AI in Government: Smart Cities, Services & Ethical Policy Planning
- Introduction: Why AI is a Game-Changer for Government and Urban Life
- Introduction: Why AI is a Game-Changer for Government and Urban Life
- The Significance of AI Adoption in Government
- Scope: Smart Cities, Public Services, and Policy Planning
- Navigating the Interplay of Possibility and Ethical Responsibility
- Provoking Reflection: Promise or Pitfall?
- Foundations of AI Technologies in Government Applications
- Foundations of AI Technologies in Government Applications
- Core AI Technologies Driving Government Innovation
- Technical Specifications Tailored to Government Needs
- Architectural Design in Municipal and Federal AI Systems
- Smart Cities: AI-Driven Urban Infrastructure and Sustainability
- Smart Cities: AI-Driven Urban Infrastructure and Sustainability
- AI, IoT, and Big Data: The Urban Brain and Nervous System
- Case Studies: Real-World AI Transformations in Cities
- Technical Hurdles: Data Fusion, Scalability, and Privacy
- Building Resilient, Sustainable Urban Ecosystems
- Final Thoughts
- Enhancing Public Services through AI: Efficiency, Transparency, and Citizen Engagement
- Enhancing Public Services through AI: Efficiency, Transparency, and Citizen Engagement
- Streamlining Bureaucracy with AI-Powered Automation and Virtual Assistants
- Predictive Analytics: Smarter Resource Allocation in Healthcare, Social Services, and Public Safety
- Data Integrity, Security, and Building Public Trust in AI Systems
- Navigating Workforce Challenges and Human-AI Collaboration
- AI in Policy Planning and Decision-Making: From Predictive Analytics to Ethical Governance
- AI in Policy Planning and Decision-Making: From Predictive Analytics to Ethical Governance
- Leveraging Machine Learning and Natural Language Processing for Evidence-Based Policymaking
- The Promise and Challenges of AI-Driven Impact Assessment
- Navigating Ethical Challenges: Bias, Transparency, and Accountability
- Toward Responsible AI Governance in Public Policy
- Conclusion: Balancing Technological Promise with Ethical Prudence
- Benchmarking AI Adoption: Comparative Analysis of Global Government Initiatives
- Benchmarking AI Adoption: Comparative Analysis of Global Government Initiatives
- Contrasting AI Strategies and Implementations
- Variances in Governance, Investment, and Partnerships
- Implications for Innovation, Societal Acceptance, and Risk Management
- Lessons Learned and Best Practices for Responsible Scaling
- Future Directions and Challenges: Navigating the Road Ahead for AI in Government
- Future Directions and Challenges: Navigating the Road Ahead for AI in Government
- Emerging Trends: Generative AI, Hybrid Cloud, and Citizen Interaction
- Unresolved Challenges: Privacy, Ethics, Workforce, and Trust
- Strategic Recommendations: Balancing Innovation with Responsible Stewardship
- The Road Ahead
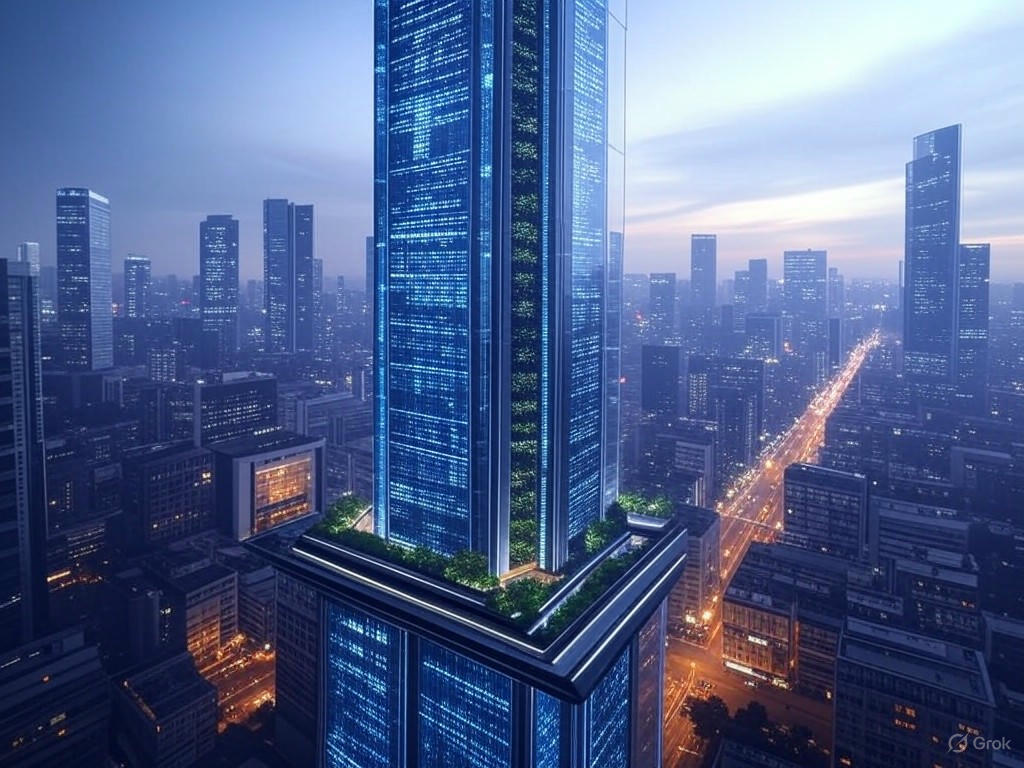
Introduction: Why AI is a Game-Changer for Government and Urban Life
Introduction: Why AI is a Game-Changer for Government and Urban Life
Why has artificial intelligence become a linchpin for the future of government and urban living? The answer lies in a decisive shift from cautious exploration to accelerated adoption across public sectors. After years of lagging behind the private sector, government agencies are now embracing AI not only as a tool but as a foundational technology to transform city management, public service delivery, and policy formulation.
The Significance of AI Adoption in Government
Recent U.S. federal government policy updates underscore a clear commitment to AI integration. For instance, the White House’s 2025 Fact Sheet outlines revised policies aimed at removing barriers to AI procurement and use, signaling a fundamental pivot: AI is no longer a futuristic experiment but a core driver of public service innovation.
The Office of Management and Budget (OMB) mandates agencies to accelerate AI adoption while embedding rigorous risk management for “high-impact AI” systems. This balance between rapid innovation and responsible deployment reflects a mature approach to integrating AI in government functions.
Key government technology trends reveal this momentum:
- Over 60% of public sector organizations plan to increase investments in automation by 2026, up from 35% in 2022.
- Challenges remain, including a shortage of skilled personnel and the complexity of modernizing legacy systems.
- The stakes extend beyond efficiency—AI offers pathways to economic competitiveness, national security, and enhanced citizen well-being, as emphasized in Executive Order 14179.
The GovTech market mirrors this surge, growing at a steady 15% annually. Innovations in generative AI, automation, and cybersecurity fuel this growth. Companies like ServiceNow are launching AI Agent platforms that streamline government workflows securely and comply with updated FedRAMP standards. These developments illustrate AI’s expanding and serious role in public sector digital transformation.
Scope: Smart Cities, Public Services, and Policy Planning
What does AI deployment look like in practice across cities and government offices? The focus has shifted from theoretical potential to tangible, real-time impact.
- In cities such as Hamburg and Beijing, AI platforms process social aid applications and connect medical institutions through cloud-based infrastructures, enabling more responsive and efficient public services.
- Lagos, Nigeria, is investing heavily in AI to address urban challenges, demonstrating this transformation’s global scope.
- Smart city AI has evolved from passive data collection to active decision-making. For example, “city brains” under development in over 500 Chinese cities autonomously adjust traffic signals to reduce congestion and optimize energy consumption in public buildings based on real-time demand.
- Policy planning is entering a new era with AI-driven analytics that predict social service needs, optimize resource allocation, and simulate urban futures. This goes beyond data crunching to harness predictive power for adaptive, evidence-based policymaking.
Navigating the Interplay of Possibility and Ethical Responsibility
With AI’s growing power comes an imperative for ethical stewardship. Transparency, accountability, and fairness are non-negotiable principles underpinning public trust.
- Clear communication about AI decision-making processes is critical, especially when systems influence benefits delivery, law enforcement, or public safety.
- A recent federal leaders’ roundtable emphasized ethical AI as central to preserving trust in government institutions.
- Challenges include risks from generative AI, such as misinformation, intellectual property concerns, and embedded biases within datasets.
- AI-driven “city brain” projects raise questions about inclusivity and the potential reinforcement of existing inequalities when urban futures are shaped by AI.
Emerging AI governance frameworks provide vital guardrails. These frameworks include legal regulations, ethical oversight boards, and robust data governance practices to prevent bias and safeguard privacy. Agencies must assess their readiness regarding data quality, infrastructure, and workforce capabilities before AI deployment.
Provoking Reflection: Promise or Pitfall?
As governments accelerate AI integration, critical questions arise:
- Are public institutions prepared to balance innovation with the imperative to protect citizens’ rights and societal values?
- Will AI in government become a tool for empowerment or a catalyst for new forms of exclusion?
Evidence shows enormous potential for AI to revolutionize government efficiency and urban life. Success, however, requires more than technology alone—it demands thoughtful governance, ethical foresight, and inclusive policymaking. At this inflection point, the challenge is clear: harness AI’s transformative power while safeguarding the democratic principles that underpin society.
Aspect | Details |
---|---|
Government AI Policy | White House 2025 Fact Sheet: Removing barriers to AI procurement and use |
OMB Directive | Mandates accelerated AI adoption with risk management for high-impact AI systems |
Investment Trends | 60%+ public sector organizations plan increased automation investments by 2026 (up from 35% in 2022) |
Challenges | Skilled personnel shortage, legacy system modernization complexity |
Strategic Stakes | Economic competitiveness, national security, enhanced citizen well-being (Executive Order 14179) |
GovTech Market Growth | 15% annual growth fueled by generative AI, automation, cybersecurity |
Example Companies | ServiceNow AI Agent platforms with secure, FedRAMP-compliant workflows |
Smart City Examples | Hamburg & Beijing: AI for social aid, medical connectivity; Lagos: urban AI investment; 500+ Chinese cities “city brains” traffic & energy optimization |
Policy Planning | AI-driven analytics for social needs prediction, resource allocation, urban futures simulation |
Ethical AI Principles | Transparency, accountability, fairness, clear communication |
Ethical Challenges | Misinformation, IP concerns, data bias, inclusivity in AI urban projects |
Governance Frameworks | Legal regulations, ethical oversight boards, data governance practices |
Critical Questions | Balancing innovation with rights protection, AI as empowerment or exclusion tool |
Foundations of AI Technologies in Government Applications
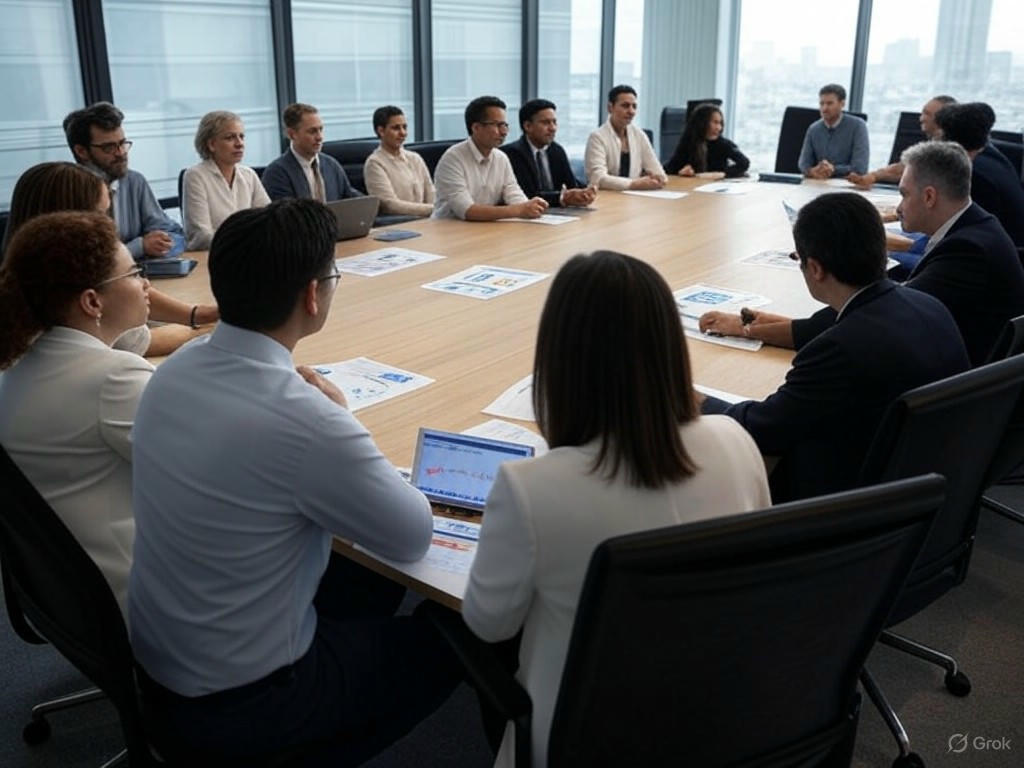
Foundations of AI Technologies in Government Applications
What fuels the rapid adoption of AI across government initiatives? At its core, a synergy of machine learning, natural language processing (NLP), computer vision, and the integration of Internet of Things (IoT) devices is driving transformation. These technologies convert vast streams of raw data into actionable insights that enhance city management and public service responsiveness.
Core AI Technologies Driving Government Innovation
Machine learning serves as the backbone of AI, enabling systems to identify patterns and predict outcomes from extensive datasets. Governments leverage machine learning to optimize traffic flows, forecast energy demand, and detect anomalies in public health surveillance. A prime example is Ouster BlueCity’s deployment of deep learning perception models that process lidar data from over 800 urban locations. Powered by NVIDIA Jetson AGX Orin modules, these edge devices perform real-time inference onsite, delivering responsive traffic management that reduces congestion and enhances road safety without the latency inherent in cloud-based systems.
Natural Language Processing has become integral to citizen engagement. Modern government chatbots employ advanced NLP models capable of understanding complex inquiries, offering multilingual support, and providing contextual recommendations. The UK’s HM Revenue and Customs chatbot, which handles millions of inquiries annually, exemplifies this evolution. By 2026, 60% of governments plan to prioritize business process automation through AI-powered agents, projecting billions in saved work hours and operational costs.
Computer vision revolutionizes smart city operations by enabling real-time analysis of visual data. AI-augmented surveillance cameras monitor crowd densities to prevent disasters, identify vacant parking spaces, and enforce social distancing protocols. The convergence of computer vision with IoT sensor networks forms intelligent, autonomous systems that perceive and react without human intervention. Deep neural networks detect individuals in public spaces to enable proactive public safety measures, reducing reliance on manual monitoring.
IoT integration acts as the sensory nervous system underpinning these AI technologies. With over 29 billion connected devices expected globally by 2030, governments harness IoT to capture granular environmental, traffic, and infrastructure data. When combined with AI—forming the AIoT paradigm—cities can self-optimize systems such as energy-efficient lighting and climate-conscious resource management. While Tesla’s Autopilot system is a landmark AIoT success in autonomous driving, municipal examples abound: smart sensors track air quality, monitor soil moisture for urban farming, and manage utilities with unprecedented precision.
Technical Specifications Tailored to Government Needs
Government AI applications face distinct technical demands compared to commercial deployments.
-
Data volume and quality: Public sector AI relies on diverse datasets, including sensor feeds, public records, and citizen inputs. These datasets require meticulous curation to ensure fairness and accuracy while complying with stringent privacy laws and transparency mandates such as GDPR.
-
Model interpretability: Explainable AI is essential for maintaining public trust and regulatory compliance. Unlike many private sector black-box models, government agencies demand transparency on how AI systems make decisions, especially in rights-impacting or safety-critical contexts. The White House Office of Management and Budget (OMB) stresses this in its memoranda, urging agencies to document AI decision processes and implement risk management frameworks.
-
Real-time processing: Many government functions—traffic control, emergency response, public safety—require low-latency decision-making. Edge computing decentralizes data processing to local nodes embedded in city infrastructure, minimizing latency and bandwidth constraints. For example, AI-powered edge devices on traffic cameras enable split-second adjustments of signal timings and rapid alerts to first responders.
Architectural Design in Municipal and Federal AI Systems
Government AI architectures typically feature layered, modular designs that prioritize scalability, security, and interoperability.
At the municipal level, smart city deployments usually begin with extensive sensor networks embedded in streetlights, traffic signals, transit vehicles, and infrastructure. These IoT sensors stream data to edge computing hubs where localized machine learning models analyze conditions in real time. Aggregated insights are then transmitted to centralized cloud platforms for comprehensive analytics and policy planning. This hybrid approach balances the need for immediate responsiveness with broader strategic oversight.
For instance, BlueCity’s smart traffic management system deploys lidar sensors feeding data to NVIDIA-powered edge modules that detect vehicles and pedestrians instantly. The system forecasts congestion and dynamically adjusts traffic signals to improve flow and safety. This edge intelligence reduces cloud dependency, critical for uninterrupted urban operations.
On the citizen engagement front, AI-enabled chatbots serve as frontline interfaces for public interaction. These systems integrate sophisticated NLP models with backend databases and workflows to manage tasks such as appointment scheduling, FAQ responses, and form processing. Approximately half of U.S. states have deployed such chatbots, streamlining communication, reducing wait times, and alleviating staff workloads. Architecturally, these chatbots leverage cloud-based NLP engines, secure data access layers, and continuous learning pipelines that refine responses based on citizen feedback.
At the federal level, AI systems are more complex, often involving multi-agency data sharing and stringent compliance frameworks. The OMB mandates cross-functional teams to oversee AI procurement, ensuring adherence to performance, transparency, and data governance standards before deployment. Continuous monitoring is required to detect and remediate unacceptable behaviors, safeguarding public trust in AI-driven government decisions.
In summary, the foundation of AI in government rests on the strategic integration of machine learning, NLP, computer vision, and IoT technologies. These are tailored to meet rigorous standards for data governance, model interpretability, and real-time processing. Far from theoretical constructs, these AI systems are actively reshaping urban management and citizen engagement. The ongoing challenge will be to balance innovation with accountability, ensuring AI serves as a responsible and equitable tool for public service transformation.
AI Technology | Government Applications | Examples | Technical Considerations |
---|---|---|---|
Machine Learning | Optimize traffic flows, forecast energy demand, detect anomalies in public health surveillance | Ouster BlueCity’s deep learning perception models processing lidar data with NVIDIA Jetson AGX Orin for real-time traffic management | Requires large datasets, edge computing for low latency, model interpretability for public trust |
Natural Language Processing (NLP) | Citizen engagement via chatbots, multilingual support, contextual recommendations | UK HM Revenue and Customs chatbot handling millions of inquiries; 60% of governments prioritizing AI-powered business process automation by 2026 | Integration with backend databases, continuous learning pipelines, cloud-based NLP engines |
Computer Vision | Real-time visual data analysis for crowd monitoring, parking space detection, social distancing enforcement | AI-augmented surveillance cameras with deep neural networks detecting individuals for public safety | Integration with IoT sensor networks, autonomous systems, real-time processing |
Internet of Things (IoT) | Capturing environmental, traffic, and infrastructure data; enabling AIoT for energy-efficient systems and resource management | Smart sensors monitoring air quality, soil moisture, utilities management; Tesla Autopilot as AIoT landmark | Massive device connectivity (29 billion by 2030), data curation, privacy compliance (e.g., GDPR) |
Smart Cities: AI-Driven Urban Infrastructure and Sustainability
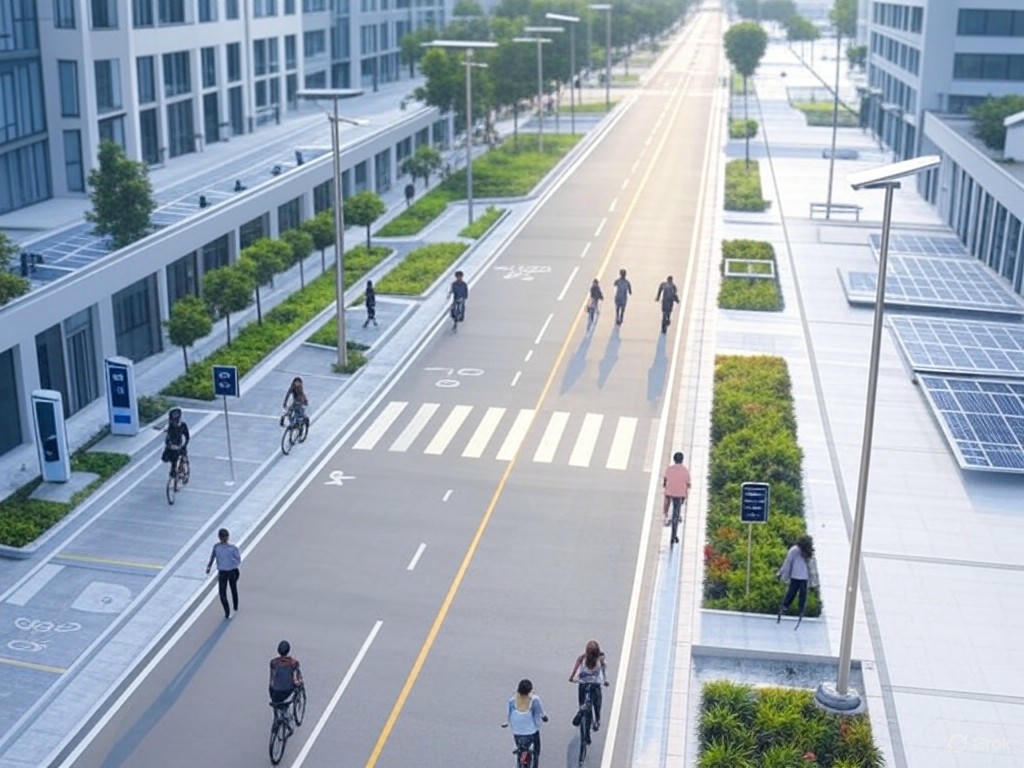
Smart Cities: AI-Driven Urban Infrastructure and Sustainability
What happens when the vast streams of data from IoT devices converge with the analytical power of artificial intelligence? The result is a transformative force reshaping how cities manage energy, traffic, public safety, and environmental health. By 2034, the AI in smart cities market is projected to exceed USD 460 billion—a clear indicator of the urgency and scale of urban challenges that AI seeks to address.
AI, IoT, and Big Data: The Urban Brain and Nervous System
IoT devices act as the sensory organs of a city—billions of sensors embedded in streetlights, vehicles, buildings, and infrastructure continuously capture data on everything from temperature and air quality to traffic volume. This data streams into cloud and edge computing platforms, where AI algorithms analyze patterns, detect anomalies, and generate real-time predictions.
For instance, smart grids equipped with IoT sensors monitor energy consumption down to the household level. AI then dynamically optimizes energy distribution to reduce waste and balance demand. In EcoVille, the SmartGrid AI platform achieved a 20% reduction in overall energy consumption alongside a 30% increase in renewable energy integration. This case exemplifies AI’s capacity to accelerate sustainable energy transitions while enhancing grid resilience.
Traffic management is another area where AI delivers significant impact. Cities like London and Dubai deploy AI systems that analyze live traffic feeds from cameras and sensors, adjusting signal timings and rerouting vehicles to alleviate congestion. These interventions have improved traffic flow efficiency by up to 40%, simultaneously reducing emissions and shortening commute times.
Public safety also benefits tremendously from AI’s ability to process diverse data streams. AI-powered video analytics detect unusual behavior or emerging threats, enabling faster emergency responses. In California, AI-coordinated drone fleets surveil wildfire zones, cutting response times by 58% and increasing survival rates by nearly one third.
Case Studies: Real-World AI Transformations in Cities
-
Technopolis: The HealthWatch system uses AI analytics to predict health emergency hotspots, improving response times by 40%. This predictive capability supports targeted health campaigns that mitigate outbreaks before escalation.
-
EcoVille: The success of SmartGrid AI in energy optimization highlights AI’s role in reducing urban carbon footprints while strengthening energy infrastructure.
-
Vista City: Facing overcrowded public transit, Vista City deployed TransitAI, which improved transit efficiency by 40% and significantly reduced commuter wait times, easing pressure on strained infrastructure amid rapid urban growth.
-
Kenya’s Ministry of Health: In partnership with IBM Watson, the SafeHealth AI system forecasts disease outbreak hotspots with 85% accuracy, enabling proactive interventions in vulnerable communities.
These examples underscore AI’s versatility in addressing complex urban challenges—from health and energy to transportation—while illustrating the intricacies involved in seamless system integration.
Technical Hurdles: Data Fusion, Scalability, and Privacy
Despite these advances, deploying AI in smart cities faces significant technical challenges. One major issue is sensor data fusion. IoT devices differ widely in type, quality, and communication protocols, complicating the aggregation of heterogeneous data streams into coherent inputs for AI models. Overcoming this requires robust middleware solutions and ongoing standardization efforts.
Scalability remains a critical concern. As urban sensor networks expand and data volumes surge, AI systems must maintain low latency and high accuracy. Emerging architectures that combine edge computing—processing data near its source—with cloud resources strike a balance between speed and computational power, enabling real-time responsiveness.
Privacy safeguards are paramount throughout AI deployment. The vast collection of personal and location data raises ethical and legal questions. Citizens’ concerns about surveillance and data ownership are legitimate. Regulatory frameworks such as the GDPR enforce stringent data protection standards, while cities experiment with encryption, anonymization, and blockchain technologies to enhance security.
Transparency is equally essential. AI decision-making in public services must be explainable and auditable to sustain public trust. The World Economic Forum emphasizes embedding human oversight and ethical principles into AI design to uphold fairness and accountability.
Building Resilient, Sustainable Urban Ecosystems
AI’s role in smart cities transcends operational efficiency to foster resilience and sustainability. Real-time monitoring and predictive maintenance enable early identification of infrastructure vulnerabilities, preventing failures that could disrupt essential services. For example, AI models can forecast the likelihood of bridge or water main failures, facilitating timely repairs that save costs and avert disasters.
Additionally, AI supports sustainability goals by optimizing resource consumption and reducing emissions. Smart lighting systems adjust brightness based on pedestrian presence, minimizing energy waste. Waste management leverages IoT sensors to track bin fill levels, allowing for efficient collection routes that decrease fuel use.
However, technology alone cannot guarantee success. Many smart city initiatives falter due to governance and social factors. Inclusive planning, community engagement, and equitable access are vital components. Without these, advanced infrastructures risk becoming “ghost grids”—technically sophisticated but disconnected from residents’ real needs.
Final Thoughts
Smart cities powered by AI, IoT, and big data represent a paradigm shift in urban living, offering unprecedented opportunities for sustainability, safety, and operational efficiency. Yet, realizing this potential requires balancing technological innovation with ethical responsibility, privacy protection, and the cultivation of civic trust.
To fully harness AI’s transformative power, cities must invest not only in advanced infrastructure but also in transparent policies, multidisciplinary expertise, and inclusive governance models. Only through this comprehensive approach can urban ecosystems become truly resilient, sustainable, and responsive to the diverse needs of their inhabitants.
City/Entity | AI System | Function | Impact/Results |
---|---|---|---|
Technopolis | HealthWatch | Predict health emergency hotspots | Improved response times by 40% |
EcoVille | SmartGrid AI | Energy consumption optimization and renewable energy integration | 20% reduction in energy consumption; 30% increase in renewable energy integration |
Vista City | TransitAI | Improve public transit efficiency | 40% improvement in transit efficiency; reduced commuter wait times |
Kenya’s Ministry of Health | SafeHealth AI (in partnership with IBM Watson) | Forecast disease outbreak hotspots | 85% accuracy enabling proactive health interventions |
Enhancing Public Services through AI: Efficiency, Transparency, and Citizen Engagement
Enhancing Public Services through AI: Efficiency, Transparency, and Citizen Engagement
What if the frustration and delays traditionally associated with government services could be drastically reduced—not over years, but within months? This is not mere speculation. Governments worldwide are actively deploying AI to transform bureaucratic workflows and public service delivery, making systems more responsive, transparent, and citizen-centric.
Streamlining Bureaucracy with AI-Powered Automation and Virtual Assistants
Complex case processing workflows involving multiple approvals and handoffs have long challenged government agencies. AI-driven automation offers a powerful solution to these inefficiencies. A Boston Consulting Group report highlights that generative AI can reshape core government processing functions, enabling staff to make decisions faster and process cases more efficiently.
The benefits are tangible and rapid. Agencies implementing AI have reported significant cost savings and productivity improvements realized within weeks or months, not years. For example, Portugal’s public services employ an AI chatbot powered by ChatGPT-3.5, offering accessible, 24/7 assistance to citizens navigating bureaucratic procedures. This virtual assistant substantially reduces wait times and eases the burden on human staff, ensuring timely and accurate information delivery.
Similarly, India’s Ministry of Consumer Affairs uses conversational AI to expedite grievance redressal, enhancing responsiveness and fostering public trust. Beyond chatbots, advanced AI agents—referred to as “agentic AI” systems—are deployed at scale by agencies including the U.S. federal government. These systems triage common citizen problems, providing consistent, data-driven responses around the clock. Their infinite scalability streamlines public engagement without compromising accuracy.
Predictive Analytics: Smarter Resource Allocation in Healthcare, Social Services, and Public Safety
AI’s promise extends beyond automation into predictive analytics, where governments leverage vast datasets to optimize resource allocation and improve outcomes.
In healthcare, predictive models forecast patient inflows, enabling hospitals to optimize staffing and reduce wait times. Tools like Olive automate repetitive administrative tasks, freeing clinical staff to focus on patient care while reducing human error. Population health analytics further demonstrate AI’s potential: identifying high-risk patients and tailoring interventions has improved chronic disease control by nearly 16%, while saving $42 million annually by curbing unnecessary utilization. These insights empower evidence-based decision-making, transforming both patient experience and financial sustainability.
Social services are also embracing AI to enhance decision-making and resource coordination. Emerging AI-driven community resource data infrastructures enable social workers to concentrate on outcomes and impact rather than paperwork and case management. However, ethical and legal considerations remain paramount to ensure client privacy and mitigate biases.
Public safety agencies increasingly harness AI to shift from reactive to proactive strategies. With 90% of law enforcement agencies using AI for real-time crime prevention and resource management, technologies such as Real-Time Crime Centers and predictive policing analytics improve situational awareness and operational efficiency. This progress necessitates robust cybersecurity measures, especially as cyberattacks targeting government systems have surged.
Data Integrity, Security, and Building Public Trust in AI Systems
No discussion of AI in public services is complete without addressing data integrity and security. The quality and governance of data underpin AI’s effectiveness and legitimacy.
Alarmingly, only about 12% of organizations report having data of sufficient quality and accessibility for effective AI deployment. Governments are responding by prioritizing comprehensive data governance frameworks, increasing adoption from 60% to over 70% within a year.
Security is another critical front. AI-driven cyber threats are evolving rapidly, with attackers exploiting mainstream AI platforms. Government agencies invest in AI-based threat detection and automated incident response to safeguard sensitive information. For example, the U.S. Department of Health and Human Services is strengthening cybersecurity protections for electronic protected health information (ePHI), reflecting a broader trend of tightening digital defenses.
Building public trust also requires transparency and explainability. Federal mandates encourage agencies to procure AI systems that provide clear documentation and mechanisms for performance monitoring. Incorporating feedback loops from end users and stakeholders is essential to ensure AI systems operate fairly and effectively, especially given the high stakes of public service delivery.
Navigating Workforce Challenges and Human-AI Collaboration
The AI revolution in government is as much about people as technology. Despite the promise of automation, human expertise remains indispensable.
The civil service faces a pronounced AI skills gap, with many agencies still in early stages of AI maturity. Prioritizing AI literacy, specialist training, and cross-disciplinary collaboration is critical to maximizing AI’s benefits. According to McKinsey’s 2025 research, 87% of companies report skill gaps related to AI adoption, underscoring the urgency for workforce readiness.
Successful AI integration should be viewed as augmentation rather than replacement of human workers. For instance, AI virtual assistants handle routine queries, allowing human agents to focus on complex cases requiring empathy and judgment. This collaborative model enhances efficiency while preserving the human touch essential in public services such as healthcare and social work.
Government leadership plays a pivotal role in setting clear AI visions and governance frameworks. Empowered Chief Data and AI Officers (CDAIOs), who bridge technical, ethical, and operational considerations, are increasingly recognized as key drivers of responsible AI adoption.
AI applications across government services are advancing rapidly, transforming how citizens interact with their governments and how resources are deployed for maximum impact. Yet, the pathway is neither simple nor risk-free. Success hinges on high-quality data, robust security, transparent governance, and a workforce prepared to collaborate with AI. When these elements align, the result is a smarter, more responsive government—one that serves its people with greater efficiency, fairness, and trustworthiness.
AI Application Area | Examples / Implementations | Benefits | Challenges / Considerations |
---|---|---|---|
AI-Powered Automation & Virtual Assistants |
|
|
|
Predictive Analytics for Resource Allocation |
|
|
|
Data Integrity, Security & Public Trust |
|
|
|
Workforce Challenges & Human-AI Collaboration |
|
|
|
AI in Policy Planning and Decision-Making: From Predictive Analytics to Ethical Governance
AI in Policy Planning and Decision-Making: From Predictive Analytics to Ethical Governance
Imagine governments with the ability to anticipate future outcomes before enacting policies. This capability is fast becoming reality. AI-powered tools are transforming policy planning by enabling data-driven forecasting, detailed scenario simulations, and sophisticated impact assessments. However, alongside these technological advances, ethical and governance challenges must be addressed—especially when decisions influence millions of lives.
Leveraging Machine Learning and Natural Language Processing for Evidence-Based Policymaking
Machine learning (ML) models have become essential for interpreting complex trends and forecasting policy outcomes. Governments now deploy these models to analyze vast datasets—from economic indicators to public health statistics—detecting emerging patterns that inform timely, evidence-based decisions.
A notable example is the COVID-19 pandemic, where adaptive forecasting techniques helped policymakers anticipate infection surges and allocate healthcare resources effectively. This precision-driven approach improved patient outcomes and optimized costs. Today, similar ML-driven forecasting is expanding into areas such as budget optimization and disaster response.
Complementing ML, Natural Language Processing (NLP) unlocks insights from unstructured text data. Policy documents, legislative texts, and regulatory filings can be analyzed at scale to identify key themes, inconsistencies, or compliance risks. For instance, FEMA leverages large language models (LLMs) to process extensive policy materials, generate concise summaries, and simulate policy impacts across diverse scenarios.
NLP capabilities have matured beyond keyword extraction, now supporting multilingual analysis and integrating multimodal data like images and audio. This evolution enhances their applicability across varied government functions.
Moreover, no-code machine learning platforms are democratizing AI access. These tools empower policymakers without programming expertise to engage directly with data analytics, fostering more agile and responsive governance.
The Promise and Challenges of AI-Driven Impact Assessment
AI extends beyond prediction to evaluating the broader effects of policy decisions. By simulating alternative scenarios, AI tools forecast economic, social, and environmental outcomes prior to policy implementation. This strategic foresight—often termed “wind tunnel testing”—helps identify unintended consequences and optimize interventions.
For example, Rapid Innovation’s AI policy analysis platforms enable near real-time measurement of initiative effectiveness, bolstering transparency and accountability. Adaptive forecasting software flags emerging risks such as budget shortfalls or compliance gaps, providing decision-makers with early warnings to adjust strategies proactively.
However, AI-driven impact assessments are only as reliable as their input data. Biased, incomplete, or outdated datasets can mislead forecasts rather than clarify them. Additionally, the opacity of some AI models, especially deep learning systems, complicates policymakers’ ability to interpret or trust outputs fully.
Navigating Ethical Challenges: Bias, Transparency, and Accountability
Algorithmic bias remains one of the most pressing challenges in AI-enabled governance. AI systems trained on historical data risk perpetuating existing social inequities, resulting in discriminatory outcomes. Automated decision-making processes may disproportionately impact marginalized communities, raising critical fairness and justice concerns.
Transparency is equally vital but difficult to achieve. Many AI models function as “black boxes,” with decision-making logic that is hard to interpret. Without clear explanations, affected stakeholders cannot meaningfully scrutinize or challenge AI-driven decisions, undermining public trust.
Accountability frameworks are still evolving. In the United States, the policy landscape is patchy, lacking comprehensive federal AI legislation. Instead, a mosaic of state laws, such as California’s AI Transparency Act and Texas’s regulations on “high-risk AI systems,” impose requirements for AI disclosure and proactive bias audits.
Internationally, the EU AI Act exemplifies emerging governance models with risk-based classifications guiding AI deployment according to potential harm. Enforcement challenges persist, underscoring the need for adaptive governance balancing innovation with risk mitigation.
Toward Responsible AI Governance in Public Policy
Given AI’s complexity and societal impact, governance frameworks for public policy must embody several core principles:
- Dynamic and Collaborative: Foster public-private partnerships and mandate independent third-party audits to ensure compliance and stimulate innovation.
- Human-Centered: Incorporate human-in-the-loop or human-on-the-loop models to preserve essential human judgment in critical decisions.
- Privacy-Conscious: Protect sensitive data rigorously while enabling meaningful analysis to inform policymaking.
- Transparent and Explainable: Prioritize AI interpretability to build public trust and facilitate regulatory oversight.
Thought leaders advocate for layered governance approaches, where regulations adapt alongside AI’s evolving capabilities rather than imposing rigid, one-size-fits-all mandates. This model acknowledges AI’s unique risks and opportunities, aiming to safeguard democratic values without hindering technological progress.
Conclusion: Balancing Technological Promise with Ethical Prudence
AI is revolutionizing policy planning, shifting governments from reactive to proactive decision-making. Yet, the promise of AI must be tempered by awareness of its limitations and ethical pitfalls.
Effective AI integration requires an unwavering commitment to transparency, accountability, and inclusivity. Policymakers and technologists alike face a clear mandate: harness AI’s predictive and analytical power to improve governance while embedding robust ethical safeguards.
Only by striking this balance can AI fulfill its potential to serve the public good—enabling policies that are not just smart, but just, trustworthy, and equitable.
Aspect | Description | Examples / Notes |
---|---|---|
AI Techniques | Machine Learning (ML), Natural Language Processing (NLP), No-code ML platforms | ML for forecasting policy outcomes; NLP for analyzing policy documents; No-code platforms empower non-programmers |
Applications in Policy Planning | Data-driven forecasting, scenario simulations, impact assessments | COVID-19 infection surge predictions; budget optimization; disaster response |
AI-Driven Impact Assessment | Simulation of economic, social, environmental outcomes; near real-time initiative effectiveness measurement | Rapid Innovation platforms; adaptive forecasting for risk detection |
Challenges | Data bias, model opacity, reliability of input data | Biased datasets causing unfair outcomes; deep learning “black box” issues |
Ethical Concerns | Algorithmic bias, transparency, accountability | Discriminatory outcomes; lack of clear explanations; evolving legal frameworks (e.g., CA AI Transparency Act, EU AI Act) |
Governance Principles | Dynamic collaboration, human-centered, privacy-conscious, transparent & explainable AI | Public-private partnerships, human-in-the-loop, data protection, interpretability |
Regulatory Examples | US state laws, EU AI Act | California AI Transparency Act; Texas high-risk AI regulations; EU risk-based AI classifications |
Goals | Balanced AI adoption with ethical safeguards | Transparency, accountability, inclusivity; trustworthy and equitable policies |
Benchmarking AI Adoption: Comparative Analysis of Global Government Initiatives
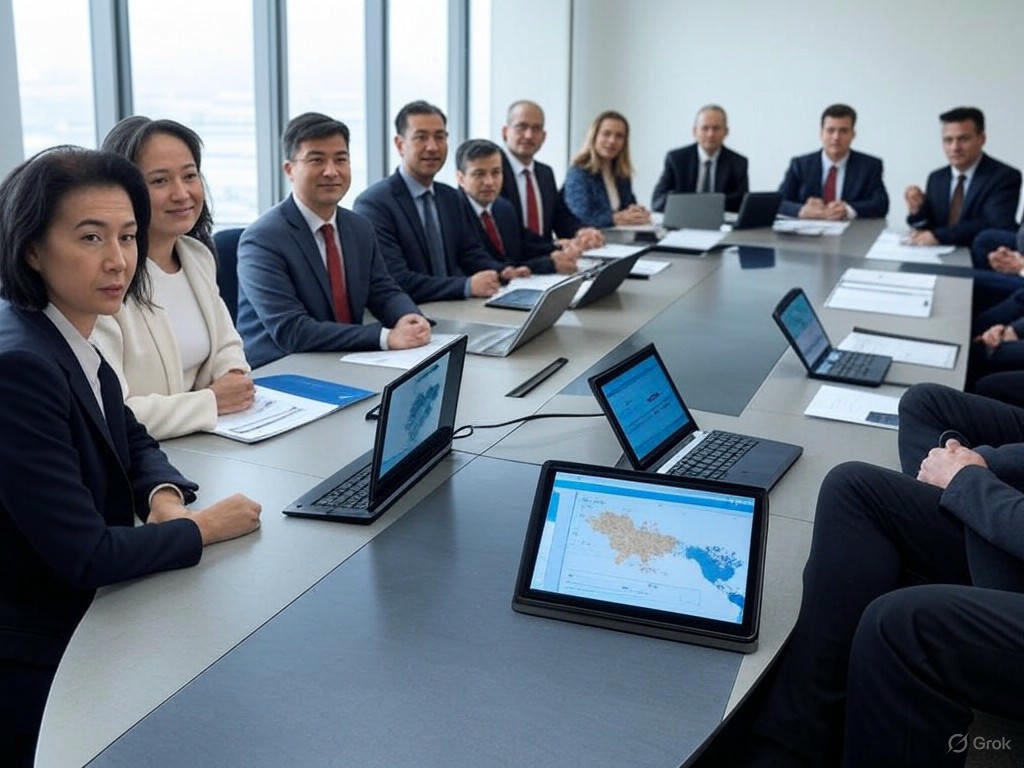
Benchmarking AI Adoption: Comparative Analysis of Global Government Initiatives
How do governments around the world compare in their AI ambitions and implementations? When integrating artificial intelligence into smart cities, public services, and policy planning, approaches vary widely, shaped by distinct political, cultural, and economic contexts. This section analyzes the AI strategies, investments, regulatory frameworks, and governance models of five key players—the United States, China, Japan, Brazil, and Europe—to uncover the drivers behind their successes and challenges.
Contrasting AI Strategies and Implementations
United States:
The U.S. government pursues a decentralized AI strategy anchored by strong public-private partnerships. Recent Executive Orders under President Biden emphasize responsible AI adoption across federal agencies, promoting innovation aligned with public interest. The Department of State’s “Enterprise Artificial Intelligence Strategy FY 2024-2025” exemplifies this by integrating AI tools like Northstar for social media analytics to enhance diplomatic policy planning.
Education and workforce development are central pillars. Initiatives such as the White House Task Force on AI Education foster talent through collaborations with tech leaders including Microsoft, OpenAI, and Google. This ecosystem encourages agile innovation while embedding responsible AI principles. The U.S. also supports AI adoption via sector-specific regulations, favoring flexible oversight that encourages experimentation and rapid deployment.
China:
China takes a highly centralized, state-driven approach aimed at achieving global AI leadership by 2030. With over 1 trillion yuan in strategic funding, China’s AI ecosystem tightly integrates industrial, military, and technological ambitions. The New Generation Artificial Intelligence Development Plan (AIDP) and “Made in China 2025” initiatives guide this expansive effort.
China leads in generative AI patents, filing more than 38,000 since 2014, and fosters innovation through institutions like Alibaba’s DAMO Academy. However, this rapid scale-up is accompanied by stringent content controls and surveillance applications, reflecting a governance model that prioritizes state control alongside technological advancement.
Japan:
Japan’s AI strategy focuses on robotics, manufacturing, and integrating AI with the Internet of Things (IoT) to advance industrial automation. While Japan’s patent volume is modest compared to the U.S. and China, it leverages strengths in precision engineering and smart infrastructure.
Government efforts promote R&D partnerships between industry and academia, applying AI to enhance public services. Japan’s regulatory approach is cautious and safety-oriented, emphasizing ethical AI use and incremental innovation, consistent with societal preferences for risk mitigation.
Brazil:
Brazil’s AI ambitions are emerging, anchored by the Brazilian Artificial Intelligence Plan (PBIA) 2024-2028, which commits approximately $4 billion over four years. The plan prioritizes healthcare and urban development to position Brazil as a regional AI leader.
Brazil combines government investment with public-private partnerships to build capacity. However, infrastructure limitations and talent shortages remain significant hurdles. Its regulatory framework is evolving, with a particular focus on balancing innovation incentives and data privacy protections.
Europe:
Europe distinguishes itself through a comprehensive regulatory and ethical AI framework. The AI Act, the world’s first horizontal AI legal framework, enforces risk-based rules ranging from “unacceptable” to “minimal risk” AI systems. This regulatory rigor aims to foster trustworthy AI and establish a global standard akin to the GDPR’s impact on data privacy.
The EU’s “AI Continent Action Plan” dedicates €200 billion to AI development, emphasizing digital skills academies and supercomputing infrastructure. European initiatives prioritize human-centric AI, transparency, and societal trust, deliberately tempering innovation speed with robust risk management to ensure broad acceptance.
Variances in Governance, Investment, and Partnerships
-
Regulatory Approaches:
- The EU leads with a proactive, structured regulatory framework via the AI Act, emphasizing risk categorization and human oversight.
- China’s agile regulations adapt swiftly to emerging AI domains but incorporate strict state controls on content and data.
- The U.S. adopts a sector-specific, less centralized regulatory stance that fosters innovation-friendly environments.
- Brazil and Japan’s regulatory ecosystems are still maturing, with Brazil prioritizing privacy and data protection, while Japan emphasizes safety and ethical standards.
-
Investment Levels:
- China’s trillion-yuan investment dwarfs other nations, underscoring AI’s status as a national priority.
- Europe’s €200 billion commitment is substantial but distributed across multiple programs and years, balancing funding with regulation.
- The U.S. focuses heavily on research infrastructure and workforce development, leveraging industry partnerships for broader AI adoption.
- Brazil’s $4 billion investment signals growing commitment despite structural gaps in talent and infrastructure.
- Japan’s investments are more targeted toward industrial and robotics applications.
-
Public-Private Partnerships (PPPs):
- The U.S. exemplifies effective PPPs, with federal agencies collaborating closely with tech giants to co-develop AI tools and education programs.
- China also leverages PPPs but under stringent state oversight to align innovations with national objectives.
- The World Economic Forum highlights PPPs as essential globally for ethical and inclusive AI, a principle variably reflected across these countries.
- Brazil and Japan are developing PPPs to bridge expertise gaps and accelerate applied AI research.
Implications for Innovation, Societal Acceptance, and Risk Management
The varying approaches yield distinct outcomes:
-
Innovation Pace:
China’s centralized funding and talent mobilization enable rapid AI deployment, especially in surveillance and industrial automation. However, regulatory unpredictability and strict controls may deter some private innovation. The U.S. leads in compute capacity and AI talent deployment, sustaining innovation in foundational models and scalable AI integration. Europe’s cautious, regulation-first stance slows immediate innovation but fosters sustainable, trustworthy AI likely to gain societal acceptance. -
Societal Acceptance:
Public trust is critical. Europe’s emphasis on transparency and ethics resonates with societal demands for accountability. In the U.S., surveys reveal mixed perceptions, with nearly half expressing concern that AI risks outweigh benefits. China’s model prioritizes information control, which complicates genuine societal acceptance but facilitates government-led implementations. Brazil and Japan’s cautious regulatory stances mirror evolving public dialogues on privacy and safety. -
Risk Management:
The EU’s tiered, risk-based regulation exemplifies best practices in balancing innovation with protection, potentially serving as a global benchmark. The U.S. relies more on industry standards and sectoral oversight, offering agility but risking regulatory gaps. China’s model integrates aggressive AI use with pervasive surveillance, raising ethical concerns but ensuring compliance. Brazil and Japan face challenges in developing governance frameworks that keep pace with AI’s rapid evolution.
Lessons Learned and Best Practices for Responsible Scaling
-
Embed Governance Early:
Europe’s AI Act illustrates the value of establishing clear, enforceable rules early to build trust and manage risk effectively. -
Harness Public-Private Synergies:
The U.S. experience shows how dynamic collaboration between government and industry accelerates innovation and workforce readiness. -
Align Innovation with Societal Values:
Japan’s incremental, safety-first approach and Brazil’s focus on healthcare and urban AI applications demonstrate the importance of tailoring AI development to local priorities. -
Invest in Talent and Infrastructure:
China’s scale of investment underscores that robust compute resources and skilled human capital are prerequisites for AI leadership. -
Promote Transparency and Inclusive Dialogue:
Across all regions, rising public concerns about AI risks highlight the necessity for transparent communication and collaborative governance frameworks.
In conclusion, no universal AI strategy fits all governments. The interplay of regulation, investment, partnerships, and societal context shapes how AI can be responsibly scaled to enhance public services and urban life. Governments must navigate complex trade-offs—between speed and caution, innovation and ethics, control and openness—to chart sustainable AI futures. Continuous benchmarking and shared learning will be essential to harness AI’s transformative potential while safeguarding democratic values and human rights.
Aspect | United States | China | Japan | Brazil | Europe |
---|---|---|---|---|---|
AI Strategy | Decentralized; public-private partnerships; responsible AI; innovation aligned with public interest | Centralized, state-driven; goal for global leadership by 2030; integration of industrial, military, tech ambitions | Focus on robotics, manufacturing, IoT; precision engineering; cautious and safety-oriented | Emerging AI; Brazilian AI Plan 2024-2028; focus on healthcare and urban development | Comprehensive regulatory and ethical framework; human-centric AI; transparency; societal trust |
Investment | Focus on research infrastructure and workforce development; leveraging industry partnerships | Over 1 trillion yuan strategic funding; largest investment globally | Targeted investments in industrial and robotics applications | Approximately $4 billion over four years | €200 billion dedicated to AI development and infrastructure |
Regulatory Approach | Sector-specific; flexible oversight fostering innovation | Agile regulations with strict state control on content and data | Cautious; safety and ethical standards emphasized | Evolving; focus on innovation incentives and data privacy | AI Act with risk-based rules; proactive and structured |
Public-Private Partnerships (PPPs) | Strong; federal agencies collaborate with tech giants (Microsoft, OpenAI, Google) | Leveraged under stringent state oversight | Developing to bridge expertise gaps and accelerate research | Developing to accelerate applied AI research | Encouraged as essential for ethical and inclusive AI |
Innovation Pace | High; leads in compute capacity and AI talent; supports rapid innovation | Rapid AI deployment enabled by centralized funding and talent mobilization | Incremental innovation; cautious and safety-first | Growing, but constrained by infrastructure and talent shortages | Cautious; slower innovation but fosters sustainable and trustworthy AI |
Societal Acceptance | Mixed perceptions; concerns about AI risks | Information control complicates acceptance but facilitates implementation | Cautious; aligned with privacy and safety concerns | Developing; evolving public dialogues on privacy and safety | High emphasis on transparency and ethics; strong public demand for accountability |
Risk Management | Industry standards and sectoral oversight; agile but with potential gaps | Integrated with surveillance; raises ethical concerns but ensures compliance | Ethical AI use emphasized; cautious incremental approach | Challenges in evolving governance frameworks | Tiered, risk-based regulation; seen as global benchmark |
Key Initiatives | Executive Orders; Enterprise AI Strategy FY 2024-2025; White House Task Force on AI Education | New Generation AI Development Plan; Made in China 2025; Alibaba DAMO Academy | R&D partnerships between industry and academia; smart infrastructure projects | Brazilian Artificial Intelligence Plan (PBIA) 2024-2028 | AI Act; AI Continent Action Plan; digital skills academies; supercomputing infrastructure |
Future Directions and Challenges: Navigating the Road Ahead for AI in Government
Future Directions and Challenges: Navigating the Road Ahead for AI in Government
What lies beyond the horizon for AI in government? As we approach 2025, rapid advances in technology and evolving governance frameworks are poised to fundamentally reshape how public institutions operate and engage with citizens.
Emerging Trends: Generative AI, Hybrid Cloud, and Citizen Interaction
Generative AI has moved from a futuristic concept to a practical, increasingly adopted tool across government operations. According to Google Cloud’s 2025 State of AI Infrastructure Report, 98% of organizations are exploring generative AI, with 39% already deploying it in production. For governments, this translates into new opportunities—not only automating routine coding tasks but also transforming citizen engagement through AI agents that offer real-time assistance and personalized services.
AI-powered chatbots are a prime example of this transformation. Rapid Innovation highlights that governments are leveraging these AI interfaces to improve accessibility and responsiveness, handling everything from permit applications to emergency alerts. These systems streamline interactions, allowing human staff to focus on more complex, high-impact cases.
Underpinning this AI surge is the broader adoption of hybrid AI-cloud infrastructures. Hybrid cloud environments combine public and private cloud resources, offering scalability and flexibility beyond traditional on-premises systems. However, this also introduces increased complexity and an expanded attack surface. Security teams must implement robust, layered defenses and utilize native security tools from providers like Microsoft and AWS to protect sensitive government data. TierPoint emphasizes the critical role of seamless connectivity and centralized resource management in ensuring disaster recovery and operational continuity within hybrid clouds.
Moreover, hybrid architectures facilitate edge computing, which processes data closer to its source. This is crucial for real-time decision-making in smart city applications such as traffic management and public safety, enabling faster, localized responses without depending solely on cloud connectivity.
Unresolved Challenges: Privacy, Ethics, Workforce, and Trust
Despite technological progress, several core challenges remain unresolved. Data privacy stands at the forefront, given governments’ access to vast amounts of sensitive personal information. The State of Cloud in Government report identifies data quality and security as major obstacles to AI adoption.
Ethical considerations add further complexity. UNESCO’s global standard on AI ethics underscores the imperative to protect human rights and dignity. Governments must ensure AI systems are transparent, accountable, and free from biases that could perpetuate social inequities. Achieving fairness demands ongoing vigilance and strong data governance to prevent historical biases from seeping into training datasets.
Workforce transformation presents both opportunities and challenges. McKinsey’s 2025 research reveals that employees are generally more ready to embrace AI than many leaders expect. Nevertheless, successful integration requires new skill sets and transparent communication about AI’s role in the workplace. Governments should invest in comprehensive upskilling programs and cultivate organizational cultures that view AI ethics not merely as compliance but as a means to bolster trust and collaboration.
Public trust itself remains a fragile and essential element. As Paul Tierney of Dataminr points out, scaling AI responsibly in government depends on inclusivity and fairness. Without citizen confidence, even the most advanced AI initiatives risk backlash or underutilization. Building trust requires clear, honest communication about AI’s benefits and limitations, alongside robust human oversight models—whether human-in-the-loop or human-on-the-loop—to maintain accountability and safeguard public interests.
Strategic Recommendations: Balancing Innovation with Responsible Stewardship
How can governments harness AI’s potential while addressing these critical responsibilities? The following strategic recommendations offer a balanced path forward:
-
Adopt a layered AI governance framework: There is no universal solution. Agencies must develop tailored policies addressing privacy, ethics, transparency, and security. Splunk’s 2025 AI Governance guide recommends adaptable human oversight models ranging from direct intervention to supervisory roles, calibrated to the risk level of each AI application.
-
Invest in continuous evaluation: AI systems evolve rapidly, necessitating dynamic governance. Agencies should embed real-time monitoring tools to observe AI behavior, assess performance, and detect anomalies or biases promptly.
-
Engage inclusive stakeholder ecosystems: Broad public input, interdisciplinary expertise, and private sector collaboration are vital. The Partnership on AI emphasizes building vibrant assurance and accountability ecosystems to align AI deployment with societal values.
-
Prioritize workforce development: Upskilling and ethical AI education must be central components of transformation strategies. Organizations like OneAdvanced demonstrate the effectiveness of internal AI steering committees and comprehensive training programs in embedding responsibility throughout government operations.
-
Leverage hybrid cloud and managed services: Transitioning legacy systems to hybrid cloud architectures, supported by managed services, enhances agility and security. This shift enables government IT teams to focus more on innovation rather than routine maintenance.
-
Build transparent citizen interfaces: AI-powered tools should clearly communicate their capabilities and limitations to users. Governments must prioritize user-centric design and data privacy in all deployments to foster public trust and usability.
The Road Ahead
AI integration in government is no longer a question of if, but how—and how wisely it is implemented. The rapid pace of AI development, coupled with profound societal implications, demands an agile, evidence-based approach that embraces innovation while protecting public interests.
Going forward, governments must resist the allure of hype and instead focus on pragmatic, accountable deployment strategies. Building trust through transparency, investing in human capital, and fostering multi-stakeholder collaboration will be decisive factors in realizing AI’s promise to transform public services into more efficient, equitable, and responsive systems.
Uncertainty will persist on this journey. Yet, with thoughtful governance and steadfast commitment to ethical principles, AI can become a powerful tool for the public good rather than a source of risk or division.
Category | Details |
---|---|
Emerging Trends |
|
Unresolved Challenges |
|
Strategic Recommendations |
|