AI in Banking: Automating Loans, Credit, and Customer Service
- Introduction: Why AI is a Game Changer in Banking Loans, Credit Scoring, and Customer Service
- Introduction: Why AI is a Game Changer in Banking Loans, Credit Scoring, and Customer Service
- The Scale and Speed of AI Adoption in Banking
- Why Loans, Credit Scoring, and Customer Service Are Critical Frontiers
- Navigating Innovation with Ethical Responsibility
- The Road Ahead: Balancing Opportunity and Risk
- Foundations of AI in Banking: Core Technologies and How They Power Automation
- Foundations of AI in Banking: Core Technologies and How They Power Automation
- Natural Language Processing (NLP): From Understanding Queries to Intelligent Conversations
- Computer Vision: Automating Document Processing and Fraud Detection
- Predictive Analytics: Smarter Credit Scoring and Risk Assessment
- Robotic Process Automation (RPA): Streamlining Workflows with AI Intelligence
- Connecting the Dots: How These Technologies Work Together
- Automating Loan Origination and Underwriting: Technical Workflows and Challenges
- Automating Loan Origination and Underwriting: Technical Workflows and Challenges
- From Paper to Pixels: Intelligent Document Processing (IDP) and Verification Pipelines
- AI’s Crucible: Credit Risk Scoring and Fraud Detection Models
- Navigating the Minefield: Data Quality, Explainability, Bias, and Compliance
- Putting It All Together: Real-World Impact and Performance Metrics
- AI-Driven Credit Scoring: Enhancing Accuracy While Addressing Bias and Fairness
- AI-Driven Credit Scoring: Enhancing Accuracy While Addressing Bias and Fairness
- Traditional vs. Machine Learning Credit Scoring Models
- Detecting and Mitigating Bias: Adversarial Models and Fairness-Aware Algorithms
- Ensuring Model Validation, Transparency, and Ethical Oversight
- Broader Societal Implications and the Path Forward
- Transforming Customer Service with AI: From Chatbots to Sentiment Analysis
- Transforming Customer Service with AI: From Chatbots to Sentiment Analysis
- The Rise of Virtual Agents and Predictive Call Routing
- Core AI Technologies Powering Automation
- Operational Benefits: Efficiency, Personalization, and Consistency
- Limitations and Challenges: Complexity, Trust, and Human Integration
- Looking Ahead: The Human-AI Balance
- Benchmarking AI Solutions in Banking: Comparative Analysis of Platforms and Performance
- Benchmarking AI Solutions in Banking: Comparative Analysis of Platforms and Performance
- Leading AI Platforms for Loan Automation, Credit Scoring, and Customer Service
- Evaluating AI Solutions: Ease of Deployment, Scalability, and Explainability
- Integration Capabilities and Cost-Effectiveness
- Case Studies: Real-World Strengths and Challenges
- Guidance for Banks Evaluating AI Investments
- Closing Thoughts
- Future Directions and Ethical Considerations: Navigating Opportunities and Risks in AI-Enabled Banking
- Future Directions and Ethical Considerations: Navigating Opportunities and Risks in AI-Enabled Banking
- Emerging AI Technologies: From Automation to Strategic Insight
- Navigating the Regulatory and Cultural Landscape
- Ethical Considerations: Building Trust as the Cornerstone
- Strategic Roadmap for Sustainable AI Adoption
- Looking Ahead: Building Trust and Creating Long-Term Value
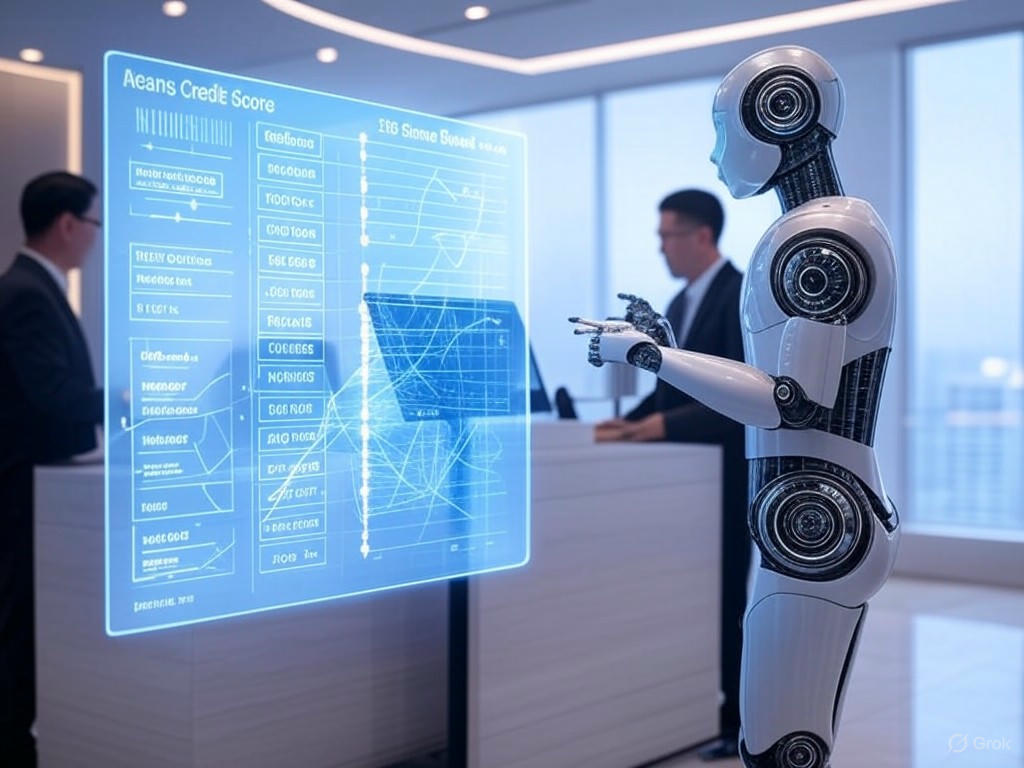
Introduction: Why AI is a Game Changer in Banking Loans, Credit Scoring, and Customer Service
Introduction: Why AI is a Game Changer in Banking Loans, Credit Scoring, and Customer Service
What happens when a technology shifts from cautious experimentation to becoming a central pillar of an industry’s strategy within a few years? In banking, that technology is artificial intelligence (AI), whose impact on loans, credit scoring, and customer service is profoundly transformative.
The Scale and Speed of AI Adoption in Banking
By 2025, approximately 70% of financial services executives expect AI to directly drive revenue growth, signaling a dramatic evolution from AI’s early role as a cost-cutting and operational efficiency tool. Today, banks leverage generative AI (GenAI) to accelerate product development, modernize IT infrastructure, and create more humanlike, connected customer experiences.
For example, Banco Montepio’s collaboration with Devoteam led to the implementation of an advanced chatbot-powered Intelligent Knowledge Management system. This innovation revolutionized internal information access and streamlined employee workflows. Similarly, Bank of America’s multibillion-dollar technology investment embeds AI deeply across its global technology, operations, and client service functions.
These examples illustrate a broader trend: banks are moving beyond tentative steps to full-scale AI integration. However, this rapid adoption brings challenges such as data security concerns, regulatory uncertainty, and a shortage of AI-specialized talent. Additionally, the pace of AI innovation often outstrips regulators’ ability to fully grasp emerging risks, complicating compliance and governance.
Why Loans, Credit Scoring, and Customer Service Are Critical Frontiers
Focusing on loans, credit scoring, and customer service is strategic because these pillars profoundly influence a bank’s competitiveness and customer satisfaction.
-
Loans form the lifeblood of banking profitability. AI-driven automation streamlines loan origination, reduces processing times, and enhances underwriting accuracy. Banks embed AI decision engines to optimize risk assessment and expedite approvals, improving both customer experience and operational efficiency.
-
Credit scoring has traditionally relied on limited, static data. AI expands this by incorporating alternative data sources—ranging from digital footprints to behavioral analytics—enabling more inclusive and precise risk assessments. This reduces default rates and extends credit access to underserved populations, addressing persistent financial exclusion.
-
Customer service is undergoing a radical transformation with AI-powered conversational agents projected to handle up to 70% of interactions by 2025. These systems use natural language processing and machine learning to deliver personalized, 24/7 support, freeing human agents to focus on complex queries. The result is faster resolution times, higher satisfaction, and significant cost savings—potentially adding $200 to $340 billion annually in operating profits industry-wide.
Together, these three areas constitute the core battleground where banks vie for customer loyalty, regulatory compliance, and financial performance. AI’s ability to simultaneously enhance loans, credit scoring, and customer service underscores why it is a genuine game changer.
Navigating Innovation with Ethical Responsibility
Technological innovation alone does not guarantee success or trust. The ethical dimension of AI in banking is as critical as the technical one. Banks must address algorithmic bias, data privacy, and transparency to maintain customer trust and meet regulatory expectations.
Currently, only a minority of banks have publicly committed to Responsible AI (RAI) principles, despite increasing consumer concerns over privacy and personalization. Empowering customers with control over their data—such as options to opt into AI-driven personalization or notification frequency—is essential to prevent alienation.
Robust governance frameworks are equally important to enforce accountability and transparency. The Bank for International Settlements warns that ignoring these issues risks eroding trust and invites regulatory backlash. Proactive ethical strategies are thus critical to safeguarding reputations and ensuring long-term viability.
The Road Ahead: Balancing Opportunity and Risk
AI’s potential to reshape banking is immense but accompanied by uncertainty. As financial institutions accelerate AI adoption, they must balance innovation with rigorous risk management, talent development, and infrastructure modernization.
Successful banks will be those that:
-
Invest strategically across AI capability layers—from foundational data and core technologies to decision-making engines and customer engagement platforms.
-
Foster collaboration between business and technology teams to ensure AI initiatives align with clear value creation objectives.
-
Embrace transparency and ethical AI governance to build and sustain customer trust.
-
Prepare for evolving regulatory landscapes by adopting flexible, phased AI integration approaches.
In essence, AI is not merely a tool but a strategic imperative poised to redefine banking’s future. By recognizing both its transformative power and ethical complexities, banks can unlock unprecedented growth and customer value while avoiding the pitfalls of hype and misapplication.
Aspect | Details |
---|---|
AI Adoption by 2025 | 70% of financial services executives expect AI to directly drive revenue growth |
Examples of AI in Banking | Banco Montepio’s chatbot-powered Intelligent Knowledge Management system; Bank of America’s AI integration in technology, operations, and client services |
Challenges | Data security concerns, regulatory uncertainty, shortage of AI-specialized talent, regulatory lag behind AI innovation |
Loans | AI automates origination, reduces processing time, enhances underwriting accuracy, optimizes risk assessment, expedites approvals |
Credit Scoring | AI incorporates alternative data (digital footprints, behavioral analytics) for inclusive, precise risk assessments reducing default rates and enabling credit access |
Customer Service | AI conversational agents projected to handle 70% of interactions by 2025, 24/7 personalized support, faster resolutions, $200-$340 billion annual operating profit potential |
Ethical Responsibility | Address algorithmic bias, data privacy, transparency; few banks committed to Responsible AI; importance of customer data control and robust governance |
Strategies for Success | Strategic AI investment, collaboration between business and tech teams, transparency and ethical AI governance, preparation for regulatory changes |
Foundations of AI in Banking: Core Technologies and How They Power Automation
Foundations of AI in Banking: Core Technologies and How They Power Automation
What enables banks to automate complex tasks like loan approvals, credit scoring, and customer service with increasing accuracy and speed? At the heart of this transformation are a few key AI technologies—each addressing distinct aspects of the banking workflow. Let’s explore the major players: natural language processing (NLP), computer vision, predictive analytics, and robotic process automation (RPA). Understanding these core technologies clarifies not only their capabilities but also their limitations and associated risks.
Natural Language Processing (NLP): From Understanding Queries to Intelligent Conversations
Natural language processing has evolved from a niche research topic into a foundational technology in banking automation. The rise of transformer-based generative AI models—such as OpenAI’s GPT-4—enables banks to deploy systems that do more than parse text: they generate human-like responses. This advancement powers chatbots that handle customer inquiries around the clock and AI agents that analyze lengthy loan applications or regulatory documents in seconds.
At the core of these models is the transformer architecture, which uses self-attention mechanisms to consider relationships between all words in a sentence or paragraph simultaneously, rather than sequentially processing text as earlier models did. This enables a deep contextual understanding, much like a skilled loan officer interpreting nuances in a customer’s request.
However, training such models requires vast amounts of data—often billions of words from diverse sources—to capture language subtleties effectively. Banking-specific NLP applications depend on domain-tailored datasets to accurately comprehend financial terminology and regulatory jargon. For instance, Named Entity Recognition (NER) algorithms extract key entities such as customer names, dates, and transaction amounts from unstructured documents, converting them into structured data that banks can readily act upon.
A concrete example is Wells Fargo’s generative AI assistant “Fargo,” which has handled 20 million customer interactions since early 2023. Fargo reduces response times and personalizes financial advice, demonstrating how GenAI can scale customer service efficiently. Yet, the power of these models comes with responsibility. Algorithmic bias remains a significant concern, especially if training data lacks diversity, potentially perpetuating unfair lending practices. Banks must implement continuous monitoring and auditing to mitigate these risks.
Computer Vision: Automating Document Processing and Fraud Detection
If NLP is the AI “brain” parsing text, computer vision acts as the “eyes” scanning images and documents. Banks deal with mountains of paperwork—loan forms, credit reports, identity documents—and manual review is slow and error-prone. Computer vision models, powered by deep learning, automate this process by recognizing text and visual cues in scanned documents.
Optical Character Recognition (OCR) technology converts printed or handwritten text into machine-readable formats and has been a long-standing staple. Modern Intelligent Document Processing (IDP) systems extend OCR by integrating vision-language models (VLMs) that jointly interpret textual and visual content. This allows extraction of complex structures like tables or handwritten notes, cutting manual data entry by up to 95%.
Beyond document handling, computer vision enhances fraud detection. AI inspects fonts, holograms, lighting inconsistencies, and Machine Readable Zone (MRZ) patterns on passports or IDs to detect forgery attempts that human inspectors might overlook. This layered defense is critical for protecting banks from financial losses and regulatory penalties.
Think of computer vision as a seasoned bank teller who verifies identity not just by reading a passport but by observing subtle security features like hologram reflections or microprinting. AI replicates and scales this expertise digitally, enabling real-time identity verification at scale.
Predictive Analytics: Smarter Credit Scoring and Risk Assessment
Traditional credit scoring models rely on static, limited financial data, but AI-driven predictive analytics are transforming this landscape. By analyzing vast datasets—including transaction histories, payment behaviors, and alternative data sources—machine learning models forecast credit risk with unprecedented precision.
Banks employ algorithms such as gradient boosting machines, random forests, and neural networks to uncover nuanced patterns predictive of default risk. These models update dynamically, reflecting real-time changes in borrower behavior, enabling early warning and proactive risk mitigation.
For example, fintech lenders adopting AI credit models have achieved up to a 25% reduction in default rates. Integration of open banking data further enhances accuracy: markets with advanced open banking infrastructure report credit default predictions that are 41% more precise than traditional bureau-only approaches.
A helpful analogy is to think of AI as a seasoned underwriter who remembers millions of past cases, spotting subtle red flags no human could process in time. Yet, banks must remain vigilant to ensure fairness and transparency, as opaque AI decision-making risks perpetuating biases or excluding underserved populations.
Robotic Process Automation (RPA): Streamlining Workflows with AI Intelligence
Robotic process automation addresses the critical need to automate repetitive, rule-based tasks that burden banking operations—such as data entry, document routing, and compliance checks. Unlike AI models that learn from data, RPA bots execute predefined instructions but increasingly integrate AI components to handle exceptions and unstructured inputs.
The combination of AI with RPA—often termed Intelligent Process Automation—enables end-to-end workflow automation. For instance, an AI-powered system can extract loan application data through NLP and computer vision, score creditworthiness via predictive analytics, and trigger RPA bots to process approvals, update backend systems, and notify customers.
Imagine RPA as a tireless virtual assistant who copies data across systems without fatigue—but now enhanced with AI “vision” and “language skills” to understand what data to process and when to escalate issues. This synergy drives operational cost reductions of 20–30% and accelerates loan processing times significantly.
Connecting the Dots: How These Technologies Work Together
The true power of AI in banking lies not in any single technology but in their orchestration. A typical automated loan process might begin with computer vision scanning submitted documents, NLP parsing application text and customer queries, predictive models assessing credit risk, and RPA orchestrating backend workflows.
This layered architecture ensures high accuracy, speed, and scalability while maintaining regulatory compliance. However, banks must invest in high-quality, diverse training data and maintain human oversight to avoid pitfalls such as bias, security vulnerabilities, and model drift.
In summary, the foundations of AI-driven banking automation rest on combining advanced machine learning architectures—especially transformer-based generative AI models—with robust data pipelines and intelligent automation tools. This integration is reshaping how banks serve customers and manage risk. Yet, realizing AI’s full potential demands thoughtful implementation, continuous monitoring, and a commitment to responsible, ethical use.
AI Technology | Core Function | Key Features | Examples & Benefits | Risks & Considerations |
---|---|---|---|---|
Natural Language Processing (NLP) | Understanding and generating human language | Transformer-based models, Named Entity Recognition, Generative AI (e.g., GPT-4) | Wells Fargo’s “Fargo” handles 20M+ interactions, reduces response time, personalizes advice | Algorithmic bias, need for diverse training data, continuous auditing required |
Computer Vision | Automating document processing and fraud detection | OCR, Intelligent Document Processing (IDP), Vision-Language Models (VLMs), fraud pattern recognition | Up to 95% reduction in manual data entry, real-time identity verification, forgery detection | Potential errors in complex visual recognition, dependency on image quality |
Predictive Analytics | Credit scoring and risk assessment | Machine learning algorithms (gradient boosting, random forests, neural networks), dynamic model updating | 25% reduction in default rates, 41% more accurate with open banking data | Opaque decision-making, risk of bias, fairness and transparency concerns |
Robotic Process Automation (RPA) | Automating repetitive, rule-based tasks | Predefined instruction execution, integration with AI for exception handling | 20–30% operational cost reduction, faster loan processing, end-to-end workflow automation | Limited learning ability alone, requires AI integration for complex tasks |
Automating Loan Origination and Underwriting: Technical Workflows and Challenges
Automating Loan Origination and Underwriting: Technical Workflows and Challenges
What unfolds behind the scenes when a borrower applies for a loan online and receives an almost instantaneous decision? The answer lies in a sophisticated, increasingly AI-powered pipeline that transforms traditional, manual loan origination and underwriting processes into streamlined, efficient workflows. This section explores how AI is revolutionizing these critical banking functions while addressing the technical and ethical challenges that accompany this transformation.
From Paper to Pixels: Intelligent Document Processing (IDP) and Verification Pipelines
A major bottleneck in loan origination has historically been document handling. Applicants submit vast volumes of paperwork—income proofs, tax returns, bank statements, identity documents—each requiring accurate verification. Artificial intelligence-powered Intelligent Document Processing (IDP) platforms are reshaping this landscape.
Leading IDP solutions, such as Fenergo’s platform, claim to reduce manual document handling times by up to 72%. These systems leverage machine learning and natural language processing (NLP) to automatically extract, classify, and verify data from both structured and unstructured documents. This shift from manual to automated ingestion accelerates throughput, minimizes human error, and reduces compliance risks.
IDP operates as the gateway to the loan origination pipeline, delivering clean, structured data downstream into credit risk scoring and fraud detection modules. Integration examples include cloud-native loan origination software like Finlux by M2P, which manages portfolios exceeding $3.2 billion, demonstrating the scalability of AI-driven document workflows. Additionally, platforms such as Encompass by ICE Mortgage Technology are embedding generative AI to further streamline application processing and enhance predictive modeling capabilities.
However, automation introduces new complexities. AI-driven document verification must now detect increasingly sophisticated forgery attempts, as AI itself can generate highly convincing fraudulent documents. The digital identity verification sector, which surpassed 70 billion checks in 2024, combats this threat through biometric authentication and multi-factor verification. Despite these advances, trust remains fragile, demanding continuous innovation in AI robustness and fraud detection techniques.
AI’s Crucible: Credit Risk Scoring and Fraud Detection Models
How does AI improve the accuracy and inclusivity of creditworthiness assessments? Traditional credit scoring models rely on a narrow set of financial variables and rigid rules, often excluding individuals with limited credit histories. AI and machine learning models overcome these limitations by incorporating a broader spectrum of data and uncovering complex, nonlinear relationships that human underwriters might overlook.
Banks adopting AI-based credit scoring report a 20–30% increase in approval rates for previously unscorable individuals, alongside default rate reductions of up to 15%. These gains result from AI’s ability to integrate alternative data sources such as utility and rent payment histories, applying advanced predictive analytics for more nuanced risk evaluation.
For example, Fannie Mae’s Collateral Underwriter system uses machine learning models to enhance mortgage risk assessment, enabling appraisal waivers for eligible borrowers and reducing operational inefficiencies. Concurrently, AI-powered fraud detection platforms like those described by Durango Merchant Services employ deep learning to identify suspicious transactional patterns in real time—critical for countering fraud schemes that evolve within milliseconds.
Nevertheless, AI models are not without pitfalls. Their extensive data dependencies introduce risks of bias and overfitting. Furthermore, the opacity of many AI algorithms—the “black box” phenomenon—complicates explainability, a key regulatory and trust requirement. Regulatory frameworks such as the EU AI Act emphasize transparency and fairness, compelling financial institutions to rigorously validate and document AI model behavior.
Navigating the Minefield: Data Quality, Explainability, Bias, and Compliance
Deploying AI in loan origination extends beyond technical implementation into ethical and regulatory terrain. High-quality data is foundational; without accurate, complete, and current datasets, AI systems cannot perform reliably. KlearStack highlights that data verification inefficiencies cause delays of 4–6 hours per mortgage case, underscoring the need for robust automated data quality controls.
Explainability remains a crucial challenge. Banking CIOs stress that AI systems must be interpretable and trustworthy to gain internal acceptance and meet evolving regulatory demands. Without transparent decision rationales, banks risk compliance violations and erosion of customer confidence. Emerging tools and frameworks for AI model risk management aim to demystify AI decisioning, ensuring rigorous validation, documentation, and governance.
Addressing bias is equally imperative. AI trained on historical data can perpetuate societal inequities if not carefully audited and adjusted. Some institutions leverage AI proactively to promote responsible, equitable lending by expanding access to underserved communities. Achieving this requires ongoing monitoring, bias mitigation algorithms, and recalibration.
Regulatory compliance is integral, not an afterthought. Lending compliance programs increasingly incorporate AI governance alongside traditional risk management. Financial institutions must balance automation benefits with adherence to anti-discrimination laws, data privacy regulations like GDPR and CCPA, and prepare for new AI-specific rules. Effective governance demands cross-functional collaboration among data scientists, compliance officers, and business leaders.
Putting It All Together: Real-World Impact and Performance Metrics
The transformative impact of AI-powered loan origination systems is evident in key performance indicators. Applications that once took up to 96 hours to process manually can now be handled in real or near-real time. Improved accuracy reduces default risk and fraud losses, while automated compliance checks mitigate costly violations.
For instance, integrating AI document verification has been shown to cut manual review times by over 70%, significantly boosting throughput without compromising quality. AI credit scoring models not only increase approval rates but also enable lenders to tap into previously underserved markets overlooked by traditional credit bureaus.
Yet, vigilance remains essential. Rapid AI adoption requires sustained investment in talent, technology, and governance to maintain performance gains and manage emerging risks. In this evolving frontier of lending automation, success hinges on balancing speed, accuracy, fairness, and transparency—qualities that will distinguish leaders in responsible, efficient lending as we move deeper into 2025.
Area | AI Application | Benefits | Challenges | Examples / Metrics |
---|---|---|---|---|
Document Processing | Intelligent Document Processing (IDP) using ML and NLP | Reduces manual handling by up to 72%, accelerates throughput, minimizes errors, reduces compliance risks | Detecting sophisticated forgeries, maintaining trust, combating AI-generated fraudulent documents | Fenergo’s platform; Finlux managing $3.2B+ portfolios; Encompass embedding generative AI |
Credit Risk Scoring & Fraud Detection | Machine learning models for credit scoring; deep learning for fraud detection | 20-30% increase in approval rates; up to 15% default rate reduction; real-time fraud detection | Bias, overfitting, explainability (“black box”), regulatory compliance | Fannie Mae Collateral Underwriter; Durango Merchant Services fraud detection |
Data Quality & Compliance | Automated data quality controls; AI governance frameworks | Reduces verification delays (4-6 hours per case), ensures regulatory compliance, improves explainability | Ensuring data accuracy, mitigating bias, meeting GDPR/CCPA and AI-specific regulations | KlearStack data delays; cross-functional governance teams |
Performance and Impact | End-to-end AI loan origination automation | Reduces processing time from 96 hours to near real-time; boosts throughput by over 70%; expands lending to underserved markets | Need for sustained investment, balancing speed, fairness, transparency, and accuracy | 70%+ reduction in manual review; increased approval and reduced default rates |
AI-Driven Credit Scoring: Enhancing Accuracy While Addressing Bias and Fairness
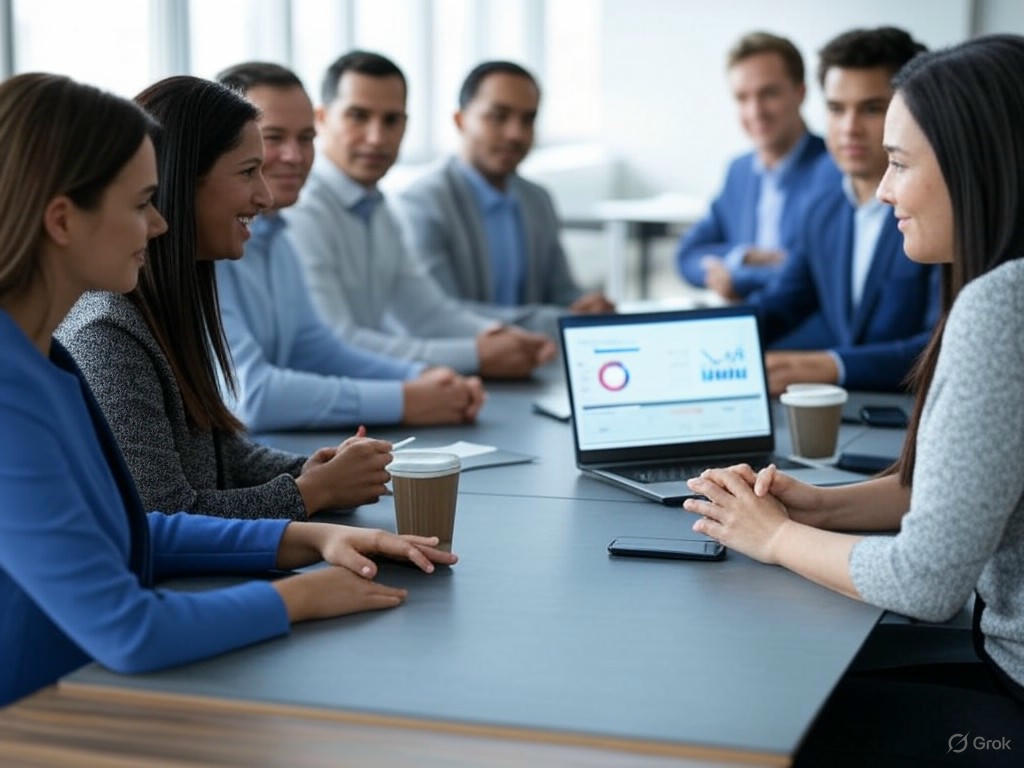
AI-Driven Credit Scoring: Enhancing Accuracy While Addressing Bias and Fairness
Imagine a credit evaluation system that goes beyond the narrow constraints of past credit history to assess your financial behavior through a richer, more nuanced lens. This is the promise—and the challenge—of AI-driven credit scoring. Traditional models like FICO and VantageScore 4.0 have long shaped lending decisions, relying primarily on standard metrics such as payment history and credit utilization. However, these conventional systems often penalize groups with limited or non-traditional credit histories—such as Native communities hesitant to engage with federal institutions or young adults new to the financial system—thereby perpetuating financial exclusion.
Traditional vs. Machine Learning Credit Scoring Models
Traditional credit scoring approaches face criticism for their limited scope and potential to exclude many consumers. The Federal Reserve Bank of Kansas City highlights that such models “disproportionately punish certain consumers” by depending heavily on historical credit data that may not fully capture an individual’s repayment ability. For instance, reliance solely on FICO scores can create significant barriers to affordable credit among underserved populations.
In contrast, AI and machine learning models leverage a broader spectrum of data sources—including alternative data and open banking information—to create a more comprehensive and dynamic picture of creditworthiness. The latest iterations of VantageScore incorporate these alternative data points and have demonstrated up to a 10% improvement in predictive accuracy compared to earlier versions. This expanded data landscape enables financial institutions to extend credit to previously “unscorable” individuals, resulting in loan approval rate increases of 20–30% and reductions in default rates by up to 15%.
Beyond incorporating more data, AI-powered credit scoring models excel at making smarter sense of complex information. Techniques like natural language processing (NLP) monitor real-time financial health signals, while hybrid AI models optimize the balance between computational power and efficiency. These innovations are particularly impactful in areas such as commercial real estate lending, where traditional models struggle to adapt rapidly to market fluctuations.
Detecting and Mitigating Bias: Adversarial Models and Fairness-Aware Algorithms
With AI’s expanded capabilities comes a heightened responsibility to ensure fairness. Bias in AI credit scoring often arises from historical inequities embedded within training data, measurement errors, or label assignments. For example, datasets skewed toward certain demographic groups can cause models to underperform for others, reinforcing systemic discrimination.
To address these challenges, researchers and practitioners employ fairness-aware algorithms and adversarial models designed to detect and mitigate bias. These interventions typically fall into three categories:
- Pre-processing: Modifying datasets before training, such as rebalancing samples or removing sensitive attributes to reduce bias.
- In-processing: Incorporating fairness constraints or penalties directly into the learning algorithms during model training.
- Post-processing: Adjusting model outputs after training to satisfy fairness criteria before final decisions are made.
Empirical evaluations using datasets like the German credit data have tested more than a dozen bias mitigation strategies with mixed results. Algorithms such as Prejudice Remover and Gerry Fair show potential but also reveal inconsistencies, highlighting the delicate balance between fairness and predictive accuracy in profit-driven financial environments.
Regulatory bodies are increasingly vigilant. The Consumer Financial Protection Bureau (CFPB) has made clear that “there is no ‘advanced technology’ exception to Federal consumer financial laws,” underscoring that AI credit scoring models must undergo rigorous disparate impact analyses and fair lending tests. This regulatory scrutiny emphasizes the need for ongoing bias monitoring and timely corrective action.
Ensuring Model Validation, Transparency, and Ethical Oversight
The growing complexity of AI credit scoring models introduces new challenges around model risk management, transparency, and accountability. Robust validation processes are essential to prevent errors caused by overfitting, poor data quality, or shifting economic conditions. Model risk management has become a critical component for ensuring both reliability and fairness.
Transparency remains a significant obstacle. Many AI models, especially deep learning architectures, operate as “black boxes,” making it difficult for lenders and borrowers alike to understand the rationale behind credit decisions. To improve explainability, institutions are adopting several approaches:
- Designing inherently interpretable models that deliver clear, actionable insights.
- Applying post hoc explainability techniques such as SHAP (Shapley Additive Explanations) and LIME (Local Interpretable Model-agnostic Explanations) to provide understandable justifications for individual decisions.
- Disclosing the use of algorithms to consumers to build trust and comply with regulatory expectations.
Despite these efforts, regulatory frameworks have yet to establish definitive standards for transparency, leaving many institutions navigating a cautious experimentation phase. The prevailing best practice is “trust but verify”: deploying AI with oversight mechanisms that enable independent auditing and challenge.
Ethical AI governance further demands human oversight and accountability structures. Automated credit decisions should not function in isolation; human experts must have the authority and capability to review, override, or investigate AI-generated outcomes. This checkpoint is vital to prevent AI systems from inadvertently entrenching societal inequities or operationalizing flawed assumptions.
Broader Societal Implications and the Path Forward
AI-driven credit scoring offers significant promise for enhancing financial inclusion, improving operational efficiency, and refining risk management. However, it also raises profound ethical questions regarding fairness, transparency, and accountability. As banks increasingly rely on AI, it is imperative to establish governance frameworks that:
- Embed fairness by design, with continuous monitoring and active bias mitigation.
- Promote transparency to demystify AI-driven decisions for both consumers and regulators.
- Integrate human judgment as an essential safeguard against algorithmic errors or injustices.
- Align practices with evolving regulatory landscapes, including the EU AI Act and emerging U.S. state laws.
Ultimately, AI in credit scoring is a powerful tool—one that can unlock credit access for millions sidelined by traditional models but only through a rigorous commitment to fairness, transparency, and ethical responsibility.
From my experience architecting AI systems in banking, this balance is the true frontier. Success lies not only in building smarter algorithms but in creating trustworthy, equitable, and transparent systems. The future of lending depends on it.
Aspect | Traditional Credit Scoring | AI-Driven Credit Scoring |
---|---|---|
Data Sources | Standard metrics (payment history, credit utilization) | Broader data including alternative data, open banking info, real-time signals |
Coverage | Limited, excludes non-traditional credit histories | Includes previously unscorable individuals |
Predictive Accuracy | Baseline (e.g., early VantageScore versions) | Up to 10% improvement (latest VantageScore versions) |
Loan Approval Rate Impact | Baseline | Increase of 20–30% |
Default Rate Impact | Baseline | Reduction up to 15% |
Bias Mitigation Techniques | Limited or none | Pre-processing, in-processing, post-processing fairness-aware algorithms |
Transparency | More interpretable | Often black-box; uses explainability tools (SHAP, LIME) |
Regulatory Compliance | Established frameworks | Requires rigorous disparate impact analysis and fair lending tests |
Human Oversight | Standard review processes | Essential for review, override, and investigation of AI decisions |
Transforming Customer Service with AI: From Chatbots to Sentiment Analysis
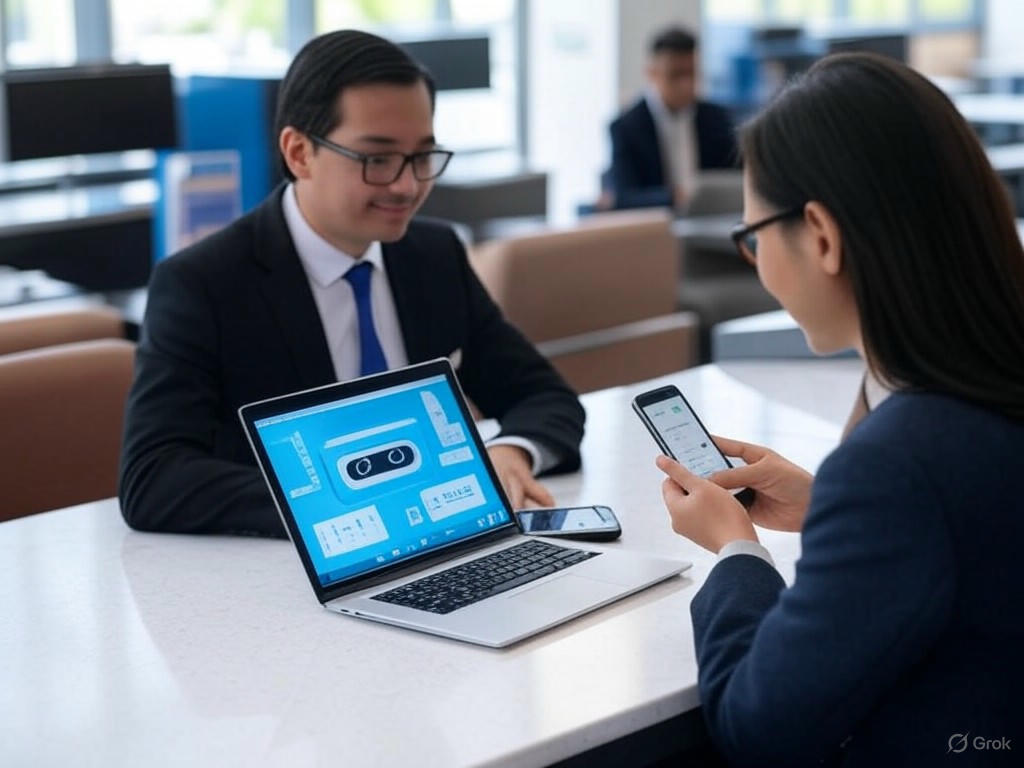
Transforming Customer Service with AI: From Chatbots to Sentiment Analysis
AI is not merely enhancing banking customer service incrementally—it is fundamentally transforming how customers interact with their banks and how banks deliver support. From intelligent virtual agents managing routine inquiries to sophisticated sentiment analysis detecting customer emotions in real time, AI technologies are now indispensable pillars of modern banking customer service.
The Rise of Virtual Agents and Predictive Call Routing
Virtual agents—advanced chatbots powered by natural language processing (NLP) and machine learning—have become the primary interface between banks and their customers. Unlike early rule-based bots, these AI-driven agents understand conversational context and autonomously execute tasks such as balance inquiries, transaction history retrieval, and basic troubleshooting.
For example, Bank of America’s AI-powered chatbot, Erica, exemplifies how conversational AI enables customers to conduct transactions and receive personalized financial advice outside traditional banking hours. By 2025, Gartner predicts 37% of customers will rely on virtual assistants for service interactions, reflecting widespread adoption.
Moreover, 85% of IT executives in banking have clear AI adoption plans for customer-facing solutions, underscoring the strategic importance of virtual agents. These systems are increasingly proactive—guiding users through processes and escalating complex queries to human agents seamlessly.
Complementing virtual agents, predictive call routing leverages real-time analytics and historical interaction data to direct callers to the most appropriate agent. This technology reduces wait times, improves service consistency, and ensures customers receive accurate and uniform information regardless of the agent they reach. Together, virtual agents and predictive routing optimize workflows and elevate customer satisfaction.
Core AI Technologies Powering Automation
Several core AI technologies underpin these customer service innovations:
-
Natural Language Processing (NLP): NLP enables chatbots and virtual agents to comprehend and generate human language effectively. Transformer-based models like GPT-4 have revolutionized this field, allowing banks to interpret complex queries and deliver coherent, context-aware responses. In addition, NLP drives sentiment analysis by scanning customer communications for emotional cues—such as frustration or satisfaction—enabling dynamic, empathetic responses.
-
Speech and Voice Recognition: Voice banking is increasingly popular, allowing customers to access services via spoken commands. Voice biometric authentication enhances security by verifying identities through unique voiceprints. Despite advances, voice recognition still faces challenges with accuracy across diverse accents and noisy environments. Regulatory constraints also limit voice-activated financial transactions in certain jurisdictions.
-
Real-Time Analytics: AI systems continually analyze customer behavior, transaction patterns, and interaction histories to personalize services. Real-time data feeds empower predictive features, anticipating customer needs or early signs of dissatisfaction to improve engagement.
Together, these technologies provide 24/7 availability, personalized experiences, and rapid response times, while significantly reducing manual errors common in human-operated service channels.
Operational Benefits: Efficiency, Personalization, and Consistency
The operational advantages of AI in banking customer service are substantial:
-
24/7 Availability: AI-powered chatbots and voice assistants operate around the clock, meeting modern customer expectations for immediate support at any time.
-
Personalization: Banks utilize AI-driven insights from transactional data and customer preferences to offer tailored advice and product recommendations. For example, chatbots can suggest saving plans based on spending habits or alert customers to unusual account activity in real time.
-
Reduced Manual Errors: Automating repetitive tasks such as balance inquiries and payment scheduling minimizes human errors and ensures service consistency.
-
Cost Efficiency: By automating up to 90% of routine interactions, banks achieve significant operational cost savings. Studies indicate AI-powered customer service solutions can reduce service costs by 25%, with businesses realizing an average return of $3.50 for every dollar invested.
-
Improved Customer Experience: Faster response times and predictive routing reduce customer frustration. According to Zendesk, 59% of consumers believe AI will fundamentally change their interactions with companies within two years, highlighting growing customer acceptance.
Limitations and Challenges: Complexity, Trust, and Human Integration
Despite these benefits, AI-driven customer service faces important limitations:
-
Handling Complex Queries: AI virtual agents often struggle with nuanced or multi-layered issues requiring human judgment, empathy, or ethical considerations. Although sentiment analysis can detect customer frustration, resolving these cases typically requires escalation to skilled human agents. Ensuring seamless handoffs is critical to maintaining service quality.
-
Customer Trust and Bias Concerns: A substantial 63% of consumers express concerns about potential bias and discrimination in AI decision-making. Transparency regarding AI use, data privacy safeguards, and clear communication are essential to foster trust.
-
Integration with Human Agents: AI should augment rather than replace human service representatives. The most effective customer service models combine AI handling of routine tasks with human intervention for complex needs. However, integrating AI tools with existing CRM systems and operational workflows remains a technical and organizational challenge.
-
Security and Compliance: Voice recognition and AI chatbots must comply with stringent regulatory requirements, especially for financial transactions. Robust cybersecurity measures and adherence to regulatory frameworks are vital to prevent fraud and protect customer confidence.
Looking Ahead: The Human-AI Balance
The banking industry is at a pivotal moment where AI can redefine customer service, but success hinges on striking the right balance. The most compelling customer experiences will arise from symbiotic collaboration—where AI delivers scale, speed, and personalization, and human agents provide empathy, problem-solving, and accountability.
As conversational AI matures, banks must invest not only in cutting-edge technology but also in ethical frameworks, staff training, and transparent customer communication. Doing so will elevate AI-powered customer service beyond a cost-saving tool to a genuine competitive differentiator in an increasingly digital and customer-centric market.
Category | Details |
---|---|
Virtual Agents & Predictive Call Routing | AI-powered chatbots (e.g., Erica by Bank of America) handle routine inquiries; predictive routing directs customers to appropriate agents; 37% of customers expected to use virtual assistants by 2025; 85% of IT executives have AI adoption plans. |
Core AI Technologies |
|
Operational Benefits |
|
Limitations & Challenges |
|
Future Outlook | Balance AI scale and speed with human empathy and accountability; invest in ethical frameworks, staff training, and transparent communication to enhance customer experience. |
Benchmarking AI Solutions in Banking: Comparative Analysis of Platforms and Performance
Benchmarking AI Solutions in Banking: Comparative Analysis of Platforms and Performance
How do banks determine which AI platforms genuinely drive progress in automating loans, credit scoring, and customer service? The rapidly evolving landscape of AI solutions presents a complex choice, especially as institutions demand scalability, transparency, seamless integration, and cost efficiency. This section breaks down the leading AI platforms and tools defining banking in 2025, emphasizing the criteria that distinguish meaningful impact from mere hype.
Leading AI Platforms for Loan Automation, Credit Scoring, and Customer Service
Several platforms have risen to prominence by addressing banking’s diverse needs with tailored capabilities:
-
Loan Automation: The ABLE Platform™ and ICE Mortgage Technology’s Encompass lead the loan origination software (LOS) market in 2025. ABLE provides fully customizable loan workflows that automate the entire loan lifecycle, from application to closing. Encompass integrates generative AI to reduce human errors and enhance predictive modeling, particularly in mortgage servicing. Trade Ledger is notable for its ultra-fast time-to-market and “auto-pilot” intelligence, accelerating commercial loan processing through advanced automation.
-
Credit Scoring: AI-powered platforms such as Lendbuzz and Scienaptic AI are transforming risk assessment. Lendbuzz’s proprietary technology aggregates comprehensive borrower financial histories, extending beyond traditional credit bureau data to broaden fair lending opportunities. Scienaptic focuses on AI-driven credit decisioning that offers real-time, granular risk evaluations with an emphasis on model explainability to satisfy regulatory scrutiny. Zest AI and Gaviti complement these efforts by employing machine learning to incorporate alternative data sources like utility payments and rental history, promoting greater financial inclusivity.
-
Customer Service: Banking chatbots have evolved into sophisticated, predictive assistants. Citibank’s AI-powered chatbots, deployed across digital channels, exemplify enhanced customer engagement by efficiently handling routine inquiries and freeing human agents for complex issues. Banco Montepio’s Intelligent Knowledge Management system, developed in partnership with Devoteam, leverages advanced chatbot technology to streamline internal information access and improve employee workflows. Similarly, TD Bank’s AI-driven kiosks enrich in-branch interactions, blending automation with personalized service.
Evaluating AI Solutions: Ease of Deployment, Scalability, and Explainability
Selecting the right AI solution involves balancing technical strength with operational practicality:
-
Ease of Deployment: Platforms offering low-code customization, like Newgen Digital Loan Origination System, enable rapid time-to-market by allowing banks to tailor workflows with minimal coding. Crucially, seamless integration with existing core banking systems mitigates operational disruptions. AI-powered chatbots and virtual assistants must connect smoothly with legacy infrastructure to ensure consistent user experiences and data flows.
-
Scalability: Solutions such as Microsoft’s Intelligent Data Platform and Workiva’s unified data and process management platforms empower banks to extend AI initiatives across multiple departments. Scalability entails managing increasing data volumes and user interactions without performance loss. For example, Upstart’s AI lending marketplace connects thousands of consumers with financial institutions in real time, demonstrating robust scalability in dynamic environments.
-
Model Explainability: Transparency is non-negotiable in regulated domains like credit scoring. Regulatory bodies including the European Central Bank underscore the tradeoff between explainability and complexity, noting that interpretable models may incur additional costs. Platforms like Scienaptic AI prioritize building explainable AI models that meet regulatory standards while maintaining predictive accuracy. Explainability not only ensures compliance but also fosters trust among internal stakeholders and customers.
Integration Capabilities and Cost-Effectiveness
-
Integration: AI platforms that provide comprehensive APIs and pre-built connectors gain a competitive advantage. For instance, Rows Bank Account Integration utilizes Plaid to link live banking data with AI tools, streamlining workflows and enhancing data accuracy. Ocrolus transforms unstructured documents into actionable data, addressing integration challenges inherent in document-intensive lending processes.
-
Cost-Effectiveness: AI-driven automation can reduce operational expenses by up to 22%, representing billions in savings for Tier 1 banks. However, upfront investments in data cleansing, infrastructure upgrades, and talent acquisition remain significant hurdles. Emerging generative AI (GenAI) models demonstrate improved efficiency, lowering resource consumption and making AI adoption more accessible to mid-sized banks and credit unions.
Case Studies: Real-World Strengths and Challenges
Concrete examples highlight the nuanced benefits and limitations of these platforms:
-
JP Morgan Chase deployed AI systems automating loan approvals, achieving reduced processing times and enhanced risk assessment accuracy. Nonetheless, integrating these solutions with legacy systems presented significant challenges.
-
Bank of America’s Erica virtual assistant enriches mobile banking by delivering personalized, context-aware customer interactions, effectively reducing call center volumes through automation of routine queries.
-
Santander’s predictive analytics bolster fraud detection and compliance monitoring but require ongoing model retraining to keep pace with sophisticated threats.
-
SwiftCredit Lending reported a 40% increase in approved loans and a 25% reduction in default rates within six months after adopting AI-driven credit scoring, demonstrating rapid return on investment.
-
Union Financial’s AI-powered customer service platform halved handling times and increased satisfaction scores by 45%, illustrating the operational and experiential gains achievable through effective AI deployment.
Despite these successes, banks face persistent challenges, including shortages of specialized AI talent, maintaining high-quality data, and ensuring transparency to mitigate bias in credit decisions.
Guidance for Banks Evaluating AI Investments
Given the diversity of AI offerings and organizational contexts, banks should consider the following strategic points:
-
Favor platforms with modular, low-code architectures that support rapid customization and smooth integration to reduce deployment friction.
-
Insist on explainability, especially in credit scoring, to comply with regulations and build customer confidence.
-
Assess scalability holistically, encompassing both technical capacity and organizational readiness, including data governance and talent availability.
-
Calculate total cost of ownership, considering infrastructure, maintenance, training, and licensing fees.
-
Leverage peer case studies to identify AI solutions that have demonstrably improved loan processing efficiency, risk management accuracy, or customer satisfaction.
-
Adopt phased implementation approaches, starting with pilot projects that allow iterative refinement before full-scale rollout.
Closing Thoughts
The AI landscape in banking is advancing rapidly, but extracting value requires informed, evidence-based benchmarking. Platforms excelling in deployment speed and integration often face tradeoffs in explainability, while those emphasizing regulatory compliance may demand higher upfront investments. Real-world successes affirm AI’s transformative potential across loan automation, credit scoring, and customer service, yet underscore operational and ethical complexities that banks must navigate carefully.
In 2025, financial institutions that balance opportunity with responsibility—investing strategically, fostering collaboration, and prioritizing transparency—will harness AI’s promise to redefine banking’s future without falling prey to hype or unforeseen risks.
Category | Platform/Tool | Key Features | Strengths | Challenges |
---|---|---|---|---|
Loan Automation | ABLE Platform™ | Fully customizable loan workflows; automates entire loan lifecycle | End-to-end automation, customization | Integration complexity with legacy systems |
ICE Mortgage Technology’s Encompass | Generative AI integration; reduces human errors; predictive modeling | Enhanced mortgage servicing accuracy | Requires advanced AI expertise for maintenance | |
Trade Ledger | Ultra-fast time-to-market; “auto-pilot” intelligence for commercial loans | Accelerated loan processing | Potential scalability limits in complex cases | |
Credit Scoring | Lendbuzz | Aggregates borrower financial history beyond traditional data | Broadened fair lending opportunities | Data sourcing and privacy concerns |
Scienaptic AI | Real-time granular risk evaluation; model explainability | Regulatory compliance and transparency | Higher implementation costs | |
Zest AI | Machine learning with alternative data (utility, rental history) | Financial inclusivity | Data accuracy and integration challenges | |
Gaviti | Alternative data integration; machine learning | Expanded credit access | Model complexity vs explainability | |
Customer Service | Citibank AI Chatbots | AI-powered chatbots for digital channels; handles routine inquiries | Improved customer engagement; frees human agents | Limited handling of complex queries |
Banco Montepio Intelligent Knowledge Management | Advanced chatbot technology for internal workflows | Streamlined employee information access | Requires continuous content updates | |
TD Bank AI-Driven Kiosks | In-branch AI kiosks blending automation & personalized service | Enhanced in-branch experience | High initial investment | |
Ease of Deployment & Scalability | Newgen Digital Loan Origination System | Low-code customization; rapid deployment | Faster time-to-market; minimal coding | May need customization for complex workflows |
Microsoft Intelligent Data Platform | Unified data & process management; scalable | Supports large-scale AI initiatives | Requires skilled IT resources | |
Upstart AI Lending Marketplace | Real-time consumer-institution connections; scalable | Robust scalability in dynamic environments | Dependent on continuous data quality | |
Integration & Cost-Effectiveness | Rows Bank Account Integration (via Plaid) | APIs for live banking data connection | Streamlined workflows; enhanced data accuracy | Dependency on third-party services |
Ocrolus | Transforms unstructured documents into data | Improves document-intensive lending processes | Requires ongoing data validation | |
Case Studies | JP Morgan Chase | AI automates loan approvals; faster processing; better risk assessment | Significant efficiency gains | Legacy system integration difficulties |
Bank of America’s Erica | Virtual assistant for personalized mobile banking interactions | Reduced call center volume; enhanced customer experience | Complex query limitations | |
Santander Predictive Analytics | Fraud detection & compliance monitoring | Improved security and regulatory adherence | Needs ongoing model retraining | |
SwiftCredit Lending | AI credit scoring; 40% more loans approved; 25% default reduction | Rapid ROI; improved risk management | Data quality dependency | |
Union Financial Customer Service Platform | AI-powered service; halved handling times; 45% satisfaction increase | Operational and experiential gains | Continuous training required |
Future Directions and Ethical Considerations: Navigating Opportunities and Risks in AI-Enabled Banking
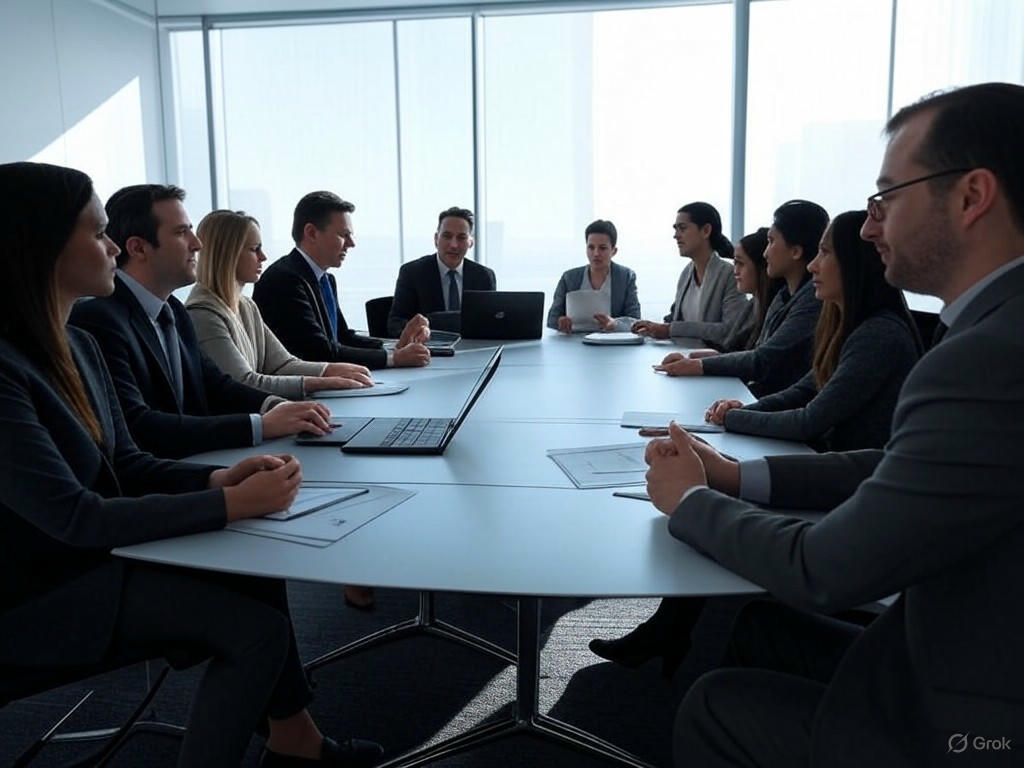
Future Directions and Ethical Considerations: Navigating Opportunities and Risks in AI-Enabled Banking
What will banking look like when AI evolves from a helpful assistant to a strategic partner? The next frontier is already taking shape around generative AI, real-time IoT data integration, and ultra-advanced fraud detection—innovations poised to reshape loans, credit scoring, and customer service. However, this surge in technological capability comes with equally complex regulatory and ethical challenges that banks must carefully navigate.
Emerging AI Technologies: From Automation to Strategic Insight
Generative AI is revolutionizing financial institutions by automating routine processes and unlocking new avenues for value creation. Wells Fargo’s virtual assistant, Fargo, handled 20 million customer interactions in under two years, showcasing how generative AI scales personalized service efficiently. Yet, this is just the beginning.
By 2025, banks are expected to unlock approximately $1 trillion annually through AI-enhanced capabilities across multiple domains:
-
Fraud detection and risk mitigation: Advanced AI models, including those leveraging large language models, identify fraud patterns that evade traditional systems. For example, American Express improved fraud detection by 6% using LSTM-based AI, while platforms like DataDome detect fraudulent activities within milliseconds, providing real-time defense against evolving threats.
-
Real-time IoT data integration: Connected sensors and devices supply banks with continuous data streams, enabling dynamic credit scoring and loan adjustments based on actual asset usage or customer behavior. Telematics data for vehicle loans exemplifies this innovation, allowing risk assessments to reflect real-world conditions.
-
Back-office automation: Generative AI streamlines complex tasks such as document processing, report generation, and content creation, reducing operational costs and human error. Solutions like Fenergo’s Intelligent Document Processing (IDP) can cut manual document handling times by up to 72%, accelerating loan origination.
-
Customer engagement: AI-powered conversational agents provide near-human interaction quality, enhancing digital experiences with personalized, 24/7 support. Virtual assistants like Bank of America’s Erica demonstrate how AI can reduce call center workloads while delivering contextual financial advice.
At the same time, generative AI also equips adversaries with sophisticated tools. Fraudsters now employ AI to fabricate synthetic identities, complicating identity verification and underscoring the need for comprehensive, omnichannel fraud prevention strategies.
Navigating the Regulatory and Cultural Landscape
AI adoption in banking has outpaced regulatory frameworks, resulting in a fragmented compliance environment. Key regulatory developments include the EU AI Act, the General-Purpose AI Code of Practice in Europe, Singapore’s AI risk management guidelines, and evolving U.S. advisory notices. These frameworks emphasize transparency, risk management, and ethical deployment, but differing regional requirements create complexity for multinational banks.
Banks face several critical challenges:
-
Regulatory fragmentation: Inconsistent mandates across jurisdictions and agencies mean banks must continuously adapt to a moving compliance target.
-
Governance and oversight: Boards need to develop AI literacy to clearly define ownership and oversight responsibilities, ensuring AI initiatives align with strategic objectives and ethical standards.
-
Organizational culture: Resistance to AI persists, particularly where innovation appears misaligned with business goals or threatens existing roles. According to research, 80% of AI proofs of concept fail to scale due to data quality or integration issues, highlighting operational barriers.
Leading U.S. banks tend to adopt centralized AI strategies through dedicated Centers of Excellence and research labs, while European institutions often prefer federated, business-line-focused AI initiatives. Regardless of approach, scaling AI from pilot to production remains a significant hurdle.
Ethical Considerations: Building Trust as the Cornerstone
Ethics are foundational to sustainable AI in banking. Customers must understand how AI influences decisions impacting their financial lives, especially in sensitive areas like credit scoring and loan approvals, where bias can lead to unfair outcomes and regulatory scrutiny.
Banks should prioritize:
-
Transparency and explainability: Clear disclosures about AI’s role in decision-making and options for human oversight can help build customer trust. Efforts to develop interpretable AI models and post hoc explanation techniques are gaining traction to meet these needs.
-
Data privacy and cybersecurity: The proliferation of AI models expands attack surfaces, necessitating robust data protection measures. With cybercriminals harnessing AI themselves, financial institutions must cultivate cybersecurity-aware cultures and incorporate privacy-by-design principles aligned with regulations such as GDPR and CCPA.
-
Algorithmic bias: AI trained on incomplete or skewed data risks perpetuating discrimination, undermining fairness and compliance. Bias mitigation strategies, including fairness-aware algorithms and ongoing monitoring, are essential to prevent lending discrimination that disproportionately affects marginalized communities.
-
Workforce impact: AI’s productivity benefits come with workforce transformation. Accenture forecasts a 30% increase in employee productivity by 2028, while half of financial services CEOs report hiring for AI-related roles that didn’t exist a year ago. Banks must invest in reskilling and talent acquisition to balance automation with human capital development.
Strategic Roadmap for Sustainable AI Adoption
To fully harness AI’s potential while mitigating risks, banks should pursue a strategic, phased approach:
-
Modernize IT infrastructure: Upgrading legacy systems to support AI integration ensures smoother transitions and scalability.
-
Implement AI governance frameworks: Establish comprehensive policies covering ethics, compliance, and operational oversight, with active board-level engagement.
-
Foster cross-disciplinary collaboration: Engage technologists, legal experts, ethicists, and business leaders to align AI initiatives with organizational values and market realities.
-
Invest in talent and culture: Prioritize upskilling, change management, and AI fluency to overcome cultural resistance and empower employees.
-
Enhance transparency and customer trust: Communicate AI’s role clearly and implement mechanisms for human intervention and dispute resolution.
Looking Ahead: Building Trust and Creating Long-Term Value
AI’s transformative impact on banking is undeniable, but thoughtful deployment is crucial. The future will favor banks that treat AI as a partnership between human judgment and machine intelligence, recognizing trust as their ultimate competitive advantage.
Successful institutions will:
-
Embrace governance as a strategic enabler rather than a compliance burden.
-
View AI adoption as a cultural journey, not merely a technical upgrade.
-
Balance innovation with ethical stewardship to maintain customer confidence.
In the coming years, AI will not only automate loans and credit scoring but also redefine how banks build relationships, manage risk, and contribute to a fairer financial ecosystem. Navigating this complex landscape requires more than technology—it demands vision, discipline, and an unwavering commitment to responsible innovation.
Category | Details |
---|---|
Emerging AI Technologies |
|
Regulatory and Cultural Challenges |
|
Ethical Considerations |
|
Strategic Roadmap for Sustainable AI Adoption |
|
Future Outlook |
|