AI in Cybersecurity: Transforming Threat Detection and Defense
- Introduction: Why AI Is a Game Changer in Cybersecurity Threat Detection
- Introduction: Why AI Is a Game Changer in Cybersecurity Threat Detection
- The Escalating Threat Landscape: Volume, Sophistication, and Velocity
- Why Traditional Security Approaches Are Falling Short
- AI: The Transformative Force in Threat Detection and Prevention
- Setting the Stage for Smarter Cyber Defense
- Foundations of AI-Powered Threat Detection: Core Technologies and Mechanisms
- Foundations of AI-Powered Threat Detection: Core Technologies and Mechanisms
- Supervised, Unsupervised Learning, and Anomaly Detection: The Triad of Machine Learning in Cybersecurity
- Deep Learning and Natural Language Processing: Extracting Intelligence from Complexity
- Hybrid Models: Bridging Rule-Based Systems with AI for Robust Defense
- Why This Matters: Beyond Technology to Strategic Cyber Defense
- Technical Deep Dive: Architecture and Performance Metrics of AI Cybersecurity Systems
- Technical Deep Dive: Architecture and Performance Metrics of AI Cybersecurity Systems
- Architectural Foundations: From Data Ingestion to SOC Integration
- Evaluating Performance: Beyond Accuracy
- The Achilles’ Heel: Data Quality, Feature Selection, and Retraining
- Real-World Examples: Endpoint Protection and Real-Time Threat Analysis
- Final Thoughts
- Practical Applications and Case Studies: AI in Real-World Cyber Threat Detection and Prevention
- Practical Applications and Case Studies: AI in Real-World Cyber Threat Detection and Prevention
- AI in Action: Detecting Ransomware, Phishing, Insider Threats, and Dark Web Surveillance
- Industry Case Studies: AI’s Quantifiable Impact on Breach Reduction and Incident Response
- Automating Threat Neutralization and Vulnerability Patching: The Next Frontier
- Operational Considerations: Integration and Collaboration Challenges
- Balancing Promise and Peril: Limitations, Risks, and Ethical Considerations of AI in Cybersecurity
- Balancing Promise and Peril: Limitations, Risks, and Ethical Considerations of AI in Cybersecurity
- Limitations of AI Threat Detection: Adversarial Attacks, Automation Overreliance, and Bias
- Privacy Implications and the Imperative for Explainable AI (XAI)
- Ethical Dilemmas: Surveillance, Accountability, and Governance
- The Indispensable Role of Human Intelligence
- Key Takeaways
- Comparative Analysis: AI-Powered Threat Detection Versus Traditional Cybersecurity Approaches
- Comparative Analysis: AI-Powered Threat Detection Versus Traditional Cybersecurity Approaches
- Detection Speed and Adaptability to Emerging Threats
- False Alarm Rates and Resource Efficiency
- The Hybrid Approach: Best of Both Worlds?
- Cost-Benefit Considerations and Organizational Readiness
- Final Thoughts: Navigating the AI Cybersecurity Frontier
- Future Outlook: Emerging Trends and the Evolving Landscape of AI in Cybersecurity
- Future Outlook: Emerging Trends and the Evolving Landscape of AI in Cybersecurity
- Advances in AI Technologies: Deep Learning, Real-Time Adaptation, and Threat Intelligence Integration
- The AI Arms Race: AI-Powered Cyberattacks and Autonomous Defense Systems
- Regulatory and Standardization Efforts: Navigating Governance in a Complex Terrain
- Recommendations for Practitioners: Staying Ahead through Learning, Ethics, and Collaboration

Introduction: Why AI Is a Game Changer in Cybersecurity Threat Detection
Introduction: Why AI Is a Game Changer in Cybersecurity Threat Detection
What if you knew that the window between the disclosure of a new vulnerability and its exploitation by attackers has shrunk from months to just five days? This is not a hypothetical scenario—it is the stark reality in 2025. Traditional cybersecurity defenses, built for a slower and less complex threat landscape, are struggling to keep up with an increasingly rapid and high-stakes battlefield.
The Escalating Threat Landscape: Volume, Sophistication, and Velocity
Cyber threats are escalating not only in volume but also in sophistication and speed. Over 30,000 new vulnerabilities were disclosed worldwide in the past year alone—a 17% increase from previous figures. This surge is driven by the ever-expanding digital footprint: remote work, cloud adoption, and the explosion of IoT devices have vastly increased attack surfaces, exposing more endpoints and data flows to malicious actors.
Attackers have evolved beyond static tactics. They now weaponize machine learning to mutate malicious code in real time, effectively evading traditional signature-based detection systems. The imminent rise of quantum computing poses an additional threat, with the potential to break existing encryption standards in hours rather than millennia. Consequently, critical sectors such as healthcare, manufacturing, finance, and government are under relentless pressure to defend themselves.
The stakes are enormous. Cybercrime is projected to cost the global economy $10.5 trillion annually by 2025, exceeding many conventional economic threats. For small and medium-sized businesses, the impact is often fatal: 60% fail within six months following a breach. Even large corporations like Boeing and American Express have recently suffered multi-million-dollar incidents, underscoring that no organization is immune.
Why Traditional Security Approaches Are Falling Short
Conventional cybersecurity tools—firewalls, signature-based antivirus, and periodic vulnerability scans—were never designed for this hyper-agile adversary. The timeline from vulnerability disclosure to exploitation has compressed twelvefold in just a few years, leaving organizations with minimal margin for error or delay.
Moreover, the sheer scale of data and security events overwhelms the limited pool of skilled analysts and threat hunters. Only about 14% of organizations feel confident they have adequate cybersecurity talent, while two-thirds report moderate-to-critical skills gaps. Budget constraints and organizational resistance to adopting newer technologies further compound this challenge.
Traditional methods also tend to rely on static configurations and reactive strategies. Ransomware attacks have evolved to target critical suppliers, triggering cascading disruptions across supply chains. Phishing remains the most prevalent attack vector, accounting for over one-third of attacks in recent quarters. Despite widespread adoption of multi-factor authentication and zero-trust models, attackers continue to bypass defenses through stolen credentials and automated reconnaissance.
AI: The Transformative Force in Threat Detection and Prevention
This is where artificial intelligence comes into play—not as a panacea, but as a transformative enabler that fundamentally shifts cybersecurity paradigms. AI-powered threat detection systems analyze vast volumes of network traffic, user behavior, and endpoint data in real time, identifying subtle anomalies that evade traditional tools.
For instance, Darktrace leverages machine learning to detect nuanced indicators of compromise across complex environments, alerting security teams to “invisible intruders” before they inflict damage. IBM’s Watson for Cybersecurity automates incident response workflows, significantly reducing breach containment times. Predictive analytics enable organizations to uncover vulnerabilities before threat actors do, facilitating proactive defense rather than reactive firefighting.
AI’s capacity to process and correlate diverse data streams at scale is critical in an environment where threats—including AI-driven malware—mutate dynamically. Recent surveys indicate that over 70% of security professionals believe AI solutions outperform traditional tools in blocking advanced cyberattacks.
However, AI adoption is not without challenges. Overreliance on AI risks sidelining essential human judgment, and the absence of comprehensive AI governance frameworks may introduce new vulnerabilities. Emerging threats such as data poisoning attacks targeting AI models themselves require vigilant defense. Successful integration demands a balanced approach—leveraging AI’s speed and scale while preserving expert human oversight.
Setting the Stage for Smarter Cyber Defense
In summary, the cybersecurity landscape of 2025 demands an evolution beyond legacy defenses. The explosive volume and velocity of attacks, combined with a critical shortage of skilled personnel, render traditional methods insufficient. Artificial intelligence offers a powerful means to enhance threat detection and prevention, but must be adopted thoughtfully, with due regard for its capabilities and limitations.
As we delve deeper, this article will explore how AI is reshaping threat detection, examine the practical capabilities it brings, and discuss the ethical and operational considerations organizations must address to build resilient, forward-looking cybersecurity defenses. The game has changed—understanding AI’s role is no longer optional but essential for survival in today’s high-stakes cyber battleground.
Aspect | Details |
---|---|
Vulnerability Exploitation Window | Shrunk from months to 5 days (2025) |
New Vulnerabilities Disclosed (Past Year) | Over 30,000 (17% increase) |
Cybercrime Economic Cost (Projected 2025) | $10.5 trillion annually |
SMBs Failure Rate Post-Breach | 60% fail within six months |
Confidence in Cybersecurity Talent | Only 14% of organizations confident |
Skills Gap in Cybersecurity | Two-thirds report moderate-to-critical gaps |
Most Prevalent Attack Vector | Phishing (over one-third of attacks) |
AI Adoption Benefits | Real-time anomaly detection, automated incident response, predictive analytics |
Notable AI Tools | Darktrace, IBM Watson for Cybersecurity |
Security Professional Belief in AI | Over 70% say AI outperforms traditional tools |
Challenges of AI Adoption | Overreliance risks, lack of governance, data poisoning threats |
Foundations of AI-Powered Threat Detection: Core Technologies and Mechanisms
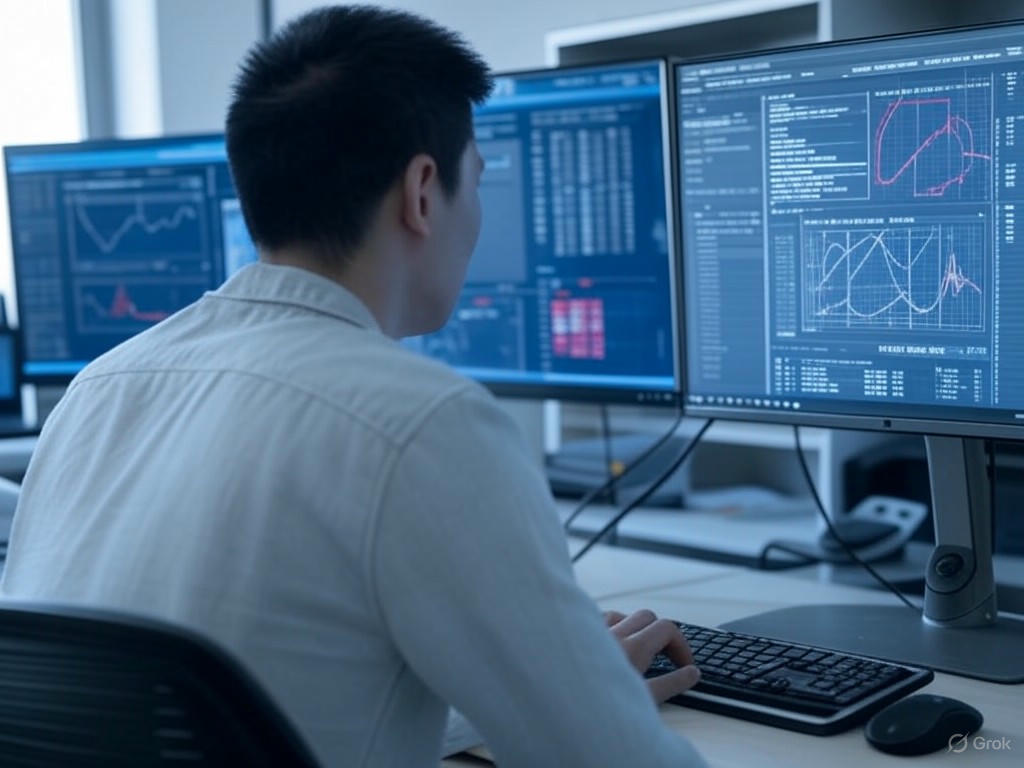
Foundations of AI-Powered Threat Detection: Core Technologies and Mechanisms
What enables AI to sift through mountains of cyber data and pinpoint threats that humans might miss? The answer lies in a strategic blend of machine learning techniques, deep learning architectures, and natural language processing (NLP). Together, these technologies identify patterns, anomalies, and early warning signals within complex digital environments. This fusion marks a fundamental shift away from traditional cybersecurity methods toward more adaptive, intelligent defenses.
Supervised, Unsupervised Learning, and Anomaly Detection: The Triad of Machine Learning in Cybersecurity
At the core of AI-driven threat detection are machine learning (ML) methods tailored to different data types and security objectives.
-
Supervised Learning depends on labeled datasets—similar to training a security guard by showing examples of known threats versus safe behavior. It excels at recognizing known attack signatures and classifying activities as malicious or benign. However, its limitation is the dependence on extensive, high-quality labeled data, which can be scarce or outdated in a rapidly evolving threat landscape.
-
Unsupervised Learning works without explicit labels, detecting patterns or clusters in unlabeled data. It flags deviations indicative of novel or unknown threats, making it essential for spotting zero-day attacks. This approach is especially valuable in complex environments such as industrial IoT, where traditional detection methods often fail. For instance, an unsupervised model might detect unusual traffic spikes or unexpected device behaviors that do not match any known profile, triggering alerts for investigation.
-
Anomaly Detection is a specialized form of unsupervised learning focused on identifying deviations from a defined “normal” baseline. Imagine a seasoned detective familiar with a neighborhood’s typical activity; any outlier—like a stranger lurking at odd hours—warrants scrutiny. In cybersecurity, anomaly detection algorithms establish behavior baselines for networks, users, or devices, then continuously monitor for deviations that might signal intrusion, data exfiltration, or insider threats.
Darktrace’s multi-layered AI system exemplifies this blend by employing various machine learning techniques to deliver behavioral analytics, real-time detection, and autonomous response across an organization’s digital estate. This multi-pronged approach mitigates the risks of relying solely on one method and adapts faster to evolving attack tactics compared to traditional signature-based systems.
Deep Learning and Natural Language Processing: Extracting Intelligence from Complexity
While classical ML techniques are powerful, deep learning (DL) elevates threat detection by leveraging artificial neural networks capable of modeling complex relationships in data.
Deep learning algorithms excel at recognizing subtle patterns hidden in vast datasets—ranging from network traffic logs to endpoint telemetry—that conventional methods might miss. For example, DL models can analyze sequences of system calls or network packets to identify sophisticated malware behaviors or lateral movements within a network. These models continuously refine their understanding, improving detection accuracy over time.
Natural Language Processing (NLP), a branch of AI focused on understanding human language, plays a critical role in combating cyber threats that use linguistic channels—such as phishing emails, social engineering, and fake news campaigns.
NLP-powered systems analyze the text, context, and sentiment of communications to detect:
- Suspicious email language patterns deviating from normal correspondence.
- Social engineering tactics manipulating recipients through crafted narratives.
- Impersonation or spear-phishing attacks with near-human precision.
For example, BytePlus ModelArk’s large language models identify nuanced cues in emails or messages, dramatically reducing false positives and enabling security teams to focus on genuine threats.
Hybrid Models: Bridging Rule-Based Systems with AI for Robust Defense
Despite the surge in AI adoption, traditional rule-based systems—relying on predefined signatures or heuristics—remain foundational in cybersecurity. The true innovation lies in hybrid models that combine the strengths of both approaches.
Think of a hybrid system as a seasoned security team supported by an AI assistant. Rules handle known, well-understood threats efficiently and reliably, while AI models address ambiguous or novel cases through behavior baselining and pattern recognition.
Behavior baselining is akin to establishing a “normal rhythm” for network and user activities. When AI detects deviations—such as an employee accessing sensitive files at unusual hours or a device communicating with unexpected external servers—it raises alerts for further analysis or triggers automated interventions.
Pattern recognition enables AI to identify recurring tactics, techniques, and procedures (TTPs) used by attackers, even when exact signatures are unknown. This capability is vital for keeping pace with rapidly evolving threat actors who constantly modify their methods to bypass detection.
Hybrid AI systems also support adaptive defense by continuously learning from new data and integrating human expertise. Security Operations Centers (SOCs) increasingly leverage these models to reduce alert fatigue, automate incident response, and accelerate threat hunting. For instance, Microsoft’s Security Copilot combines identity, endpoint, and network telemetry to prioritize alerts and suggest remediation, reducing analyst triage time by up to 40%.
Why This Matters: Beyond Technology to Strategic Cyber Defense
The convergence of supervised and unsupervised learning, deep learning, NLP, and hybrid models forms the backbone of modern AI-powered threat detection. This multi-layered approach is not merely a technological upgrade—it fundamentally transforms how organizations anticipate, detect, and respond to cyber threats.
By analyzing vast, heterogeneous datasets in near real-time, AI systems provide critical early warning signals in a landscape where attackers exploit every second of delay. Yet, AI is not infallible; challenges such as false positives, adversarial evasion, and evolving attack vectors require ongoing human oversight and continual model refinement.
In sum, AI-powered threat detection technologies offer a powerful toolkit to navigate the complexity and scale of modern cyber threats. Success depends on integrating these tools thoughtfully—combining machine precision with human judgment—and maintaining vigilance around both the promise and limitations of AI in cybersecurity defense.
AI Technology | Description | Key Features | Examples / Applications |
---|---|---|---|
Supervised Learning | ML method using labeled datasets to classify and detect known threats | Relies on labeled data, recognizes known attack signatures, classifies activities as malicious or benign | Detecting known malware, classifying network traffic |
Unsupervised Learning | ML method without labeled data to find patterns and clusters | Detects unknown or novel threats, flags anomalies, useful in complex environments like industrial IoT | Spotting zero-day attacks, identifying unusual device behavior |
Anomaly Detection | Specialized unsupervised learning focusing on deviations from normal baselines | Establishes normal behavior baselines, monitors deviations indicating intrusion or insider threats | Detecting unusual network activity, insider threat detection |
Deep Learning (DL) | Uses neural networks to model complex data relationships | Recognizes subtle patterns in large datasets, improves detection accuracy over time | Analyzing system calls, detecting sophisticated malware and lateral movement |
Natural Language Processing (NLP) | AI branch for understanding human language in cybersecurity context | Analyzes text, context, sentiment; detects phishing, social engineering, impersonation | Phishing email detection, social engineering identification (e.g., BytePlus ModelArk) |
Hybrid Models | Combination of rule-based systems and AI for robust defense | Integrates predefined signatures with AI behavior baselining and pattern recognition, supports adaptive defense | Microsoft Security Copilot, automated incident response, reduced alert fatigue |
Technical Deep Dive: Architecture and Performance Metrics of AI Cybersecurity Systems
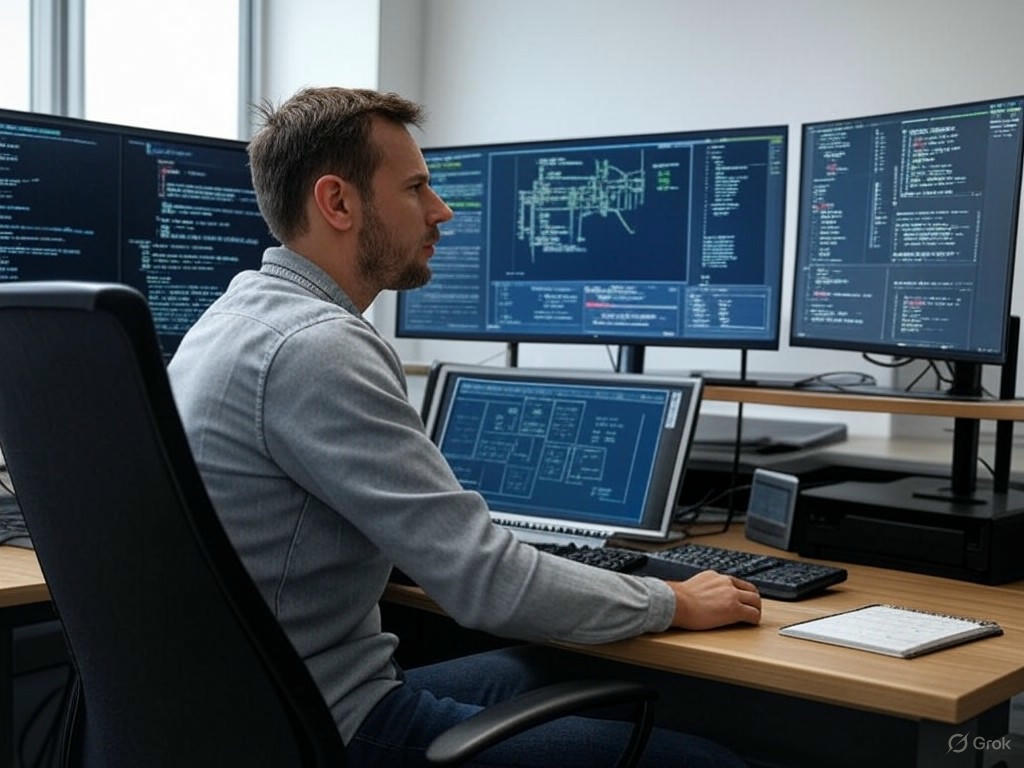
Technical Deep Dive: Architecture and Performance Metrics of AI Cybersecurity Systems
How do AI-driven cybersecurity platforms function beneath the surface, and which metrics truly capture their effectiveness? Let’s delve deeper into the architecture and evaluation criteria that define these advanced systems.
Architectural Foundations: From Data Ingestion to SOC Integration
At the heart of AI cybersecurity systems lies a sophisticated ecosystem that orchestrates data ingestion pipelines, model training workflows, inference engines, and seamless integration with Security Operations Centers (SOCs). Success depends not just on building powerful models, but on creating a dynamic environment where AI continuously learns, adapts, and responds in real time.
Data Ingestion Pipelines
Modern AI platforms ingest massive volumes of security telemetry from endpoints, networks, cloud environments, and identity systems. These raw data streams undergo normalization, enrichment, filtering, and efficient routing across hybrid infrastructures. Specialized Security Data Pipeline Platforms (SDPPs) address this complexity, ensuring AI models receive clean, contextualized data without overwhelming processing resources. For example, agentic AI use cases—where multi-agent systems autonomously hunt and respond to threats—rely heavily on these pipelines to deliver timely, actionable intelligence.
Model Training Workflows
Effective threat detection models require carefully curated datasets blending real-world telemetry with synthetic data. This hybrid approach compensates for limited labeled examples and enhances model generalization across diverse attack patterns. Given the rapid evolution of cyber threats, continuous retraining is essential. Automated workflows must integrate fresh threat intelligence, cleanse training data of biases or inaccuracies, and recalibrate model parameters to prevent drift and maintain detection fidelity.
Inference Engines and Hardware Acceleration
Inference—the real-time application of trained models to identify threats—demands ultra-low latency and high throughput. Specialized AI inference hardware, such as AMD’s Versal AI Core adaptive SoCs, delivers the compute power necessary at the edge or in data centers. These platforms accelerate neural processing for critical tasks like network anomaly detection and endpoint behavior analysis, enabling near-instantaneous response. The rise of edge computing means inference engines embedded within endpoint protection solutions reduce reliance on cloud connectivity, minimizing detection lag and improving resilience in disconnected environments.
Integration with Security Operations Centers (SOCs)
AI systems operate as integral components within SOC workflows rather than standalone tools. Advanced AI copilots—like Microsoft’s Security Copilot—combine identity, endpoint, and network telemetry to prioritize alerts, reduce false positives, and recommend remediation actions. Far from replacing human analysts, these copilots amplify their capabilities, cutting time spent on routine triage by up to 40%. Real-time AI threat analysis frameworks feed contextual insights directly into SOC dashboards, enabling faster, more informed decision-making in high-pressure environments.
Evaluating Performance: Beyond Accuracy
Assessing AI cybersecurity systems requires a nuanced set of performance metrics that reflect operational realities beyond mere accuracy.
- Detection Accuracy: High true positive rates are vital, but must be balanced against alert volume to prevent analyst overload.
- False Positive Rate: Excessive false alarms erode trust and cause alert fatigue. For instance, DataDome’s bot protection platform achieves an exceptionally low false positive rate of 0.01%, striking an effective balance between security and user experience.
- False Negative Rate: Missing threats can have catastrophic consequences. Models must be tuned to minimize blind spots, especially against novel or polymorphic attacks.
- Inference Latency: Real-time detection hinges on low-latency inference. Advances in AI hardware and edge deployment help reduce delays, enabling immediate containment of threats.
- Scalability: Cybersecurity environments generate petabytes of data daily. Systems must scale horizontally across cloud and on-premises infrastructure without degradation in performance or accuracy.
AI-driven platforms like IBM Watson for Cybersecurity monitor over 150 billion security events daily, exemplifying the scale and responsiveness necessary for modern defense.
The Achilles’ Heel: Data Quality, Feature Selection, and Retraining
Even the most advanced AI architectures are only as effective as the data they process and the features they learn from. This remains one of the most persistent challenges in cybersecurity AI.
Data Quality
Poor data governance leads to noisy, incomplete, or biased datasets, which cause model drift, hallucinations, and misaligned insights. Organizations rushing AI adoption often neglect robust data governance frameworks, risking compliance violations and security gaps. Identifying sensitive data and enforcing privacy through masking and anonymization is critical, especially when handling personally identifiable information (PII) or payment card data (PCI).
Feature Selection Complexities
Selecting predictive features is a moving target. Endpoint behaviors, network flows, identity attributes, and cloud telemetry each provide unique threat indicators. Effective feature engineering demands deep domain expertise to identify signals that reliably correlate with malicious activity, avoiding overfitting to current attack signatures that would reduce adaptability to emerging threats.
Model Retraining and Adaptation
Cyber adversaries continuously refine tactics, techniques, and procedures, compressing the window between vulnerability disclosure and exploitation to just days. AI models must be retrained frequently with updated threat intelligence and patched training data. Automated retraining pipelines, incorporating human-in-the-loop validation, strike a balance between agility and reliability. For example, Emsisoft’s endpoint protection solutions use behavior blockers enhanced with machine learning to reduce false positives during software updates, illustrating effective retraining and adaptation.
Real-World Examples: Endpoint Protection and Real-Time Threat Analysis
Endpoint Detection and Response (EDR) platforms embed AI engines capable of analyzing behavioral telemetry locally. Leveraging adaptive SoCs and neural accelerators, these systems detect ransomware and zero-day exploits with near-zero latency—even in disconnected environments.
On the network front, Darktrace’s AI-driven cyber defense continuously analyzes network traffic, identifying subtle deviations indicative of supply chain attacks or insider threats. Their platform fuses cloud, IoT, and traditional infrastructure telemetry into a unified threat picture, exemplifying the evolving complexity and integration in AI cybersecurity architectures.
Final Thoughts
AI’s promise in cybersecurity is tremendous but grounded in engineering rigor and operational discipline. Robust architectures combining advanced data pipelines, scalable inference engines, and human-centric SOC collaboration form the backbone of effective AI-driven defense.
Evaluations must move beyond accuracy to encompass false positive management, inference speed, and scalability. Meanwhile, persistent challenges around data quality, feature selection, and continuous retraining require ongoing attention and investment.
As attackers increasingly weaponize AI, defenders must respond with systems designed not only to react but to anticipate and adapt—always with transparency and ethical oversight. This is the critical frontier where technology meets trust, defining the future of cybersecurity.
Performance Metric | Description | Example / Note |
---|---|---|
Detection Accuracy | Measures true positive rates to capture actual threats effectively. | Must balance with alert volume to avoid analyst overload. |
False Positive Rate | Rate of incorrect alerts that are not threats. | DataDome achieves 0.01% false positive rate to reduce alert fatigue. |
False Negative Rate | Rate of missed threats or blind spots. | Critical to minimize, especially for novel or polymorphic attacks. |
Inference Latency | Time taken for real-time threat detection using AI models. | Low latency enabled by AI hardware and edge deployment. |
Scalability | Ability to handle massive data volumes without performance loss. | IBM Watson monitors over 150 billion security events daily. |
Practical Applications and Case Studies: AI in Real-World Cyber Threat Detection and Prevention
Practical Applications and Case Studies: AI in Real-World Cyber Threat Detection and Prevention
How effective is AI at catching threats the moment they emerge—before damage spirals out of control? The cybersecurity landscape of 2025 offers compelling evidence that AI-powered detection and prevention systems are not just hype; they are vital tools reshaping how organizations defend themselves against sophisticated attacks.
AI in Action: Detecting Ransomware, Phishing, Insider Threats, and Dark Web Surveillance
Ransomware remains one of the most devastating cyber threats, causing operational downtime and significant financial losses. Although ransomware detections dropped by 18% from 2023 to 2024 (Kaspersky, 2025), targeted attacks by groups like RansomHub continue to pose serious risks. AI platforms such as Palo Alto Networks’ Cortex XDR and XSIAM leverage behavioral threat protection to prevent all known ransomware variants “out of the box” and rapidly identify novel attack patterns (Unit 42, 2025). This enables detection often within seconds, dramatically reducing the breach window and limiting damage.
Phishing attacks are escalating at an alarming rate, surging 1,000% between 2022 and 2024 (CybelAngel, 2025; VIPRE, 2025). Attackers exploit multiple channels—including email, SMS, and QR codes—to harvest credentials and infiltrate networks. AI’s role here is multifaceted: it powers adaptive email filters and fuels behavioral training platforms like Hoxhunt, which customize phishing simulations based on user roles and behaviors. This dynamic defense builds user resilience as attackers automate real-time adaptations in their campaigns (Hoxhunt, 2025).
Insider threat monitoring benefits immensely from AI’s capacity to analyze vast amounts of data in real time. Advanced models sift through logs, communications, and access patterns to flag anomalous behavior invisible to traditional rule-based systems. Additionally, natural language processing (NLP) models like DarkBert specialize in scanning dark web content to interpret intent, enabling security teams to detect discussions about planned attacks or leaked credentials as they happen (LinkedIn, 2025).
Dark web monitoring has evolved from a passive intelligence-gathering task into a proactive security measure. AI-driven tools continuously collect, classify, and prioritize threats from underground forums and marketplaces, integrating these insights into intrusion detection systems, endpoint protection, and firewalls (Cyble, 2025; Web Asha Technologies, 2025). This comprehensive approach alerts organizations to compromised credentials or emerging threats days or weeks before they manifest in active attacks.
Industry Case Studies: AI’s Quantifiable Impact on Breach Reduction and Incident Response
The financial sector exemplifies AI’s transformative impact. CardGuard Bank’s implementation of AI-based behavioral analytics resulted in a 70% reduction in credit card fraud within the first year, alongside an 80% drop in false positives and customer complaints (DigitalDefynd, 2025). Likewise, global insurer GlobalTrust streamlined operations and enhanced customer satisfaction by automating manual review processes with AI (DigitalDefynd, 2025).
In healthcare—where cyberattacks have surged 300% since 2015 (Chief Healthcare Executive, 2025)—AI is revolutionizing defense strategies. Automated threat detection cuts incident response times significantly, while AI-enabled workflow prioritization reduces administrative overhead, allowing security teams to focus on critical threats (HealthTech Magazine, 2025; Exploding Topics, 2025). Given healthcare’s regulatory environment and the sensitivity of protected health information (PHI), AI solutions are designed to ensure explainability and compliance.
National security agencies leverage AI’s ability to process vast, heterogeneous data sources to detect cyber-physical threats. Emerging AI-powered Security Operations Centers (SOCs) deploy “agentic AI”—multi-agent systems capable of semi-autonomous threat hunting and response—accelerating detection and mitigation cycles (Google Cloud, 2025). This is especially crucial for sectors reliant on IoT and operational technology, where expanding attack surfaces demand rapid, adaptive defense (Darktrace, 2025; Industrial Cyber, 2025).
Automating Threat Neutralization and Vulnerability Patching: The Next Frontier
Detection is only half the battle. AI-guided remediation is becoming essential, automating threat neutralization and patch management to close vulnerabilities before exploitation. Crucially, these AI tools integrate seamlessly with existing security infrastructure—such as endpoint protection, firewalls, and intrusion detection systems—without disrupting ongoing operations (Strapi, 2025).
Predictive analytics enable organizations to prioritize patch deployment based on exploit likelihood and business impact, a significant advantage given that the time between vulnerability disclosure and exploitation has shrunk to just five days (Darktrace, 2025). AI-driven orchestration platforms can automatically isolate infected endpoints, deploy patches, or execute tailored countermeasures with minimal human intervention.
Despite this automation, human-AI collaboration remains indispensable. AI’s strength lies in processing scale and speed, but human analysts provide crucial context, creativity, and ethical judgment—essential for interpreting nuanced threats and minimizing false positives (USC Viterbi, 2024; RONIN OWL CTI, 2025). The future of cybersecurity operations is a symbiotic partnership: AI augments human expertise, freeing analysts from repetitive tasks and empowering them to focus on strategic defense.
Operational Considerations: Integration and Collaboration Challenges
Deploying AI in cybersecurity is far from plug-and-play. Organizations face challenges including legacy system integration, data quality issues, and organizational readiness. Successful AI adoption requires robust governance frameworks to manage risks such as model bias, explainability gaps, and vulnerabilities within AI itself (Optiv, 2025; RSAC Conference, 2025).
Fostering collaboration between AI systems and human analysts demands transparency and trust. Security teams require AI tools that provide clear rationales for alerts and remediation recommendations to enable informed, timely decisions. Continuous training and feedback loops enhance AI accuracy and build analyst confidence, creating a virtuous cycle of improvement (Dark Reading, 2025).
In practice, successful AI-powered cybersecurity deployments represent a well-orchestrated ecosystem—integrating AI-driven threat intelligence, automated response, and human insight—that collectively reduces breach windows, limits damage, and strengthens organizational resilience.
AI in cybersecurity is no longer speculative; it is a proven force multiplier against ever-more sophisticated threats. Yet, as we embrace AI’s capabilities, we must remain vigilant about operational complexities and ethical imperatives, ensuring these powerful tools serve as trustworthy guardians of our digital infrastructure.
Application Area | AI Solutions / Tools | Impact / Benefits | Sources / Examples |
---|---|---|---|
Ransomware Detection | Palo Alto Networks Cortex XDR, XSIAM | Behavioral threat protection prevents known variants; rapid novel attack detection within seconds; reduces breach window | Kaspersky (2025), Unit 42 (2025) |
Phishing Prevention | Adaptive Email Filters, Hoxhunt Behavioral Training Platforms | Dynamic defense adapting to attack campaigns; customized phishing simulations based on user roles | CybelAngel (2025), VIPRE (2025), Hoxhunt (2025) |
Insider Threat Monitoring | AI Models analyzing logs, communications, DarkBert NLP for dark web scanning | Real-time anomaly detection beyond rule-based systems; interprets dark web intent for early threat warning | LinkedIn (2025) |
Dark Web Surveillance | AI-driven threat collection and classification tools | Proactive threat alerts integrating with IDS, endpoint protection, firewalls; early warning of compromised credentials | Cyble (2025), Web Asha Technologies (2025) |
Financial Sector | AI-based Behavioral Analytics | 70% reduction in credit card fraud; 80% drop in false positives and complaints | DigitalDefynd (2025), CardGuard Bank |
Healthcare Sector | Automated Threat Detection, AI-enabled Workflow Prioritization | 300% surge in attacks since 2015; faster incident response; reduced admin overhead; compliance with PHI regulations | Chief Healthcare Executive (2025), HealthTech Magazine (2025), Exploding Topics (2025) |
National Security Agencies | Agentic AI Multi-agent Systems in SOCs | Semi-autonomous threat hunting and response; accelerated detection and mitigation; crucial for IoT and OT security | Google Cloud (2025), Darktrace (2025), Industrial Cyber (2025) |
Threat Neutralization and Patch Management | AI-guided remediation, predictive analytics, orchestration platforms | Automated vulnerability patching and endpoint isolation; prioritizes patches by exploit likelihood; minimal human intervention | Strapi (2025), Darktrace (2025) |
Operational Considerations | Governance frameworks, transparency tools, continuous training | Manages model bias, explainability, AI vulnerabilities; builds trust and collaboration between AI and human analysts | Optiv (2025), RSAC Conference (2025), Dark Reading (2025) |
Balancing Promise and Peril: Limitations, Risks, and Ethical Considerations of AI in Cybersecurity
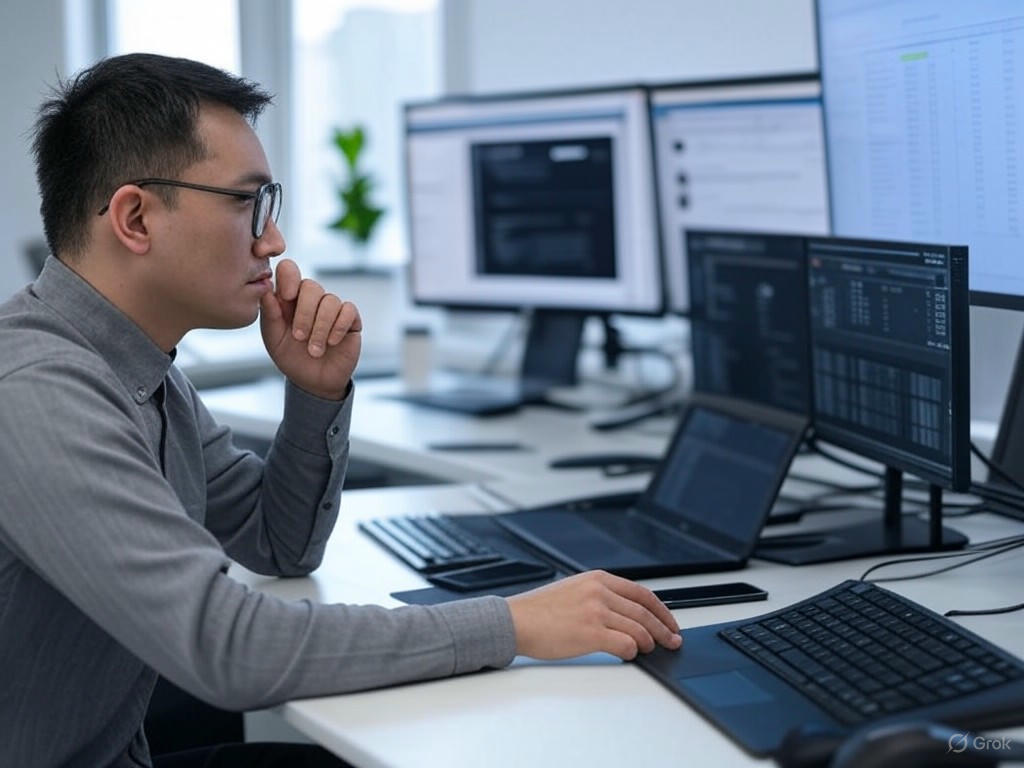
Balancing Promise and Peril: Limitations, Risks, and Ethical Considerations of AI in Cybersecurity
What happens when the very technology designed to protect us becomes a double-edged sword? AI’s expanding role in cybersecurity is undeniably transformative, yet it is far from infallible. Understanding its limitations, risks, and ethical challenges is critical to building resilient defenses that neither blindly trust automation nor sacrifice privacy and accountability.
Limitations of AI Threat Detection: Adversarial Attacks, Automation Overreliance, and Bias
AI-powered cybersecurity tools have revolutionized threat detection by processing massive datasets and identifying patterns invisible to human analysts. However, this capability brings inherent vulnerabilities. Adversarial attacks, where attackers subtly manipulate inputs to deceive AI models, pose a growing threat. For example, polymorphic malware that rewrites itself dynamically can evade AI detection, as can synthetic personas that infiltrate networks weeks before discovery.
The National Institute of Standards and Technology (NIST) has issued guidelines to defend AI systems against such adversarial tactics, but effective mitigation remains an ongoing challenge. These attacks exploit AI’s reliance on learned patterns, highlighting the fragility beneath its apparent sophistication.
Overreliance on AI automation also creates dangerous blind spots. The 2017 Equifax breach, which exposed sensitive data of 147 million Americans, was partly due to a missed patch—something an automated system might have flagged, but human oversight failed to catch. Experts emphasize that automation should augment, not replace, human vigilance. Excessive dependence on AI risks eroding critical thinking and situational awareness, ultimately weakening cybersecurity defenses.
Bias in AI training data is another critical concern. AI models inherently reflect the data they learn from—if that data is skewed, the system’s decisions will be too. In cybersecurity, bias can lead to disproportionate scrutiny or neglect of certain user groups or behaviors, potentially exacerbating inequalities or missing novel attack vectors. Studies reveal such bias manifests in subtle ways—for instance, when voice recognition fails with elderly users or facial recognition struggles with diverse demographics.
Addressing bias requires diverse development teams, continuous data audits, and a data-centric approach to AI system design. Without these measures, AI risk detection tools may unwittingly perpetuate systemic flaws, undermining fairness and effectiveness.
Privacy Implications and the Imperative for Explainable AI (XAI)
AI’s hunger for data powers its threat detection capabilities but also raises profound privacy concerns. Extensive monitoring of network traffic and user behavior increases the risk of unauthorized data exposure. Privacy regulations like the GDPR and CCPA impose strict requirements on data collection, storage, and use, challenging organizations to balance security needs with individual rights.
AI can be a double-edged sword: it automates privacy protections—such as anomaly detection and adaptive encryption—while simultaneously amplifying surveillance capabilities. Integrating AI thoughtfully with traditional security protocols helps safeguard sensitive information without disrupting operations or eroding trust.
Transparency is pivotal for trust and effective incident response. Conventional AI models often operate as “black boxes,” leaving cybersecurity professionals uncertain about why specific alerts were triggered. This opacity undermines confidence and complicates remediation efforts.
Explainable AI (XAI) offers a path forward by providing interpretable insights into AI decision-making. Research shows that rule-based and tree-based models are preferred in intrusion detection systems (IDS) for their interpretability. Techniques like LIME and SHAP provide explanations for individual predictions, though computational overhead can challenge real-time deployment.
XAI not only enhances operational effectiveness but also supports compliance with emerging regulations demanding transparency and accountability in AI-driven cybersecurity systems.
Ethical Dilemmas: Surveillance, Accountability, and Governance
AI-driven cybersecurity thrusts organizations into ethically complex territory. How much surveillance is justified to protect networks without infringing on privacy? This tension between security and privacy is not theoretical—it plays out in daily operational decisions.
Approximately 75% of companies now deploy AI-driven cybersecurity solutions, yet many struggle to establish clear ethical frameworks. Transparency about AI’s inner workings, ownership of errors, and robust data protection are foundational principles gaining traction. Some organizations employ sandbox environments to ethically test AI-driven attack simulations, limiting collateral damage and preserving ethical standards.
Accountability presents another thorny issue. AI systems operate based on training data and human-crafted algorithms, but when failures occur, AI cannot be held responsible. This raises complex questions about liability—whether it lies with developers, operators, or organizations. Clear governance frameworks are essential, incorporating risk assessments, human oversight models (such as human-in-the-loop or human-on-the-loop), and adherence to legal standards.
Governance becomes more challenging as AI capabilities evolve rapidly, often outpacing regulatory frameworks. Corporate leaders increasingly prioritize AI governance within cybersecurity strategies, aligning programs with international standards like the EU’s AI Act. Navigating the triad of privacy, cybersecurity, and legal compliance is now a strategic imperative.
The Indispensable Role of Human Intelligence
Despite AI’s sophistication, human expertise remains irreplaceable. AI excels at processing data and suggesting actions, but cybersecurity demands contextual judgment, ethical reasoning, and strategic foresight beyond algorithmic reach.
The future of cybersecurity is a collaboration between humans and machines. Successful defense strategies combine AI’s speed and scale with human intuition and accountability. As Andrew Keen observes, the AI security revolution “won’t be won by technology alone.” Organizations must cultivate interdisciplinary teams where AI augments human decision-making rather than supplants it.
Key Takeaways
- AI threat detection is powerful but vulnerable to adversarial attacks, bias, and complacency from automation overreliance.
- Privacy protections must be integral to AI cybersecurity solutions, balancing security objectives with regulatory mandates.
- Explainable AI is critical to fostering transparency, trust, and effective incident response.
- Ethical frameworks and governance models must evolve alongside AI capabilities to ensure accountability and respect for individual rights.
- Human intelligence remains central to cybersecurity defenses, providing oversight, ethical guidance, and strategic direction.
Navigating AI in cybersecurity demands a sober, evidence-based approach that embraces both its promise and its perils. Only by acknowledging limitations, risks, and ethical complexities can we harness AI’s transformative potential safely and responsibly.
Aspect | Description | Key Points |
---|---|---|
Limitations of AI Threat Detection | AI tools revolutionize detection but face adversarial attacks, automation overreliance, and bias | Adversarial attacks (e.g., polymorphic malware), human oversight needed, bias in training data affects fairness |
Privacy Implications & Explainable AI (XAI) | AI’s data use raises privacy concerns; XAI improves transparency and trust | Compliance with GDPR/CCPA, balance surveillance with privacy, XAI techniques like LIME/SHAP, preferred interpretable models |
Ethical Dilemmas | Challenges in surveillance ethics, accountability, and governance | Transparency, liability issues, ethical testing environments, governance aligned with standards like EU AI Act |
Role of Human Intelligence | Human expertise essential alongside AI | Contextual judgment, ethical reasoning, AI augments human decision-making not replaces |
Key Takeaways | Summary of core insights on AI in cybersecurity | Vulnerabilities in AI, privacy protections, importance of XAI, evolving ethical frameworks, centrality of human oversight |
Comparative Analysis: AI-Powered Threat Detection Versus Traditional Cybersecurity Approaches
Comparative Analysis: AI-Powered Threat Detection Versus Traditional Cybersecurity Approaches
What truly distinguishes AI-powered threat detection from traditional cybersecurity methods? To answer this, we must examine key performance metrics: detection speed, adaptability to emerging threats, false alarm rates, and resource efficiency. We’ll also explore the merits of hybrid strategies and practical considerations around adoption.
Detection Speed and Adaptability to Emerging Threats
Traditional cybersecurity still relies heavily on signature-based detection and heuristic rules—a largely static defense line that struggles to keep pace in a threat landscape evolving by the hour. According to BitLyft, these conventional systems depend on manual investigation and prioritization of alerts, introducing latency that attackers readily exploit.
In sharp contrast, AI-powered systems operate on fundamentally different principles. Machine learning models analyze thousands of features simultaneously, identifying anomalous patterns that often defy human intuition. Hornetsecurity’s Advanced Threat Protection, for example, uses ML to rapidly detect zero-day exploits and fileless malware, frequently in near real-time.
John Keister, CEO of MixMode, summarizes this shift succinctly: “AI is shifting cybersecurity from reactive to predictive.” This transition is critical, as modern attacks—including hybrid and AI-driven phishing campaigns highlighted by Zscaler—are increasingly context-driven and adaptive.
The advantage in detection speed is not just theoretical. Industry benchmarks consistently demonstrate that AI systems identify threats faster, sometimes by orders of magnitude, compared to signature-based tools. This rapid response is vital to counter ransomware and lateral movement attacks, which can cripple networks within minutes.
False Alarm Rates and Resource Efficiency
False positives have long burdened traditional systems, resulting in alert fatigue among security analysts. Studies from ScienceDirect and ResearchGate reveal that signature-based detection often generates high false alarm rates due to rigid pattern matching, which struggles to distinguish benign anomalies from genuine threats.
AI approaches excel at reducing false positives through context-aware anomaly detection. By weighting multiple behavioral factors, AI systems can better discriminate between legitimate deviations and malicious activity. This precision not only bolsters security posture but also conserves valuable human analyst time—a critical benefit amid the persistent cybersecurity skills gap emphasized by the World Economic Forum and Cisco’s Cybersecurity Readiness Index 2025.
Resource efficiency extends beyond human capital. AI-powered solutions automate routine Security Operations Center (SOC) tasks such as alert triage and playbook execution, enabling security teams to focus on complex investigations. However, this efficiency comes with caveats: AI systems require substantial computational resources and ongoing model retraining, which can strain budgets and infrastructure if not managed carefully.
The Hybrid Approach: Best of Both Worlds?
Is it a binary choice between AI and traditional methods? Increasingly, the answer is no. Hybrid cybersecurity strategies that integrate AI with signature-based and heuristic techniques are emerging as the most pragmatic path forward.
Security firms like Xantrion and Optiv advocate for AI as a complementary layer within a broader security architecture. Barracuda Networks’ next-generation threat detection exemplifies this approach by employing multimodal AI to enhance URL inspection, while still leveraging established heuristics to filter known threats. This layered defense harnesses AI’s speed and adaptability without discarding the proven reliability of traditional detection.
Benchmarking data from BCU and Covatic’s recent research supports the effectiveness of hybrid models. These systems achieve superior detection accuracy and improved resilience against adversarial AI attacks by combining AI’s pattern recognition capabilities with heuristic guardrails that reduce false negatives.
Yet, hybrid adoption is not plug-and-play. Organizations face challenges including integration complexities, legacy system compatibility issues, and the shortage of AI-savvy cybersecurity professionals. The 2025 AI Cyber Benchmark by Wavestone reports that while 71% of organizations have adapted project lifecycle processes to manage AI risks, only 64% have formal AI security frameworks—highlighting readiness gaps that could hinder hybrid deployments.
Cost-Benefit Considerations and Organizational Readiness
From a cost-benefit standpoint, AI-powered cybersecurity solutions present a compelling case, albeit with nuanced trade-offs.
On the positive side, AI adoption correlates with measurable productivity gains. Syracuse University’s 2025 report projects global productivity growth of 7% annually over the next decade, driven in part by AI efficiencies. Coursera’s research forecasts the AI cybersecurity market soaring from $24.82 billion in 2024 to $146.52 billion by 2034, reflecting strong commercial momentum.
However, initial investments in AI infrastructure, skilled personnel, and governance frameworks can be substantial. A mere 4% of organizations globally have achieved ‘mature’ cybersecurity readiness, according to Help Net Security’s 2025 assessment. Cultural resistance and budget constraints remain significant barriers, with many companies hesitant to fully invest in advanced endpoint monitoring or AI-driven detection technologies.
Moreover, organizations face a paradox: the very AI that empowers defense is also exploited by attackers to mutate malware and bypass traditional filters, as SentinelOne warns. This escalating arms race demands continuous innovation and vigilance, driving ongoing operational costs.
Successful adoption hinges on several strategic factors:
-
Talent readiness: AI cybersecurity requires hybrid skill sets blending traditional security expertise with AI/ML proficiency. The scarcity of such talent remains a bottleneck.
-
Governance and ethics: Robust frameworks are essential to manage AI risks, uphold privacy, and ensure compliance with evolving data protection laws, as outlined by LexisNexis.
-
Incremental integration: Phased hybrid deployments that augment existing defenses instead of wholesale replacements minimize disruption and optimize return on investment.
-
Vendor partnerships: Collaborations with specialized AI innovators—such as DTEX partnering with AWS—can accelerate maturity and reduce implementation risks.
Final Thoughts: Navigating the AI Cybersecurity Frontier
The comparative landscape between AI-powered and traditional cybersecurity approaches is not a simple contest but a dynamic synthesis. AI’s superior speed, adaptability, and precision are undeniable, yet these advantages bring complexities in cost, expertise, and evolving threat paradigms.
Hybrid models that judiciously combine AI with traditional heuristics currently offer the most resilient and efficient strategy for threat detection. Still, the journey requires organizational commitment to skill development, governance, and continuous innovation.
As someone deeply involved in architecting AI systems for cybersecurity, I believe the question is no longer whether to adopt AI—but how to do so thoughtfully, ethically, and strategically. The stakes have never been higher, and the time to act decisively is now.
Aspect | Traditional Cybersecurity | AI-Powered Threat Detection | Hybrid Approach |
---|---|---|---|
Detection Speed | Relies on manual investigation and signature-based detection, causing latency | Uses machine learning to detect threats in near real-time, including zero-day exploits | Combines AI’s rapid detection with traditional heuristic filtering for enhanced speed and reliability |
Adaptability to Emerging Threats | Largely static, struggles to keep pace with evolving threats | Predictive and context-driven, adapts to new and sophisticated attacks quickly | Integrates AI adaptability with proven heuristic methods to improve resilience |
False Alarm Rates | High false positives due to rigid pattern matching causing alert fatigue | Reduces false positives with context-aware anomaly detection and multi-factor weighting | Uses heuristic guardrails to reduce false negatives while leveraging AI precision |
Resource Efficiency | Heavy human analyst involvement, prone to alert fatigue | Automates routine SOC tasks, conserves analyst time but requires high computational resources | Balances automation with existing systems, though integration complexity may increase resource needs |
Cost and Investment | Lower initial investment but limited scalability and adaptability | High initial cost for infrastructure, talent, and ongoing retraining | Moderate costs with phased integration; requires skilled personnel and governance frameworks |
Organizational Readiness | Established but less effective against modern threats | Requires AI/ML expertise and governance, which are often scarce | Demands hybrid skill sets and incremental adoption strategies to minimize disruption |
Threat Landscape Suitability | Effective against known threats, less so for novel or AI-driven attacks | Effective against zero-day, fileless malware, and adaptive AI-driven threats | Offers robust defense combining strengths of both approaches against evolving threats |
Future Outlook: Emerging Trends and the Evolving Landscape of AI in Cybersecurity
Future Outlook: Emerging Trends and the Evolving Landscape of AI in Cybersecurity
What does the future hold for AI in cybersecurity? The trajectory is unmistakable: AI is transitioning from a powerful analytical tool to an increasingly autonomous agent, transforming both defensive and offensive cyber operations. However, this evolution presents a double-edged sword, requiring a nuanced grasp of technological innovations, emerging threats, and the ethical frameworks that must guide their use.
Advances in AI Technologies: Deep Learning, Real-Time Adaptation, and Threat Intelligence Integration
Cutting-edge deep learning architectures continue to push the boundaries of automated threat detection. By combining supervised, unsupervised, and reinforcement learning techniques, modern cybersecurity systems emulate digital immune systems—learning from every interaction to detect both known and novel attack patterns in real time. This continuous adaptation enhances vigilance and accelerates response capabilities, vital in a landscape where zero-day exploits and fileless malware evolve rapidly.
Integration with AI-enriched threat intelligence feeds is revolutionizing how organizations prioritize and respond to risks. Platforms like Anomali’s ThreatStream unify millions of indicators—from CVEs to dark web chatter—and apply AI-driven correlation to distill actionable insights. This centralization reduces alert noise, enabling security teams to focus resources on the most critical threats.
AI-powered systems are increasingly embedded within Security Operations Centers (SOCs), delivering real-time analytics and decision support. By automating routine tasks such as triage and alert prioritization, AI liberates human analysts to engage in complex investigations, fostering a symbiotic human-machine collaboration that strengthens overall cybersecurity posture.
The AI Arms Race: AI-Powered Cyberattacks and Autonomous Defense Systems
AI is not solely a defender’s asset—threat actors are leveraging it with alarming sophistication. AI-powered cyberattacks, including automated spear-phishing campaigns, polymorphic malware, and adaptive intrusion methods, are escalating in frequency and complexity. CrowdStrike reports adversaries now deploy AI to evade conventional detection and exploit vulnerabilities faster than ever before.
This dynamic fuels an ongoing arms race in AI security. Defensive teams are developing autonomous AI-driven response systems capable of detecting and mitigating threats instantaneously without human intervention. For instance, Lockheed Martin is pioneering AI solutions that autonomously counter threats targeting critical transportation infrastructure, highlighting how real-time AI defense safeguards high-stakes environments.
Nonetheless, autonomous systems are not a panacea. They introduce challenges such as false positives, unintended operational consequences, and adversarial tactics designed to deceive AI models. This interplay between AI-powered attackers and defenders demands relentless innovation and vigilance.
Regulatory and Standardization Efforts: Navigating Governance in a Complex Terrain
The expanding role of AI in cybersecurity has prompted regulatory bodies and industry groups to strengthen governance frameworks. The Cloud Security Alliance identifies five major cybersecurity laws becoming effective by 2025, emphasizing the imperative for compliance that balances innovation with risk mitigation.
Chief Information Security Officers (CISOs) must now integrate AI governance into their security strategies, ensuring transparency, accountability, and ethical AI use. This includes adherence to data privacy regulations like GDPR and CCPA, explainability requirements for AI decisions, and rigorous auditing mechanisms. Thought leaders at IBM and KPMG stress that ethical AI implementation must balance enhanced security with user privacy protections, bias mitigation, and misuse prevention.
Standardization efforts also focus on harmonizing threat intelligence sharing and AI model evaluation procedures to facilitate interoperability across platforms and organizations. Such unified frameworks are critical to prevent fragmented defenses and build collective resilience against evolving threats.
Recommendations for Practitioners: Staying Ahead through Learning, Ethics, and Collaboration
Navigating this rapidly evolving landscape requires more than advanced technology—it demands a proactive mindset centered on continuous learning and ethical stewardship.
-
Invest in ongoing education: AI in cybersecurity evolves at breakneck speed. Security teams must stay abreast of emerging techniques, threat trends, and best practices to maintain effectiveness.
-
Prioritize ethical AI use: Deploy AI systems transparently, incorporating mechanisms to detect and mitigate biases. Ensure AI-driven decisions are explainable and auditable to foster trust and accountability.
-
Foster human-machine synergy: While AI excels at processing vast data and automating routine tasks, human expertise remains indispensable for contextual judgment, strategic thinking, and ethical considerations.
-
Engage with regulatory developments: Proactively align AI cybersecurity initiatives with evolving laws and standards to ensure compliance and build stakeholder trust.
In essence, the future of AI in cybersecurity is a complex, dynamic interplay of innovation, adversarial ingenuity, regulatory oversight, and human values. Success will depend on embracing this complexity, recognizing that the strongest defense arises from the collaboration of smart machines and thoughtful people.
Aspect | Details |
---|---|
Advances in AI Technologies | Deep learning architectures combining supervised, unsupervised, and reinforcement learning for real-time threat detection; AI-enriched threat intelligence integration (e.g., Anomali’s ThreatStream); AI embedded in Security Operations Centers (SOCs) automating triage and alert prioritization. |
The AI Arms Race | AI-powered cyberattacks like automated spear-phishing, polymorphic malware, adaptive intrusion; Autonomous AI-driven defense systems (e.g., Lockheed Martin’s real-time countermeasures); Challenges include false positives and adversarial tactics. |
Regulatory and Standardization Efforts | Implementation of cybersecurity laws by 2025; AI governance focusing on transparency, accountability, ethical use; Compliance with GDPR, CCPA; Standardization of threat intelligence sharing and AI model evaluation for interoperability. |
Recommendations for Practitioners | Invest in ongoing education; Prioritize ethical AI use with bias mitigation and explainability; Foster human-machine synergy; Engage with regulatory developments for compliance and trust. |