AI in Drug Discovery: Transforming Pharma with Smarter Medicine
- Introduction: Why AI is a Game-Changer in Drug Discovery
- Introduction: Why AI is a Game-Changer in Drug Discovery
- The Disruptive Potential of Artificial Intelligence
- The Complexity and Inefficiency of Traditional Drug Discovery
- Key AI Technologies Reshaping Pharma
- Framing the Scope: Capabilities, Applications, and Challenges
- What’s Next?
- Foundations of AI in Drug Discovery: Algorithms, Data, and Models
- Foundations of AI in Drug Discovery: Algorithms, Data, and Models
- Core AI Methodologies in Drug Discovery
- Essential Data Types: Chemical Structures to Multi-Omics
- Model Training, Validation, and Interpretability Challenges
- Applications Across the Drug Development Pipeline: From Target Identification to Clinical Trials
- Applications Across the Drug Development Pipeline: From Target Identification to Clinical Trials
- Target Identification and Validation, Hit Discovery, and Lead Optimization
- Toxicity and ADMET Prediction
- Drug Repurposing: Mining Existing Drugs for New Therapies
- Integration with Experimental Methods: Molecular Dynamics and Autonomous Laboratories
- Clinical Trial Design and Monitoring
- Comparative Analysis: AI-Driven Approaches Versus Traditional Drug Discovery
- Comparative Analysis: AI-Driven Approaches Versus Traditional Drug Discovery
- Speed and Cost-Effectiveness: Quantitative Gains with Important Caveats
- Accuracy and Scalability: AI’s Strengths and Persistent Challenges
- When AI Supplements Rather Than Replaces Human Expertise
- Summary: A Balanced Perspective on AI’s Role in Drug Discovery
- Ethical, Regulatory, and Practical Challenges in Deploying AI for Pharma
- Ethical, Regulatory, and Practical Challenges in Deploying AI for Pharma
- Ethical Considerations: Navigating Beyond the Algorithm
- Regulatory Hurdles: Adapting to AI’s Transformative Role
- Practical Challenges: Ensuring Reproducibility, Validation, and Collaboration
- The Path Forward: Balancing Innovation with Responsibility
- Future Directions: Frontier Technologies and Emerging Trends in AI-Driven Drug Discovery
- Future Directions: Frontier Technologies and Emerging Trends in AI-Driven Drug Discovery
- The Rise of Self-Driving Laboratories: Automating Science Itself
- Multi-Omics Data Integration: Towards Holistic Biological Insights
- Generative Models and Reinforcement Learning: Designing Molecules from Scratch
- Explainable AI and Real-Time Adaptive Clinical Trials: Bridging the Gap to the Clinic
- The Power of Partnerships and Computational Advances: Genentech and NVIDIA Leading the Charge
- Navigating the Uncertainties Ahead
- Conclusion: Balancing Optimism and Caution in the AI-Powered Pharma Revolution
- Balancing Optimism and Caution in the AI-Powered Pharma Revolution
- The Transformative Potential and Technical Hurdles
- The Imperative of High-Quality Data and Human Oversight
- Ethical Considerations and Regulatory Alignment
- Looking Ahead: Synergy, Societal Impact, and Responsibility
- Key Takeaways
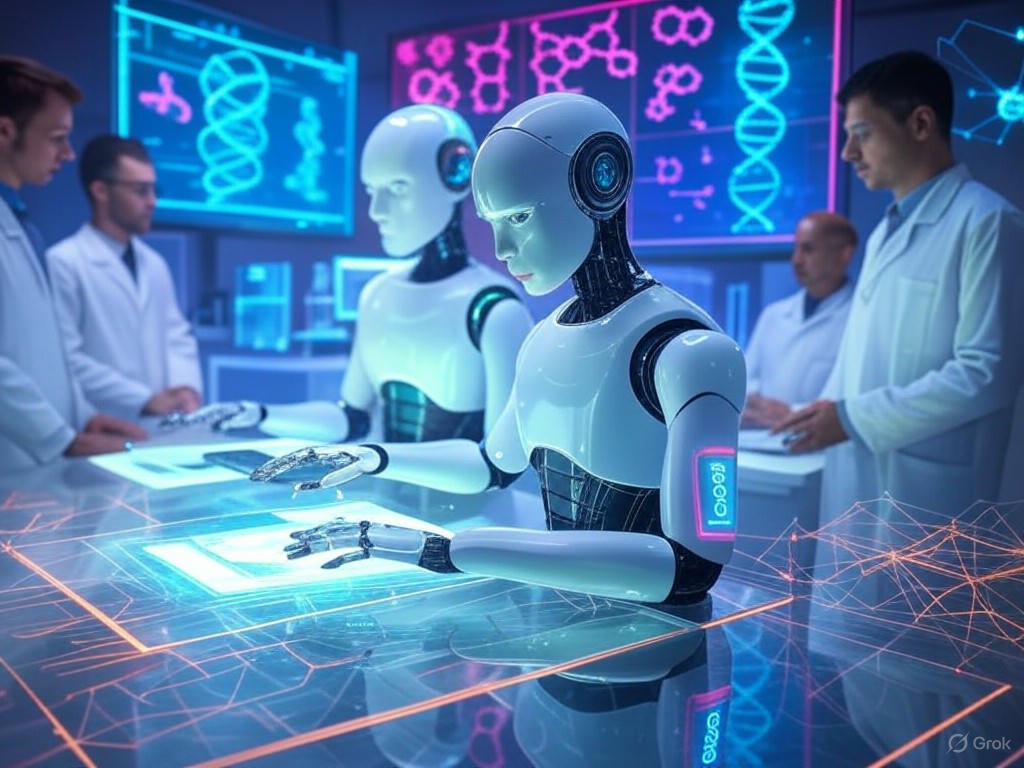
Introduction: Why AI is a Game-Changer in Drug Discovery
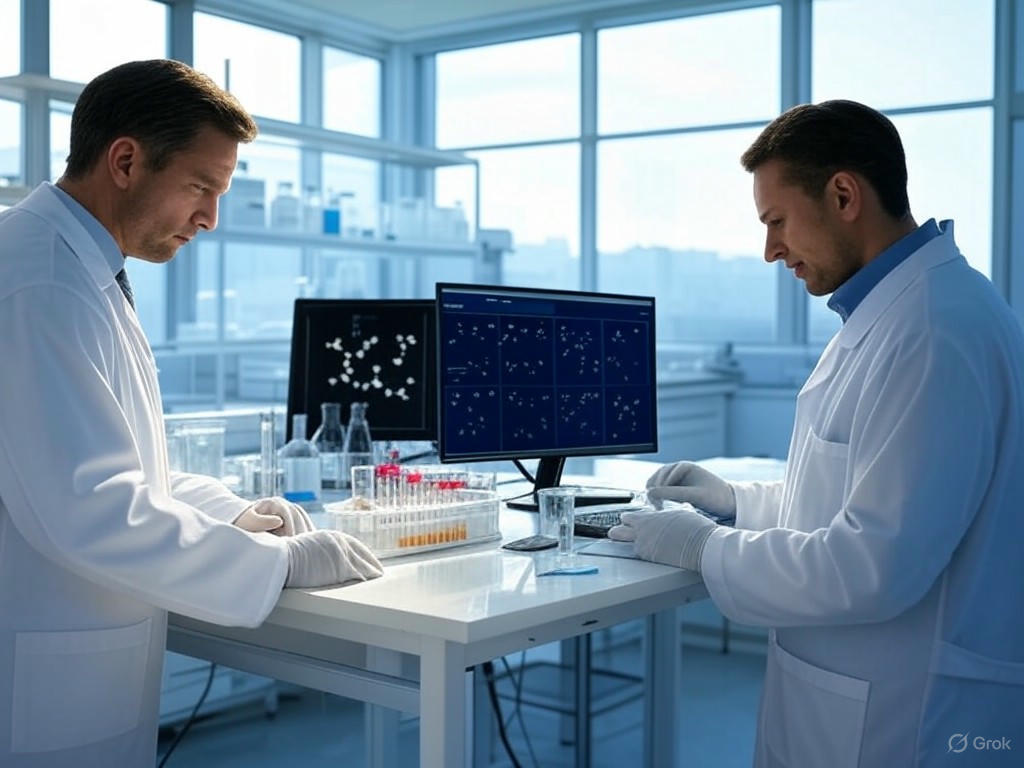
Introduction: Why AI is a Game-Changer in Drug Discovery
How long does it take to bring a new drug to market? Traditionally, this process spans approximately 12 years on average, sometimes extending up to 30 years for advanced therapies such as gene editing. Alongside these lengthy timelines, the costs are staggering. Recent estimates, including Deloitte’s 2024 report, place the average price tag for developing a single drug at over $2 billion, though median costs may be closer to $150 million when accounting for outliers. Despite this massive investment, the success rate remains low—only about 10-12% of drug candidates entering clinical trials ultimately gain FDA approval.
This conventional drug discovery paradigm is fraught with inefficiencies. It involves sequential stages including target discovery, preclinical testing, clinical trials, and regulatory review, each demanding extensive resources and rigorous safety validation. The process is often likened to searching for a needle in a molecular haystack, as even promising candidates frequently fail late in development, resulting in billions of dollars in sunk costs and delayed patient access to potentially life-saving therapies.
The Disruptive Potential of Artificial Intelligence
Artificial intelligence is poised to transform this landscape profoundly. By 2025, AI-driven approaches are projected to contribute to roughly 30% of new drug discoveries—a dramatic shift from the current state. Historically cautious, the pharmaceutical industry is increasingly embracing AI as a catalyst for transformative innovation rather than incremental improvement.
Market forecasts underscore this trend: the AI drug discovery sector is expected to grow from $1.7 billion in 2024 to over $8.5 billion by 2030. This surge is powered by advances in key AI technologies such as machine learning (ML), deep learning, and graph neural networks (GNNs), which collectively enable more efficient and insightful exploration of complex biological data.
The Complexity and Inefficiency of Traditional Drug Discovery
To fully appreciate AI’s disruptive potential, it is essential to understand the inherent challenges of the traditional drug discovery process:
- Lengthy Timelines: Developing a market-ready drug often takes more than a decade, with clinical trials alone (Phases I-III) consuming 6 to 8 years.
- High Attrition Rates: Approximately 88-90% of drug candidates fail during clinical development, despite significant prior investment.
- Escalating Costs: Deloitte’s 2024 estimate places the average development cost at $2.2 billion per asset, driven by inflation and the complexity of novel modalities like biologics.
- Regulatory Hurdles: Stringent safety and efficacy standards, while critical, contribute to prolonged timelines and high failure rates.
- Fragmented Data Sources: Integrating and analyzing heterogeneous datasets—from laboratory assays to real-world clinical evidence—remains a significant bottleneck.
These factors collectively impede not only pharmaceutical profitability but also the timely delivery of new therapies to patients in need.
Key AI Technologies Reshaping Pharma
How does AI address these entrenched challenges? Several AI methodologies have emerged as pivotal in drug discovery:
- Machine Learning (ML): Leveraging large-scale datasets, ML algorithms uncover hidden patterns, accelerating target identification and enabling optimization of candidate molecules with greater precision.
- Deep Learning: Neural network architectures model complex biological interactions, supporting breakthroughs such as protein structure prediction and drug-target interaction analysis.
- Graph Neural Networks (GNNs): By representing molecules as interconnected graphs, GNNs capture subtle structural details essential for predicting drug efficacy and safety more accurately.
Notable examples illustrate these advances. DeepMind’s AlphaFold revolutionized protein folding predictions, delivering atomic-level structural insights that traditionally required years of experimental work. Generative AI platforms like Genie are designing novel molecular structures with unprecedented speed and accuracy, enhancing the quality of candidates entering preclinical studies.
Framing the Scope: Capabilities, Applications, and Challenges
AI’s impact extends across the entire drug development pipeline:
- Practical Applications: From virtual screening of millions of compounds to predictive modeling of clinical trial outcomes, AI optimizes discovery and development stages. Digital twins and intelligent automation streamline manufacturing and supply chain processes.
- Clinical Trial Innovation: AI-powered platforms can reduce control arm sizes in Phase III trials, increasing patient access to experimental treatments and accelerating recruitment timelines.
- Operational Efficiency: AI enhances scheduling, logistics, and resource allocation by managing complex interdependencies with high precision.
However, integration of AI into pharma is not without hurdles:
- Data Privacy and Trust: Protecting sensitive patient data remains paramount, with privacy concerns slowing adoption.
- Cultural and Regulatory Barriers: Conservative regulatory environments and institutional inertia challenge rapid AI integration.
- Model Transparency: The black-box nature of many AI models complicates interpretability, hindering regulatory acceptance and clinical trust.
- Bias and Ethical Considerations: AI systems trained on biased datasets risk perpetuating healthcare disparities, necessitating rigorous oversight.
Despite these challenges, optimism is strong. Surveys indicate nearly 80% of pharmaceutical executives anticipate intelligent automation will significantly influence their operations within five years.
What’s Next?
This article will explore how AI’s technical capabilities translate into tangible advances in drug discovery. We will examine real-world applications reshaping pharmaceutical pipelines, analyze persistent challenges, and discuss the broader societal implications of this technological revolution.
The stakes are high. AI promises more than faster or cheaper drug development—it offers the potential to fundamentally redefine our understanding of disease and the delivery of therapies. By enabling more precise, inclusive, and efficient drug discovery, AI stands to make healthcare more accessible and life-saving. Yet, realizing this promise requires a balanced approach that embraces innovation while maintaining ethical vigilance and regulatory compliance.
Aspect | Traditional Drug Discovery | AI-Driven Drug Discovery |
---|---|---|
Timeline | Average 12 years, up to 30 years for advanced therapies | Projected to accelerate discovery, contributing to ~30% of new drugs by 2025 |
Cost | Over $2 billion average, median ~$150 million (Deloitte 2024) | Potential reduction through efficiency and predictive modeling |
Success Rate | 10-12% of candidates entering clinical trials gain FDA approval | Improved candidate selection and optimization via ML and GNNs |
Key Challenges | Lengthy timelines, high attrition, escalating costs, regulatory hurdles, fragmented data | Data privacy, regulatory acceptance, model transparency, bias and ethics |
Core Technologies | Sequential experimental processes and manual analysis | Machine Learning, Deep Learning, Graph Neural Networks, Generative AI |
Applications | Target discovery, preclinical testing, clinical trials, regulatory review | Virtual screening, protein structure prediction, clinical trial optimization, digital twins |
Market Forecast | Traditional pharma market | AI drug discovery market: $1.7B in 2024 to $8.5B+ by 2030 |
Operational Impact | Resource-intensive, manual scheduling and logistics | Enhanced scheduling, logistics, resource allocation, intelligent automation |
Foundations of AI in Drug Discovery: Algorithms, Data, and Models
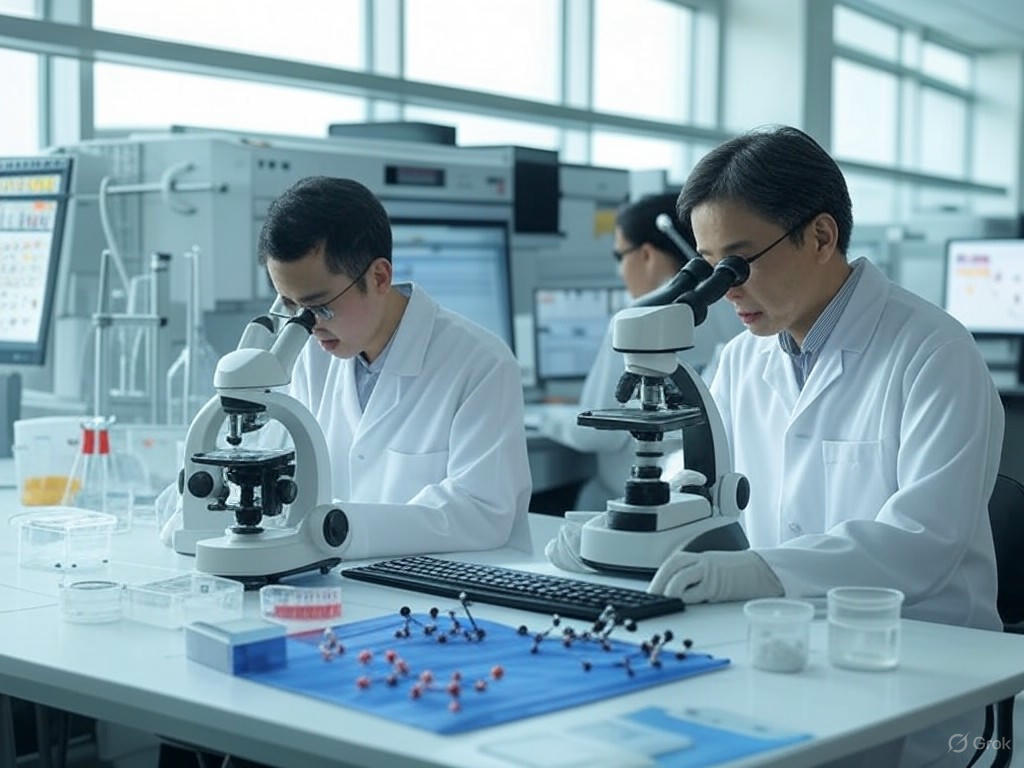
Foundations of AI in Drug Discovery: Algorithms, Data, and Models
What powers AI’s transformative role in drug discovery? It is a sophisticated interplay of advanced algorithms, diverse and high-quality data types, and complex modeling techniques. Together, these components are reshaping how new medications are identified, optimized, and validated, accelerating a process that traditionally spans over a decade.
Core AI Methodologies in Drug Discovery
AI-driven drug discovery leverages multiple machine learning paradigms, each tailored for specific challenges within the drug development pipeline:
-
Supervised Learning
The backbone of predictive modeling in drug discovery, supervised learning uses labeled datasets to forecast molecular properties, binding affinities, or bioactivity. For example, models trained on known protein-ligand interactions can predict how strongly a candidate compound might bind to its target, expediting lead identification and prioritization. -
Unsupervised Learning
This paradigm uncovers hidden structures in unlabeled data, enabling clustering of similar chemical scaffolds or biological response profiles. Such exploratory analyses can reveal novel drug classes or mechanistic insights, supporting hypothesis generation without explicit guidance. -
Reinforcement Learning (RL)
RL is particularly promising for molecular design, where algorithms like MOLRL and ACARL iteratively optimize molecules within defined chemical spaces. ACARL’s incorporation of “activity cliffs”—instances where minor structural changes cause large shifts in biological activity—demonstrates how domain knowledge enhances RL’s efficiency in de novo drug discovery. -
Deep Learning Architectures
Neural networks, especially graph neural networks (GNNs), model molecules as graphs with atoms as nodes and bonds as edges, preserving intricate structural information essential for accurate predictions. Models like PIGNet, a physics-informed deep learning framework, predict binding free energies with remarkable accuracy, achieving an 87% top-1 docking success rate and a screening enrichment factor of 19.6, outperforming many traditional docking methods. -
Graph-Based Models
These represent a paradigm shift by operating directly on molecular graphs instead of relying on simplified fingerprints or sequences. For instance, GraphBAN accelerates preclinical drug candidate discovery by inductively learning from graph-structured data, improving both speed and cost-efficiency in early-stage development.
Together, these methodologies form a robust, multi-modal toolkit that addresses the complexity of biological systems holistically—an essential approach given the intricate interplay of molecular, cellular, and clinical factors influencing drug efficacy and safety.
Essential Data Types: Chemical Structures to Multi-Omics
AI models in drug discovery are only as effective as the data they are trained on. Key data types include:
-
Chemical Structures
Represented commonly as SMILES (Simplified Molecular Input Line Entry System) strings or directly as molecular graphs. SMILES provide a compact textual format linearizing molecular graphs, while graph representations inherently retain molecular topology and bonding, enabling more nuanced modeling. Tools like RDKit facilitate conversions between formats and generate 3D conformations critical for structure-based predictions. -
Biological Assays
Experimental measurements of compound activity, phenotypic responses, or toxicity profiles provide labeled datasets essential for supervised learning and model validation. -
Omics Data
Genomics, transcriptomics, proteomics, and metabolomics data deliver a systems-level view of biological states and disease mechanisms. Integrating multi-omics datasets helps AI capture complex disease biology, identify novel targets, and predict drug responses with greater accuracy. For example, phenotypic assays combined with multi-omics analyses reveal cellular responses without prior assumptions about targets, enriching early-stage discovery.
Ensuring data quality, curation, and representation is critical. Poorly curated or incompatible datasets can introduce bias, reduce model generalizability, and lead to misleading predictions. Data curation involves systematic organization, error correction, and standardization to maintain accuracy and facilitate accessibility—a foundational step often underestimated in AI workflows.
Emerging representation techniques, such as embedded one-hot encoding (eOHE), reduce computational overhead while preserving data integrity, enabling scalable and energy-efficient deep learning applications.
Model Training, Validation, and Interpretability Challenges
Building AI models for drug discovery involves meticulous training, validation, and interpretability efforts:
-
Model Training and Performance
Models like PIGNet integrate physics-based insights with deep learning to predict binding free energies, delivering substantial improvements in docking success and screening enrichment. Achieving such performance requires careful integration of domain expertise into learning architectures. -
Validation Hurdles
Although AI accelerates early discovery timelines dramatically—from traditional 2.5–4 years to as little as 9–18 months—it remains to be seen how consistently these gains translate into clinical success. Rigorous validation protocols, including cross-validation, external test sets, and retrospective analyses, are essential to build confidence among researchers and regulators. -
Interpretability and Explainability
Many powerful AI models, such as CGMega—a graph-based system—function as “black boxes,” limiting transparency. Explainable AI (XAI) approaches like the eXplainable Graph-based Drug response Prediction (XGDP) method seek to elucidate underlying drug-target interaction mechanisms, offering actionable insights rather than opaque scores. -
Regulatory Considerations
Regulatory bodies are evolving frameworks to address AI-specific challenges. The FDA’s January 2025 draft guidance on AI use in drug and biological product regulatory decision-making emphasizes transparency, risk assessment, and validation standards. These efforts underscore that interpretability and robustness are becoming non-negotiable prerequisites for AI-driven therapeutics.
By unpacking AI’s core methodologies, critical data foundations, and complex validation landscapes, it is clear that AI is not a silver bullet but a sophisticated enabler in drug discovery. Success depends on algorithmic innovation, meticulous data stewardship, and a steadfast commitment to explainability—where cutting-edge technology intersects with scientific rigor and ethical responsibility.
AI Methodology | Description | Examples / Notes |
---|---|---|
Supervised Learning | Uses labeled datasets to predict molecular properties, binding affinities, or bioactivity. | Models trained on protein-ligand interactions for lead identification and prioritization. |
Unsupervised Learning | Finds hidden structures in unlabeled data, enabling clustering and hypothesis generation. | Reveals novel drug classes and mechanistic insights. |
Reinforcement Learning (RL) | Optimizes molecules iteratively within chemical spaces using domain knowledge like activity cliffs. | Algorithms like MOLRL and ACARL enhance de novo drug design efficiency. |
Deep Learning Architectures | Neural networks, especially graph neural networks, model molecules preserving structural information. | PIGNet predicts binding free energies with 87% docking success and 19.6 enrichment factor. |
Graph-Based Models | Operate directly on molecular graphs to improve speed and cost-efficiency in drug candidate discovery. | GraphBAN accelerates early-stage development by learning from graph-structured data. |
Data Type | Description | Examples / Tools |
---|---|---|
Chemical Structures | Represented as SMILES strings or molecular graphs retaining topology and bonding. | RDKit for format conversion and 3D conformation generation. |
Biological Assays | Experimental data measuring compound activity, toxicity, or phenotypic responses. | Provide labeled datasets essential for supervised learning and validation. |
Omics Data | Genomics, transcriptomics, proteomics, and metabolomics delivering systems-level biological views. | Integration helps identify targets and predict drug responses accurately. |
Data Representation Techniques | Methods like embedded one-hot encoding reduce computational overhead while preserving data integrity. | Enable scalable and energy-efficient deep learning applications. |
Aspect | Details | Examples / Notes |
---|---|---|
Model Training and Performance | Integration of physics-based insights with deep learning improves prediction accuracy. | PIGNet achieves high docking success and screening enrichment. |
Validation Hurdles | Rigorous protocols needed to confirm clinical translation of AI-accelerated discovery timelines. | Includes cross-validation, external tests, and retrospective analyses. |
Interpretability and Explainability | Many AI models act as black boxes; XAI methods aim to provide actionable insights. | XGDP elucidates drug-target interactions for better understanding. |
Regulatory Considerations | Emerging FDA guidance emphasizes transparency, risk assessment, and validation standards. | Draft guidance effective January 2025 targets AI in drug regulatory decision-making. |
Applications Across the Drug Development Pipeline: From Target Identification to Clinical Trials
Applications Across the Drug Development Pipeline: From Target Identification to Clinical Trials
How exactly is AI reshaping the painstaking journey from a biological target in the lab to a drug that reaches patients? The transformation is profound yet nuanced—it’s not magic, but a sophisticated orchestration of data, algorithms, and experimental science that is redefining each stage of drug development.
Target Identification and Validation, Hit Discovery, and Lead Optimization
Traditionally, identifying and validating drug targets demanded years of laborious wet-lab experimentation with a high failure rate. AI platforms now accelerate this phase by integrating vast, multimodal datasets—including molecular, phenotypic, and clinical data—into holistic models of disease biology. For instance, Insilico Medicine’s Pharma.AI system leverages policy-gradient reinforcement learning combined with deep generative recurrent neural networks to navigate biological complexity and propose novel therapeutic targets. This marks a shift from reductionist methods toward “Holistic Drug Development,” an approach that aims to understand disease mechanisms within their full systemic context rather than isolated components.
Following target identification, AI-driven virtual screening algorithms rapidly evaluate millions of compounds against these targets, dramatically speeding up hit discovery. Unlike legacy computational methods, modern AI employs deep learning ensembles that capture subtle molecular interactions, enhancing the likelihood of identifying promising hits. Exscientia exemplifies this approach by designing structurally novel molecules that have advanced into Phase 1 clinical trials—a milestone underscoring AI’s tangible impact in pharmaceutical R&D.
Subsequent lead optimization benefits from AI models predicting how molecular modifications influence efficacy and pharmacokinetics. This iterative cycle of design, prediction, and experimental validation is increasingly supported by integrated AI platforms connecting computational insights with laboratory results. Such integration has compressed the typical 2.5 to 4-year discovery timeline down to as little as 9 to 18 months in some cases. However, accelerated discovery does not guarantee clinical success; translating computational promise into human efficacy remains a critical challenge.
Toxicity and ADMET Prediction
Late-stage failure due to toxicity or unfavorable pharmacokinetics remains one of the most costly bottlenecks in drug development. AI addresses this by deploying machine learning models to predict drug-induced toxicities—such as cardiotoxicity and hepatotoxicity—well before clinical trials commence. Stanford’s cardiovascular AI models, for example, can forecast adverse cardiac events like arrhythmias, enabling early elimination of harmful candidates.
Similarly, AI-powered ADMET prediction platforms—including Aurigene.AI and ADMET Predictor—offer comprehensive in silico evaluations of absorption, distribution, metabolism, excretion, and toxicity properties. This preclinical insight prioritizes safer and more efficacious compounds, reducing attrition rates and aligning with regulatory trends to minimize animal testing. Despite these advances, toxicity mechanisms’ complexity means no single AI model suffices; robust comparative analyses and expert interpretation remain essential for responsible decision-making.
Drug Repurposing: Mining Existing Drugs for New Therapies
Could a drug shelved decades ago hold the key to treating today’s diseases? AI proves invaluable in drug repurposing by mining extensive pharmacopeias and clinical datasets to uncover unexpected therapeutic potentials. Machine learning models analyze drug-target interaction profiles alongside side effect data to identify novel indications. The life-saving story of Joseph Coates, who benefited from an AI-discovered repurposed treatment, exemplifies this practical impact.
Conferences such as iDR25 highlight that AI-driven repurposing is not merely computational prowess but also involves navigating ethical considerations around patient data privacy and equitable access. While repurposing offers a faster, lower-cost route to new therapies, rigorous clinical validation remains indispensable. AI thus serves as a powerful complement to expert judgment rather than a replacement.
Integration with Experimental Methods: Molecular Dynamics and Autonomous Laboratories
AI’s predictive power gains additional depth when integrated with experimental techniques. Molecular dynamics simulations enhanced by AI provide detailed temporal insights into molecular behavior, informing more accurate drug design. This synergy enables researchers to predict not only static binding affinities but also dynamic interactions within complex biological environments.
Beyond simulation, AI-powered autonomous laboratories—or “self-driving labs”—are revolutionizing experimental workflows. These labs automate high-throughput tasks and iteratively refine hypotheses based on real-time data, freeing scientists to focus on strategic decisions. Platforms like Scibot and Coscientist showcase how AI agents autonomously conduct chemistry experiments, accelerating research cycles and reducing human error. Experts like Andrew Bostrom anticipate such self-driving labs becoming widely adopted within 5 to 10 years, transforming the experimental landscape.
Clinical Trial Design and Monitoring
AI’s role extends into clinical development, where efficient trial design and patient monitoring are critical. By analyzing patient-centric data, AI assists in selecting ideal trial participants, predicting outcomes, and detecting adverse events earlier. Decentralized clinical trials, bolstered by AI analytics, are gaining traction, enabling more inclusive and adaptive study designs.
Exscientia’s end-to-end integration exemplifies AI’s potential—from generative molecular design through experimental validation to clinical development—aiming to “change the underlying economics of drug discovery” by reducing timeline and cost. AI tools like TrialGPT further accelerate clinical trial processes by reducing screening times by over 40%, highlighting AI’s capacity to streamline regulatory pathways.
The integration of AI across the drug development pipeline is not about replacing scientists but augmenting their capabilities. It creates a dynamic feedback loop where computational insights and experimental evidence continuously refine each other. While challenges remain—particularly in aligning accelerated discovery with clinical success—the emerging paradigm promises to reshape pharmaceutical innovation, potentially delivering safer, more effective medicines at an unprecedented pace.
Drug Development Stage | AI Applications | Examples / Platforms | Impact |
---|---|---|---|
Target Identification and Validation | Integrating multimodal datasets for holistic disease models; reinforcement learning and generative neural networks to propose novel targets | Insilico Medicine’s Pharma.AI | Accelerates target discovery; shifts from reductionist to holistic approaches |
Hit Discovery | AI-driven virtual screening using deep learning ensembles to evaluate millions of compounds | Exscientia | Speeds hit identification; enables structurally novel molecules entering clinical trials |
Lead Optimization | Predicting molecular modifications on efficacy and pharmacokinetics; integrating computational and experimental data | Integrated AI platforms | Reduces discovery timeline from years to months; improves molecular design iteration |
Toxicity and ADMET Prediction | Machine learning models to predict toxicities and pharmacokinetics; in silico ADMET evaluation | Stanford cardiovascular AI models, Aurigene.AI, ADMET Predictor | Reduces late-stage failures; supports safer compound prioritization; helps minimize animal testing |
Drug Repurposing | Mining clinical and pharmacopeia data to identify new indications; analyzing drug-target and side effect profiles | AI-driven models highlighted at iDR25 conference | Faster, lower-cost route to therapies; complements expert clinical validation |
Integration with Experimental Methods | AI-enhanced molecular dynamics simulations; autonomous laboratories automating experiments and hypothesis testing | Platforms like Scibot, Coscientist | Improves dynamic interaction understanding; accelerates research cycles; reduces human error |
Clinical Trial Design and Monitoring | AI-assisted patient selection, outcome prediction, adverse event detection; decentralized trials; clinical trial process acceleration | Exscientia’s end-to-end integration, TrialGPT | Improves trial efficiency and inclusivity; reduces screening times by over 40% |
Comparative Analysis: AI-Driven Approaches Versus Traditional Drug Discovery
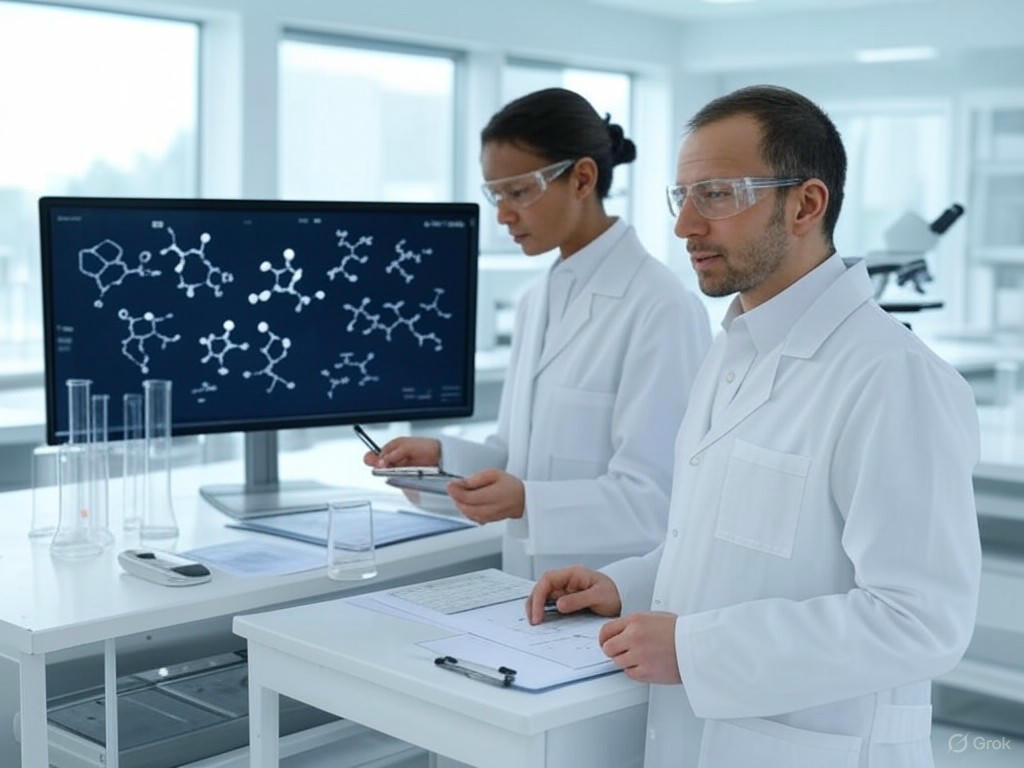
Comparative Analysis: AI-Driven Approaches Versus Traditional Drug Discovery
How do AI-driven drug discovery methods truly compare to the longstanding traditional processes? The promise of dramatically shortening timelines and reducing costs has generated considerable excitement. Yet, a careful, evidence-based examination reveals a more nuanced reality.
Speed and Cost-Effectiveness: Quantitative Gains with Important Caveats
Traditional drug discovery is infamously slow and costly. Bringing a new drug to market often takes around 12 years, sometimes stretching to 30 years for advanced therapies, with costs averaging over $2 billion per asset (Deloitte estimates $2.2 billion in 2024). Moreover, clinical trial attrition rates are high, with only about 10-12% of candidates ultimately approved by regulatory agencies.
In contrast, AI-driven approaches have compressed the early discovery phase—from the typical 2.5 to 4 years (30–50 months)—to as little as 9 to 18 months in some instances (BiopharmaTrend 2024). For example, Insilico Medicine reported that a drug candidate for idiopathic pulmonary fibrosis moved from concept to Phase I trials within 30 months, showcasing a remarkable acceleration.
Cost savings are equally promising. McKinsey estimates AI adoption can reduce drug development costs by 30–50%, primarily by automating labor-intensive tasks such as data handling, target identification, and validation workflows.
However, these figures warrant cautious interpretation. While AI accelerates early-stage discovery, these speed gains have yet to fully translate into improved clinical success rates. Promising AI-identified candidates still face the high failure and discontinuation rates typical of drug development pipelines. Thus, faster discovery does not guarantee quicker market approval.
Accuracy and Scalability: AI’s Strengths and Persistent Challenges
AI excels at analyzing vast and complex datasets—molecular structures, phenotypic profiles, multi-omics data, and clinical records—to detect patterns beyond human capacity. Breakthroughs such as DeepMind’s AlphaFold have revolutionized protein folding predictions, while generative AI platforms like Genie innovate molecular design with enhanced precision and speed.
AI’s scalability is a critical advantage. Platforms like Recursion OS integrate multimodal data to map biological and chemical relationships at a scale unattainable by manual methods, enabling what is termed Holistic Drug Development (HDD). This capability facilitates simultaneous evaluation of trillions of molecular interactions, accelerating hypothesis generation and candidate prioritization.
Yet, AI accuracy remains tightly linked to the quality, diversity, and volume of training data. Data scarcity, especially in rare diseases or novel therapeutic areas, limits model generalizability. Overfitting—where models perform well on training data but poorly on new data—is a persistent issue. Biological complexity and heterogeneous datasets exacerbate reproducibility challenges, as highlighted in studies from PMC 2024 and Springer AI Review 2024.
Moreover, subtle non-deterministic factors in AI pipelines—random seed initialization, hardware differences, and data preprocessing variations—complicate reproducibility. Without rigorous standardization and validation protocols, AI-derived insights risk irreproducibility, undermining confidence among researchers and regulators.
When AI Supplements Rather Than Replaces Human Expertise
Despite AI’s impressive capabilities, the idea that therapeutics can be developed “at the push of a button” is a misconception (BiopharmaTrend 2024). Instead, the emerging reality is a hybrid ecosystem where AI augments human expertise rather than replaces it.
Pharmaceutical companies such as Astellas employ “Human-in-the-Loop” frameworks that integrate AI, robotics, and domain experts. In these models, chemists, biologists, and clinicians critically assess AI-generated hypotheses, validate predictions through experiments, and iteratively refine models.
This collaborative approach grounds AI insights in biological plausibility and clinical relevance. For example, while AI may propose novel molecular structures, medicinal chemists evaluate synthetic feasibility and safety profiles. Similarly, AI can optimize clinical trial designs, but human oversight remains essential to navigate ethical and regulatory considerations.
Furthermore, AI-driven drug discovery is evolving toward comprehensive integration of molecular, phenotypic, and clinical data streams—demanding continuous human input for data curation, validation, and interpretation.
Summary: A Balanced Perspective on AI’s Role in Drug Discovery
- Speed: AI shortens early discovery phases from years to months, accelerating candidate identification.
- Cost: Automation and predictive analytics contribute to 30–50% cost reductions by streamlining labor-intensive tasks.
- Accuracy: AI improves candidate selection but depends heavily on high-quality, diverse datasets and faces challenges such as overfitting and reproducibility.
- Scalability: AI’s capacity to analyze massive datasets enables exploration of complex biological systems at unprecedented scale.
- Limitations: Data scarcity, model overfitting, and non-deterministic training factors limit AI’s standalone efficacy.
- Human-AI Synergy: The most effective workflows combine AI’s computational power with human scientific expertise in iterative, collaborative cycles.
In essence, AI is not a magic wand but a transformative tool reshaping drug discovery. Its greatest value lies in complementing human insight and enhancing workflows—not replacing the foundational need for rigorous experimentation and clinical validation. As the pharmaceutical sector approaches 2025 and beyond, embracing this hybrid paradigm will be critical to realizing AI’s full potential in delivering safer, more effective medicines faster and more affordably.
Aspect | Traditional Drug Discovery | AI-Driven Drug Discovery |
---|---|---|
Speed | Typically 12 years (up to 30 years for advanced therapies); early discovery 2.5 to 4 years | Early discovery phase reduced to 9 to 18 months; example: 30 months from concept to Phase I trial |
Cost | Over $2 billion per asset (Deloitte estimate $2.2B in 2024) | 30–50% cost reduction by automating labor-intensive tasks (McKinsey estimate) |
Clinical Success Rate | Approval rate ~10-12% of candidates | High failure rates persist; AI accelerates early stages but does not guarantee quicker market approval |
Data Handling & Analysis | Manual analysis; limited scalability | Excels at analyzing vast, complex datasets; enables holistic drug development via multimodal data integration |
Accuracy | Dependent on experimental and clinical validation | Improved candidate selection but dependent on data quality; challenges include overfitting and reproducibility issues |
Scalability | Limited by manual methods | Can evaluate trillions of molecular interactions simultaneously; scalable computational platforms |
Reproducibility | Established experimental protocols ensure reproducibility | Complicated by non-deterministic factors and data variability; requires rigorous standardization and validation |
Human Involvement | Central role of chemists, biologists, clinicians in all phases | Hybrid model; AI augments human expertise with iterative validation and refinement |
Limitations | Time-consuming, costly, high attrition | Data scarcity in rare diseases, overfitting, reproducibility challenges, not a standalone solution |
Ethical, Regulatory, and Practical Challenges in Deploying AI for Pharma
Ethical, Regulatory, and Practical Challenges in Deploying AI for Pharma
What happens when cutting-edge AI technologies intersect with the high-stakes, tightly regulated world of drug discovery? The potential to develop faster, smarter, and more effective medications is tremendous. Yet, alongside this promise lie significant ethical, regulatory, and practical challenges that pharmaceutical companies must navigate to unlock AI’s full benefits responsibly.
Ethical Considerations: Navigating Beyond the Algorithm
Implementing AI in drug discovery is far more than a technical endeavor—it raises profound ethical questions. The foremost concern is data privacy. The drug development process involves vast datasets, often containing sensitive patient information. Adrian Stevens from Chemaxon observes that the industry is moving away from a “wild west” phase toward instituting central corporate guardrails that safeguard privacy and ensure regulatory compliance. Without robust privacy protections, patient trust can erode, and regulatory approval becomes jeopardized.
Another critical issue is algorithmic bias. AI models trained on incomplete or non-representative data risk perpetuating existing healthcare disparities. For instance, biases observed in healthcare AI—such as diagnostic tools underperforming on darker skin tones—serve as cautionary examples applicable to drug discovery AI models. Addressing bias demands rigorous auditing, inclusion of diverse datasets, and collaboration among multidisciplinary teams comprising ethicists, data scientists, and clinicians.
Transparency and explainability are equally vital. Many AI models, particularly deep learning architectures, function as “black boxes,” making their decision-making opaque. Pharmaceutical companies face growing pressure to produce interpretable AI outputs that satisfy regulators and clinicians alike. Emerging accountability frameworks seek to clarify responsibility when AI-driven decisions result in adverse outcomes—an inherently complex challenge given AI’s probabilistic nature.
Regulatory Hurdles: Adapting to AI’s Transformative Role
Regulatory bodies have adopted a cautious yet proactive stance toward AI’s integration in drug development. A landmark step was the FDA’s January 2025 draft guidance titled Considerations for the Use of Artificial Intelligence To Support Regulatory Decision-Making for Drug and Biological Products. This guidance introduces a risk-based framework emphasizing the “context of use” (COU) to determine the appropriate level of regulatory scrutiny.
Key elements of this framework include:
- Encouraging early engagement with regulators to clarify expectations and align development plans.
- Implementing a seven-step credibility assessment process that spans from defining AI’s intended role to executing thorough validation protocols.
- Mandating rigorous validation and verification of AI tools, especially those influencing clinical trial analyses.
Notably, as of mid-2025, no AI-discovered drug has yet received FDA approval, underscoring the regulatory environment’s cautious approach. Both the FDA and EMA continue refining guidelines to balance innovation with safety and efficacy, recognizing AI as a transformative but still maturing technology.
Beyond drug discovery, advanced manufacturing technologies (AMTs) powered by AI are prompting regulatory updates focused on ensuring product quality and supply chain resilience. Pharma companies adopting AI-enabled manufacturing must navigate evolving compliance requirements that address these novel processes.
Practical Challenges: Ensuring Reproducibility, Validation, and Collaboration
While AI promises accelerated drug discovery, real-world implementation encounters significant reproducibility challenges. Models that perform well in controlled research settings may not generalize effectively across heterogeneous datasets or diverse clinical environments. This discrepancy raises risks of false positives—pursuing ineffective compounds—and false negatives—overlooking promising candidates.
Robust validation standards remain nascent but critical. The FDA’s draft guidance provides an initial roadmap, yet pharmaceutical companies face steep learning curves in building and maintaining AI models credible enough for regulatory acceptance.
Addressing these complexities requires multidisciplinary collaboration. AI specialists, clinicians, ethicists, regulatory experts, and data scientists must jointly design, validate, and govern AI systems. This cross-functional teamwork ensures that multiple perspectives inform AI development, reducing risks and enhancing innovation.
Pharmaceutical organizations increasingly adopt governance frameworks that embed transparency, accountability, and ethical principles from project inception. Models such as the Minimum Viable Governance (MVG) approach offer structured mechanisms to maintain oversight and control over AI initiatives without hindering creativity or speed.
The Path Forward: Balancing Innovation with Responsibility
Integrating AI into drug discovery is not simply a technological upgrade; it represents a paradigm shift that challenges established ethical norms, regulatory frameworks, and operational practices. Success in this arena hinges on companies that:
- Prioritize data privacy and ethical AI, embedding fairness, transparency, and patient-centric safeguards.
- Engage proactively with regulators, aligning AI development with emerging guidelines and risk-based frameworks.
- Invest in multidisciplinary teams and governance structures that ensure AI models are reliable, reproducible, and clinically relevant.
This journey is an intricate balancing act. AI’s transformative potential is undeniable, yet without deliberate oversight and ethical vigilance, missteps could delay patient access to life-saving therapies or erode public confidence.
Drawing on over 15 years of experience architecting AI systems, it is clear that enthusiasm alone cannot drive success in pharma. What is required is rigor, humility, and collaborative governance. The path to responsible, AI-driven drug discovery is underway—and its outcome will profoundly shape the future of medicine.
Category | Challenges | Details & Considerations |
---|---|---|
Ethical Considerations | Data Privacy | Handling sensitive patient information with central corporate guardrails to ensure privacy and regulatory compliance. |
Ethical Considerations | Algorithmic Bias | Risks from non-representative data causing healthcare disparities; requires auditing, diverse datasets, and multidisciplinary collaboration. |
Ethical Considerations | Transparency and Explainability | AI models often act as “black boxes”; need for interpretable outputs and accountability frameworks for adverse outcomes. |
Regulatory Hurdles | Risk-Based Framework | FDA guidance emphasizes “context of use” with early regulator engagement and a seven-step credibility assessment process. |
Regulatory Hurdles | Validation and Verification | Mandatory rigorous validation of AI tools, especially for clinical trial analysis; no AI-discovered drug approved as of mid-2025. |
Regulatory Hurdles | Advanced Manufacturing Technologies | AI-powered manufacturing requires compliance with evolving regulations ensuring product quality and supply chain resilience. |
Practical Challenges | Reproducibility | Models may not generalize well beyond controlled settings, risking false positives and false negatives. |
Practical Challenges | Validation Standards | Standards are nascent; companies face steep learning curves to meet regulatory acceptance. |
Practical Challenges | Multidisciplinary Collaboration | Requires joint design and governance by AI experts, clinicians, ethicists, and regulatory specialists. |
Practical Challenges | Governance Frameworks | Adoption of models like Minimum Viable Governance (MVG) to maintain oversight without hindering innovation. |
Future Directions: Frontier Technologies and Emerging Trends in AI-Driven Drug Discovery
Future Directions: Frontier Technologies and Emerging Trends in AI-Driven Drug Discovery
What lies on the horizon of AI-driven drug discovery when cutting-edge technologies like self-driving laboratories, multi-omics data integration, generative models combined with reinforcement learning, and explainable AI frameworks converge? The answer reveals a transformative yet intricate landscape rapidly unfolding, accompanied by significant challenges and open questions.
The Rise of Self-Driving Laboratories: Automating Science Itself
Imagine laboratories where robots and AI systems autonomously design, conduct, and analyze experiments continuously. Self-driving laboratories are transitioning from science fiction to reality, poised to revolutionize pharmaceutical R&D within the next 5 to 10 years.
These autonomous labs employ AI agents such as Scibot, Coscientist, and DOLPHIN to handle routine, well-structured tasks like high-throughput screening and media optimization. This automation frees human scientists to focus on creative problem-solving and strategic decision-making.
For instance, the Quantos robot delivers precise weighing and dosing of organic compounds, achieving consistency beyond manual capabilities. Research teams at Harvard and Seoul National University are pioneering swarm robots that leverage linked particle technology to accelerate biological discovery.
While promising dramatic reductions in drug discovery timelines, self-driving labs remain in development. Life science organizations must address challenges related to reliability, ease of use, and seamless integration with existing workflows. As Andrew Bostrom insightfully notes, “self-driving labs will manage routine, well-structured tasks,” suggesting a phased adoption starting with incremental automation rather than full replacement.
Multi-Omics Data Integration: Towards Holistic Biological Insights
Next-generation drug discovery increasingly relies on systems-level biological understanding. Integrating genomics, transcriptomics, proteomics, and metabolomics—collectively termed multi-omics—provides a comprehensive, 360-degree view of disease mechanisms from onset to progression.
Powered by advanced machine learning, multi-omics integration uncovers insights unattainable through reductionist methods. Companies like Freenome and Tempus are constructing extensive, standardized clinical data ecosystems to fuel predictive analytics, enabling precise target selection and patient stratification.
This approach is especially critical for complex modalities such as cell and gene therapies, where molecular interactions intricately influence therapeutic outcomes.
However, multi-omics integration introduces substantial challenges:
- Standardizing methodologies and developing interoperable protocols remain pressing needs.
- Ethical considerations around data privacy and bias require transparent AI frameworks.
- Modular solutions must fit seamlessly into existing R&D pipelines.
The promise is clear: multi-omics research is set to advance personalized medicine beyond static biomarkers toward dynamic, actionable profiles. Realizing this potential demands cross-disciplinary collaboration and rigorous governance.
Generative Models and Reinforcement Learning: Designing Molecules from Scratch
Could AI soon design novel drug molecules at the push of a button? While not quite at that stage, generative models combined with reinforcement learning (RL) are rapidly pushing the boundaries of de novo drug design.
These algorithms explore vast chemical spaces, proposing and optimizing candidate molecules for desired bioactivity and synthetic accessibility.
Insilico Medicine’s Pharma.AI platform exemplifies this innovation, applying policy-gradient RL with deep generative recurrent neural networks to design inhibitors targeting idiopathic pulmonary fibrosis. This has compressed discovery phases from the traditional 40–50 months down to 9–18 months.
Similarly, Recursion OS integrates multimodal data to map trillions of biological and chemical relationships, capturing complex phenotypic responses alongside patient data. Such platforms exemplify a paradigm shift from legacy computational tools toward holistic drug development frameworks, sometimes referred to as “Holistic Drug Development (HDD).”
Despite accelerated discovery timelines, translating AI-generated molecules into approved drugs remains challenging. Some AI-identified candidates have faced clinical trial failures or strategic discontinuations, underscoring the need for rigorous validation and cautious optimism.
Explainable AI and Real-Time Adaptive Clinical Trials: Bridging the Gap to the Clinic
A critical challenge in applying AI to drug development is overcoming the “black box” problem—opaque decision-making processes that hinder clinical and regulatory trust.
Explainable AI (XAI) frameworks are emerging to provide transparent, interpretable AI-driven decisions, a prerequisite for regulatory acceptance and clinician confidence.
In clinical trials, AI-enabled tools are transforming trial design, patient recruitment, monitoring, and data analysis. For example, TrialGPT reduces screening times by over 40%, while predictive models identify adverse event risks earlier, enabling proactive interventions.
More revolutionary are real-time adaptive clinical trials that incorporate continuous AI feedback loops. Unlike static, linear approaches, these dynamic frameworks allow AI systems to learn and adapt during trial execution, promising more efficient and resilient evaluation aligned with the evolving nature of AI models.
The FDA’s January 2025 draft guidance on AI in regulatory decision-making reflects increasing regulatory focus on AI credibility, transparency, and risk management. Nonetheless, challenges persist in ensuring data quality, ethical safeguards, and patient safety within these adaptive designs.
The Power of Partnerships and Computational Advances: Genentech and NVIDIA Leading the Charge
Underlying these technological advances is a critical enabler: exponential growth in computational power and strategic collaborations.
The multi-year partnership between Genentech and NVIDIA exemplifies how pharmaceutical and technology leaders combine forces to accelerate AI-driven drug discovery. Leveraging NVIDIA’s DGX Cloud infrastructure and BioNeMo generative AI platform, Genentech optimizes AI models within its “lab in a loop” framework, enhancing molecular design speed and experimental iteration quality.
This collaboration reflects broader industry trends. AI spending in pharma is projected to reach $3 billion by 2025, with an estimated 30% of new drugs expected to be discovered using AI methodologies. Generative AI models, including DeepMind’s AlphaFold and emerging platforms like Genie, are already revolutionizing molecular modeling and protein folding.
Despite these gains, uncertainties remain. Integrating AI into drug discovery workflows is complex and not a plug-and-play solution. Data quality, model validation, and clinical translation challenges endure. The well-known “Eroom’s Law” reminds us that drug development complexity historically resists efficiency gains, and AI, though promising, is no silver bullet.
Navigating the Uncertainties Ahead
As AI-driven drug discovery advances rapidly, it is essential to balance enthusiasm with scientific rigor. Key research gaps include:
- Data Integration and Standardization: Developing interoperable, high-quality multi-omics and clinical datasets to fuel AI models reliably.
- Model Transparency and Validation: Ensuring explainability and regulatory compliance for AI-driven decisions.
- Clinical Translation: Bridging accelerated discovery timelines with successful clinical outcomes.
- Ethical and Social Implications: Addressing data privacy, algorithmic bias, and equitable access to AI-enabled therapies.
Meeting these challenges requires a collaborative ecosystem of biologists, data scientists, ethicists, regulators, and industry leaders working in concert. Ultimately, success will be measured not only by speed or novelty but by meaningful improvements in patient outcomes.
In summary, the future of AI in drug discovery is a dynamic interplay of autonomous laboratories, holistic biological data integration, innovative generative algorithms, and adaptive clinical trial frameworks. Powered by unprecedented computational capacity and strategic partnerships, navigating this frontier demands a balance of bold innovation with meticulous validation—an endeavor the pharmaceutical industry is only beginning to master.
Technology / Trend | Description | Key Examples | Challenges | Impact / Potential |
---|---|---|---|---|
Self-Driving Laboratories | Robots and AI autonomously design, conduct, and analyze experiments continuously. | AI agents: Scibot, Coscientist, DOLPHIN; Quantos robot; Harvard & Seoul National University swarm robots | Reliability, ease of use, integration with existing workflows | Revolutionize pharmaceutical R&D; reduce drug discovery timelines; phased adoption starting with incremental automation |
Multi-Omics Data Integration | Integration of genomics, transcriptomics, proteomics, metabolomics for holistic biological insights. | Companies: Freenome, Tempus | Standardization, interoperable protocols, ethical concerns (data privacy, bias), modular pipeline integration | Advance personalized medicine; enable precise target selection and patient stratification |
Generative Models and Reinforcement Learning | AI algorithms design novel drug molecules by exploring chemical space and optimizing candidates. | Insilico Medicine’s Pharma.AI; Recursion OS | Translating AI-generated molecules to approved drugs; validation; clinical trial failures | Accelerate drug discovery phases; push boundaries of de novo drug design |
Explainable AI and Real-Time Adaptive Clinical Trials | Transparent AI frameworks and adaptive trial designs with continuous AI feedback. | TrialGPT; FDA draft guidance on AI (Jan 2025) | “Black box” issues, data quality, ethical safeguards, patient safety, regulatory acceptance | Improve trial efficiency; enhance clinician and regulator trust; enable dynamic trial adjustments |
Partnerships and Computational Advances | Collaboration between pharma and tech companies leveraging powerful computing and AI platforms. | Genentech & NVIDIA partnership; NVIDIA DGX Cloud; BioNeMo; DeepMind AlphaFold; Genie | Complexity of integration; data quality; model validation; clinical translation challenges | Accelerate AI-driven drug discovery; enhance molecular design speed and experiment quality |
Navigating Uncertainties Ahead | Key research gaps and open questions for AI in drug discovery. | — | Data integration & standardization, model transparency & validation, clinical translation, ethical & social implications | Require cross-disciplinary collaboration; ensure meaningful patient outcome improvements |
Conclusion: Balancing Optimism and Caution in the AI-Powered Pharma Revolution
Balancing Optimism and Caution in the AI-Powered Pharma Revolution
How can we reconcile the undeniable promise of AI in drug discovery with the sobering realities of its current limitations and ethical complexities? The pharmaceutical sector stands at a critical inflection point where AI-driven innovation is reshaping traditional paradigms. Yet, it remains essential to temper enthusiasm with a clear-eyed assessment of what AI can—and cannot—achieve today.
The Transformative Potential and Technical Hurdles
AI’s capacity to accelerate drug discovery has moved beyond theory into tangible practice. Foundation models, trained on vast and diverse biological datasets, are enabling a shift from linear, reductionist methods toward holistic modeling of complex biological systems. By 2025, AI agents are expected to automate at least 50% of conventional bioinformatics workflows, such as RNA-seq analysis, dramatically speeding up data interpretation and enabling more rapid hypothesis generation.
Despite these advances, this acceleration has not yet fully translated into improved clinical success rates. The drug discovery timeline, long constrained by Eroom’s Law—the observation that drug development efficiency declines despite technological progress—has seen some compression. For example, Insilico Medicine has reduced its discovery phase from the industry standard 2.5–4 years to as little as 9–18 months for select candidates, illustrating AI’s potential to condense early-stage development.
However, many AI-developed drug candidates remain in preclinical or early clinical phases, with discontinuations highlighting the persistent gap between computational promise and biological complexity. The challenge extends beyond technical constraints. Cultural and institutional inertia within pharma slows AI adoption. Concerns around data misuse and algorithmic opacity persist, as many AI models operate as “black boxes” with decision-making processes that defy straightforward interpretation—complicating their integration into highly regulated environments.
The Imperative of High-Quality Data and Human Oversight
Data quality remains the cornerstone of effective AI drug discovery. Not all data is created equal; the reliability of AI models depends heavily on the quality, consistency, and comprehensiveness of biological and chemical datasets. Fragmented, biased, or poorly annotated data can mislead even the most sophisticated algorithms. Initiatives like the Human Cell Atlas strive to provide standardized, high-resolution cellular maps, offering robust inputs to feed AI models and enhance their predictive power.
Moreover, proprietary data silos within pharmaceutical companies limit opportunities for collaborative breakthroughs. The tension between commercial interests and open science challenges the development of “broad-spectrum” datasets essential for holistic AI modeling.
Human oversight remains indispensable. While AI can automate routine analyses and generate hypotheses, expert judgment is critical for validation, interpretation, and ethical governance. The FDA’s January 2025 draft guidance on AI in regulatory decision-making emphasizes transparency, life cycle maintenance, and early engagement with regulators to ensure AI tools meet rigorous safety and efficacy standards. This regulatory alignment is not mere bureaucracy; it is essential to maintain public trust and patient safety in an increasingly automated discovery process.
Ethical Considerations and Regulatory Alignment
The ethical landscape of AI in pharma is multifaceted, encompassing data privacy, bias mitigation, intellectual property rights, and accountability for AI-driven decisions. Patients have a right to know who is responsible when AI-derived predictions or treatments fail. The irreplaceable human elements of compassion and empathy—hallmarks of clinical care—cannot be outsourced to machines.
Regulatory frameworks are evolving to address these challenges. The European Union’s GDPR and ethics guidelines for “Trustworthy AI” set high standards for transparency and fairness. Agencies such as the UK’s MHRA and the FDA have published risk-based frameworks to assess AI credibility, reflecting a global trend toward harmonized standards that balance innovation with oversight.
Pharmaceutical companies, including GSK, have proactively established internal AI governance frameworks emphasizing ethical innovation, privacy, robustness, fairness, and transparency. Such internal discipline is becoming a competitive necessity as regulatory scrutiny intensifies, ensuring that AI adoption aligns with ethical principles and compliance mandates.
Looking Ahead: Synergy, Societal Impact, and Responsibility
AI is poised to fundamentally redefine pharmaceutical R&D. By 2025, it is projected that approximately 30% of new drugs will be discovered using AI—up from about 10% via traditional methods. Generative AI models like DeepMind’s AlphaFold and platforms such as Genie exemplify breakthroughs in molecular design, while innovations like decentralized clinical trials and digital twins are revolutionizing drug development and manufacturing processes.
This technological synergy promises tangible societal benefits: faster access to novel therapies, personalized medicine tailored to patient genotypes and phenotypes, and more efficient clinical trial recruitment through AI-enabled patient stratification. Additionally, reduced R&D costs open possibilities for addressing diseases in underfunded therapeutic areas, expanding equity in healthcare innovation.
However, with great power comes great responsibility. The pharmaceutical industry must cultivate a culture that balances collective success with humility, emotional intelligence, and ethical vigilance to prevent overhype and preserve creativity. Equally important is ensuring equitable access to AI-driven innovations, guarding against the exacerbation of health disparities.
Key Takeaways
-
Accelerated Discovery: AI is speeding up drug discovery workflows and enabling holistic biological modeling but is not a magic bullet; clinical translation still faces significant hurdles.
-
Data and Oversight: The foundation of effective AI integration lies in high-quality, standardized data, coupled with transparent, human-led validation and regulatory compliance.
-
Ethical and Regulatory Maturation: Emerging frameworks focus on transparency, accountability, fairness, and patient safety to build trust in AI-driven therapeutics.
-
Responsible Governance: Leading pharmaceutical organizations embed responsible AI governance internally while embracing generative AI, digital twins, and decentralized trials to reshape development.
-
Balanced Approach: The ultimate success of AI in pharma depends on marrying innovation with thoughtful oversight and societal responsibility—not technology alone.
In sum, the AI-powered pharma revolution is real and accelerating, but it demands a nuanced, evidence-based approach—one that celebrates breakthroughs without losing sight of the complexity, ethics, and human elements at the heart of medicine.
Category | Key Points |
---|---|
Accelerated Discovery | AI speeds up drug discovery workflows and enables holistic biological modeling; clinical translation still faces significant hurdles. |
Data and Oversight | High-quality, standardized data is essential; human-led validation and regulatory compliance are critical. |
Ethical and Regulatory Maturation | Frameworks emphasize transparency, accountability, fairness, and patient safety to build trust in AI therapeutics. |
Responsible Governance | Pharma companies implement internal AI governance and use generative AI, digital twins, and decentralized trials to innovate. |
Balanced Approach | Success depends on combining innovation with oversight and societal responsibility, not technology alone. |