Adapting Your Career for AI: Practical Upskilling to Stay Relevant
- Introduction: Navigating the AI Era in Your Career
- Navigating the AI Era in Your Career
- AI as a Transformative Force and Workforce Challenge
- The Value Proposition of Upskilling in AI
- Balancing Enthusiasm with Critical Awareness
- What to Expect from This Guide
- Prerequisites: Foundational Knowledge and Mindset for AI Upskilling
- Prerequisites: Foundational Knowledge and Mindset for AI Upskilling
- Building Core Technical Fluency: Data Literacy and Programming Fundamentals
- Cultivating the Right Mindset: Growth, Curiosity, and Psychological Safety
- Digital Fluency: Navigating AI-Enabled Tools and Environments
- Summary: Your Launchpad for AI Upskilling
- Preparation: Assessing Your Current Skills and Setting Clear Learning Objectives
- Preparation: Assessing Your Current Skills and Setting Clear Learning Objectives
- Conducting a Structured Self-Assessment: What Do You Bring to the Table?
- Setting Personalized Learning Objectives: Aligning Ambition with Reality
- Choosing the Right AI Tools and Platforms: Not All AI Is Created Equal
- Time Management: Building a Realistic, Sustainable Learning Schedule
- Step-by-Step Upskilling Pathways: From Fundamentals to Applied AI Competencies
- Step-by-Step Upskilling Pathways: From Fundamentals to Applied AI Competencies
- Building a Solid Foundation: Grasping Core AI Concepts
- Gaining Practical Skills: From Prompt Engineering to Workflow Integration
- Applying Skills Through Projects and Real-World Challenges
- Blending Learning Modalities: Formal Courses, Peer Learning, and Mentorship
- Tailoring Upskilling for Non-Technical Roles: Translating AI Literacy into Impact
- Embracing Iteration and Lifelong Learning
- Technical Insights: Why Each Upskilling Step Matters and How AI Technologies Function
- Technical Insights: Why Each Upskilling Step Matters and How AI Technologies Function
- Demystifying Large Language Models: What They Are and Why They Matter
- Data Quality: The Foundation of Trustworthy AI
- The Limits of AI: Why Knowing What AI Can’t Do Makes You Smarter
- Connecting Technical Understanding to Practical Upskilling
- Troubleshooting Common Challenges in AI Upskilling and Adoption
- Troubleshooting Common Challenges in AI Upskilling and Adoption
- Navigating Information Overload and Skill Obsolescence
- Overcoming Resistance to Change
- Ethical Dilemmas in AI Adoption
- Aligning AI Upskilling With Business Outcomes
- Evaluating Training Effectiveness and Return on Learning Investment (ROLI)
- Managing Uncertainty and Maintaining Adaptability
- Advanced Strategies and Future-Proofing Your AI Skillset
- Advanced Strategies and Future-Proofing Your AI Skillset
- Diving Deep into Frontier AI Specializations
- Shaping AI Governance: From Policy to Practice
- Riding the Waves of Emerging AI Trends
- Lifelong Learning: The Ultimate Hedge Against Obsolescence
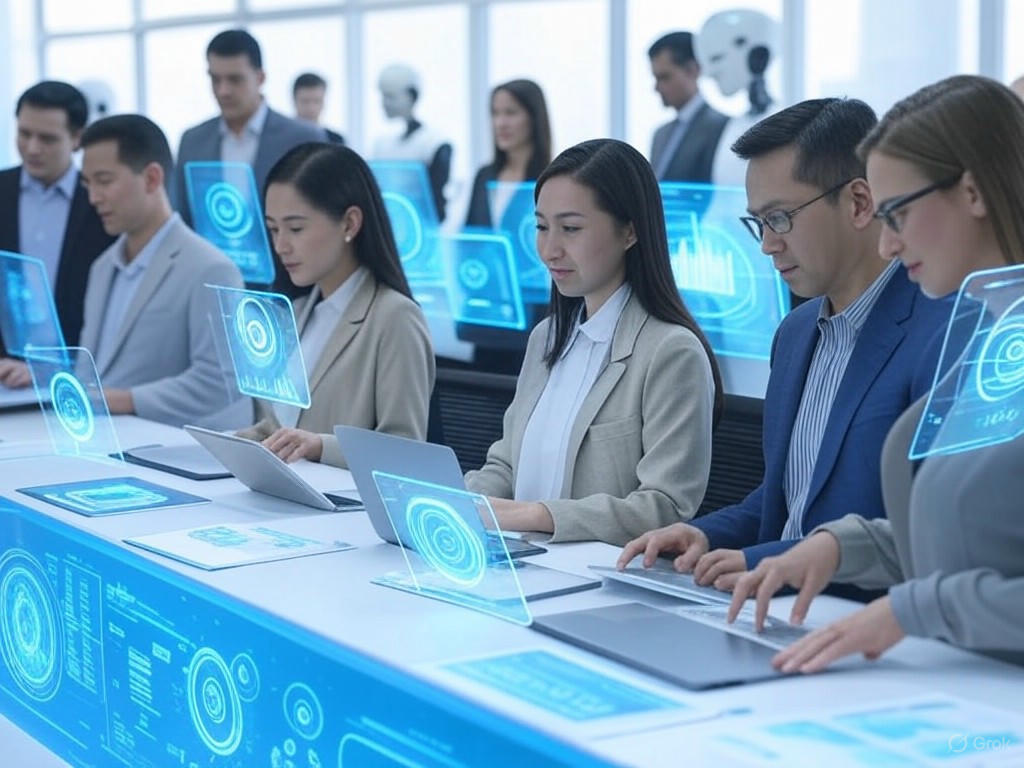
Introduction: Navigating the AI Era in Your Career
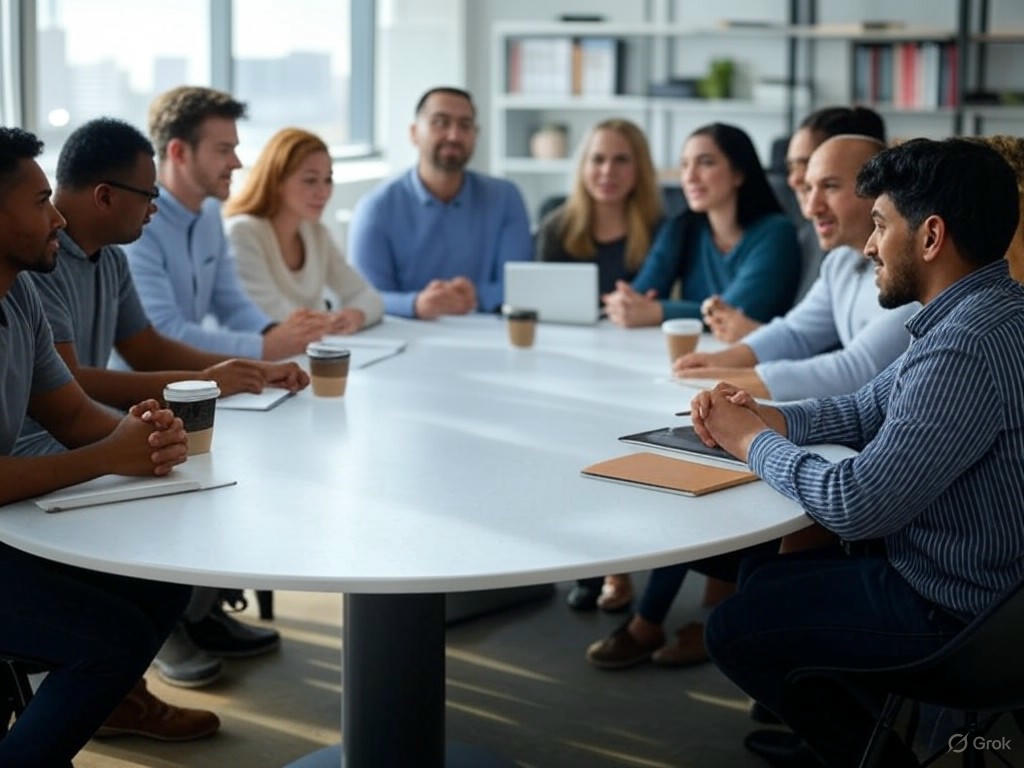
Navigating the AI Era in Your Career
What does it mean to stay relevant in an era where artificial intelligence is reshaping not just industries but the very nature of work? The numbers tell a compelling story: by 2025, approximately 60% of all jobs will have at least 30% of their tasks automated or augmented by AI. This shift is less about disruption and more about reinventing how work gets done.
According to McKinsey, the long-term AI opportunity is valued at an astounding $4.4 trillion, yet only 1% of companies consider themselves mature in AI deployment. This gap between potential and readiness presents both a challenge and a tremendous opportunity for professionals across sectors.
AI as a Transformative Force and Workforce Challenge
AI is not merely automating routine tasks; it is also creating new roles and redefining job responsibilities. For example, human-machine teaming managers are emerging to ensure AI systems augment rather than replace human workers effectively. The World Economic Forum projects a net positive of 12 million new jobs by 2025, with 97 million roles created to offset the displacement of 85 million jobs.
However, these opportunities are unevenly distributed. Entry-level roles, particularly in certain industries and regions, face significant pressure, compelling younger workers to rethink traditional career paths.
Deskless workers—who make up to 80% of the global workforce—are experiencing these shifts firsthand. AI is transforming blue-collar jobs from the ground up, demanding new skills such as critical thinking, software literacy, and equipment maintenance. Organizations must rethink recruitment, training, and job design with a human-in-the-loop mindset to manage this transition smoothly.
The key insight is that thriving in the AI era means developing skills that complement AI, not compete against it. This hybrid intelligence model combines human creativity and judgment with machine speed and data processing. For instance, data analysts use AI tools to uncover deeper insights, while customer service representatives leverage AI agents to tailor interactions personally and efficiently.
The Value Proposition of Upskilling in AI
Why should professionals invest in upskilling around AI? The answer lies in enhancing career resilience, boosting productivity, and unlocking new creative potentials.
Organizations increasingly recognize this imperative: 92% plan to increase AI investments over the next three years, yet only a small fraction consider themselves AI-mature. The demand for AI literacy and complementary skills is growing rapidly but remains underserved.
Upskilling is not solely about acquiring technical expertise. It involves cultivating a mindset oriented toward continuous learning and adaptability. The World Economic Forum notes that 70% of the skills required in jobs today will change by 2030, making digital literacy, critical thinking, and problem-solving foundational capabilities.
For example, AI-powered learning analytics are transforming corporate training by enabling personalized, data-driven development plans that accelerate productivity and innovation.
AI also revolutionizes creativity. From automating mundane tasks to generating novel ideas, AI amplifies human ingenuity when wielded skillfully. This dual benefit—enhanced efficiency and creative augmentation—makes AI upskilling a strategic career investment rather than a survival tactic.
Balancing Enthusiasm with Critical Awareness
While it’s easy to get excited about AI’s transformative potential, a balanced and critical perspective is essential.
AI systems come with limitations and ethical challenges that professionals must understand to use them responsibly. Issues such as algorithmic bias, data privacy, and cybersecurity are not abstract—they have real impacts on business outcomes and societal trust.
For instance, algorithmic bias can perpetuate systemic inequalities if AI models are trained on unrepresentative or prejudiced data. Ethical AI governance requires rigorous testing, diverse datasets, and transparent decision-making processes. Behavioral red lines—rules that prevent AI from autonomously taking harmful actions—are critical to aligning AI with societal norms and safety.
Tech leaders caution that AI can influence human behavior in profound ways, both positively and negatively. Research suggests that while AI integration can enhance capabilities, it may also risk diminishing aspects of human decision-making or creativity if misapplied.
This nuanced reality calls for a mindset that is radically optimistic about AI’s promise but grounded in ethical vigilance and critical scrutiny.
What to Expect from This Guide
This article aims to bridge the gap between AI’s hype and practical reality. It will guide you through:
- Understanding AI’s evolving impact on jobs and industries.
- Preparing technically without needing to become an AI researcher.
- Executing targeted learning strategies through formal courses, certifications, and hands-on projects.
- Cultivating adaptability to keep pace with fast-evolving AI capabilities.
- Maintaining ethical awareness as you integrate AI into your work.
Navigating AI in your career is not about competing against machines but partnering with them intelligently. The future belongs to those who harness AI’s power while upholding human values and creativity.
With over 15 years of experience architecting AI systems, I’ve seen firsthand that success in this era requires both enthusiasm for innovation and a rigorous commitment to thoughtful, ethical engagement. Balancing these will be your greatest asset as you adapt and thrive in the AI-powered workplace.
Topic | Details |
---|---|
AI Job Impact by 2025 | 60% of jobs will have at least 30% of tasks automated or augmented by AI |
AI Economic Opportunity | $4.4 trillion long-term value, only 1% companies AI-mature |
Job Creation vs Displacement | 97 million new jobs created, 85 million jobs displaced, net positive 12 million (World Economic Forum) |
Deskless Workforce Impact | Up to 80% of global workforce affected, new skills needed like critical thinking, software literacy |
Human-AI Collaboration Model | Combines human creativity and judgment with AI speed and data processing |
Organizational AI Investment | 92% plan to increase AI investments in next 3 years, few AI-mature |
Skill Change by 2030 | 70% of current job skills will change; emphasis on digital literacy, critical thinking, problem-solving |
Ethical Concerns | Algorithmic bias, data privacy, cybersecurity, and behavioral impacts require critical awareness |
Career Adaptation Strategies | Technical preparation without deep research, targeted learning, adaptability, ethical awareness |
Prerequisites: Foundational Knowledge and Mindset for AI Upskilling
Prerequisites: Foundational Knowledge and Mindset for AI Upskilling
Before embarking on AI upskilling, it’s essential to establish a solid foundation of both technical knowledge and the right mindset. Think of this as assembling your toolkit: without strong basics, advanced AI skills will be fragile and less effective. In the rapidly evolving AI landscape, grounding yourself in core competencies and psychological readiness is key to staying relevant and impactful.
Building Core Technical Fluency: Data Literacy and Programming Fundamentals
If AI is the new electricity of the 21st century, data literacy is the wiring that powers it. You don’t need to be a data scientist, but understanding data’s role, limitations, and risks is critical. The 2025 State of Data & AI Literacy Report by DataCamp highlights data literacy as the ability to critically and confidently engage with data. This skill enables you to identify AI opportunities, prototype solutions, and contribute meaningfully to AI-driven strategies.
Data literacy is more than just numbers—it’s about interpreting what data reveals and recognizing its blind spots. For example, machine learning models learn only from patterns in their training data, which may embed biases or gaps. A foundational understanding helps you ask informed questions and avoid blind trust in AI outputs.
Alongside data literacy, basic programming skills—especially in Python—form a cornerstone of AI fluency. Python’s simplicity and extensive AI libraries make it the predominant language for AI development. Platforms like DeepLearning.AI and Coursera offer beginner-friendly courses covering Python essentials: variables, control structures, and data manipulation. Learning to use libraries such as pandas for data analysis or TensorFlow and PyTorch for building neural networks empowers you to move from passive consumption to active AI tool creation.
Familiarity with key AI concepts and terminology is equally important. Understanding these terms demystifies AI and positions you to critically evaluate its tools and claims:
- Machine Learning (ML): Algorithms that learn patterns from data without explicit programming.
- Generative AI: Systems capable of creating new content such as text, images, or music based on learned patterns.
- Neural Networks: Computing architectures inspired by the brain’s structure, fundamental to deep learning.
For instance, grasping the difference between supervised learning (requiring labeled data) and unsupervised learning (inferring structure autonomously) clarifies why certain AI applications need human input while others can self-organize.
Cultivating the Right Mindset: Growth, Curiosity, and Psychological Safety
Technical skills alone won’t ensure sustained relevance if your mindset isn’t aligned with the realities of AI evolution. A critical prerequisite is adopting a growth mindset—the belief that abilities can be developed through effort and learning.
AI is inherently experimental. Failure is not an exception but an integral part of learning. Research from GP Strategies emphasizes that a growth mindset encourages trying, failing, and iterating without fear. This attitude transforms setbacks into opportunities for advancement. Leaders who embody this mindset foster innovation-friendly environments that unlock AI’s potential.
Complementing this is the need for psychological safety—feeling secure enough to experiment and fail without fear of punitive consequences. Studies show that workplaces punishing mistakes inhibit creativity and slow AI adoption. Environments where failures can be openly discussed and learned from accelerate organizational AI maturity.
Non-technical traits such as curiosity and critical thinking are equally vital. AI outputs are not infallible; hallucinations and biased recommendations occur. Critical thinking enables you to question AI-generated insights, verify data sources, and make informed decisions. For example, when a generative AI suggests a business insight, it’s your responsibility to validate its plausibility rather than accepting it uncritically.
Finally, ethical awareness is non-negotiable in AI upskilling. AI’s societal impacts—on jobs, privacy, fairness, and governance—are profound. Understanding these helps you anticipate risks and advocate for responsible AI use that aligns with human values and legal frameworks such as the EU AI Act.
Digital Fluency: Navigating AI-Enabled Tools and Environments
Digital fluency transcends basic software use; it embodies agility with evolving digital ecosystems that underpin AI learning and application.
By 2025, digital fluency includes competencies such as:
- Navigating AI-powered learning platforms like adaptive Learning Management Systems (LMS), which tailor content pacing and style to individual learners.
- Collaborating effectively with AI tools integrated into daily workflows—from AI assistants that automate scheduling and code debugging to platforms streamlining routine tasks.
- Understanding cloud-based and edge computing environments where AI models are trained, deployed, and scaled.
EdSurge emphasizes that adaptability in digital fluency is more critical than static knowledge, given rapid technological evolution. Without this agility, even those with solid foundational skills may struggle to keep pace.
Organizations increasingly embed digital fluency as a baseline expectation. The Air Education and Training Command (AETC), for example, integrates data literacy into performance metrics and mandates AI training for senior staff, signaling its growing importance in workforce readiness.
Summary: Your Launchpad for AI Upskilling
To effectively upskill in AI and thrive amid technological transformation, ensure you cultivate:
- Data literacy: Ability to read, interpret, and critically engage with data.
- Basic programming skills: Proficiency in Python to understand and build AI models.
- Familiarity with AI concepts: Understanding machine learning, generative AI, neural networks, and related terminology.
- A growth mindset and psychological safety: Openness to experimentation, failure, and continuous learning.
- Curiosity and critical thinking: Questioning AI outputs and appreciating ethical implications.
- Digital fluency: Comfort with AI-enabled tools, adaptive learning platforms, and cloud/edge environments.
These prerequisites form a robust launchpad, equipping you not just to survive but to lead and innovate in the AI era. Without this foundation, the AI revolution risks overwhelming you rather than empowering your career trajectory.
Prerequisite | Description | Key Points / Examples |
---|---|---|
Data Literacy | Ability to critically and confidently engage with data, understanding its role, limitations, and risks. |
– Interpret data insights and recognize blind spots – Awareness of biases in training data – Identify AI opportunities and prototype solutions |
Basic Programming Skills | Proficiency in Python for AI development and data manipulation. |
– Learn variables, control structures, data manipulation – Use libraries like pandas, TensorFlow, PyTorch – Move from passive consumption to AI tool creation |
Familiarity with AI Concepts | Understanding core AI terms and architectures. |
– Machine Learning: algorithms learning from data – Generative AI: creating new content – Neural Networks: brain-inspired computing – Supervised vs unsupervised learning |
Growth Mindset & Psychological Safety | Openness to learning through experimentation and failure in a supportive environment. |
– Embrace failure as part of learning – Encourage innovation-friendly workplace culture – Psychological safety to experiment without fear |
Curiosity & Critical Thinking | Question AI outputs and verify insights for informed decision-making. |
– Detect hallucinations and bias in AI – Validate AI-generated business insights – Ethical awareness regarding AI’s societal impact |
Digital Fluency | Agility with AI-enabled tools, adaptive platforms, and computing environments. |
– Navigate AI-powered learning platforms (adaptive LMS) – Collaborate with AI assistants and automation tools – Understand cloud and edge computing for AI deployment |
Preparation: Assessing Your Current Skills and Setting Clear Learning Objectives
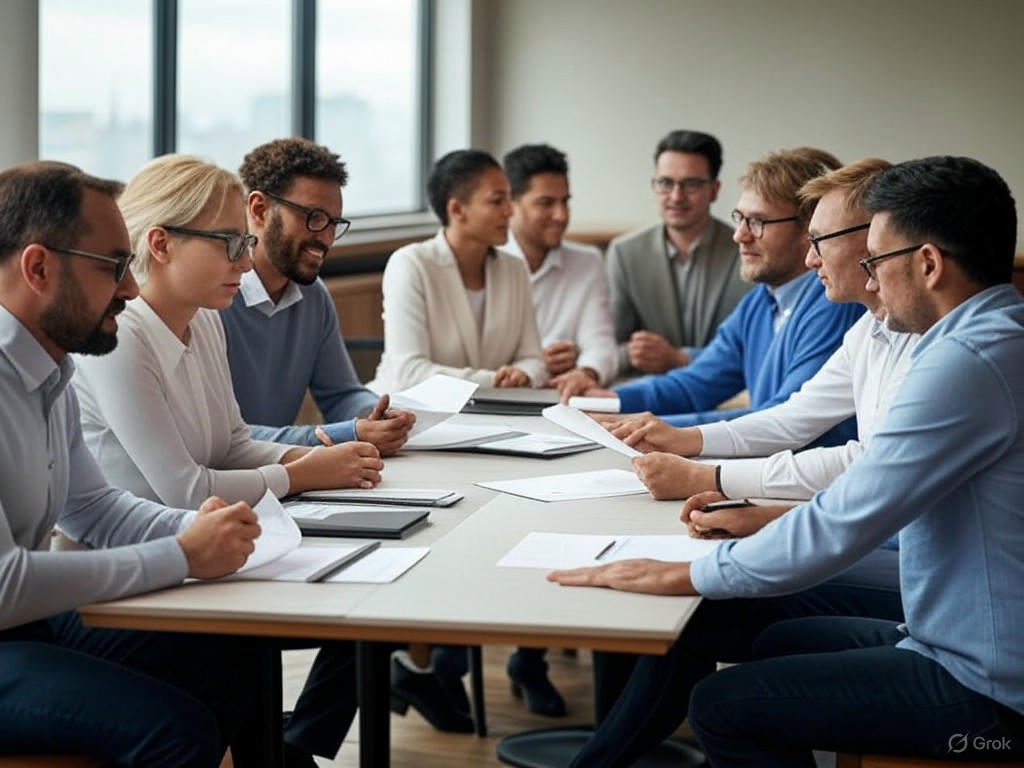
Preparation: Assessing Your Current Skills and Setting Clear Learning Objectives
Adapting your career to thrive in an AI-driven world begins with a clear, honest evaluation of your current skills, knowledge gaps, and how these align with the AI-enabled future you envision. This foundational step sets the stage for purposeful upskilling and sustained relevance.
Conducting a Structured Self-Assessment: What Do You Bring to the Table?
Before pursuing AI training or certifications, it is essential to take stock of your capabilities across three pivotal dimensions:
-
Technical Skills
These encompass coding languages such as Python and R, fundamental for AI roles. Mastery of statistics, data handling, and familiarity with AI frameworks is equally important. According to DataCamp’s 2025 Skill Map, essentials include understanding machine learning algorithms, deep learning, and data manipulation. If you belong to software or data professionals (classified as Category 1 talent in the global AI workforce taxonomy), assess your proficiency against these benchmarks. -
Domain Expertise
AI’s effectiveness depends heavily on contextual knowledge. Whether in healthcare, finance, or logistics, domain expertise enables the development of defensible, accurate AI models. Dr. Janna Lipenkova emphasizes that domain experts contribute significantly through data annotation, synthetic data generation, and prompt fine-tuning. Reflect on how your specialized knowledge can complement AI capabilities to enhance outcomes. -
Soft Skills
As AI automates technical tasks, human-centric skills such as creativity, empathy, judgment, and adaptability gain prominence. Research forecasts that by 2027, nearly half of workers’ core skills will be disrupted, with behavioral skills bridging the human-AI collaboration gap. Evaluate your communication, problem-solving, and emotional intelligence—these competencies will underpin your ability to collaborate effectively within AI-augmented teams.
Taking a structured, data-driven approach to self-assessment is not merely administrative; it uncovers your unique strengths and areas for growth. Leverage AI-powered skills assessments and platforms like LinkedIn Learning or Coursera to complement your introspection with objective insights.
Setting Personalized Learning Objectives: Aligning Ambition with Reality
With a clear understanding of your current profile, you can now chart a targeted learning path. The secret lies in crafting specific, measurable, and relevant goals aligned with your career aspirations and market dynamics.
-
Define Clear, Measurable Goals
Adopt the SMART framework—Specific, Measurable, Achievable, Relevant, Time-bound. For instance, rather than vague intentions, set a goal like: “Gain proficiency in Python for data analysis using pandas and NumPy within three months.” -
Consider Your Career Stage and Role Aspirations
Are you pursuing a path as an AI researcher, machine learning engineer, or a domain expert integrating AI tools? Each requires distinct levels of technical mastery, strategic insight, and ethical oversight. The 2025 AI Jobs Report highlights diverse roles from prompt designers to AI ethics leads, reflecting the expanding landscape of AI careers. -
Stay Informed on Market Trends
AI job markets are dynamic and regionally nuanced. Europe leads in female AI talent and specialized Category 2 roles, while U.S. coastal hubs dominate AI hiring. Recognizing these trends enables realistic and strategic objective setting. -
Leverage AI to Craft and Refine Objectives
Generative AI tools can assist in articulating learning goals, suggesting metrics, and creating assessment rubrics. This AI-assisted approach streamlines your learning plan development, aligning it with best practices and evolving standards.
Choosing the Right AI Tools and Platforms: Not All AI Is Created Equal
Selecting AI platforms and tools that align with your learning needs and organizational context is crucial for effective upskilling.
-
IBM watsonx™
Adopted by over 77 companies globally, watsonx™ offers comprehensive capabilities for model training, tuning, and deployment. Its blend of institutional knowledge with cutting-edge AI makes it ideal for enterprises seeking robust end-to-end workflows. -
OpenAI’s ChatGPT and Microsoft Copilot
These conversational AI tools have become staples in business workflows. Microsoft Copilot’s integration within Microsoft 365 enables seamless productivity enhancements, while ChatGPT offers versatile natural language processing for diverse applications. -
Google Gemini
For organizations embedded in Google’s ecosystem, Gemini provides advanced, business-aware AI assistance leveraging Google’s data infrastructure to enhance operational intelligence. -
Other Notables: Claude and DataRobot
Claude excels in regulated industries due to its safety mechanisms and ability to handle large context windows, making it suitable for complex document analysis and compliance-heavy environments.
When evaluating tools, consider:
-
Performance and Flexibility
Does the platform support your specific learning objectives—be it simulation, model tuning, or prompt engineering? -
Community and Support
Active user communities enrich learning through shared resources and troubleshooting. -
Organizational Alignment
Tools that integrate with your workplace’s AI maturity and existing technology stack ensure practical applicability and smoother adoption.
Time Management: Building a Realistic, Sustainable Learning Schedule
A well-crafted learning plan requires disciplined execution. Upskilling in AI is a marathon, not a sprint.
-
Prioritize Regular Learning Blocks
Allocate consistent, focused time slots weekly. Research favors short, frequent sessions (30–60 minutes daily) over infrequent, extended ones for better retention. -
Leverage AI to Optimize Your Schedule
AI-powered learning platforms adapt content delivery to your pace and style, enhancing efficiency. -
Balance Learning with Workload
Integrate AI skill development into your daily tasks. Applying AI tools on real projects reinforces learning and demonstrates tangible value to your organization. -
Set Milestones and Reflect
Regularly review your progress against objectives. Be flexible to refine goals and timelines as you gain insights or as market conditions evolve.
Adapting to AI in your professional journey demands more than enthusiasm; it requires a rigorous, evidence-based approach to evaluate your current skills, define purposeful learning objectives, and select appropriate tools—all while managing your time realistically. By embracing this precision, you position yourself not only to survive but to thrive in the AI era.
Dimension | Key Components | Details and Examples | Resources and Tools |
---|---|---|---|
Technical Skills | Coding languages, statistics, AI frameworks | Python, R, machine learning algorithms, deep learning, data manipulation; Category 1 talent benchmarks | DataCamp 2025 Skill Map, AI-powered skills assessments |
Domain Expertise | Contextual knowledge in specific fields | Healthcare, finance, logistics; contributions via data annotation, synthetic data generation, prompt fine-tuning | Subject matter experts, domain-specific AI applications |
Soft Skills | Human-centric behavioral competencies | Creativity, empathy, judgment, adaptability, communication, problem-solving, emotional intelligence | Behavioral skills assessments, collaboration tools |
Aspect | Description | Examples / Notes |
---|---|---|
Define Clear, Measurable Goals | Use the SMART framework to set learning objectives | Example: “Gain proficiency in Python for data analysis using pandas and NumPy within three months.” |
Consider Career Stage and Role Aspirations | Align goals with targeted AI roles and required skills | AI researcher, machine learning engineer, domain expert; roles like prompt designers and AI ethics leads |
Stay Informed on Market Trends | Understand regional and sector-specific AI job market dynamics | Europe leads in female AI talent; U.S. coastal hubs dominate AI hiring |
Leverage AI Assistance | Use generative AI tools to create and refine learning plans | Helps articulate goals, suggest metrics, and develop assessment rubrics |
AI Tool / Platform | Description | Ideal Use Case | Considerations |
---|---|---|---|
IBM watsonx™ | Comprehensive model training, tuning, and deployment platform | Enterprise-level end-to-end AI workflows | Integration with institutional knowledge; robust capabilities |
OpenAI’s ChatGPT and Microsoft Copilot | Conversational AI and productivity enhancement tools | Business workflows, natural language processing | Integration within Microsoft 365; versatile applications |
Google Gemini | Advanced AI assistance within Google’s ecosystem | Organizations using Google’s data infrastructure | Business-aware AI, operational intelligence enhancement |
Claude | AI suited for regulated industries with safety and compliance features | Complex document analysis, compliance-heavy environments | Large context windows, safety mechanisms |
DataRobot | AI platform for automated machine learning and model deployment | Data science automation and rapid prototyping | Focus on ease of use and scalability |
Strategy | Details | Benefits |
---|---|---|
Prioritize Regular Learning Blocks | Schedule consistent, focused sessions weekly; favor short, frequent learning (30–60 min daily) | Improves retention and steady progress |
Leverage AI to Optimize Schedule | Use AI-powered platforms that adapt to your pace and learning style | Enhances learning efficiency and personalization |
Balance Learning with Workload | Integrate AI skill development into daily work tasks | Reinforces learning and shows organizational value |
Set Milestones and Reflect | Regularly review progress and adjust goals/timelines as needed | Maintains motivation and alignment with market changes |
Step-by-Step Upskilling Pathways: From Fundamentals to Applied AI Competencies
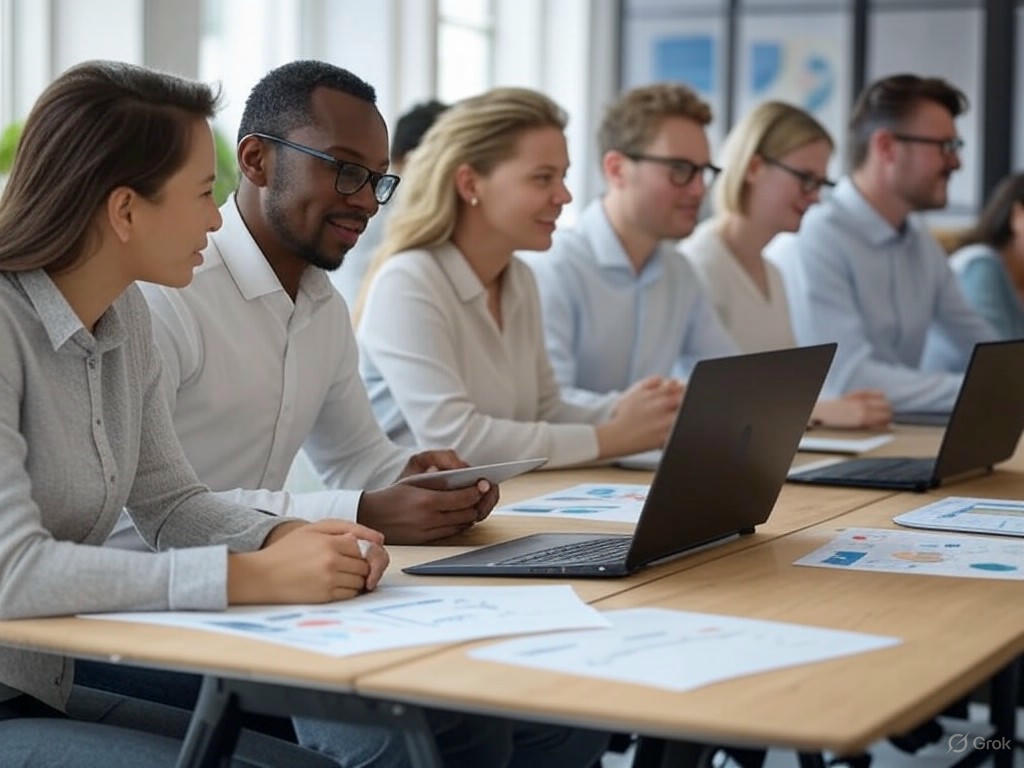
Step-by-Step Upskilling Pathways: From Fundamentals to Applied AI Competencies
How do you transform curiosity about AI into confident use—or even creation—of AI-powered solutions at work? Achieving AI fluency is a deliberate, phased journey: start by building a strong foundation, then gain hands-on experience, and finally apply your skills in real-world projects. This stepwise approach prevents overwhelm and ensures your learning translates into meaningful workplace impact.
Building a Solid Foundation: Grasping Core AI Concepts
Before diving into AI tools, it’s essential to understand fundamental AI principles. Artificial Intelligence is a broad field encompassing machine learning (ML), deep learning (DL), natural language processing (NLP), and emerging areas like generative AI. Think of these concepts as the grammar of a new language you’re learning before writing poetry.
Key foundational topics include:
-
AI Subfields: Machine learning enables systems to learn patterns from data without explicit programming. Deep learning uses neural networks to handle complex data types such as images, video, or text.
-
Types of Learning: Supervised learning uses labeled data, unsupervised learning discovers hidden structures in unlabeled data, and reinforcement learning optimizes decision-making through trial and error.
-
Programming and Math Basics: Python is the lingua franca of AI, supported by libraries like TensorFlow and PyTorch. While coding mastery isn’t mandatory for everyone, familiarity with these tools and concepts enhances your ability to engage with AI meaningfully.
-
Ethical Considerations: AI systems can inherit biases from their training data and creators. Recognizing these limitations helps you use AI responsibly and advocate for fairness.
Platforms such as DataCamp and DeepLearning.AI offer beginner-friendly courses in Python fundamentals and data manipulation. Complement structured learning by following AI blogs, reading accessible research summaries, and participating in communities like AI Village or Kaggle. The goal is to develop a mental model of AI’s capabilities and constraints—not to become a data scientist overnight.
Gaining Practical Skills: From Prompt Engineering to Workflow Integration
Understanding theory is one thing; applying AI tools effectively at work is another. Practical competencies like prompt engineering—crafting precise inputs to generative AI models such as ChatGPT—are increasingly vital. However, as AI interfaces grow more intuitive, the role of prompt engineering evolves toward guiding AI behavior contextually, emphasizing roles like AI trainers or specialists.
Best practices for effective prompt engineering include:
-
Using clear, succinct language tailored to the specific task.
-
Employing chain-of-thought prompting, where prompts guide AI reasoning stepwise.
-
Iteratively refining prompts based on AI outputs to improve accuracy.
Beyond prompt crafting, leveraging generative AI to automate repetitive tasks boosts productivity. For example, Microsoft 365 Copilot automates workflows, allowing employees to focus on strategic activities rather than administrative drudgery. Companies like AT&T and Balfour Beatty have reported saving several hours weekly through such AI integration.
Autonomous AI agents represent the next frontier—semi-independent systems capable of performing complex tasks with minimal human oversight. JPMorgan Chase’s COIN platform, which annually saves 360,000 hours by automating loan agreement analysis, exemplifies this trend. AI agents are increasingly integrated into finance, HR, and customer service workflows to enhance efficiency.
To build these practical skills, start experimenting with familiar tools like ChatGPT or Microsoft Copilot for tasks such as drafting emails or summarizing data. Gradually explore AI agents tailored to your industry to identify opportunities for automation, freeing time for higher-value work.
Applying Skills Through Projects and Real-World Challenges
Knowledge and tool familiarity solidify when applied in projects. Hands-on experience reveals knowledge gaps, builds confidence, and demonstrates AI’s business value.
Beginner projects might include:
-
Classifying animal species using image datasets.
-
Detecting pneumonia from X-ray images with deep learning.
-
Creating sentiment analyzers for social media comments.
More advanced initiatives could involve embedding AI into business processes, such as automating lead scoring or generating customer support responses with conversational AI.
Selecting projects relevant to your role maximizes impact. For non-technical professionals, focus on how AI augments decision-making or customer interactions rather than building models from scratch. For example, marketing teams might use generative AI to draft content, while finance professionals could pilot AI agents for invoice processing.
Blending Learning Modalities: Formal Courses, Peer Learning, and Mentorship
No single learning method suffices for AI mastery. A successful upskilling program combines formal coursework, peer collaboration, and real-world application. Given AI’s complexity, this ecosystem supports ongoing engagement and continuous feedback.
-
Formal Courses: Platforms like Coursera and DataCamp offer structured AI certificates and skill tracks with guided exercises.
-
Peer Learning: Collaborative environments such as AI Village, Kaggle, or workplace study groups foster discussion, clarify doubts, and build psychological safety. Initiatives like Polaris Movement’s mentorship model demonstrate how juniors and seniors can co-create knowledge, accelerating skill transfer and leadership development.
-
Real-World Projects: Applying skills in your daily work provides immediate feedback and reinforces learning while showcasing tangible value to stakeholders.
-
Continuous Feedback Loops: Modern performance management favors ongoing feedback over annual reviews. AI-powered learning analytics track progress, recommend targeted resources, and visualize skill development, making upskilling an iterative, dynamic process.
Tailoring Upskilling for Non-Technical Roles: Translating AI Literacy into Impact
AI literacy benefits everyone, not just coders. Understanding AI’s capabilities and limitations enables you to:
-
Identify tasks ripe for AI augmentation.
-
Collaborate effectively with technical teams.
-
Advocate for ethical and responsible AI deployment.
-
Innovate workflows by combining domain expertise with AI tools.
For instance, a project manager might use AI to automate meeting summaries and risk assessments, while a sales professional could leverage AI-driven lead scoring to prioritize prospects.
Low-code and no-code AI platforms further democratize access, empowering domain experts to build AI applications without deep programming skills. This inclusive approach aligns with the hybrid intelligence model, where human insight augments AI capabilities.
Embracing Iteration and Lifelong Learning
AI technologies evolve rapidly, demanding continuous skill development. Upskilling is not a one-off event but an ongoing journey. Embrace iterative learning—try, fail, adjust, and improve.
Practical strategies include:
-
Allocating regular weekly time blocks (e.g., 30 to 60 minutes daily) for AI skill development.
-
Participating in AI-focused workshops, hackathons, or peer study groups.
-
Sharing insights and lessons learned with your team to foster a culture of collective growth.
By adopting this phased, practical, and blended learning approach, you position yourself to thrive in the AI era—turning potential disruption into opportunity and becoming a confident partner to AI in your career.
Upskilling Stage | Focus Areas | Key Topics & Activities | Recommended Resources & Examples |
---|---|---|---|
Building a Solid Foundation | Core AI Concepts | AI Subfields (ML, DL, NLP, Generative AI); Types of Learning (Supervised, Unsupervised, Reinforcement); Programming & Math Basics (Python, TensorFlow, PyTorch); Ethical Considerations | Courses: DataCamp, DeepLearning.AI; Communities: AI Village, Kaggle; AI blogs and accessible research summaries |
Gaining Practical Skills | Prompt Engineering & Workflow Integration | Prompt engineering best practices (clear language, chain-of-thought prompting, iterative refinement); Using generative AI to automate tasks; Exploring autonomous AI agents | Tools: ChatGPT, Microsoft 365 Copilot; Company examples: AT&T, Balfour Beatty, JPMorgan Chase COIN platform |
Applying Skills Through Projects | Hands-on AI Projects | Beginner projects (image classification, pneumonia detection, sentiment analysis); Advanced projects (lead scoring automation, conversational AI for support); Role-relevant project selection | Marketing use: content drafting; Finance use: AI agents for invoice processing |
Blending Learning Modalities | Formal, Peer, & Real-World Learning | Formal courses with certificates; Peer collaboration and mentorship; Real-world projects; Continuous AI-powered feedback loops | Platforms: Coursera, DataCamp; Communities: AI Village, Kaggle; Mentorship: Polaris Movement; AI learning analytics tools |
Tailoring for Non-Technical Roles | AI Literacy & Impact | Identifying AI augmentation opportunities; Collaborating with technical teams; Ethical AI advocacy; Using low-code/no-code AI platforms | Examples: Project managers automating summaries; Sales using AI lead scoring; Hybrid intelligence model |
Embracing Iteration & Lifelong Learning | Continuous Skill Development | Regular practice (30-60 mins daily); Participating in workshops, hackathons, study groups; Sharing knowledge to foster team growth | Strategies: Scheduled learning time; Peer learning events; Culture of collective growth |
Technical Insights: Why Each Upskilling Step Matters and How AI Technologies Function
Technical Insights: Why Each Upskilling Step Matters and How AI Technologies Function
Understanding the inner workings of AI fundamentally changes how you engage with these tools in your professional life. In today’s AI era, grasping the core concepts behind large language models (LLMs), appreciating the critical role of data quality, and recognizing the inherent limitations of AI systems are not just academic exercises—they are practical necessities. These insights empower you to use AI tools more effectively, anticipate potential pitfalls, and make ethical decisions grounded in reality rather than hype.
Demystifying Large Language Models: What They Are and Why They Matter
At the heart of many AI tools reshaping workplaces are large language models such as GPT-4, Google’s Gemini, and open-source variants like Llama and Vicuna. These models function as massive pattern recognizers, trained on vast amounts of text data to generate coherent, contextually relevant language.
Think of LLMs as highly skilled predictive text engines. When you input a prompt, the model doesn’t “know” facts like a human but predicts the most probable next word based on learned patterns. This prediction mechanism, enabled by the transformer architecture introduced in 2014, allows the generation of surprisingly fluent and context-aware responses.
Understanding this mechanism is crucial for practical AI use. For example:
-
Multimodal Capabilities: Gemini models can interpret images, audio, and video alongside text, enabling richer interactions beyond plain language. This expands possibilities in creative and analytical tasks.
-
Autonomous Agents: Emerging AI agents perform complex sequences of tasks with minimal human intervention, transforming traditional workflows.
-
Limitations in Reasoning: Although LLMs mimic some reasoning patterns, their process is statistical, not cognitive. They do not truly “understand” meaning but approximate it through token patterns.
By appreciating these mechanics, you avoid overestimating AI’s “intelligence.” This knowledge helps you craft precise prompts, critically interpret AI outputs, and integrate AI tools as collaborative partners rather than infallible authorities.
Data Quality: The Foundation of Trustworthy AI
If LLMs are the engines powering AI, data is their fuel—and not just any fuel, but premium-grade quality. The accuracy, reliability, and fairness of AI systems hinge directly on the quality of their training data.
Poor or biased data leads to flawed outputs, which can cascade into real-world harm. Key reasons why data quality must be a focus in your upskilling journey include:
-
Bias Mitigation: AI models inherit biases present in their training data. For instance, gender bias in healthcare AI can cause misdiagnoses by favoring male symptom profiles. Using diverse, representative datasets helps reduce such systemic errors.
-
Error Reduction: Inconsistent or incomplete data can cause AI hallucinations—confident but factually incorrect outputs. This remains a significant challenge in current LLMs, requiring careful data curation and validation.
-
Ethical Responsibility: Data privacy demands rigorous governance. Organizations must balance the need for rich datasets with compliance to regulations like GDPR and CCPA, and respect for individual rights.
-
Continuous Curation: High-quality data management is an ongoing commitment involving organizing, cleaning, labeling, and monitoring datasets over time.
By learning about data curation, bias detection, and privacy-preserving techniques such as federated learning, professionals contribute to building AI systems that are effective, ethical, and trustworthy.
The Limits of AI: Why Knowing What AI Can’t Do Makes You Smarter
It’s tempting to view AI as a magic wand solving all problems instantly. The reality is far more nuanced. Even the most advanced LLMs and AI systems have clear limitations that must inform how you adopt and integrate them.
Key limitations to keep in mind include:
-
Algorithmic Bias: AI can perpetuate or amplify societal inequalities if not carefully audited and managed. This necessitates ongoing human oversight and adherence to ethical guidelines.
-
Lack of True Creativity and Understanding: AI generates outputs based on learned patterns without genuine innovation or comprehension. This restricts its use in tasks requiring deep insight or moral judgment.
-
Interpretability Challenges: Many AI models operate as “black boxes,” making it difficult to trace how outputs are derived. Explainable AI (XAI) techniques like LIME and SHAP provide transparency but are still evolving.
-
Resource and Environmental Costs: Training and running large AI models consume significant energy, raising sustainability concerns organizations must weigh.
-
Security Risks: AI systems are vulnerable to adversarial attacks, data poisoning, and other exploits that can degrade performance or induce harmful behaviors.
Awareness of these constraints enables you to set realistic expectations, advocate for responsible AI policies, and design workflows that leverage AI’s strengths while mitigating risks.
Connecting Technical Understanding to Practical Upskilling
Why invest time in unpacking these technical layers? Because each upskilling step—from understanding how LLMs generate text, to mastering data quality principles, to grasping AI’s limitations—translates directly into smarter, safer, and more ethical AI usage.
-
Better Tool Use: Understanding AI models allows you to craft precise prompts, critically evaluate outputs, and integrate AI tools to amplify your expertise rather than replace it.
-
Ethical Judgment: Knowledge of bias origins and data curation practices empowers advocacy for fairness and transparency, essential for sustaining trust among colleagues and clients.
-
Future-Proofing: Foundational understanding equips you to adapt to evolving AI modalities and capabilities, such as multimodal AI and autonomous agents, without being caught off guard.
In essence, upskilling is not merely about proficiency with tools; it’s about cultivating a mindset attuned to AI’s possibilities and pitfalls. This balanced perspective distinguishes effective AI adopters from passive users caught in hype cycles.
By anchoring your AI journey in these technical realities, you position yourself not just as a user but as an informed steward of AI’s transformative potential—ready to harness its power responsibly and innovatively in your professional life.
Aspect | Key Points | Practical Implications |
---|---|---|
Large Language Models (LLMs) |
|
|
Data Quality |
|
|
Limits of AI |
|
|
Practical Upskilling Benefits |
|
|
Troubleshooting Common Challenges in AI Upskilling and Adoption
Troubleshooting Common Challenges in AI Upskilling and Adoption
Despite substantial investments in AI, mastering its integration remains elusive for many organizations and individuals. The McKinsey 2025 report reveals a striking disconnect: nearly all companies are investing heavily in AI, yet only 1% consider themselves mature adopters. This maturity gap underscores that successful AI adoption—and the upskilling that underpins it—is hindered by complex technical, cultural, and ethical challenges.
Navigating Information Overload and Skill Obsolescence
AI advances at a breakneck pace, creating a double-edged sword for learners and organizations alike. The abundance of learning resources comes with a flood of often conflicting or outdated information, which can overwhelm and exhaust learners.
An effective strategy to counteract this is incremental learning. Breaking AI education into manageable, targeted modules prevents burnout and sustains engagement. AI-powered learning platforms such as Docebo and 360 Learning exemplify this approach by personalizing learning paths. These platforms analyze learner progress and skill gaps to recommend the most relevant next steps, thereby reducing information overload.
Closely tied to this is the issue of skill obsolescence. AI capabilities evolve so rapidly that yesterday’s expertise can quickly become outdated. Organizations must cultivate a culture of continuous learning rather than treating upskilling as a one-off event. Agile AI strategies that tightly integrate training with real-world application enable teams to adapt swiftly and maintain relevant skills.
Overcoming Resistance to Change
Resistance to AI adoption often stems less from technical hurdles and more from psychological barriers. Research by Julian De Freitas highlights prevalent fears among employees, including job insecurity, loss of autonomy, and distrust of AI’s opaque decision-making processes. Many perceive AI as emotionless and rigid, fueling reluctance to embrace it fully.
Building psychological safety is critical to overcoming these fears. When employees feel safe to experiment, ask questions, and fail without judgment, they are far more likely to engage with AI tools. Leaders play a pivotal role by fostering open dialogue and emphasizing that AI is designed to augment—not replace—human skills.
Leveraging peer networks also mitigates resistance. Communities like AI Village and Kaggle offer collaborative environments where learners share experiences, reducing isolation and normalizing the learning curve.
Additionally, focusing on small wins can build confidence incrementally. Introducing AI through low-risk, personal tasks helps employees develop familiarity and reduce anxiety. This mirrors consumer-facing examples like Amazon’s Alexa, which anthropomorphizes AI to make it more approachable and less intimidating.
Ethical Dilemmas in AI Adoption
Ethical considerations have moved from the periphery to the core of sustainable AI integration. Issues such as algorithmic bias, privacy, and transparency demand deliberate attention. A PwC survey found that although 73% of U.S. companies have adopted AI, many overlook these ethical imperatives, risking long-term trust and compliance.
To navigate ethical challenges, organizations should establish clear ethical guidelines aligned with their values and business goals. This human-centric approach acknowledges that AI systems inherit human biases and that transparency about AI’s limitations fosters trust both internally and externally.
Practical measures include:
- Diversifying training data to minimize bias
- Ensuring compliance with privacy standards like GDPR and CCPA
- Involving cross-disciplinary teams—including ethicists, domain experts, and legal advisors—in AI development and deployment
Embedding ethical AI governance frameworks supports accountability and aligns AI initiatives with emerging regulations such as the EU AI Act.
Aligning AI Upskilling With Business Outcomes
AI upskilling efforts that are not aligned with clear business objectives often fail to generate meaningful impact. McKinsey’s research underscores that organizations linking AI training directly to measurable outcomes—such as productivity gains, innovation, or safety improvements—experience greater success.
Google’s AI Works pilot serves as a compelling example. Targeted training empowered frontline workers to save an average of 122 hours annually on routine tasks, demonstrating tangible returns on upskilling investments.
To replicate such success, leaders should:
- Define relevant KPIs before launching AI upskilling programs
- Monitor metrics such as task efficiency, error rates, and employee engagement with AI tools
- Communicate the value and impact of AI skills clearly to motivate continuous learning
This strategic alignment ensures AI upskilling translates into operational benefits and sustained adoption.
Evaluating Training Effectiveness and Return on Learning Investment (ROLI)
Measuring the impact of AI training is essential yet challenging. Traditional assessments like quizzes often fail to capture real-world application or behavioral change.
Emerging best practices involve using AI-powered learning analytics to track not only knowledge acquisition but also practical application on the job. Modern platforms provide dashboards that display personalized progress, engagement levels, and skill mastery, enabling dynamic course adjustments and targeted support.
Calculating Return on Learning Investment (ROLI) should encompass immediate productivity improvements and long-term adaptability gains. According to Boston Consulting Group (BCG), organizations that integrate AI into their learning cultures become more resilient amidst uncertainty.
Continuous evaluation helps justify investments, identify gaps, and refine training programs to maximize organizational value.
Managing Uncertainty and Maintaining Adaptability
The rapid evolution of AI guarantees ongoing uncertainty. Organizations must prepare for shifting AI capabilities and emerging technologies by fostering organizational learning agility.
Successful companies combine AI-powered learning with flexible, iterative strategies, encouraging:
- Continuous experimentation and innovation
- Breaking down silos to promote cross-functional knowledge sharing
- Embracing open-source AI frameworks and active participation in AI communities
This adaptability is exemplified by “AI natives” who view agility as a competitive advantage rather than a survival necessity.
By cultivating a culture that values continuous learning and psychological safety, organizations and individuals can navigate AI’s uncertainties proactively and thrive in the evolving landscape.
In summary, overcoming common challenges in AI upskilling and adoption requires a holistic approach that balances technical mastery with psychological safety, ethical rigor, and strategic alignment. Organizations and professionals who invest thoughtfully in these areas will not only stay relevant but excel in the unfolding AI era.
Challenge | Issues | Strategies & Solutions | Examples / Tools |
---|---|---|---|
Navigating Information Overload and Skill Obsolescence |
– Flood of conflicting/outdated information – Rapid skill obsolescence due to AI evolution |
– Incremental learning with targeted modules – Personalized learning paths to reduce overload – Cultivating continuous learning culture – Agile AI strategies integrating training with real-world application |
– AI-powered platforms: Docebo, 360 Learning |
Overcoming Resistance to Change |
– Psychological barriers: job insecurity, loss of autonomy, distrust – Fear of AI’s opaque decision-making |
– Building psychological safety for experimentation – Fostering open dialogue by leaders – Leveraging peer networks for collaborative learning – Emphasizing AI as augmentation, not replacement – Focusing on small wins to build confidence |
– Communities: AI Village, Kaggle – Consumer example: Amazon Alexa (anthropomorphizing AI) |
Ethical Dilemmas in AI Adoption |
– Algorithmic bias – Privacy concerns – Transparency issues |
– Establishing clear ethical guidelines – Diversifying training data – Ensuring compliance with GDPR, CCPA – Involving ethicists, domain experts, legal advisors – Embedding ethical AI governance frameworks |
– Alignment with emerging regulations like the EU AI Act |
Aligning AI Upskilling With Business Outcomes |
– Lack of measurable impact – Misalignment with business objectives |
– Define relevant KPIs before training – Monitor efficiency, error rates, employee engagement – Communicate value and impact clearly to motivate learners |
– Example: Google’s AI Works pilot (122 hours saved annually per worker) |
Evaluating Training Effectiveness and Return on Learning Investment (ROLI) |
– Traditional assessments fail to capture real-world application – Difficulty measuring behavioral change |
– Use AI-powered learning analytics dashboards – Track knowledge and practical application – Calculate ROLI including productivity and adaptability gains – Continuous evaluation to refine programs |
– Boston Consulting Group insights on resilient learning cultures |
Managing Uncertainty and Maintaining Adaptability |
– Rapid evolution of AI creates ongoing uncertainty – Need for flexible response to emerging technologies |
– Foster organizational learning agility – Encourage continuous experimentation and innovation – Break down silos for cross-functional knowledge sharing – Embrace open-source AI and community participation – Cultivate continuous learning and psychological safety cultures |
– “AI natives” exemplify agility as competitive advantage |
Advanced Strategies and Future-Proofing Your AI Skillset
Advanced Strategies and Future-Proofing Your AI Skillset
How do you move beyond the basics and position yourself as a critical AI player within your organization? For experienced professionals, the key is not just mastering AI tools but delving into frontier AI subfields, influencing governance, and cultivating hybrid expertise that blends domain knowledge with AI proficiency. The coming years will usher in a seismic shift in how AI integrates into work, making it essential to future-proof your skillset strategically.
Diving Deep into Frontier AI Specializations
Explainable AI (XAI) is rapidly becoming a foundational competency. By 2025, transparency and trust in AI systems won’t be optional—they’ll be prerequisites. In high-stakes industries like healthcare and finance, where decisions impact lives and livelihoods, organizations demand AI systems that can justify their reasoning rather than operate as inscrutable black boxes.
XAI techniques enable users and auditors to trace how machine learning models arrive at conclusions, mitigating risks and fostering accountability. For example, “human-centered explainable AI” (HCXAI) emphasizes designing AI systems with people—not just algorithms—at the core. Libraries and academic institutions are pioneering standards ensuring AI systems meet criteria for authority, credibility, and accuracy. This work is both a technical challenge and an ethical imperative.
Ethics frameworks form another critical frontier. With AI regulation evolving globally—from the EU’s AI Act to emerging policies in Africa and Latin America—professionals versed in ethical AI considerations and compliance will be in high demand. These frameworks address privacy, transparency, fairness, and accountability. Embedding such principles into AI development helps ensure that AI technologies serve humanity’s best interests while minimizing harm.
Understanding and contributing to explainability and ethical frameworks will elevate your influence in your organization’s AI maturity journey, aligning technical expertise with responsible innovation.
Shaping AI Governance: From Policy to Practice
Why does AI governance matter, and how can you actively contribute? Governance is the connective tissue that balances innovation with risk mitigation throughout the AI lifecycle—from development and deployment to continuous monitoring. Without effective governance, organizations risk reputational damage, legal penalties, and operational disruption.
Governance frameworks typically include policies, processes, and clearly defined roles overseeing AI systems. Leading enterprises such as IBM and Lockheed Martin invest heavily in governance structures that align with evolving ethical standards and regulatory mandates. As an individual professional, your contributions might involve:
- Designing and refining risk assessment protocols tailored to specific AI applications.
- Advocating for transparency and fairness in AI-driven decisions.
- Facilitating education and training programs that foster a responsible AI culture.
Importantly, AI governance extends beyond IT or compliance teams. It requires cross-functional collaboration involving legal, HR, data science, and business units. Developing hybrid skills—integrating your domain expertise with AI literacy and governance insights—positions you as a strategic partner in AI integration.
Hybrid AI models exemplify the fusion of traditional domain knowledge with AI capabilities. For instance, AspenTech’s hybrid modeling combines first principles-based simulations with AI analytics, optimizing industrial assets more effectively than either approach alone. This synergy accelerates problem-solving and decision-making by leveraging human expertise embedded in domain-specific knowledge. Mastering the architecture and management of such hybrid systems will distinguish your skillset.
Riding the Waves of Emerging AI Trends
The AI landscape is shifting from exclusive, complex technology toward broadly accessible tools empowering diverse users. The democratization of AI means that by 2025, even small and mid-sized businesses can harness advanced AI without massive budgets or specialized teams.
Low-code and no-code platforms drive this trend, enabling domain professionals to create AI-powered applications tailored to their workflows. The rise of citizen AI developers calls for new competencies:
- Integrating AI responsibly within existing processes.
- Critically evaluating AI outputs for accuracy and bias.
- Maintaining data privacy and compliance.
Another transformative trend is the emergence of AI agents—autonomous digital workers capable of performing complex tasks with minimal human intervention. Organizations like Wells Fargo and Bayer already deploy AI agents that save thousands of employee hours annually. These agents are collaborators, augmenting rather than replacing human potential.
As AI assumes routine or data-intensive tasks, uniquely human skills—such as judgment, creativity, and ethical reasoning—become even more valuable. Your future-proof skillset should include the ability to work alongside AI agents, orchestrate their outputs, and apply nuanced understanding that only humans can provide.
In Human Resources, AI is reshaping talent management through data-driven decision-making, predictive analytics, and personalized learning paths. HR leaders integrate AI to align talent, technology, and culture, facilitating organizational adaptation to AI’s cultural shift. Upskilling in AI literacy within HR or related domains can position you as a pivotal player in this transformation.
Lifelong Learning: The Ultimate Hedge Against Obsolescence
If there’s one constant in AI, it’s change. The rapid pace of innovation demands a disciplined commitment to continuous learning. This doesn’t mean chasing every new tool but cultivating a strategic, sustainable approach:
- Follow reputable sources and research to distinguish hype from substance.
- Engage with communities focused on explainable AI, ethics, and governance.
- Participate in workshops and training sessions that deepen both technical skills and conceptual understanding.
- Experiment with AI agents and hybrid models to build practical, hands-on experience.
- Reflect regularly on how AI amplifies your uniquely human strengths—judgment, creativity, and empathy.
Remember, AI is a tool to augment human intelligence, not replace it. Those who thrive will be the professionals who leverage AI to elevate strategic thinking and creative problem-solving, rather than merely automating tasks.
The trajectory is clear: by specializing in frontier AI subfields, actively engaging in governance, developing hybrid expertise, and embracing emerging trends thoughtfully, you can future-proof your career. The AI era rewards those who combine technical mastery with ethical insight and a human-centered perspective. It’s not just about keeping pace; it’s about shaping the future of work itself.
Strategy | Description | Key Focus Areas | Examples / Applications |
---|---|---|---|
Diving Deep into Frontier AI Specializations | Mastering advanced AI subfields like Explainable AI and ethical frameworks to build transparency and trust in AI systems. |
– Explainable AI (XAI) – Human-centered Explainable AI (HCXAI) – Ethics frameworks and compliance – Transparency, fairness, accountability |
– AI systems justifying decisions – AI regulation (EU AI Act, others) – Academic standards for AI credibility |
Shaping AI Governance: From Policy to Practice | Contributing to governance frameworks that balance innovation with risk mitigation and ethical standards. |
– Risk assessment protocols – Transparency and fairness advocacy – Cross-functional collaboration – Hybrid AI models combining domain knowledge with AI |
– IBM, Lockheed Martin governance investments – AspenTech hybrid modeling – Facilitating responsible AI culture |
Riding the Waves of Emerging AI Trends | Adapting to AI democratization, low-code/no-code platforms, AI agents, and the growing role of uniquely human skills. |
– Low-code/no-code AI tools – Citizen AI developers – AI agents as collaborators – Human skills: judgment, creativity, ethics |
– AI agents at Wells Fargo, Bayer – AI in HR for talent management – Data-driven decision-making |
Lifelong Learning: The Ultimate Hedge Against Obsolescence | Commitment to continuous, strategic learning to stay current and effectively leverage AI. |
– Following reputable sources – Engaging with AI ethics and governance communities – Hands-on experience with AI agents and hybrid models – Emphasizing human strengths alongside AI |
– Workshops and training – Reflecting on AI’s role in augmenting intelligence – Elevating strategic thinking and problem-solving |