guidance, speeds up regulatory understanding, reduces human misinterpretation | Banco Montepio with Devoteam, Citigroup’s generative AI for capital regulations |
Fraud Detection | Machine learning at the edge, real-time detection | Blocks fraudulent transactions within milliseconds, adapts to evolving fraud techniques | DataDome platform |
Process Efficiency & Risk Assessment | AI-powered capital adequacy assessments and risk management | Enables swift response to market and regulatory changes, reduces errors | Not specified |
Challenges in Scaling AI | Data governance, regulatory compliance, talent shortages, infrastructure modernization, risk management | Requires robust data frameworks, regulatory alignment, skilled experts, modern infrastructure, phased and ethical AI deployment | IBM hybrid AI platforms, NIST AI Risk Management Framework |
AI in Investments and Wealth Management: Enhancing Decision-Making and Personalization
AI in Investments and Wealth Management: Enhancing Decision-Making and Personalization
How is AI transforming the traditionally human-centric domain of investment and wealth management? The answer lies in the rising sophistication of predictive analytics, personalized financial advice, and synthetic data-driven simulations. These innovations are reshaping portfolio construction, risk assessment, and strategy optimization, enabling more precise and adaptive investment decisions.
Predictive Analytics and Personalized Financial Advice: From Data Deluge to Decision Precision
AI-powered predictive analytics has become foundational in asset management, with the global market projected to grow from $3.4 billion in 2024 to $21.7 billion by 2034. This rapid expansion highlights AI’s ability to unlock insights previously beyond human reach.
By analyzing vast, heterogeneous datasets—including market trends, economic indicators, and subtle behavioral patterns—AI models reveal asset correlations that human analysts might miss. According to Professional Wealth Management, AI could redefine asset management over the next decade, evolving investment professionals into hybrid strategists fluent in both finance and machine learning.
A key differentiator is AI’s scalability in delivering personalized financial advice. For example, WealthFlow Solutions leverages generative AI to tailor investment recommendations precisely to individual clients’ goals and risk tolerances. This customization fosters stronger client engagement and trust, as 80% of investors now show growing openness to AI-supported portfolio advice.
Personalization extends beyond static recommendations. AI tools continually adjust portfolio allocations in response to real-time data and shifting market conditions, optimizing returns while managing downside risks. Financial institutions report that this dynamic approach improves efficiency, reduces operational costs, and enhances scalability in asset management.
Generative AI and Synthetic Data: Simulating the Unseen to Manage the Unknown
Imagine a financial crystal ball capable of simulating thousands of market scenarios—including those never observed historically. Generative AI and synthetic data are turning this vision into reality, enabling more robust risk modeling and portfolio optimization.
Synthetic data—artificial datasets that statistically replicate real financial data without compromising privacy—accelerates AI development cycles by up to 65%. JPMorgan’s AI research team uses synthetic data to amplify rare but critical examples, enhancing machine learning training and model resilience.
Agent-based market simulators advance this further by modeling diverse trader behaviors and complex market dynamics over extended periods. These simulators provide a sandbox for testing investment strategies across varied economic assumptions. The AWS HPC Blog notes that such simulations uncover risk factors often missed by traditional historical backtesting, increasing confidence in strategy robustness.
Generative AI also empowers “what-if” analyses for dynamic financial planning. CFOs increasingly rely on these models to anticipate the impacts of geopolitical events, regulatory changes, and market volatility on their portfolios. This proactive use transforms compliance from a reactive task into a strategic advantage.
The Human-AI Partnership: Validating Models, Mitigating Bias, and Maintaining Oversight
Despite AI’s impressive capabilities, the financial sector faces critical challenges in ensuring model reliability, fairness, and ethical application. Investment AI systems often employ complex algorithms such as deep learning and natural language processing, whose decision-making processes can be opaque.
Model validation is essential—not optional. Financial institutions must rigorously test AI models, documenting assumptions, performance metrics, and potential failure modes. Experts from Coherent Solutions and Kaufman Rossin emphasize that transparency is vital, both for regulatory compliance and to build client trust.
Bias mitigation remains a top priority. Research from IJIRCT and Stanford reveals that AI models can inadvertently perpetuate systemic inequities if trained on biased data. Techniques like pruning biased behaviors in large language models show promise, but ultimate responsibility lies with firms to continuously monitor and correct biases relevant to their use cases.
Human oversight is indispensable. While AI-driven algorithmic trading and portfolio management excel at speed and pattern recognition, they are not infallible. Studies indicate the most effective approach blends AI’s analytical power with human judgment and contextual understanding. Humans remain superior at interpreting qualitative factors such as sudden geopolitical risks or regulatory shifts that AI may not fully capture.
Ethical considerations further demand accountability and transparency. Firms must ensure AI systems operate fairly, avoid exacerbating market volatility, and comply with evolving regulatory frameworks. The future is not about AI replacing human advisors but augmenting them—empowering financial professionals to deliver superior outcomes with AI as an essential tool.
Real-World Impact: AI Augmenting Financial Planners and Algorithmic Trading
Several industry leaders exemplify AI’s transformative impact. Platforms like CAIS and iCapital streamline alternative investments by automating complex workflows that once required extensive manual effort. Zeplyn’s AI Support Assistant enhances advisor productivity and client satisfaction by generating personalized client meeting agendas.
In algorithmic trading, AI-driven models execute trades within milliseconds, analyzing market signals far faster than human traders. However, this speed introduces regulatory scrutiny to prevent unintended volatility. As YourRoboTrader highlights, integrating human oversight is critical to detect algorithmic anomalies and maintain market stability.
The organizational impact is profound. The World Economic Forum observes that investment firms are becoming leaner but more technically sophisticated, blending finance professionals with AI specialists. This fusion reflects a strategic embrace of both domain expertise and technological prowess.
Key Takeaways:
- AI-driven predictive analytics and personalization are revolutionizing investment strategies, enabling nuanced and scalable financial advice.
- Synthetic data and generative AI empower scenario simulation and risk modeling beyond historical constraints, fostering more resilient portfolios.
- Rigorous model validation, bias mitigation, and ethical oversight are crucial to harness AI responsibly in finance.
- The future favors collaborative human-AI partnerships rather than wholesale automation, preserving essential human judgment.
- Real-world deployments demonstrate measurable benefits in efficiency, client engagement, and risk management, heralding a new era in wealth management.
As AI continues to advance rapidly, the investment world must navigate this frontier with both enthusiasm and caution—leveraging AI’s strengths while vigilantly managing its limitations and ethical implications.
Aspect | Description | Impact/Example |
---|---|---|
Predictive Analytics | Uses AI to analyze vast heterogeneous datasets to reveal asset correlations and market trends | Market projected to grow from $3.4B in 2024 to $21.7B by 2034; enables precise investment decisions |
Personalized Financial Advice | AI tailors investment recommendations to individual goals and risk tolerances | WealthFlow Solutions’ generative AI; 80% investors open to AI-supported advice; dynamic portfolio adjustments |
Generative AI & Synthetic Data | Creates artificial datasets to simulate thousands of market scenarios, including unseen ones | JPMorgan uses synthetic data to enhance model resilience; accelerates AI development cycles by 65% |
Agent-Based Market Simulators | Models trader behaviors and complex market dynamics over time for strategy testing | Uncovers risk factors missed by historical backtesting; improves confidence in strategy robustness |
Human-AI Partnership | Combines AI analytics with human judgment for oversight, bias mitigation, and ethical compliance | Model validation essential; addresses bias; preserves qualitative interpretation of geopolitical/regulatory risks |
Real-World AI Impact | Automates workflows, boosts advisor productivity, and accelerates algorithmic trading | Platforms like CAIS, iCapital, Zeplyn; AI-driven trades within milliseconds; requires human oversight for stability |
Key Takeaways | Summary of AI benefits and challenges in investment and wealth management | Revolutionizes strategy, improves personalization, requires ethical oversight, favors collaboration, enhances efficiency |
Fraud Detection and Cybersecurity: AI’s Double-Edged Sword
Fraud Detection and Cybersecurity: AI’s Double-Edged Sword
What happens when the very technology designed to protect financial systems becomes a tool exploited by malicious actors? AI has transformed fraud detection, enabling unprecedented precision and speed. Yet, this same technology fuels a new generation of cyber threats, creating a paradox that financial institutions must navigate carefully to safeguard assets and maintain trust.
AI’s Power in Detecting and Preventing Financial Fraud
AI-driven fraud detection is no longer a futuristic concept—it is a rapidly growing market, projected to reach $31.69 billion by 2029 with a compound annual growth rate near 20%. Machine learning algorithms lie at the heart of this expansion, analyzing vast transaction datasets to identify anomalies that human analysts might miss.
Key AI techniques include:
- Supervised learning models trained on historical fraud data to recognize known patterns.
- Unsupervised learning models that act like digital detectives, flagging unusual behaviors without prior examples.
- Reinforcement learning systems that adapt dynamically over time, much like training a dog to respond to new commands.
Together, these approaches enable detection of a broad spectrum of fraud—from credit card theft to complex money laundering schemes.
For instance, American Express enhanced its fraud detection rates by 6% using advanced long short-term memory (LSTM) networks, demonstrating how deep learning models can anticipate subtle patterns over time. Platforms like DataDome operate at the edge, delivering real-time bot detection and blocking fraudulent activities within milliseconds—critical as 65% of businesses remain vulnerable to even basic automated attacks.
Beyond detection, AI also strengthens fraud prevention through omnichannel, AI-driven identity verification systems. These solutions help banks and credit unions thwart fraud attempts without compromising customer experience, striking a crucial balance between security and usability. Poor onboarding experiences contribute to over 35% of customer defection, underscoring the need for seamless but robust verification.
The Rising Threat of AI-Enhanced Cyber Attacks
However, AI’s double-edged nature is evident as fraudsters harness the same technologies to outwit defenses. This has sparked an arms race challenging traditional security paradigms.
Malicious actors deploy AI-powered bots capable of:
- Mimicking human behavior convincingly.
- Automating social engineering attacks.
- Rapidly probing system vulnerabilities.
Bad bot activity now accounts for 37% of all internet traffic, engaging in data scraping, account takeovers, and inventory manipulation while often evading signature-based detection.
More alarming is the emergence of adversarial AI—techniques designed to deceive or manipulate AI models themselves. Attackers use data poisoning, prompt injection, and model evasion tactics to cause fraud detection systems to misclassify or overlook suspicious behavior. For example, fraud rings may train their own AI models on legitimate transaction data to mimic authentic patterns, making fraudulent activity indistinguishable from normal behavior.
The threat landscape intensifies in 2025, with a 400% increase in tracked threat actors globally. Nation-state actors from North Korea, Iran, Russia, and China are escalating attacks, blending AI-powered cyber threats with sophisticated social engineering. The FBI’s Internet Crime Complaint Center highlighted that phishing attacks nearly tripled between 2019 and 2023, amplified by AI-generated deceptive content.
Defensive Architectures and Continuous Learning Systems
Financial institutions counter these challenges with adaptive, layered defenses that combine AI innovation with human oversight and transparency.
Key strategies include:
-
AI red teaming: Ethical hacking teams simulate attacks on AI systems to uncover vulnerabilities before adversaries exploit them. Red and blue teams engage in a continuous “discover, defend, and iterate” cycle, stress-testing models against adversarial inputs and refining defenses.
-
Continuous learning systems: Fraud detection models evolve dynamically to recognize emerging threats, reducing false positives and catching novel fraud patterns. This adaptability is essential in a rapidly changing threat environment.
-
Explainable AI (XAI): Transparency is critical for trust and regulatory compliance. Explainable AI techniques reveal how models reach conclusions, answering questions like “Why was this transaction flagged?” Lucinity’s platform exemplifies this by aggregating case data and surfacing risk indicators with human-readable summaries, empowering investigators to make informed decisions.
-
Blockchain integration: Immutable, timestamped transaction records enhance traceability and reduce risks such as account takeover and friendly fraud. Blockchain complements AI’s pattern recognition with a secure audit trail.
Additionally, federated learning enables collaborative fraud detection across institutions without sharing raw data, addressing privacy and regulatory concerns. Advanced encryption techniques—such as homomorphic encryption and secure multi-party computation—allow AI computations on encrypted data, further safeguarding sensitive information.
Strategic Responses and the Path Forward
The evolving threat landscape demands a multidisciplinary and proactive approach. Financial institutions must:
- Invest in skilled AI and cybersecurity talent to bridge technical defenses with strategic risk management.
- Foster collaboration among industry peers, regulators, and technology providers to share threat intelligence and best practices.
- Modernize IT infrastructure to support modular, scalable AI-powered security systems that integrate seamlessly with legacy platforms.
- Adopt ethical frameworks governing AI use, balancing innovation with accountability and compliance.
- Prioritize continuous employee training to recognize AI-enhanced social engineering and phishing tactics.
AI’s double-edged nature in finance reminds us that technology alone is no panacea. Success hinges on combining state-of-the-art machine learning with human expertise, robust governance, and transparency.
Ultimately, maintaining trust—from customers and regulators alike—depends not only on how effectively AI detects and prevents fraud but also on how responsibly and transparently it operates in a landscape where the line between defender and attacker grows ever more blurred.
Category | Details |
---|---|
AI Techniques in Fraud Detection | Supervised learning, Unsupervised learning, Reinforcement learning |
Market Projection | $31.69 billion by 2029, ~20% CAGR |
Use Case Examples | American Express: 6% fraud detection improvement with LSTM; DataDome: real-time bot detection blocking fraudulent activities in milliseconds |
AI-Enhanced Cyber Attack Methods | Human behavior mimicking bots, Automated social engineering, Rapid vulnerability probing, Adversarial AI (data poisoning, prompt injection, model evasion) |
Bad Bot Activity | 37% of all internet traffic; activities include data scraping, account takeovers, inventory manipulation |
Emerging Threats | 400% increase in tracked threat actors by 2025; nation-state actors (North Korea, Iran, Russia, China); Tripled phishing attacks (2019-2023) amplified by AI-generated content |
Defensive Strategies | AI red teaming, Continuous learning systems, Explainable AI (XAI), Blockchain integration, Federated learning, Advanced encryption (homomorphic encryption, secure multi-party computation) |
Strategic Responses | Invest in AI & cybersecurity talent, Foster collaboration, Modernize IT infrastructure, Adopt ethical AI frameworks, Continuous employee training |
Key Challenges | Balancing security with customer experience, Misclassification risks, Privacy and regulatory compliance, Maintaining trust and transparency |
Benchmarking AI Solutions in Finance: Performance, Risks, and Ethical Considerations
Benchmarking AI Solutions in Finance: Performance, Risks, and Ethical Considerations
How do we measure the true impact of AI in finance beyond the hype? In 2025, the financial sector’s AI landscape is a mosaic of high-potential breakthroughs and sobering challenges. To cut through the noise, let’s examine how leading AI implementations in banking, investments, and fraud detection perform against key metrics—and what risks and governance frameworks must accompany their deployment.
Performance Metrics: Accuracy, Latency, Scalability, and Compliance
AI’s promise in finance often hinges on measurable improvements in accuracy, speed, scalability, and regulatory compliance. Fraud detection exemplifies this well: AI models now routinely surpass traditional rule-based systems by identifying subtle, evolving fraud patterns that humans or static algorithms miss. For instance, the Edgar Research finance agent benchmark highlights a critical performance-cost trade-off among AI models tackling complex financial queries. The top-performing model, O3, achieved 48.3% accuracy at a cost of $3.69 per question, while Claude 3.7 Sonnet balanced 42.9% accuracy with a far more economical $0.99 per session. This underscores a classic frontier tension: pushing accuracy higher demands greater computational resources and more sophisticated model architectures.
Banking AI adoption is widespread but uneven. IBM’s 2025 study reveals that only 8% of banks develop generative AI (GenAI) systematically, while 78% adopt a tactical, piecemeal approach. This cautious stance reflects banks’ prioritization of latency and scalability in customer-facing automation and risk management. JPMorgan Chase leads in AI use for risk management and payment validation, cutting operational costs by up to 22%—translating into billions saved industry-wide.
Investment management increasingly embraces specialized small language models (SLMs) as “copilots” to augment decision-making complexity without overwhelming human managers. Deloitte’s 2025 Tech Trends report details how firms orchestrate multiagent AI architectures, deploying SLMs for discrete tasks such as quantitative research, portfolio risk assessment, and regulatory reporting. The scalability of these solutions depends heavily on robust AI infrastructure investments—cloud platforms, neural networks, and dedicated hardware—that enable low latency and high throughput.
Compliance tools have evolved from static rule-checkers to adaptive, self-learning AI systems. Platforms like SAS Viya and AI chatbots automate real-time regulatory monitoring, reducing false positives and operational costs. Still, compliance accuracy must be balanced with explainability—a critical demand from regulators and customers alike—to maintain trust and satisfy legal mandates.
Navigating the Risks: Model Opacity, Data Privacy, and Cultural Resistance
What happens when AI’s black box conflicts with finance’s transparency mandates? Model opacity remains one of the most challenging issues. Financial institutions face intense regulatory pressure to explain AI-driven decisions, especially in credit scoring and fraud detection. Lack of clear model interpretability risks compliance violations and consumer distrust.
Federated learning offers a promising approach by enabling collaborative fraud detection without sharing raw data. However, its complexity introduces new governance challenges, requiring multidisciplinary oversight to ensure compliance and security.
Data privacy is another tightrope. With 72% of financial institutions adopting AI, the volume of sensitive data processed is enormous. A 2025 survey shows only 40% of banking customers trust their banks to be transparent about cybersecurity measures. AI’s insatiable appetite for data collides with emerging regulations like California’s Generative AI Accountability Act and the EU’s AI Act, both mandating transparency and accountability in AI systems.
Organizational resistance is less visible but equally impactful. Despite clear operational benefits, cultural inertia slows AI adoption. Many firms grapple with legacy systems and workforce fears about automation replacing jobs. Architech’s 2025 analysis notes that while AI could cut global banking costs by $300 billion and boost productivity by 5%, many banks still “figure out” how best to integrate these tools. Success stories like NatWest’s Cora+ pilot demonstrate that embedding AI requires not only technology but comprehensive change management and reskilling efforts.
Governance Frameworks: Ethical Guardrails, AI Champions, and Transparent Ecosystems
How do financial institutions balance rapid AI innovation with accountability? Robust governance frameworks embedding ethics, transparency, and compliance from the outset are becoming essential.
“Gen AI champions” emerge as vital cross-functional leaders who navigate the technical and ethical complexities of AI deployment. They ensure AI initiatives align with business objectives, regulatory mandates, and societal expectations. According to MIT Sloan Management Review, AI literacy is becoming a core pillar of corporate AI strategies, empowering teams to understand model capabilities and limitations.
Ethical guardrails are no longer optional. The UNESCO Recommendation on the Ethics of Artificial Intelligence sets a global standard emphasizing privacy, fairness, and human dignity—principles financial firms must operationalize. Automated compliance tools continuously audit AI models for bias, accuracy, and regulatory alignment. Firms adhering to frameworks like NIST’s AI Risk Management Framework report shorter incident lifecycles and reduced exposure to catastrophic risks.
Transparency is the keystone of trust in AI ecosystems. Financial institutions increasingly adopt open, auditable AI pipelines where data provenance, model training, and decision rationale are documented and accessible to regulators and auditors. This goes beyond regulatory box-checking; explainability becomes a competitive differentiator as customers demand clarity on AI-driven financial advice and credit decisions.
Cutting Through the Hype: Realistic Expectations for AI in Finance
AI in finance is not a magic wand—it’s a powerful tool with nuanced trade-offs. While automation delivers measurable cost savings and operational agility, overreliance on AI without human oversight risks ethical lapses and systemic vulnerabilities.
The promise of AI to revolutionize banking, investments, and fraud detection is real but bounded by data quality, infrastructure readiness, and regulatory constraints. Financial institutions must resist chasing the latest model or platform without clear understanding of fit and impact.
Success hinges on:
- Rigorous benchmarking of AI performance against real-world metrics such as latency, accuracy, and compliance adherence.
- Proactive risk management addressing model opacity, data privacy, and workforce adaptation.
- Establishing governance frameworks with dedicated AI champions, ethical guardrails, and transparent practices.
- Investing in AI literacy and infrastructure for sustainable, scalable integration.
In 2025, the most successful financial firms wield AI not as flashy innovation, but as a responsibly governed, strategically embedded asset that enhances decision-making while safeguarding trust.
AI Solution | Performance Metrics | Key Statistics | Risks | Governance Considerations |
---|---|---|---|---|
Fraud Detection AI | Accuracy, Latency, Scalability, Compliance | O3 Model: 48.3% accuracy, $3.69 per question Claude 3.7 Sonnet: 42.9% accuracy, $0.99 per session |
Model opacity, data privacy, regulatory compliance | Explainability, federated learning governance, data security |
Banking AI | Latency, Scalability, Operational Cost Reduction | 8% banks systematic GenAI adoption 78% tactical adoption JPMorgan Chase: 22% cost reduction |
Cultural resistance, legacy systems, workforce fears | Change management, reskilling, AI literacy |
Investment Management AI | Scalability, Accuracy, Throughput | Use of Small Language Models (SLMs) for quantitative research, risk assessment, reporting Heavy infrastructure investment |
Infrastructure readiness, data quality | Robust AI infrastructure, multiagent orchestration, compliance |
Compliance AI Tools | Accuracy, Explainability, Real-time Monitoring | Reduced false positives and operational costs using SAS Viya and AI chatbots | Balancing accuracy with explainability | Transparent audit trails, regulatory alignment |
Governance Frameworks | Ethics, Transparency, Accountability | Implementation of ethical guardrails (UNESCO standards), AI Risk Management Framework (NIST) | Ethical lapses, lack of accountability | AI champions, continuous auditing, open AI pipelines |
Future Directions: Navigating the AI-Enabled Financial Landscape
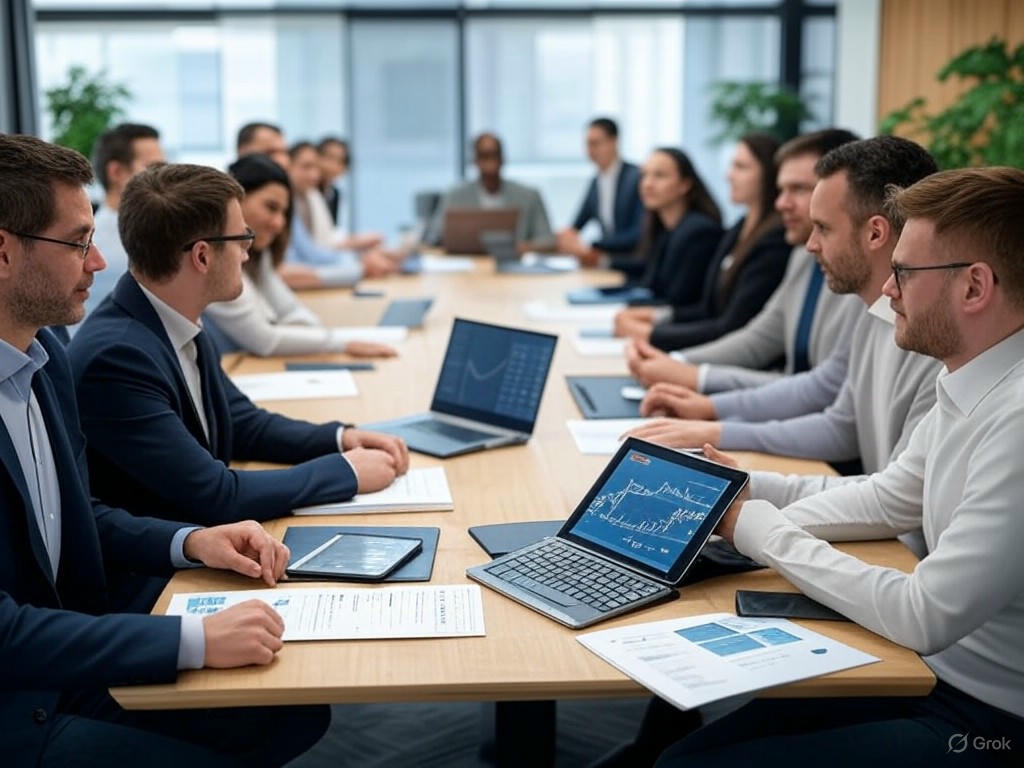
Future Directions: Navigating the AI-Enabled Financial Landscape
What lies ahead for AI in finance—not only in terms of technology but also people, trust, and systemic stability? As we progress through 2025, three interlinked trends are fundamentally reshaping the financial ecosystem: the maturation of agentic AI, the integration of generative AI into real-time decision-making, and an increasingly sophisticated regulatory environment focused on responsible AI governance.
Emerging AI Technologies and Trends Shaping Finance
Agentic AI—systems capable of autonomous goal-setting and strategic decision-making—is transitioning from speculative promise to operational reality. Financial institutions are moving beyond pilot projects, embedding agentic AI deeply into core processes. Industry experts suggest that 2025 may mark the point when AI maturity becomes “table stakes” in banking and investment services, evolving from simple automation to strategic partnership between AI and human teams.
However, this shift demands caution. The hype around agentic AI risks eclipsing the critical need for rigorous validation and risk management. Financial data complexity and stringent regulatory frameworks require AI systems to be transparent, explainable, and robust. Without these safeguards, the risks of erroneous outputs or unintended consequences could undermine trust and stability.
Parallelly, generative AI is revolutionizing data-driven decision-making. Surveys indicate that 65% of enterprises regularly use generative AI, reporting efficiency gains up to 40% and operational cost reductions near 30%. In finance, generative AI produces tailored insights in real time—customizing risk assessments, investment recommendations, and fraud detection. Platforms like Wealthfront and Numer AI illustrate how AI algorithms analyze behavioral patterns and market signals at speeds and scales unattainable by humans alone.
Yet integrating these technologies entails challenges beyond technical adaptation. Institutions must invest in specialized hardware, cloud migration, and new efficacy measurement frameworks. More importantly, they must navigate a complex and evolving regulatory landscape. The European Union’s AI Act, enacted in 2024, alongside emerging global frameworks, underscores the need for transparency, fairness, and accountability as AI systems gain autonomy and influence.
Workforce Transformation, Financial Inclusion, and Societal Trust
AI’s impact extends deeply into the human dimension of financial services. The workforce is undergoing a profound transformation as AI automates routine tasks and augments decision-making. According to McKinsey, 92% of companies plan to increase AI investments, yet only 1% consider themselves “mature” in AI deployment—highlighting a persistent implementation gap.
In wealth management, AI-driven investment tools are projected to become the primary source of advice for retail investors by 2027. Trust remains pivotal; the “trust equation” in financial advice—comprising competence, reliability, intimacy, and self-orientation—is being tested as machines assume advisory roles. While over 80% of investors express openness to AI-supported advisors, the future likely lies in hybrid models where AI empowers human advisors rather than replaces them outright.
Financial inclusion stands to benefit significantly from AI’s capabilities. By analyzing non-traditional data sources, AI can extend credit and services to underserved populations, helping reduce historic barriers. However, this promise depends on ethical AI design and stringent oversight to prevent the entrenchment of biases that could exacerbate inequality.
Building societal trust in AI requires transparency in data use and governance. Cisco’s 2025 privacy study found that 90% of respondents feel safer when their data is stored locally, and nearly half admit to sharing sensitive information with generative AI tools. Financial institutions and technology providers must prioritize governance frameworks that safeguard privacy while unlocking AI’s transformative potential.
Uncertainties and Risks in AI Adoption
No discussion of AI’s future in finance is complete without addressing the inherent risks. The rapid proliferation of AI introduces new systemic vulnerabilities alongside its benefits.
Data demands are escalating rapidly. Financial institutions face challenges in accessing, processing, and governing vast, fragmented datasets. Legacy systems—often COBOL-based mainframes designed for batch processing—impede smooth AI integration, slowing adoption despite clear potential. Furthermore, AI robustness remains a concern; models trained on historical data may falter when confronted with unprecedented market shocks or adversarial attacks.
Systemic risks also arise from market concentration. Over half of AI investment funnels into a handful of dominant firms, creating oligopolistic dynamics that could propagate failures across the financial ecosystem. Cybersecurity threats are escalating, with phishing attacks nearly tripling between 2019 and 2023, amplified by AI-generated deceptive content. While AI enhances defenses through anomaly detection and layered security, adversaries increasingly weaponize AI, underscoring a double-edged sword.
Financial regulators, including the Bank of England and the European Central Bank, actively assess these dynamics. They emphasize AI’s dual nature as both a productivity booster and a potential source of systemic risk. Macroprudential oversight must evolve to address AI’s pervasive integration and the economic risks tied to significant upfront investments.
Strategic Recommendations for Responsible AI Adoption
For financial institutions aiming to harness AI responsibly and sustainably, a comprehensive, multi-pronged strategy is essential:
-
Prioritize Explainability and Validation
Implement AI systems with transparent decision-making processes. Regularly validate AI performance against real-world outcomes, especially for agentic AI deployed in high-stakes functions such as credit underwriting and fraud detection. -
Invest in Human-AI Collaboration
Develop workforce skills that complement AI capabilities. Promote hybrid models where AI augments human judgment, preserving trust, accountability, and the “human touch” vital to financial advice. -
Enhance Data Governance and Privacy
Establish robust data management frameworks that ensure compliance with evolving regulations such as the EU AI Act and GDPR. Employ localized data storage and strict access controls to build user confidence and comply with data sovereignty requirements. -
Engage Proactively with Regulators
Collaborate with policymakers to shape balanced regulations that foster innovation while managing systemic and ethical risks. Early alignment reduces operational uncertainty and enhances compliance readiness. -
Mitigate Concentration and Cybersecurity Risks
Diversify AI vendors and build resilient cybersecurity infrastructures incorporating AI-driven defense mechanisms. Prepare for adversarial threats by adopting layered security measures, including multi-factor authentication and behavioral anomaly detection. -
Embed Ethical Frameworks
Address biases and fairness proactively. Implement ethical AI principles that promote financial inclusion and maintain societal trust, aligning with standards such as the UNESCO Recommendation on the Ethics of Artificial Intelligence.
AI’s infusion into finance is not a distant prospect—it is the present trajectory. Navigating this landscape responsibly requires a nuanced understanding of technology’s capabilities and limits, a commitment to ethical stewardship, and a strategic vision balancing innovation with systemic resilience. Institutions that master this balance will not only thrive but set the standard for the future of finance.
Strategic Recommendation | Description |
---|---|
Prioritize Explainability and Validation | Implement AI systems with transparent decision-making processes. Regularly validate AI performance against real-world outcomes, especially for agentic AI deployed in high-stakes functions such as credit underwriting and fraud detection. |
Invest in Human-AI Collaboration | Develop workforce skills that complement AI capabilities. Promote hybrid models where AI augments human judgment, preserving trust, accountability, and the “human touch” vital to financial advice. |
Enhance Data Governance and Privacy | Establish robust data management frameworks that ensure compliance with evolving regulations such as the EU AI Act and GDPR. Employ localized data storage and strict access controls to build user confidence and comply with data sovereignty requirements. |
Engage Proactively with Regulators | Collaborate with policymakers to shape balanced regulations that foster innovation while managing systemic and ethical risks. Early alignment reduces operational uncertainty and enhances compliance readiness. |
Mitigate Concentration and Cybersecurity Risks | Diversify AI vendors and build resilient cybersecurity infrastructures incorporating AI-driven defense mechanisms. Prepare for adversarial threats by adopting layered security measures, including multi-factor authentication and behavioral anomaly detection. |
Embed Ethical Frameworks | Address biases and fairness proactively. Implement ethical AI principles that promote financial inclusion and maintain societal trust, aligning with standards such as the UNESCO Recommendation on the Ethics of Artificial Intelligence. |