AI & Data Privacy: Essential Compliance Guide for Businesses 2024
- Introduction: Why AI and Data Privacy Regulations Matter Now
- Introduction: Why AI and Data Privacy Regulations Matter Now
- The Data Hunger of AI and Its Privacy Implications
- A Flourishing Regulatory Landscape
- Balancing Innovation with Compliance: The Business Stakes
- Navigating the Technical, Legal, and Ethical Dimensions
- Foundations of Data Privacy in the Age of AI
- Foundations of Data Privacy in the Age of AI
- Core Privacy Concepts in the Context of AI
- How AI Challenges Traditional Privacy Definitions
- Regulatory Frameworks and Their Relevance to AI
- Why This Matters for Businesses
- Technical Challenges of AI in Protecting Data Privacy
- Technical Challenges of AI in Protecting Data Privacy
- Data Collection, Persistence, and Spillover: The Hidden Layers of AI’s Data Appetite
- Security Risks Unique to AI: When Models Become Vulnerabilities
- Technical Safeguards: Building Privacy into AI by Design
- Balancing Utility and Privacy: An Ongoing Tradeoff
- Conclusion
- Global Regulatory Landscape and Comparative Analysis
- Global Regulatory Landscape and Comparative Analysis
- The European Union: Pioneering Comprehensive AI Regulation
- The United States: Fragmentation and Emerging Frameworks
- Australia and Other Jurisdictions: Catching Up with Nuanced Approaches
- Comparative Takeaways: Where Do Regulations Align and Diverge?
- Looking Ahead: The Compliance Imperative in an Uncertain Regulatory Future
- Practical Implications for Businesses: Compliance and Ethical AI Deployment
- Practical Implications for Businesses: Compliance and Ethical AI Deployment
- Establishing Robust Data Governance Frameworks
- Conducting AI-Specific Risk Assessments and Privacy Impact Assessments
- Embedding Transparency and Ethical Considerations in AI Deployment
- Key Takeaways for Businesses
- AI as a Tool for Enhancing Data Privacy and Security
- AI as a Tool for Enhancing Data Privacy and Security
- AI-driven Anomaly Detection: Catching Breaches Early
- Automated Compliance Monitoring and Encryption Management: Scaling Privacy Protections
- Limitations and Risks: Why AI Alone Isn’t Enough
- Looking Ahead: Future Trends and Evolving Challenges in AI and Data Privacy
- Looking Ahead: Future Trends and Evolving Challenges in AI and Data Privacy
- Anticipated AI Advancements and Their Impact on Privacy Enforcement
- Evolution of Privacy Regulations: Biometric Data, AI Transparency, and Collective Rights
- Navigating the Uncertainties: Balancing Innovation, Ethics, and Global Cooperation
- Key Takeaways for Businesses
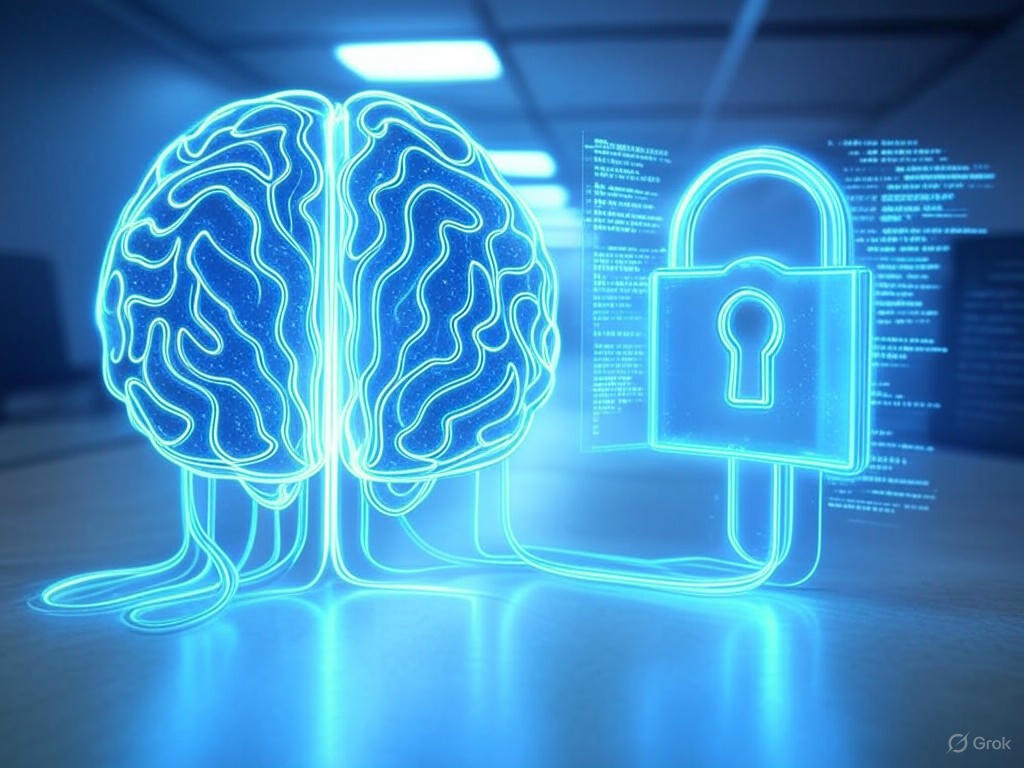
Introduction: Why AI and Data Privacy Regulations Matter Now
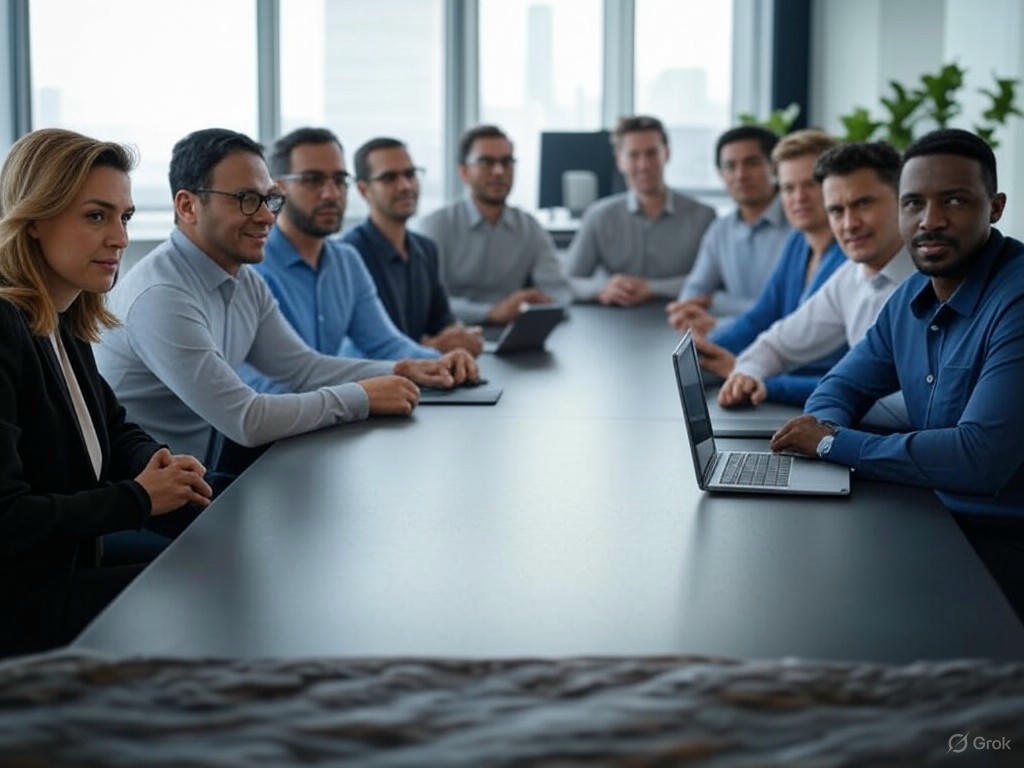
Introduction: Why AI and Data Privacy Regulations Matter Now
How did AI become such a voracious consumer of data, and why does this matter critically for businesses today? The answer lies in the rapid evolution of AI capabilities, particularly the rise of generative and agentic AI systems that depend on vast amounts of unstructured data to operate effectively. According to the 2025 AI Index Report by Stanford HAI, private investment in AI soared to $109.1 billion in 2024, with generative AI alone attracting nearly $34 billion globally. This massive influx of capital fuels increasingly sophisticated models that analyze and generate content across sectors—from healthcare and finance to retail and telecommunications (Exploding Topics, 2025). However, this growth comes with an escalating appetite for data, significantly raising the stakes for privacy and security.
The Data Hunger of AI and Its Privacy Implications
Think of AI models as engines running on data fuel. As AI systems become more complex and agentic, they require more diverse and voluminous datasets. Much of this data is unstructured—such as emails, images, voice recordings, and social media posts—which poses significant challenges for governance and protection (MIT Sloan Management Review, 2025). While businesses eagerly pursue the productivity gains AI promises, many acknowledge a lack of rigorous evaluation regarding actual business value, which can lead to unchecked data collection practices.
This insatiable data demand introduces serious privacy risks. AI’s capability to process biometric data, conduct covert data collection, and profile users without explicit consent extends beyond traditional data breach concerns (DataGuard, 2025). Such opacity erodes consumer trust, a critical currency in today’s digital economy. For instance, less than 10% of users trust chatbots for sensitive tasks like insurance claims (Exploding Topics, 2025). Without robust data privacy safeguards, businesses face not only regulatory penalties but also significant reputational damage.
A Flourishing Regulatory Landscape
Worldwide, regulators are tightening controls on the collection, storage, and processing of data, particularly that used by AI. The European Union’s GDPR and California’s CCPA set rigorous data privacy standards that many organizations strive to meet. However, the global landscape remains fragmented, adding complexity to compliance efforts. Privacy and security by design have emerged as foundational principles within AI risk management frameworks (Dentons, 2025), mandating that businesses integrate privacy considerations from the earliest stages of AI system development rather than retrofitting protections later.
The consequences for non-compliance are severe. Companies risk substantial fines, legal actions, and long-term erosion of customer confidence. As AI systems increasingly operate in sensitive domains such as law enforcement and healthcare, regulators are focusing more on ethical dimensions, including data misuse and algorithmic bias (DataGuard, 2025). Staying agile and informed in this evolving legal environment is imperative for businesses.
Balancing Innovation with Compliance: The Business Stakes
What is at stake for businesses? AI offers transformative opportunities: personalized customer experiences, streamlined operations, and new revenue models. Conversely, unchecked data practices expose companies to legal risks and ethical pitfalls that can undermine these advantages. For example, AI-driven personalization in retail and marketing relies on granular consumer data but must navigate a patchwork of privacy laws varying by jurisdiction (Exploding Topics, 2025).
To succeed, companies must balance innovation with compliance by:
- Leveraging automated data classification and real-time threat detection
- Maintaining transparency and user control over data
- Embedding cross-disciplinary expertise across technical, legal, and ethical domains
Organizations adopting structured privacy strategies and integrating AI with established cybersecurity measures—such as multi-factor authentication (MFA) and encryption—are better positioned to mitigate risks and build trust (Lumenalta, 2025).
Navigating the Technical, Legal, and Ethical Dimensions
The intersection of AI and data privacy has evolved from a niche concern to a central strategic challenge. Technically, AI architects must design systems that minimize data exposure and enhance explainability. Legally, compliance teams must interpret a shifting regulatory landscape and anticipate emerging standards. Ethically, organizations bear responsibility for addressing AI’s societal impact on privacy rights, fairness, and individual autonomy.
Consider the analogy of constructing a city: AI represents the skyscrapers reshaping the skyline, while data privacy regulations are the zoning laws and safety codes that keep the city livable and secure. Ignoring these rules risks structural collapse, just as neglecting privacy can lead to catastrophic consequences for enterprises.
In the following sections, we will explore pragmatic approaches businesses can adopt to harness AI’s power responsibly—respecting privacy and complying with global regulations. The urgency is clear: AI’s transformative potential depends on making privacy a foundational pillar, not an afterthought.
Aspect | Details |
---|---|
AI Data Consumption | Generative and agentic AI require vast amounts of unstructured data such as emails, images, voice recordings, and social media posts. |
Investment in AI (2024) | $109.1 billion total; $34 billion in generative AI globally (Stanford HAI, 2025) |
Privacy Risks | Biometric data processing, covert data collection, user profiling without consent, erosion of consumer trust. |
Regulatory Frameworks | GDPR (EU), CCPA (California), privacy and security by design principles, fragmented global landscape. |
Consequences of Non-Compliance | Fines, legal actions, reputational damage, ethical scrutiny, especially in sensitive domains like healthcare and law enforcement. |
Business Compliance Strategies | Automated data classification, real-time threat detection, transparency, user control, multidisciplinary expertise. |
Security Measures | Integration of multi-factor authentication (MFA), encryption, and cybersecurity protocols. |
Technical, Legal, Ethical Dimensions | Minimizing data exposure, explainability, interpreting regulations, addressing fairness and autonomy. |
Foundations of Data Privacy in the Age of AI
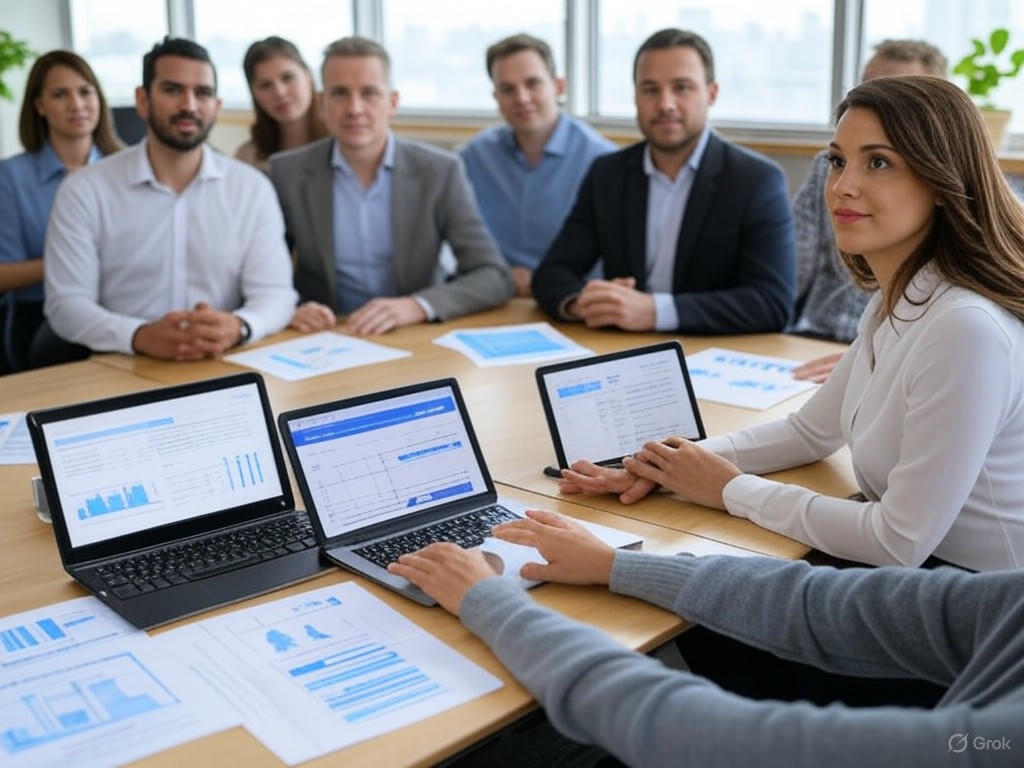
Foundations of Data Privacy in the Age of AI
What qualifies as personal data in the context of AI? Traditional definitions are evolving as AI systems do more than process raw inputs—they generate inferences, correlations, and profiles that reveal much more about individuals than the original data collected. The European Data Protection Board’s (EDPB) Opinion 28/2024 highlights this complexity, confirming that personal data encompasses not only direct identifiers but also any information capable of identifying a person indirectly through linking or inference.
This expanded notion of identifiability challenges conventional privacy boundaries and requires businesses to rethink how they classify and protect data in AI environments.
Core Privacy Concepts in the Context of AI
To navigate these challenges, it’s important to revisit fundamental privacy principles and understand how AI complicates them:
-
Personal Data & Identifiability: Under the GDPR, personal data refers to any information relating to an identified or identifiable individual. AI complicates this by relying heavily on inferred data—attributes deduced from patterns rather than explicitly provided. For example, an AI might infer a user’s health status or political views from seemingly unrelated data points. Such inferred attributes still qualify as personal data because of their potential to identify individuals (European Data Protection Board, 2024).
-
Data Minimization: This principle requires collecting only the data absolutely necessary for the intended purpose. AI’s demand for vast and diverse datasets to improve accuracy often conflicts with this mandate. The EU AI Act, adopted in 2024, explicitly reinforces data minimization, mandating that AI systems limit data intake to what is strictly essential. Far from a mere bureaucratic formality, this principle is a critical tool to reduce privacy risks and prevent “data bloat,” which complicates compliance and weakens security (Dentons, 2025).
-
Consent: Consent must be informed, specific, and freely given. However, AI’s use of unstructured data and complex data flows complicates obtaining meaningful consent. The phenomenon of “consent fatigue” is widespread—users face frequent permission requests without clear explanations, undermining true informed consent. The EU AI Act builds on GDPR’s foundation by emphasizing transparency and user-centric consent management, encouraging organizations to adopt explainable AI techniques that clarify how data is used (Exploding Topics, 2025).
How AI Challenges Traditional Privacy Definitions
AI’s reliance on data linking and inference pushes privacy concepts into new, uncertain territory. Unlike traditional data processing, AI models:
-
Generate New Personal Data: AI synthesizes new insights that qualify as personal data themselves. For example, linking disparate datasets can reveal sensitive attributes not explicitly collected—such as predicting creditworthiness or health risks from browsing habits. This expansion of personal data complicates data governance.
-
Complicate Risk Assessments: Privacy risk assessments must now account for downstream effects of AI inferences. Traditional models focus on the initial data collected, but AI’s emergent properties from combined data streams can create unanticipated privacy harms.
-
Increase Data Leakage Risks: AI models trained on sensitive data can inadvertently memorize and expose private information. Large language models, for instance, have demonstrated vulnerabilities to model inversion and membership inference attacks, which can expose confidential details. This heightens the need for technical safeguards such as pseudonymization, deidentification, and robust security controls (Gartner, 2024).
These challenges are practical and pressing. A 2025 study by Ketch found that 88% of businesses failed to fully respect consumer consent signals, exposing a gap between AI-driven marketing practices and privacy expectations. “Dirty data”—data collected without clear consent or provenance—not only threatens compliance but also undermines AI model reliability and trustworthiness.
Regulatory Frameworks and Their Relevance to AI
The GDPR remains the foundational data privacy regulation in Europe, but its application to AI is rapidly evolving. The EDPB’s Opinion 28/2024 provides critical guidance on this front:
-
Legal Basis for AI Processing: The EDPB confirms that legitimate interest can serve as a legal basis for AI development and deployment, but with strict conditions. Organizations must demonstrate that their interests are necessary and do not override individuals’ fundamental rights, requiring rigorous balancing tests and continuous reassessment.
-
Data Subject Rights: Rights such as access, rectification, erasure, and objection become more complex when AI is involved. For example, the “right to explanation” remains debated—how transparent can AI decision-making be when models are inherently opaque? The EDPB stresses that data subjects must have practical means to exercise their rights, necessitating new mechanisms tailored to AI’s unique characteristics.
-
Obligations for Controllers and Processors: Both AI developers and deployers share responsibility. Third-party users of AI models cannot evade due diligence; they must verify compliance and embed data protection principles throughout the AI lifecycle. Privacy-by-design is no longer optional but a regulatory imperative under GDPR and the EU AI Act (Dentons, 2025).
The EU AI Act, enacted in mid-2024, complements GDPR by addressing AI-specific risks—requiring detailed impact assessments for high-risk AI systems, enforcing data minimization, transparency, and mandating AI literacy. In the United States, while federal AI laws remain fragmented, state-level privacy statutes like California’s AI Transparency Act build on similar principles, signaling a trend toward global convergence in AI data governance (California AI Transparency Act, 2025).
Why This Matters for Businesses
Ignoring these evolving privacy foundations is not an option. Non-compliance risks steep fines, reputational damage, and erosion of customer trust. More importantly, AI’s effectiveness depends on trustworthy data practices—dirty data and consent violations degrade AI’s value and expose businesses to legal and ethical pitfalls.
To meet these challenges, businesses should:
-
Conduct thorough data mapping to understand what personal data AI systems process and generate.
-
Implement robust consent management frameworks that move beyond simple checkboxes toward meaningful user engagement.
-
Adopt technical safeguards such as pseudonymization, deidentification, and data minimization strategies to mitigate privacy risks.
-
Embed privacy considerations throughout AI development—from training data curation to deployment monitoring—following privacy-by-design principles.
The regulatory landscape is still settling, but the trajectory is clear: AI cannot be exempt from privacy laws. Instead, it demands an evolved interpretation of privacy principles that balances innovation with respect for individual rights. Businesses that master this balance will not only ensure compliance but also gain a competitive edge by building AI systems users can trust.
Concept | Description | Regulatory Reference | Business Implication |
---|---|---|---|
Personal Data & Identifiability | Includes direct and indirect identifiers, plus AI-inferred attributes that can identify individuals. | EDPB Opinion 28/2024, GDPR | Businesses must rethink data classification and protection, including inferred data. |
Data Minimization | Collect only data strictly necessary for the intended purpose; limits data bloat. | GDPR, EU AI Act 2024 | Restricts AI data intake; reduces privacy risks and compliance complexity. |
Consent | Consent must be informed, specific, freely given; AI complicates meaningful consent due to complex data flows and consent fatigue. | GDPR, EU AI Act 2024 | Requires transparent, user-centric consent management and explainable AI techniques. |
Generation of New Personal Data | AI synthesizes new insights from linked datasets that qualify as personal data themselves. | EDPB Opinion 28/2024 | Complicates data governance and risk assessment. |
Risk Assessments | Must include downstream effects of AI inferences beyond initial data collection. | GDPR, EU AI Act 2024 | Requires updated methodologies to account for emergent AI privacy harms. |
Data Leakage Risks | AI models can memorize and expose private information via attacks like model inversion. | Gartner 2024 | Necessitates technical safeguards such as pseudonymization and deidentification. |
Legal Basis for AI Processing | Legitimate interest allowed but requires balancing tests and ongoing reassessment. | EDPB Opinion 28/2024 | Organizations must justify AI use without overriding individual rights. |
Data Subject Rights | Access, rectification, erasure, objection become more complex with AI; right to explanation is debated. | EDPB Opinion 28/2024 | Needs tailored mechanisms for exercising rights in AI context. |
Controller & Processor Obligations | Shared responsibility for AI compliance; privacy-by-design mandatory. | GDPR, EU AI Act 2024 | Due diligence must be embedded throughout AI lifecycle. |
Regulatory Frameworks | GDPR foundational; EU AI Act complements with AI-specific rules; US state laws emerging. | GDPR, EU AI Act 2024, California AI Transparency Act 2025 | Businesses face evolving global compliance landscape. |
Technical Challenges of AI in Protecting Data Privacy
Technical Challenges of AI in Protecting Data Privacy
The scale and complexity of AI systems put immense pressure on traditional data privacy frameworks. This strain arises largely from how AI collects, stores, and repurposes data, creating novel security risks that demand new approaches.
Data Collection, Persistence, and Spillover: The Hidden Layers of AI’s Data Appetite
At the heart of AI’s data privacy challenges is its voracious consumption of data. AI systems typically gather massive volumes of information through web scraping and large-scale aggregation from diverse sources. This is not just a matter of quantity but also of variety and persistence.
For instance, data centers—critical infrastructure supporting AI innovation—play a pivotal role. Nearly 6 million employees in Germany work at companies reliant on cloud infrastructures powered by these data centers. Almost half of these companies prioritize keeping data centers within national borders to address regulatory and privacy concerns. This highlights the intersection of data sovereignty and AI scalability.
Data persistence introduces complex issues as well. Data collected for one purpose may be stored long-term and later repurposed without explicit consent or transparency. This “spillover” effect risks using data initially gathered for benign reasons in ways that raise questions about ownership, consent, and compliance. Frequent copying, movement, and analysis of data in AI pipelines expand the attack surface, increasing vulnerability to breaches.
Security Risks Unique to AI: When Models Become Vulnerabilities
AI introduces privacy risks uncommon in traditional IT systems. One particularly concerning threat is model inversion attacks, where attackers exploit model outputs—such as predictions or confidence scores—to reconstruct sensitive training data. Imagine peeling back layers of a model to reveal confidential patient records or proprietary business information embedded in the training set.
Recent research from 2024-2025 has shown these attacks growing more sophisticated. Attackers can extract trade secrets or sensitive characteristics of vulnerable groups. Another risk, membership inference attacks, enables adversaries to determine whether specific data points were part of a model’s training data, potentially exposing sensitive associations or enabling discrimination against protected classes.
Gartner’s 2024 AI Security Survey found that 73% of enterprises experienced at least one AI-related security incident in the past year. Sectors such as financial services and healthcare are especially vulnerable. The rapid rise of generative AI has outpaced existing security controls, amplifying risks like prompt injection and data poisoning attacks. These incidents threaten not only data confidentiality but also erode trust in AI technologies.
Technical Safeguards: Building Privacy into AI by Design
To address these challenges, businesses must adopt privacy by design principles—integrating privacy protections throughout the AI development lifecycle rather than treating them as afterthoughts. Key technical safeguards gaining widespread adoption include:
-
Differential Privacy: This method adds carefully calibrated noise to data or model queries, making it mathematically improbable to identify individuals. Tech giants like Apple and Google deploy differential privacy to collect aggregate user statistics while preserving individual anonymity.
-
Federated Learning: Instead of centralizing raw data, federated learning trains AI models locally on user devices or edge nodes. Only model updates—not raw data—are shared and aggregated, significantly reducing data leakage risks and better aligning with data sovereignty laws.
-
Encryption Techniques: Advanced methods such as homomorphic encryption and secure multi-party computation allow AI computations on encrypted data without first decrypting it. Although computationally intensive, these techniques hold promise for highly sensitive sectors like healthcare and finance, where data confidentiality is paramount.
-
Model Access Controls and Monitoring: Restricting who can query AI models and tracking unusual query patterns help detect and prevent model inversion and membership inference attacks early. Governance of APIs and controlling output granularity are critical components of this layered defense.
Balancing Utility and Privacy: An Ongoing Tradeoff
A fundamental tension underlies AI privacy challenges: AI’s power comes from learning on vast, detailed datasets, yet this same capability risks exposing the very data it should protect.
Techniques like differential privacy and federated learning often introduce trade-offs between model accuracy and privacy guarantees. Moreover, as AI models generalize beyond their initial training scope—a phenomenon still not fully understood—predicting and controlling unintended data leakage becomes increasingly difficult.
For businesses, managing privacy is a continuous balancing act that requires:
- Close collaboration between data scientists, security teams, and legal experts
- Strategic investments in emerging privacy-preserving technologies
- Vigilant monitoring of AI model behavior after deployment
- Transparent communication with users about data collection and usage practices
Conclusion
The technical challenges at the intersection of AI and data privacy are complex and rapidly evolving. Large-scale data collection, persistence, and repurposing create intricate risk landscapes that are further complicated by emerging attack vectors like model inversion and membership inference.
Nonetheless, promising privacy-preserving technologies such as differential privacy, federated learning, and advanced encryption offer practical paths forward. Businesses that proactively embed privacy by design, adopt robust technical safeguards, and maintain vigilant oversight will not only mitigate regulatory risks but also cultivate greater trust in their AI systems.
Navigating this terrain demands both technical rigor and ethical foresight—because in AI, protecting privacy ultimately means protecting people.
Aspect | Details |
---|---|
Data Collection | Massive volumes via web scraping and aggregation; data centers critical; data sovereignty prioritized |
Data Persistence & Spillover | Data stored long-term, repurposed without explicit consent; increases attack surface |
Security Risks Unique to AI | Model inversion attacks, membership inference attacks, prompt injection, data poisoning; 73% enterprises faced AI-related incidents |
Technical Safeguards | Differential Privacy, Federated Learning, Encryption Techniques (homomorphic, multi-party computation), Model Access Controls and Monitoring |
Balancing Utility & Privacy | Trade-off between accuracy and privacy; requires multi-disciplinary collaboration and continuous monitoring |
Global Regulatory Landscape and Comparative Analysis
Global Regulatory Landscape and Comparative Analysis
How are governments worldwide grappling with AI’s privacy challenges? The regulatory landscape is evolving rapidly, shaped by diverse legal traditions, economic priorities, and cultural values. For businesses operating across borders, understanding these differences—and their practical implications—is essential.
The European Union: Pioneering Comprehensive AI Regulation
The European Union remains at the forefront of comprehensive AI and data privacy regulation, building on its landmark General Data Protection Regulation (GDPR) from 2018. The GDPR set a global benchmark with foundational principles such as lawful processing, data minimization, and robust data subject rights.
Extending this leadership into the AI era, the EU’s AI Act, enacted in mid-2024, is the first harmonized legal framework specifically targeting AI systems. It adopts a risk-based approach, categorizing AI applications into four tiers—from unacceptable to minimal risk—to clarify obligations for developers and deployers. Key points include:
- High-risk AI systems (e.g., biometric identification, critical infrastructure) must comply with stringent requirements, including detailed risk assessments, transparency mandates, and human oversight.
- Minimal or no-risk applications, such as AI-powered video games or spam filters, are largely exempt from these burdens.
The AI Act explicitly complements the GDPR by addressing AI-specific privacy risks—such as biases, manipulation, and emergent data harms—not fully covered by existing privacy laws. It also mandates organizations to promote AI literacy among employees involved in AI deployment, recognizing that human expertise is vital to preventing unauthorized data exposure.
This layered regulatory framework is already having real-world impact. The Italian Data Protection Authority imposed a €15 million fine on OpenAI for GDPR violations linked to ChatGPT, including failure to notify regulators promptly about a data breach. This enforcement followed a temporary ban of ChatGPT in Italy, underscoring regulators’ growing intolerance for non-compliance.
Similarly, the Italian regulator warned GEDI, a major publishing group, for sharing sensitive editorial content with OpenAI for AI training without appropriate legal grounds. This case highlights the EU’s strict stance on using sensitive data for AI development and the risks businesses face if they neglect privacy requirements.
The United States: Fragmentation and Emerging Frameworks
In contrast, the United States currently lacks a comprehensive federal data privacy law, resulting in a patchwork of state-level regulations and agency guidelines. Rather than a dedicated AI regulatory agency or overarching AI statute, the U.S. relies on existing laws and evolving frameworks.
The White House’s U.S. AI Bill of Rights, issued as a non-binding framework, outlines aspirational principles such as transparency, privacy, and accountability. However, it lacks enforcement mechanisms. Instead, momentum is driven largely at the state level, where comprehensive privacy laws are proliferating rapidly.
In 2024, eight states had comprehensive privacy laws, with projections suggesting 16 states will have such laws by 2025. States like California, New York, and Utah are introducing AI-specific rules. For example, California’s AI Transparency Act mandates clear disclosure when generative AI is used in healthcare communications and requires documentation of generative AI training data.
The Federal Trade Commission (FTC) has heightened enforcement actions, particularly targeting companies that overstate AI capabilities or mishandle consumer data. The FTC’s skepticism toward “data clean rooms” exemplifies ongoing scrutiny over emerging AI data practices.
However, this decentralized and fragmented regulatory landscape poses compliance challenges. Businesses must navigate divergent state laws—some with penalties of thousands of dollars per violation per day—while anticipating potential future federal legislation. This requires flexible governance frameworks that can scale and adapt across jurisdictions.
Australia and Other Jurisdictions: Catching Up with Nuanced Approaches
Beyond the EU and U.S., other regions are beginning to develop their own AI and data privacy regulatory stances. Australia, for example, is strengthening its data protection laws and exploring AI-specific guidelines that emphasize transparency and ethical AI use.
Globally, common regulatory themes are emerging:
- Data Governance: Many jurisdictions emphasize lawful bases for data processing, with special scrutiny on sensitive and biometric data.
- Transparency: Clear user communication about AI involvement in decision-making is increasingly expected.
- Accountability: Organizations must document AI risk assessments, audits, and mitigation strategies to demonstrate compliance.
- Enforcement Trends: Regulators are transitioning from rulemaking to active investigations and litigation, as illustrated by Europe’s GDPR fines and the FTC’s enforcement actions in the U.S.
Comparative Takeaways: Where Do Regulations Align and Diverge?
-
Risk-based vs. Principle-based: The EU’s AI Act employs a risk-tiered approach with concrete obligations based on potential harm. The U.S. favors broad principles and sector-specific rules, creating a more fragmented environment.
-
Scope and Coverage: The EU’s framework aims for comprehensive regulation of AI across public and private sectors. The U.S. currently lacks a unified AI statute, relying instead on a mosaic of state laws and federal agency oversight.
-
Transparency and Consent: Both regions emphasize transparency, but the EU’s GDPR requires prior explicit consent for personal data processing. U.S. laws vary widely by state and sector, leading to inconsistent consent requirements.
-
Enforcement: The EU demonstrates strong enforcement willingness, as evidenced by Italy’s €15 million fine on OpenAI. The U.S. FTC is active but enforcement is often reactive and less centralized.
These differences compel businesses to develop nuanced, regionally informed compliance strategies. For instance, deploying AI-powered health diagnostics requires navigating the EU’s stringent AI Act and GDPR, California’s disclosure mandates, and Australia’s emerging guidelines—while preparing for ongoing regulatory evolution.
Looking Ahead: The Compliance Imperative in an Uncertain Regulatory Future
AI and data privacy regulations will continue to evolve as AI capabilities advance and new privacy risks surface. The interplay between AI-specific rules and general data privacy laws will deepen, necessitating integrated governance approaches.
Businesses that invest early in:
- Comprehensive AI risk assessments,
- Transparent disclosures about data and AI use,
- Robust accountability frameworks, and
- Employee AI literacy and training
will be better positioned not only to avoid costly fines but also to build trust with consumers and regulators.
The OpenAI GDPR cases serve as cautionary tales—demonstrating that even leading tech companies face severe consequences when compliance is overlooked. As regulatory regimes shift from soft guidance to hard enforcement, the cost of ignorance will only increase.
In summary, navigating the global AI regulatory mosaic is complex but essential. Success demands vigilance, adaptability, and a clear-eyed understanding of both the technological and legal landscapes shaping AI’s future.
Aspect | European Union | United States | Australia & Other Jurisdictions |
---|---|---|---|
Regulatory Approach | Comprehensive, harmonized AI Act with risk-based tiers complementing GDPR | Fragmented; no federal AI law, state-level privacy laws and sector-specific rules | Emerging AI-specific guidelines, strengthening data protection laws with ethical emphasis |
Scope and Coverage | Public and private sectors; AI systems categorized by risk | Varies by state; mosaic of laws and agency oversight | Developing; focus on transparency and ethical AI use |
Key Legal Frameworks | GDPR (2018), AI Act (2024) | State laws (e.g., California Privacy Laws), FTC guidelines, U.S. AI Bill of Rights (non-binding) | Data protection laws and emerging AI guidelines |
Risk Categorization | Four risk tiers: unacceptable, high-risk, limited risk, minimal/no risk | No formal risk tiers; principle-based with sector rules | Not fully defined; emphasis on risk and transparency |
Transparency Requirements | Strict; explicit prior consent required under GDPR; AI Act mandates transparency | Varies widely by state and sector; California AI Transparency Act requires disclosures | Increasing emphasis on clear communication about AI use |
Consent Requirements | Prior explicit consent for personal data processing | Inconsistent, state-dependent | Developing; aligned with data protection principles |
Enforcement | Strong and proactive; e.g., €15 million fine on OpenAI by Italian authority | Active but reactive; FTC enforcement increasing but decentralized | Emerging; focus on transparency and accountability |
Notable Enforcement Cases | OpenAI GDPR violation fine; ChatGPT temporary ban in Italy; GEDI warning for data sharing | FTC actions targeting misleading AI claims and data misuse | None specified |
AI Literacy & Training | Mandated for employees involved in AI deployment | Not mandated federally | Encouraged and emerging |
Common Regulatory Themes | Data governance, transparency, accountability, risk assessments | Transparency, accountability, evolving state laws | Transparency, lawful data processing, ethical AI |
Practical Implications for Businesses: Compliance and Ethical AI Deployment
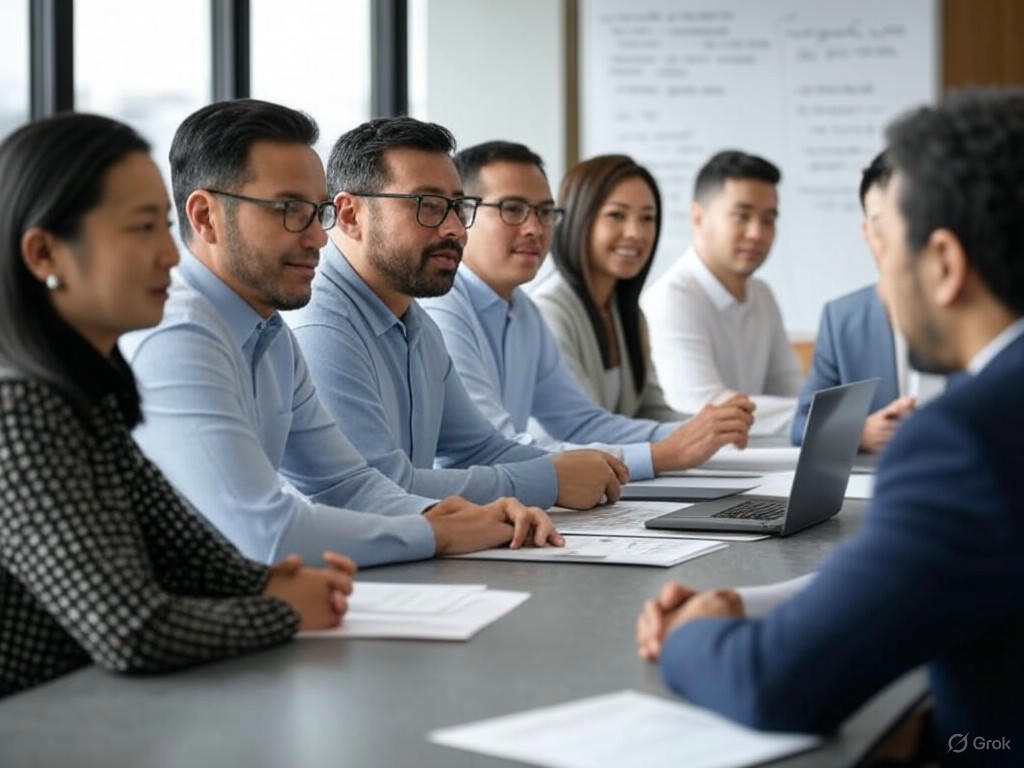
Practical Implications for Businesses: Compliance and Ethical AI Deployment
How can businesses translate complex AI privacy regulations into actionable operational strategies? Bridging the gap between legal frameworks and practical implementation is no small feat, yet it is where responsible AI begins to generate real-world impact. Companies aiming to leverage AI’s transformative potential while complying with evolving data privacy laws must adopt a structured approach encompassing governance, risk assessment, and transparency.
Establishing Robust Data Governance Frameworks
Data governance is far more than a regulatory checkbox; it forms the foundation for building compliant and ethical AI systems. Gartner’s 2024 AI Security Survey warns that by 2025, AI-specific regulations and governance mandates will dominate the landscape, making it imperative for organizations to establish clear policies that define sensitive data boundaries and protection measures.
Businesses should adopt these best practices:
-
Clear Ownership and Collaborative Control: Governance should extend beyond top-down directives. Embedding collaborative workflows, where data stewards across departments share accountability, helps maintain data integrity and regulatory compliance. As Atlan highlights, 80% of organizations risk failure if they ignore this community-led approach to data governance.
-
Automated Data Classification and Role-Based Access: Leveraging automation to classify data and enforce role-based access controls on a granular level—beyond columns or tables—minimizes the risk of unauthorized access and data leakage.
-
Centralized Data Catalogs for Transparency: A well-maintained data catalog enables rapid data discovery and lineage tracking, which are essential for audit readiness and privacy impact assessments.
-
Start Small and Scale Thoughtfully: Hatchworks recommends beginning with focused governance initiatives aligned with strategic goals, then expanding progressively. This approach prevents overwhelming teams while ensuring governance delivers tangible business value.
For instance, organizations using generative AI internally should deploy strict data loss prevention (DLP) tools to block sensitive data from leaking into model training sets or outputs. Symantec’s upcoming 2025 DLP enhancements demonstrate how AI itself can be harnessed to dynamically educate users and enforce data policies.
Conducting AI-Specific Risk Assessments and Privacy Impact Assessments
AI risk assessments pose unique challenges compared to traditional IT systems. AI models continuously evolve and often rely on vast, complex datasets that include personal or sensitive information. This dynamic environment introduces novel privacy risks—ranging from data contamination to inadvertent exposure via model outputs.
Key steps businesses must take include:
-
Implement Data Protection Impact Assessments (DPIAs): DPIAs are a regulatory cornerstone under frameworks like the EU’s GDPR and the AI Act, effective in 2025. Conducting DPIAs early and updating them throughout the AI lifecycle helps identify residual risks and maintain compliance.
-
Leverage Data Catalogs to Support DPIAs: Comprehensive data catalogs provide vital visibility into data lineage and usage, enhancing the accuracy and effectiveness of risk evaluations.
-
Address AI-Specific Risks Highlighted by Regulators: The European Data Protection Board’s 2024 guidance on large language models (LLMs) underscores risks such as unintended memorization of personal data and algorithmic bias. Mitigation strategies include rigorous data curation, strict access controls, and continuous model monitoring.
-
Adopt AI Risk Management Frameworks: International standards like ISO/IEC 27001’s 2024 amendment and OECD’s voluntary AI risk reporting framework emphasize continuous oversight to detect privacy violations, adversarial attacks, and ethical concerns.
-
Focus on the Human Element: Risk management extends beyond technology. Organizations must promote AI literacy as mandated by the EU AI Act, empowering teams to understand AI systems and manage them responsibly.
Practical examples include HiddenLayer’s AI security platform, which protects AI models without disrupting workflows, and TrustWorks’ AI governance tools that automate compliance monitoring. These technologies illustrate how AI can assist in managing inherent risks while fostering innovation.
Embedding Transparency and Ethical Considerations in AI Deployment
Transparency is the cornerstone of trust in AI systems. As AI-generated content proliferates, clear labeling has become both a regulatory expectation and a societal demand.
Essential transparency practices include:
-
Labeling AI-Generated Content: Platforms such as LinkedIn and Meta automatically label posts or media identified as AI-generated, using phrases like “AI-generated” or “Generated with AI.” This practice signals AI involvement honestly and fosters more positive audience engagement according to recent studies.
-
Avoiding Over-Labeling: Not all AI-assisted content requires explicit labeling. Minor AI enhancements to images, for example, may not necessitate disclosure if the original content remains substantially unchanged.
-
Designing for Explainability: Beyond labeling, AI systems should be built with explainability in mind, enabling stakeholders to understand decision-making processes—a critical factor for auditing and regulatory compliance.
From a leadership standpoint, CTOs and CIOs hold a pivotal role in embedding privacy and ethics throughout AI development pipelines. According to RSM US, only 20% of executives feel they have meaningfully integrated AI into their organizations, highlighting a significant opportunity.
Technology leaders should:
-
Champion Privacy-by-Design: Embedding privacy measures at the earliest stages of AI system design minimizes costly retrofits and compliance failures.
-
Balance Innovation with Ethical Responsibility: While AI drives operational efficiency and opens new revenue streams, KPMG’s 2025 survey reveals that organizations must invest heavily in training to ensure ethical AI use.
-
Adopt Flexible, Scalable Frameworks: With a fragmented regulatory landscape—including laws like California’s CCPA and the evolving EU AI Act—CTOs and CIOs must develop governance models that adapt swiftly without disrupting business continuity.
-
Foster a Culture of Accountability: Encouraging collaboration among legal, technical, and business teams ensures alignment and responsiveness as AI technologies and regulations evolve.
Key Takeaways for Businesses
Navigating AI and privacy regulations is no longer optional—it is a strategic imperative. Organizations that proactively:
- Implement decentralized, automated data governance
- Conduct thorough, AI-specific risk and privacy impact assessments
- Embrace transparency through thoughtful AI content labeling
- Empower technology leaders to embed privacy and ethics by design
will reduce regulatory risks and build trust with customers and partners. As AI’s influence expands, responsible stewardship that balances bold innovation with ethical imperatives is essential to safeguarding individuals and society alike.
Category | Key Practices | Examples & Tools | Benefits |
---|---|---|---|
Data Governance Frameworks | – Clear ownership & collaborative control – Automated data classification & role-based access – Centralized data catalogs – Start small and scale thoughtfully | – Atlan’s community-led governance approach – Symantec’s AI-driven DLP tools | – Maintains data integrity – Minimizes unauthorized access – Enables audit readiness – Aligns governance with business value |
AI-Specific Risk & Privacy Impact Assessments | – Conduct Data Protection Impact Assessments (DPIAs) – Use data catalogs for lineage and usage visibility – Address LLM risks (memorization, bias) – Adopt AI risk management frameworks (ISO/IEC 27001 amendment, OECD) – Promote AI literacy among teams | – HiddenLayer AI security platform – TrustWorks AI governance tools | – Identifies and mitigates privacy risks – Ensures continuous compliance – Enhances oversight of AI models – Empowers responsible AI management |
Transparency & Ethical AI Deployment | – Label AI-generated content appropriately – Avoid over-labeling minor AI enhancements – Design AI for explainability – Foster leadership accountability (CTOs, CIOs) | – LinkedIn and Meta AI content labeling practices – RSM US findings on AI integration challenges | – Builds trust with users – Supports regulatory compliance – Facilitates auditing – Drives ethical AI culture |
Leadership & Strategic Imperatives | – Champion privacy-by-design – Balance innovation with ethics – Develop flexible, scalable governance frameworks – Foster culture of accountability and collaboration | – KPMG 2025 survey on ethical AI investments – EU AI Act mandates on AI literacy | – Reduces regulatory risks – Promotes responsible AI use – Ensures adaptability to evolving laws – Aligns technical and business teams |
AI as a Tool for Enhancing Data Privacy and Security
AI as a Tool for Enhancing Data Privacy and Security
AI is often viewed as a double-edged sword in cybersecurity, but it can also be a powerful ally in defending data privacy. Its ability to perform real-time analysis and automate complex processes is shifting privacy protection from a largely manual, reactive task to a proactive, scalable system. This transformation is critical as businesses contend with increasingly sophisticated cyber threats and complex regulatory demands.
AI-driven Anomaly Detection: Catching Breaches Early
A key strength of AI lies in its capacity to detect anomalies—subtle deviations in user behavior or network traffic that may signal unauthorized access or data breaches. Unlike traditional rule-based systems, AI models learn the typical patterns for each user and system role, enabling them to flag unusual activity promptly.
For instance, Veza reports that 90% of organizations now utilize AI-enhanced access control systems to enforce the principle of least privilege, ensuring users access only the data they need. Deep learning techniques, such as neural networks, are increasingly employed to monitor large-scale networks and sensor data in real time, identifying even faint irregularities that could indicate intrusion attempts (IECE Transactions, 2025).
This dynamic detection is essential as cybercriminals themselves harness AI to mutate malware and evade static defenses (SentinelOne, 2025). However, AI is not infallible; false positives can overwhelm security teams, and attackers are developing AI-driven techniques sophisticated enough to mimic legitimate user behavior. These challenges highlight why AI should be integrated as part of a layered defense strategy rather than relied upon as a standalone solution.
Automated Compliance Monitoring and Encryption Management: Scaling Privacy Protections
The growing complexity of privacy regulations—including GDPR, CCPA, and the EU AI Act—makes manual compliance untenable for most organizations. AI-powered compliance tools are becoming indispensable in this landscape.
These tools leverage machine learning to continuously monitor data flows and system configurations, automatically detecting potential policy violations or exposure risks. They can analyze vast bodies of regulatory text and anticipate upcoming changes, helping companies stay ahead of compliance deadlines (CentralEyes, 2025).
Platforms like OneTrust, DataGrail, and Vanta incorporate AI-driven analytics to provide organizations with a comprehensive, 360-degree view of their data landscape. This visibility helps identify vulnerabilities, manage vendor risk, and ensure adherence to privacy policies (Enzuzo, 2025).
On the encryption front, AI is revolutionizing key management by predicting vulnerabilities and automating key lifecycle operations. Companies such as Fortanix are integrating quantum-resistant algorithms into AI-powered encryption platforms, preparing for the future threats posed by quantum computing (Fortanix, 2025). This proactive approach is vital as traditional cryptographic methods face unprecedented risks.
Despite these advances, AI tools must be integrated within existing cybersecurity frameworks. According to Lumenalta, a structured privacy strategy that blends AI automation with established measures like encryption, multi-factor authentication (MFA), and intrusion detection systems is essential for operational resilience and regulatory compliance.
Limitations and Risks: Why AI Alone Isn’t Enough
AI is not a silver bullet for data privacy. The technology introduces new privacy risks that businesses must carefully manage. For example, AI models trained on sensitive data can inadvertently leak information or be exploited by attackers. The DeepSeek AI data breach in 2025 exposed chat histories, API keys, and backend details due to an unsecured AI application, illustrating these vulnerabilities (HawkShield.ai, 2025).
Additionally, AI’s often opaque decision-making can conceal biases or unfair practices, undermining trust and potentially violating privacy laws. Ethical AI use demands regular audits for fairness and transparency, alongside rigorous data anonymization and encryption protocols (Qualys, 2025).
The regulatory environment is evolving rapidly to address these concerns. The upcoming California AI Transparency Act and the 2025 update to the NIST Privacy Framework emphasize the necessity of rigorous AI risk assessments and alignment with cybersecurity best practices (Frost Brown Todd, 2025; NIST, 2025). Organizations that neglect AI governance risk significant legal penalties and reputational harm.
Finally, AI-driven privacy tools cannot replace human expertise. Skilled privacy professionals and cybersecurity teams remain crucial to interpret AI findings, make strategic decisions, and manage complex threats beyond AI’s current capabilities (Solutions Review, 2025).
In summary, AI is a powerful enabler for enhancing data privacy and security—offering real-time anomaly detection, automated compliance monitoring, and advanced encryption management. However, it is not a panacea. Businesses must deploy AI-driven privacy tools as components of a comprehensive, integrated cybersecurity framework that includes human oversight, ethical governance, and continuous adaptation to emerging threats. This balanced approach is essential to harness AI’s potential while safeguarding the privacy rights foundational to trust in today’s digital economy.
Aspect | Description | Examples / References | Challenges / Risks |
---|---|---|---|
AI-driven Anomaly Detection | Detects subtle deviations in user behavior or network traffic to identify unauthorized access or breaches in real time. | Veza: 90% organizations use AI-enhanced access control; IECE Transactions (2025): neural networks for large-scale monitoring. | False positives can overwhelm teams; attackers mimic legitimate behavior; should be part of layered defense. |
Automated Compliance Monitoring | Uses machine learning to continuously monitor data flows, system configurations, and regulatory changes to ensure compliance. | Platforms: OneTrust, DataGrail, Vanta; CentralEyes (2025): anticipates regulatory changes. | Needs integration with existing frameworks; complexity of evolving privacy regulations. |
Encryption Management | AI predicts vulnerabilities and automates encryption key lifecycle management, incorporating future-proof algorithms. | Fortanix (2025): quantum-resistant AI-powered encryption. | Traditional cryptography faces quantum threats; requires blending with other security measures. |
Limitations and Risks | AI models can leak sensitive data, reflect biases, and conceal unfair practices, necessitating audits and ethical governance. | DeepSeek AI breach (2025); Qualys (2025): need for transparency and anonymization; upcoming regulations: California AI Transparency Act, NIST Privacy Framework update (2025). | Legal penalties and reputational harm if governance is neglected; AI cannot replace human expertise. |
Human Oversight | Essential for interpreting AI findings, decision-making, and managing complex threats. | Solutions Review (2025): importance of skilled professionals alongside AI tools. | AI tools alone insufficient; human expertise critical for operational resilience. |
Looking Ahead: Future Trends and Evolving Challenges in AI and Data Privacy
Looking Ahead: Future Trends and Evolving Challenges in AI and Data Privacy
As we move deeper into 2025, the next frontier of AI and data privacy is rapidly taking shape. AI advancements are not only transforming business operations but also reshaping how regulators approach personal data protection. For organizations, understanding these evolving dynamics is critical to staying compliant and preserving customer trust in an increasingly complex landscape.
Anticipated AI Advancements and Their Impact on Privacy Enforcement
AI’s development continues at an unprecedented pace, with generative models and autonomous agents becoming more sophisticated and embedded in everyday business workflows. For example, AI-driven automation is expected to significantly reduce administrative burdens, including in disaster recovery and cybersecurity, as companies deploy AI-powered backup and monitoring systems (TechNewsWorld, 2025).
However, this growing reliance on AI introduces heightened risks. Threats such as data poisoning—where attackers manipulate training data to mislead AI models—and hallucinations, where AI generates plausible but false information, present new enforcement challenges (Deloitte Insights, 2025).
In response, regulators worldwide are intensifying oversight. Many governments now require “privacy and security by design” as a foundational principle in AI risk management frameworks (Dentons, 2025). This means businesses must build AI systems with privacy embedded from inception, rather than treating it as an afterthought.
AI is also increasingly leveraged for real-time privacy protection. Automated systems can monitor sensitive data flows, detect vulnerabilities, and prevent leaks on a scale beyond manual capabilities (Lumenalta, 2025). Yet, integrating AI into existing cybersecurity frameworks demands careful balancing. AI tools must enhance privacy safeguards without disrupting workflows or introducing new attack surfaces.
Evolution of Privacy Regulations: Biometric Data, AI Transparency, and Collective Rights
Privacy laws are expanding rapidly, both in number and scope, with a sharp focus on AI-specific risks. Several U.S. states are leading the charge by enacting statutes addressing biometric data collection and use. For instance, Washington state requires explicit consumer consent before biometric identifiers are enrolled in databases, with enforcement by the state Attorney General (JDSupra, 2025). Pennsylvania is poised to introduce legislation mandating retail and entertainment venues disclose their biometric data practices.
AI transparency is another regulatory priority. California’s evolving privacy framework explicitly covers AI-generated data, with upcoming mandates requiring detailed documentation of generative AI training datasets (Hinshaw Law, 2025). Illinois and Rhode Island are considering bills imposing rigorous risk assessments and strict liability on AI developers, especially those working with large models, signaling a move toward heightened accountability (Inside Privacy, 2025).
A growing emphasis on collective privacy rights recognizes that AI’s inference and profiling abilities can affect groups beyond individual data subjects (Clifford Chance, 2025). This shift challenges traditional privacy frameworks that focus solely on individual rights.
As these regulations multiply, businesses face a patchwork of divergent laws—from comprehensive U.S. state statutes to evolving frameworks in Europe and Asia—making compliance increasingly complex.
Navigating the Uncertainties: Balancing Innovation, Ethics, and Global Cooperation
One of the most complex issues in AI governance is balancing innovation with privacy protection and ethical responsibility. Businesses seek to leverage AI’s transformative potential—such as faster insights, automation, and personalization—without crossing ethical boundaries or exposing users to harm.
Biometric identification technologies are becoming more widespread, but public demand for ethical transparency and scrutiny around their use is growing (Bill Franks, 2025). Ethical dilemmas also emerge from AI inference capabilities; algorithms can derive sensitive insights beyond the data explicitly provided, raising concerns about consent, fairness, and potential bias.
The fragmented regulatory environment further complicates these challenges. With over half of U.S. states enacting their own privacy laws and additional regulations emerging worldwide, organizations must adopt flexible, scalable governance frameworks that can adapt to evolving requirements (Cloud Security Alliance, 2025).
Cross-border enforcement remains a significant hurdle. For example, Italy’s data protection authority fined Clearview AI €20 million for illicit data collection, but enforcement stalled due to lack of international agreements (IAPP, 2025). Conversely, smaller nations like Singapore are influencing global AI norms through collaborations with AI safety institutes and regional partners, demonstrating the power of multilateral efforts.
Global initiatives such as the 2025 Paris AI Action Summit emphasize an open, ethical, and human-centric approach to AI development, aiming to embed accountability and transparency into AI ecosystems worldwide (Data for Policy, 2025). However, achieving harmonized standards will require patience, humility, and careful balancing of innovation speed, data sovereignty, climate impact, and public trust.
Key Takeaways for Businesses
- Privacy-by-design is essential: Embed privacy and security principles into AI systems from the start to meet rising regulatory expectations.
- Prepare for biometric data scrutiny: Regularly audit biometric data collection and consent mechanisms, especially in states with new or pending laws.
- Invest in AI transparency and fairness: Conduct routine AI model audits to identify bias and document training data sources, anticipating disclosure requirements.
- Adopt flexible governance frameworks: Build adaptable compliance programs capable of navigating a mosaic of state, national, and international privacy laws.
- Engage in ethical AI practices: Go beyond legal compliance by integrating ethical assessments into AI deployment, particularly for inference and profiling use cases.
- Monitor global standards and cooperation: Stay informed on international developments affecting cross-border data flows and enforcement, and engage in industry coalitions where feasible.
We stand at a pivotal moment where the promise of AI must be balanced with responsible stewardship. Organizations that anticipate and adapt to these evolving challenges will not only mitigate risks but also build lasting trust with customers and regulators. The future of AI and data privacy hinges on aligning technological innovation with societal values.
Category | Trend/Challenge | Details | Business Implications |
---|---|---|---|
AI Advancements | Generative Models & Autonomous Agents | Increasing integration in workflows, reducing administrative burdens like cybersecurity and disaster recovery | Opportunity to improve efficiency but requires embedding privacy and security by design |
AI Risks | Data Poisoning & Hallucinations | Manipulation of training data and generation of false information pose enforcement challenges | Need for robust AI risk management and monitoring systems |
Regulatory Response | Privacy and Security by Design | Mandated as foundational in AI risk frameworks globally | Businesses must build privacy into AI systems from inception |
Privacy Protection | AI for Real-Time Monitoring | AI tools detect vulnerabilities and prevent data leaks at scale | Integration must balance enhanced safeguards without disrupting workflows |
Privacy Laws | Biometric Data Regulations | State laws require consumer consent and disclosure for biometric data use (e.g., Washington, Pennsylvania) | Regular audits and compliance with consent mechanisms necessary |
Privacy Laws | AI Transparency Requirements | Mandates to document AI training data and conduct risk assessments (e.g., California, Illinois, Rhode Island) | Implement AI model audits and maintain documentation for compliance |
Privacy Rights | Collective Privacy Rights | Recognition that AI profiling affects groups beyond individuals | Adopt frameworks addressing group privacy impacts |
Governance | Fragmented Regulatory Environment | Patchwork of diverse laws across US states and globally | Develop flexible, scalable compliance programs |
Ethics | Balancing Innovation, Ethics, and Privacy | Ethical concerns around biometric use, AI inference, fairness, and bias | Integrate ethical assessments beyond legal compliance |
Global Cooperation | Cross-Border Enforcement Challenges | Enforcement hindered by lack of international agreements; smaller nations leading norms | Engage in global coalitions and monitor international developments |
Global Initiatives | Paris AI Action Summit 2025 | Focus on ethical, human-centric AI with accountability and transparency | Align AI practices with emerging global standards |
Key Takeaways | Privacy-by-Design | Embed privacy principles into AI systems from the start | Meet rising regulatory expectations |
Key Takeaways | Biometric Data Scrutiny | Audit biometric data collection and consent regularly | Ensure compliance with state laws |
Key Takeaways | AI Transparency & Fairness | Conduct AI model audits and document training data | Prepare for disclosure and bias mitigation |
Key Takeaways | Flexible Governance | Adopt adaptable compliance frameworks | Navigate complex regulatory environments effectively |
Key Takeaways | Ethical AI Practices | Incorporate ethical assessments, especially for profiling | Enhance trust and responsible AI use |
Key Takeaways | Monitor Global Standards | Stay informed on international laws and cooperate globally | Support cross-border data flow compliance |