AI in Agriculture: Smart Farming for Sustainable Crop Yields
- Introduction: Why AI is a Game-Changer for Modern Agriculture
- Introduction: Why AI is a Game-Changer for Modern Agriculture
- The Global Food Demand and Resource Constraints
- AI as a Transformative Tool for Smart Farming and Crop Yield Optimization
- The Dual Challenge: Increasing Productivity While Ensuring Sustainability
- Setting Expectations for an Evidence-Based Exploration
- Foundations of AI in Agriculture: Technologies and Core Principles
- Foundations of AI in Agriculture: Technologies and Core Principles
- Core AI Technologies Powering Smart Farming
- Primary Data Sources: Satellite Imagery, Drone Sensing, and Ground Sensors
- Foundational AI Models and Data Infrastructure Challenges
- Bridging Technology and Sustainability
- Precision Agriculture and Smart Farming: Technical Specifications and Operational Workflows
- Precision Agriculture and Smart Farming: Technical Specifications and Operational Workflows
- AI-Driven Techniques: From Soil Analysis to Pest Detection
- Operational Workflows: From Sensor Data to Automated Machinery
- Performance Metrics and Case Studies: Demonstrating Real-World Impact
- Comparative Analysis: AI-Powered Solutions Versus Traditional Farming Practices
- Comparative Analysis: AI-Powered Solutions Versus Traditional Farming Practices
- Yield and Resource Utilization: Quantifying the Gains
- Labor Requirements: Transitioning from Manual Effort to Skilled Oversight
- Environmental Impact: Progressing Toward Sustainable Farming Practices
- Infrastructure Gaps, Training Needs, and Data Security Risks
- Socioeconomic Implications: Navigating Labor Displacement and Skill Shifts
- Final Thoughts: Evidence Over Hype, Charting a Balanced Path Forward
- Real-World Applications and Case Studies: From Automated Robots to Mixed Reality
- Real-World Applications and Case Studies: From Automated Robots to Mixed Reality
- AI-Powered Agricultural Robots: Precision Weeding and Automated Harvesting
- AI-Driven Disease Prediction Models and Smart Irrigation Systems: Data-Driven Crop Health
- Emerging Mixed Reality Interfaces for Crop Monitoring: Augmenting Farmer Insight
- Assessing Effectiveness, Scalability, and Farmer Acceptance
- Future Trends and Innovations in AI-Driven Agriculture
- Future Trends and Innovations in AI-Driven Agriculture
- Enhanced IoT Connectivity: Building Responsive Farm Ecosystems
- Hyperspectral Imaging: Seeing Beyond the Visible Spectrum
- AI-Powered Sustainable Farming: Climate Resilience and Resource Conservation
- Navigating Regulatory, Ethical, and Security Challenges
- The Evolving Role of the Farmer in a Data-Driven Ecosystem
- Looking Ahead: An Agricultural Revolution in the Making
- Conclusion: Synthesizing AI’s Potential and Challenges for Sustainable Food Production
- Conclusion: Synthesizing AI’s Potential and Challenges for Sustainable Food Production
- The Transformative Power of AI in Agriculture
- Balancing Technology with Human Expertise
- Persistent Challenges and the Need for Collaborative Solutions
- Charting a Responsible Path Forward

Introduction: Why AI is a Game-Changer for Modern Agriculture
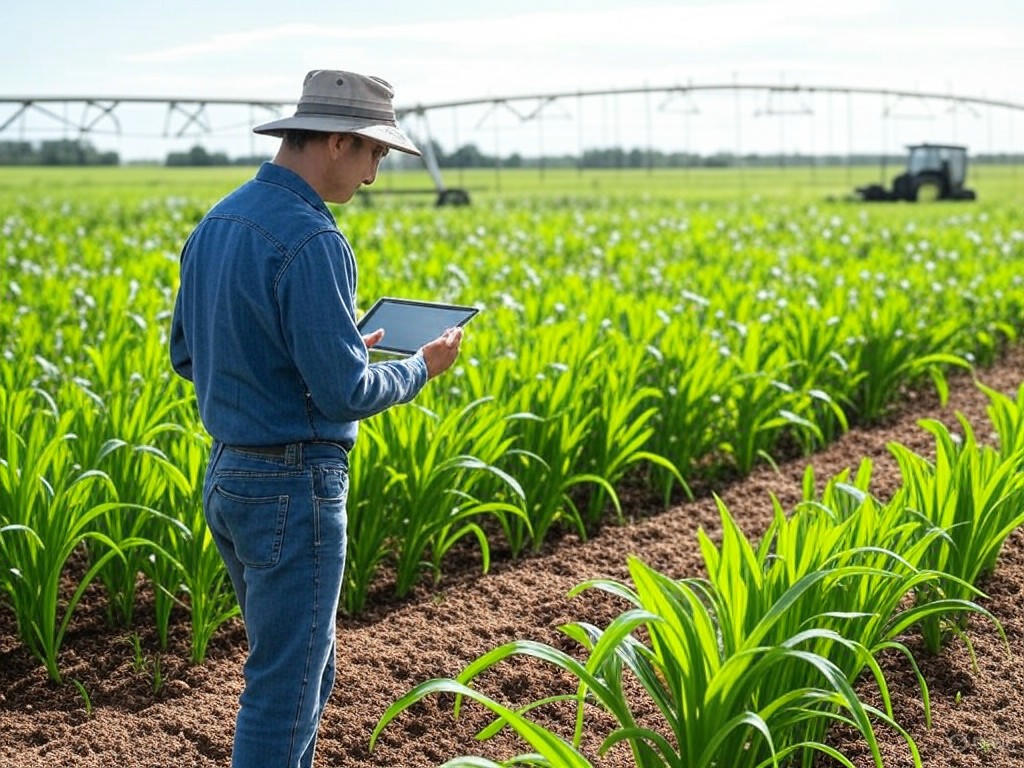
Introduction: Why AI is a Game-Changer for Modern Agriculture
How can we sustainably feed a global population projected to grow by nearly 2 billion by 2050 amid shrinking arable land, water scarcity, and increasing climate volatility? This pressing challenge sits at the nexus of food security, environmental sustainability, and technological innovation.
The Global Food Demand and Resource Constraints
Agriculture faces mounting pressure. The Food and Agriculture Organization (FAO) projects a 56% increase in global food demand by mid-century, fueled by population growth and shifting dietary patterns. Yet, resource limitations are tightening. Agriculture contributes 10 to 15 percent of global greenhouse gas emissions, while irrigation accounts for roughly 70% of freshwater use, increasingly unsustainable in many regions.
Recent market volatility underscores these vulnerabilities: maize prices have risen 9%, and rice prices surged 26%, reflecting fragile supply chains impacted by geopolitical tensions and climate events.
Food insecurity remains entrenched in vulnerable areas. Initiatives such as the Sahel Irrigation Initiative and the CGIAR Accelerating Impact project exemplify focused efforts to bolster resilience in African farming communities, emphasizing smarter resource management and enhanced productivity.
AI as a Transformative Tool for Smart Farming and Crop Yield Optimization
Artificial intelligence (AI) is no longer a futuristic concept but an integral part of modern agriculture. It is revolutionizing farming through precision agriculture, IoT sensor networks, and satellite imagery analytics, enabling smarter, data-driven decisions.
Key AI-powered capabilities include:
- Early disease detection: Using spectral analysis from drones and satellites to identify crop diseases before symptoms become visible, allowing timely interventions.
- Automated machinery: Precision spraying, fertilization, and harvesting performed by AI-guided equipment reduce waste and improve efficiency.
- Real-time monitoring: Continuous assessment of soil health, crop conditions, and weather patterns optimizes irrigation and resource use.
For example, Farmonaut’s AI-driven satellite platform achieves up to 95% accuracy in crop yield prediction, shifting decision-making from intuition to science. These technologies also mitigate labor shortages and rising input costs, enhancing farm resilience.
The Dual Challenge: Increasing Productivity While Ensuring Sustainability
AI holds immense promise, but it must align with sustainable and ethical farming practices. Boosting yields cannot come at the cost of soil degradation, biodiversity loss, or social inequities.
Regenerative agriculture, supported by AI and digital tools, offers a comprehensive framework emphasizing environmental stewardship, social equity, and economic viability. AI applications can optimize fertilizer and pesticide use to minimize environmental impact, while precision livestock farming enhances animal welfare and reduces emissions.
Yet, adoption challenges persist. High upfront costs, data limitations, and the need for farmer training remain significant hurdles. Successful integration depends on balancing advanced technology with on-the-ground expertise, ensuring farmers remain at the center of decision-making.
Setting Expectations for an Evidence-Based Exploration
This article will provide a nuanced, evidence-based examination of AI’s capabilities and constraints in agriculture. We will explore how AI-driven smart farming reshapes productivity, resource management, and sustainability, supported by real-world examples and data.
Ethical considerations and social implications will also be addressed. This is not a narrative of technology supplanting farmers but one of empowerment—enabling them to meet humanity’s urgent challenge of feeding a growing population sustainably, despite environmental constraints. The path forward is complex, but AI’s potential to spark a new green revolution is undeniable.
Aspect | Details |
---|---|
Global Food Demand Increase | 56% rise by 2050 (FAO projection) |
Population Growth | Nearly 2 billion increase by 2050 |
Greenhouse Gas Emissions from Agriculture | 10 to 15 percent of global emissions |
Freshwater Use in Irrigation | Approximately 70% of global freshwater use |
Recent Crop Price Changes | Maize +9%, Rice +26% |
AI Capabilities in Agriculture | Early disease detection, Automated machinery, Real-time monitoring |
AI Crop Yield Prediction Accuracy | Up to 95% (Farmonaut satellite platform) |
Challenges for AI Adoption | High upfront costs, Data limitations, Need for farmer training |
Foundations of AI in Agriculture: Technologies and Core Principles
Foundations of AI in Agriculture: Technologies and Core Principles
What drives the AI revolution reshaping modern agriculture? It is the convergence of advanced technologies—machine learning, computer vision, Internet of Things (IoT) devices, and robotics—that collectively transform farms into sophisticated, data-driven ecosystems. This transformation is far from speculative: the global AgTech market is projected to reach $74 billion by 2034, growing annually by over 12%. To understand how these technologies integrate, it’s essential to explore their distinct roles and the diverse data streams that fuel them.
Core AI Technologies Powering Smart Farming
Machine learning forms the backbone of AI’s impact on agriculture. It enables systems to discern intricate patterns in vast and varied datasets—ranging from soil moisture fluctuations to pest infestation signals—and to forecast outcomes such as crop yields or disease outbreaks. For example, machine learning models that combine environmental and genetic data can predict crop performance with remarkable accuracy. Igor Fernandes’ model, which nearly secured victory in the international Genome to Fields competition, exemplifies this capability.
Computer vision acts as the farmer’s digital eye, analyzing images captured by drones or stationary cameras. AI-powered vision systems can automate fruit counting, detect early signs of plant disease, and monitor livestock welfare without physical contact. This non-invasive approach scales monitoring efforts with precision unattainable by manual inspection. Companies like Averroes.ai deploy integrated machine vision and IoT solutions to automate crop monitoring and generate real-time, actionable insights.
IoT integration connects a network of sensors, drones, tractors, and weather stations, enabling continuous data streaming on environmental conditions such as temperature, humidity, and soil health. This seamless connectivity facilitates precise control over farming inputs. Optical spectroscopy sensors, for instance, provide real-time information on soil and crop conditions, allowing targeted irrigation and nutrient application. The expansion of wireless, battery-operated IoT sensors has made such connectivity feasible even in remote agricultural areas.
Robotics completes the technological ecosystem by translating AI insights into physical actions. Autonomous tractors, robotic harvesters, and AI-equipped drones are progressively automating labor-intensive tasks with high accuracy and efficiency. Colin Hurd, CEO of Mach, anticipates that within five years, advanced technologies will be embedded directly into farming machinery, moving agriculture closer to automated factory-like operations.
Primary Data Sources: Satellite Imagery, Drone Sensing, and Ground Sensors
If AI is the brain of smart farming, data serves as its lifeblood. Key data sources include satellite imagery, drone sensing, and ground-level sensors—each providing unique perspectives and resolutions.
-
Satellite imagery has become indispensable for large-scale precision agriculture. Providers like Planet Labs deliver daily, high-resolution images (down to 3 meters) capturing multiple spectral bands that assess vegetation health through indices such as the Normalized Difference Vegetation Index (NDVI). These insights enable early detection of crop stress before symptoms become visible, facilitating timely interventions. Syngenta’s collaboration with Planet demonstrates how satellite data is integral to advanced crop management platforms.
-
Drones complement satellites by offering flexible, higher-resolution monitoring. Equipped with multispectral cameras, LiDAR, and radar sensors, drones can fly at varied altitudes to evaluate soil conditions, crop stress, and pest presence. They are particularly effective for targeted inspections and validating satellite-derived data. The agricultural drone market is expected to reach $3.7 billion by 2027, with specialized models like DJI Matrice 350 RTK and Parrot Bluegrass Fields tailored for industrial and cost-sensitive farming applications.
-
Ground sensors provide granular, localized data essential for precision interventions. Soil moisture sensors, for example, measure volumetric water content to optimize irrigation scheduling, conserving water and preventing soil salinization. This market is projected to surpass $674 million by 2033. Advances in wireless, AI-enabled soil sensors support real-time data collection and integration with broader IoT networks, enhancing on-the-ground decision-making.
Foundational AI Models and Data Infrastructure Challenges
Behind the scenes, a suite of AI models and algorithms enables pattern recognition, prediction, and decision support tailored to agriculture’s complexities.
Crop yield prediction models integrate critical environmental variables—especially temperature—alongside genetic and soil data to forecast harvest outcomes with growing precision. The market for machine learning applications in crop yield prediction alone is projected to grow from $0.79 billion in 2024 to over $1 billion in 2025, reflecting rapid adoption.
Agriculture-specific challenges have inspired competitions such as the Agriculture-Vision Prize Challenge, which encourages development of innovative algorithms capable of interpreting aerial imagery to classify crop types, detect anomalies, and monitor growth stages. Deep learning models trained on multispectral data allow automated fruit counting and disease detection with impressive accuracy. However, these models require large, well-labeled datasets, which are often fragmented across institutions.
This fragmentation underscores a critical bottleneck: agricultural data infrastructure must evolve to support integration, storage, and real-time accessibility of heterogeneous data streams—from satellite and drone imagery to sensor telemetry. Edge data centers and cloud platforms provide scalable, low-latency solutions adapted to agricultural contexts. Nonetheless, rural connectivity challenges persist, necessitating hybrid architectures capable of offline operation with periodic synchronization.
Human expertise remains vital. There is a shortage of agronomists and farmers trained to interpret AI outputs and incorporate them into practical decision-making. Without accessible user interfaces and adequate training, the transformative potential of AI may not be fully realized on farms.
Bridging Technology and Sustainability
AI’s promise extends beyond productivity gains to fostering sustainable agriculture. Precision farming techniques enabled by AI can reduce water use by up to 30% and increase crop yields by approximately 20%, according to recent forecasts. By optimizing input application, minimizing chemical runoff, and lowering greenhouse gas emissions, AI tools help mitigate agriculture’s environmental footprint.
However, adoption varies widely, especially among smallholder and resource-poor farmers facing affordability and knowledge barriers. Building an inclusive ecosystem—combining robust data platforms, accessible technologies, and supportive policies—is essential to ensure AI-driven agriculture benefits all stakeholders and contributes meaningfully to global food security.
In summary, the foundation of AI in agriculture rests on the sophisticated interplay of machine learning, computer vision, IoT sensors, robotics, and diverse data sources, all supported by evolving data infrastructures. While the promise is significant, realizing it demands careful attention to technical integration, data management, and human capacity building. This evolving frontier is set to redefine not only farming practices but also our broader relationship with food, resources, and sustainability in a changing world.
Category | Technology/Data Source | Description | Examples/Market Data |
---|---|---|---|
Core AI Technologies | Machine Learning | Enables pattern recognition in diverse datasets to forecast outcomes like crop yields and disease outbreaks. | Igor Fernandes’ model in Genome to Fields competition; market growing rapidly. |
Computer Vision | Analyzes images from drones/cameras for tasks like fruit counting, disease detection, and livestock monitoring. | Averroes.ai integrates machine vision with IoT for real-time crop monitoring. | |
IoT Integration | Connects sensors, drones, tractors, weather stations for continuous environmental data streaming. | Optical spectroscopy sensors enable targeted irrigation; wireless sensors feasible in remote areas. | |
Robotics | Automates labor-intensive tasks using autonomous tractors, harvesters, AI drones. | Mach CEO predicts embedded tech in machinery within 5 years. | |
Primary Data Sources | Satellite Imagery | Provides high-res, multi-spectral images for large-scale precision agriculture and vegetation health monitoring. | Planet Labs offers daily images; Syngenta uses satellite data for crop management. |
Drones | Offer flexible, high-resolution monitoring with multispectral cameras, LiDAR, radar for soil, crop, pest evaluation. | Market projected at $3.7B by 2027; DJI Matrice 350 RTK, Parrot Bluegrass Fields models. | |
Ground Sensors | Provide localized data such as soil moisture to optimize irrigation and prevent salinization. | Market expected to exceed $674M by 2033; wireless AI-enabled sensors enhance data collection. | |
AI Models & Infrastructure | Crop Yield Prediction Models | Integrate environmental, genetic, soil data to forecast harvest outcomes. | Market growing from $0.79B (2024) to over $1B (2025). |
Data Infrastructure | Supports integration, storage, and real-time access of heterogeneous data with cloud and edge solutions. | Challenges include fragmented datasets and rural connectivity; hybrid architectures needed. | |
Sustainability & Adoption | Precision Farming | Reduces water use by up to 30% and increases yields by ~20% through optimized input application. | Helps mitigate environmental footprint including chemical runoff and emissions. |
Inclusive Ecosystem | Addresses affordability and knowledge barriers among smallholder farmers. | Requires robust data platforms, accessible tech, and supportive policies for broad AI adoption. |
Precision Agriculture and Smart Farming: Technical Specifications and Operational Workflows
Precision Agriculture and Smart Farming: Technical Specifications and Operational Workflows
Imagine treating every square meter of farmland like a custom-tailored suit—precisely matched to its unique soil composition, moisture levels, and pest pressures. This vision is becoming reality through precision agriculture powered by AI. Far from a futuristic concept, it is the cornerstone of modern smart farming, revolutionizing soil analysis, irrigation management, fertilization, and crop health monitoring.
AI-Driven Techniques: From Soil Analysis to Pest Detection
Precision agriculture thrives on detailed field variability insights. AI-powered soil analysis platforms integrate satellite imagery, IoT sensor data, and historical agronomic records to deliver granular assessments of nutrient content, moisture, and soil texture. For example, Farmonaut’s satellite-based soil analysis solutions support farmers in volcanic regions by tailoring nutrient applications to their unique soil chemistry, enhancing crop health and stabilizing yields.
Irrigation scheduling has transitioned from rigid calendars to adaptive, AI-driven systems. A notable implementation in Suceava, Romania, employs IoT sensors to gather continuous soil moisture, temperature, and humidity data. These inputs feed AI models—leveraging advanced architectures such as OpenAI’s generative AI—to dynamically predict optimal watering schedules and volumes. This near real-time adjustment minimizes evaporation losses during heatwaves and prioritizes water allocation amid drought conditions. Such approaches have reported water use efficiency gains up to 30%, a critical achievement considering agriculture accounts for roughly 70% of global freshwater consumption.
Fertilization practices benefit similarly from AI’s precision. Instead of uniform blanket applications, AI models analyze spatial variability to prescribe nutrient doses that prevent overuse and nutrient runoff, simultaneously reducing costs. Pest and disease detection have advanced significantly with AI-powered drones and computer vision. Plantix, for instance, deploys deep learning algorithms diagnosing over 800 crop symptoms across 60 crop types with accuracy exceeding 90%, surpassing typical human expert performance. Early detection enables timely interventions to combat pests and diseases responsible for about 40% of global crop losses annually.
Operational Workflows: From Sensor Data to Automated Machinery
Smart farming orchestrates a tightly integrated workflow uniting sensor networks, AI inference engines, and automated machinery. The process begins with extensive data acquisition: distributed IoT sensors continuously monitor environmental variables such as soil moisture, temperature, nutrient levels, and crop health indicators in real time. Data streams are transmitted wirelessly to cloud platforms, often aggregated in intervals (e.g., every 30 minutes) to optimize bandwidth and cost without sacrificing detail.
Once centralized, AI models analyze these data to generate actionable insights. Predictive analytics forecast irrigation requirements, disease outbreaks, and nutrient deficiencies. Farmonaut’s platform, for example, provides real-time NDVI (Normalized Difference Vegetation Index) maps and soil moisture analytics accessible directly on farmers’ mobile devices, empowering rapid, informed decisions.
The final step is actuation: AI-driven commands control autonomous tractors, sprayers, and irrigation systems. Autonomous tractors equipped with GPS-guided precision steering compliant with ISO 11783 standards can perform variable-rate fertilization or seeding with centimeter-level accuracy. Irrigation controllers, like those from Ceres AI, modulate water delivery weekly per irrigation zone based on AI prescriptions, maximizing both efficiency and profitability.
This seamless feedback loop—from sensor data collection through AI inference to automated action—dramatically reduces resource waste. Smart irrigation alone can decrease water consumption by 20–60%, while precision nutrient delivery typically cuts fertilizer use by approximately 15%. Labor cost savings are notable as well, with intelligent automation reducing operational expenses by $15–20 per acre.
Performance Metrics and Case Studies: Demonstrating Real-World Impact
The tangible benefits of AI-driven precision agriculture are evident in yield improvements, resource savings, and cost reductions. Multiple case studies indicate average crop yield increases of around 20%, water savings up to 30%, and input cost reductions ranging from 10–20%.
Consider AgriBot, an autonomous farming robot integrating computer vision for weed detection and AI for resource allocation. Its deployment across rice and palm oil plantations has maintained optimal growing conditions, resulting in yield increases without proportional input hikes.
Similarly, AI-powered smart greenhouses managed by companies like GreenAI Tech utilize environmental sensor data combined with machine learning to precisely regulate temperature, humidity, and nutrient delivery. These innovations have enhanced crop uniformity, lowered energy consumption, and reduced chemical inputs.
On a larger scale, the EU-funded AgRibot initiative exemplifies how robotics, augmented reality/virtual reality (AR/VR) interfaces, and 5G connectivity can scale precision spraying and harvesting operations, further boosting productivity and sustainability.
Key performance indicators commonly tracked include:
-
Water Use Efficiency (WUE): The ratio of crop output to water consumed, with AI-enabled systems achieving improvements of 20–40% across diverse climate zones.
-
Yield Gains: Crop yields increasing by 15–25% through optimized resource application and proactive disease management.
-
Cost Savings: Operational cost reductions of 10–20% due to automation and precision input use.
-
Environmental Impact: Reduced fertilizer runoff and pesticide application contribute to lower greenhouse gas emissions and mitigate soil degradation.
While these outcomes are promising, challenges persist. High upfront investments, data interoperability hurdles, and the need for farmer training remain barriers to widespread adoption. However, as educational programs expand—such as the Applied Science in Agriculture and precision agriculture solutions at Ivy Tech Community College—and AI tools become more affordable and accessible, even smallholder and resource-poor farmers stand to benefit.
In summary, precision agriculture powered by AI is a cohesive ecosystem where sensors, data analytics, and automated machinery converge to optimize every phase of farming. This transformation is crucial for meeting the dual imperatives of increasing productivity and ensuring sustainability amid mounting environmental and demographic pressures. The journey is ongoing, but the evidence is clear: smart farming is reshaping the future of food production, one sensor and algorithm at a time.
Metric | Description | Impact / Improvement |
---|---|---|
Water Use Efficiency (WUE) | Ratio of crop output to water consumed | 20–40% improvement across diverse climate zones |
Yield Gains | Increase in crop yields through optimized resource application and disease management | 15–25% increase |
Cost Savings | Reduction in operational costs due to automation and precision input use | 10–20% reduction |
Environmental Impact | Reduction in fertilizer runoff and pesticide application | Lower greenhouse gas emissions and mitigated soil degradation |
Water Use Efficiency Gains (Irrigation Systems) | Efficiency gains reported by AI-driven irrigation scheduling | Up to 30% gain |
Water Consumption Reduction (Smart Irrigation) | Decrease in water consumption through smart irrigation | 20–60% reduction |
Fertilizer Use Reduction | Reduction in fertilizer application through precision nutrient delivery | Approximately 15% reduction |
Labor Cost Savings | Cost reduction due to intelligent automation | $15–20 per acre |
AI Crop Diagnosis Accuracy (Plantix) | Accuracy of AI-powered pest and disease detection | Exceeding 90% |
Crop Losses Due to Pests and Diseases | Annual global crop losses caused by pests and diseases | Approximately 40% |
Comparative Analysis: AI-Powered Solutions Versus Traditional Farming Practices
Comparative Analysis: AI-Powered Solutions Versus Traditional Farming Practices
What tangible differences distinguish AI-powered farming from conventional methods? Emerging data in 2025 provides compelling evidence that AI is more than a technological novelty—it is a transformative force impacting agriculture’s core metrics: yield, resource efficiency, labor dynamics, and environmental sustainability.
Yield and Resource Utilization: Quantifying the Gains
Recent studies from UC Berkeley, alongside multiple global case studies, report that AI-driven farming can increase crop yields by an average of 20–49%, varying by crop type and geography. For example, Smart Farm Agritech’s AI systems have boosted rice and palm oil yields by approximately 20%. Meanwhile, predictive analytics combined with real-time monitoring have driven economic profits nearly 50% higher than traditional approaches across diverse regions (Omdena 2025; DigitalDefynd 2025).
This substantial improvement stems primarily from AI’s precision in resource application. IoT sensor networks integrated with machine learning algorithms enable smart irrigation systems that reduce water consumption by up to 25%, a crucial benefit in water-stressed areas (NC State MEM 2025). Similarly, AI-guided fertilizer and pesticide applications focus only on affected zones, significantly cutting chemical inputs and mitigating environmental harm.
In contrast, traditional farming often depends on heuristic-based decisions and blanket treatments, leading to inefficiencies such as overwatering, overfertilization, and delayed detection of pest or disease outbreaks. These practices not only suppress yields but also increase costs and contribute to environmental degradation.
Labor Requirements: Transitioning from Manual Effort to Skilled Oversight
AI automation of repetitive tasks—including weeding, pest control, and crop monitoring—substantially lowers labor demands. Autonomous robots and drones equipped with computer vision perform routine inspections and interventions consistently, operating around the clock without fatigue (DigitalDefynd 2025).
However, this shift does not simply eliminate jobs; it transforms labor profiles. While manual, low-skill roles may decline, there is a growing demand for agronomists and technical specialists capable of managing AI systems, interpreting complex data, and making strategic decisions. This evolution presents challenges: a significant portion of farmers currently lack the technical training needed to fully leverage AI tools, creating adoption bottlenecks (Cloud Security Alliance 2025; CAST Report 2025).
Addressing these training gaps is critical. Educational programs such as Ivy Tech Community College’s Applied Science in Agriculture emphasize precision agriculture solutions, helping bridge the skills divide and empower farmers to integrate AI effectively.
Environmental Impact: Progressing Toward Sustainable Farming Practices
AI enhances environmental sustainability by reducing excessive input use and enabling adaptive responses to climate variability. By fine-tuning irrigation and agrochemical application, AI decreases runoff and contamination risks, preserving local ecosystems (NC State MEM 2025).
Moreover, AI-enhanced satellite imagery—achieving up to 95% accuracy in crop health and yield predictions (Farmonaut 2025)—allows farmers to make proactive adjustments that mitigate crop failures and reduce waste. This contrasts sharply with traditional farming’s reactive approach, which often delays responses to environmental stresses, exacerbating resource waste and ecological strain.
Looking ahead, projections suggest that widespread AI adoption could substantially shrink agriculture’s environmental footprint over the next 25 years, provided it is coupled with policies that emphasize equity and environmental stewardship (Preprints.org 2025).
Infrastructure Gaps, Training Needs, and Data Security Risks
Despite these clear advantages, AI adoption faces tangible hurdles. Infrastructure remains a critical barrier, particularly in rural areas lacking reliable electricity and internet connectivity (USAI 2025). Without these foundational elements, AI tools cannot function effectively or consistently.
The steep learning curve for farmers presents another challenge. Many require comprehensive training and accessible educational resources to unlock AI’s full potential (Palette Skills 2025; Cloud Security Alliance 2025). Programs targeting upskilling are essential to overcome this barrier and ensure equitable technology diffusion.
Data security is an equally pressing concern. As farms become digitally interconnected, vulnerabilities to cyberattacks—including ransomware, supply chain sabotage, and insider threats—increase, threatening food production and farmer livelihoods (CybersecurityGuide 2025). Robust cybersecurity frameworks tailored to the agricultural context are vital for safeguarding these systems.
Socioeconomic Implications: Navigating Labor Displacement and Skill Shifts
AI’s impact on rural labor markets is complex and multifaceted. While it helps alleviate labor shortages caused by demographic shifts and migration, it also raises concerns about job displacement. By 2030, an estimated 14% of workers globally may need to transition to new careers due to AI-driven automation, with agriculture included among affected sectors (ExplodingTopics 2025).
However, the narrative is not one of wholesale replacement. AI acts as a powerful enabler, augmenting farmers’ decision-making capabilities and operational efficiency rather than supplanting human expertise (Global Ag Tech Initiative 2025). Success depends on balancing automation with human insight, ensuring agronomists and farmworkers evolve alongside technology.
Economically, AI adoption can revitalize farming communities through increased yields and profitability. Yet, it also risks widening the digital divide. Smallholder and resource-poor farmers may struggle to access or afford AI technologies, potentially exacerbating inequalities unless targeted support and inclusive innovation models are prioritized (Digital Planet 2025; USAII 2025).
Final Thoughts: Evidence Over Hype, Charting a Balanced Path Forward
The comparative data is unequivocal: AI-powered farming outperforms traditional practices across yield, resource efficiency, labor optimization, and environmental sustainability metrics. Nonetheless, AI is not a silver bullet. Realizing its full potential necessitates addressing infrastructure deficits, equipping farmers with new skills, securing digital ecosystems, and thoughtfully managing socioeconomic transitions.
As AI integrates into agriculture, the imperative is to do so in ways that enhance equity and resilience. Technology should serve as a tool wielded by empowered farmers—not a disruptive force that leaves communities behind. The future of farming hinges on this delicate balance between innovation and inclusion.
Aspect | AI-Powered Farming | Traditional Farming |
---|---|---|
Crop Yield Increase | 20–49% increase (varies by crop and region) | Baseline (no significant increase) |
Economic Profit | Up to ~50% higher profits | Lower profits due to inefficiencies |
Water Consumption | Reduced by up to 25% via smart irrigation | Often overwatering due to heuristic decisions |
Fertilizer & Pesticide Use | Targeted application reducing chemical inputs | Broad, blanket treatments causing overuse |
Labor Requirements | Lower manual labor; increased demand for skilled oversight | High manual labor; low technical skill requirements |
Labor Profile | More agronomists and technical specialists needed | Predominantly manual labor roles |
Environmental Impact | Reduced runoff, contamination, better climate adaptation | Higher environmental degradation and delayed responses |
Crop Health Monitoring Accuracy | Up to 95% accuracy with AI-enhanced satellite imagery | Reactive and less precise monitoring |
Infrastructure Needs | Requires reliable electricity and internet connectivity | Less dependent on infrastructure |
Training & Education | Requires technical training and upskilling programs | Minimal formal technical training needed |
Data Security Risks | Vulnerable to cyberattacks; needs robust cybersecurity | Less digital risk exposure |
Socioeconomic Impact | Potential for job displacement; need for balanced transition | Stable but less efficient labor market |
Smallholder Farmer Challenges | Risk of digital divide and affordability issues | More accessible but less efficient methods |
Real-World Applications and Case Studies: From Automated Robots to Mixed Reality
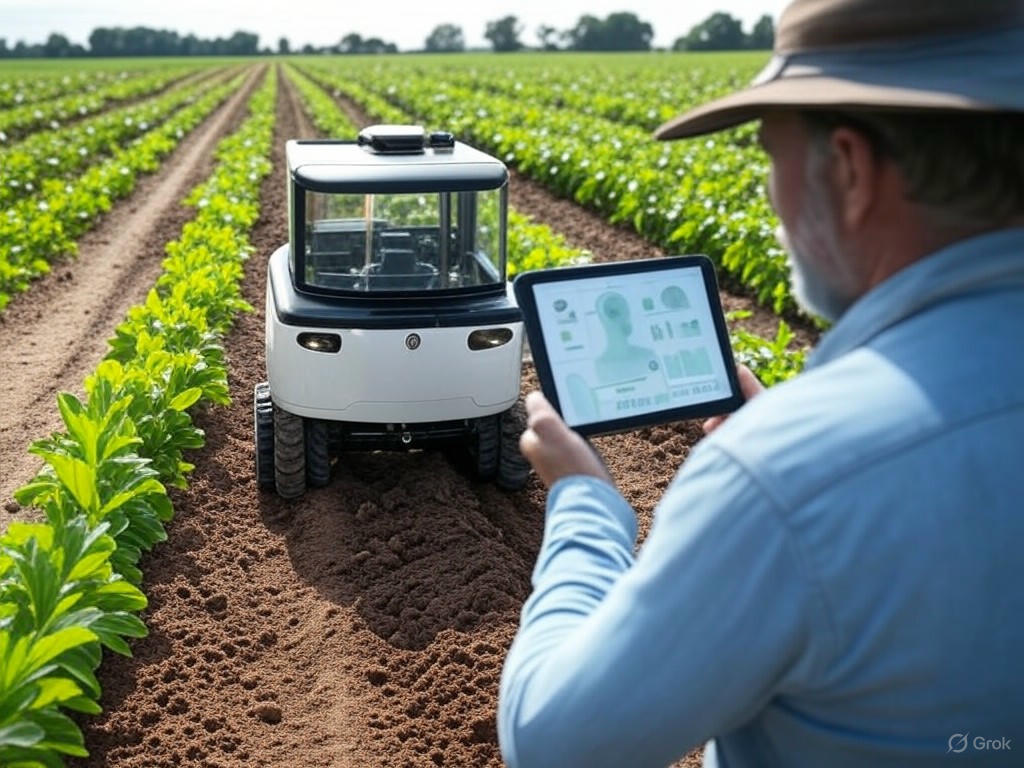
Real-World Applications and Case Studies: From Automated Robots to Mixed Reality
How close are we to farms run by intelligent machines that not only ease human labor but also boost sustainability and precision? The answer lies in a convergence of advanced technologies—from autonomous robots to AI-driven irrigation and emerging mixed reality (MR) interfaces—that are transforming agriculture in tangible, measurable ways. This section explores concrete examples, evaluates their real-world performance, highlights integration challenges, and reflects on farmer acceptance based on recent studies and pilot projects.
AI-Powered Agricultural Robots: Precision Weeding and Automated Harvesting
Autonomous agricultural robots represent a major leap toward smart farming, automating labor-intensive tasks while enhancing environmental stewardship.
-
FarmDroid FD20 is a standout example. This solar-powered field robot uses RTK GPS technology to achieve an outstanding positional accuracy of 8 mm. It automates seeding and employs a two-way mechanical weeding system that targets both inter-row and intra-row weeds. Its innovative +Spray function applies plant protection substances with precision—only 6% chemical treatments, while 94% of weed control is mechanical—significantly reducing pesticide use and environmental impact.
What makes FarmDroid particularly compelling is its energy independence; powered entirely by solar energy, it eliminates fuel dependency and frequent recharging. Remote access capabilities enable FarmDroid Care experts to troubleshoot quickly, enhancing reliability and scalability. Studies reveal an average payback period of two to three years, positioning it as a financially viable investment for small to medium farms.
-
LaserWeeder™ by Carbon Robotics takes automation further with autonomous robotic weed control using precisely targeted lasers. Serving over 100 growers across North America, Europe, and Australia, this technology offers sustainable weed eradication by minimizing soil disturbance and reducing chemical inputs, aligning with eco-friendly farming practices.
-
Harvesting robots are advancing as well, though still maturing in field mobility. Current designs use mobile platforms with wheels or tracks to navigate complex terrains. Integration challenges remain significant: robots must seamlessly coordinate with existing farm machinery, adapt to diverse crop types, and operate reliably under unpredictable weather conditions. Nevertheless, pilot projects demonstrate promising reductions in labor costs and improved operational accuracy.
Despite these technological strides, adoption is moderated by factors such as upfront costs, interoperability with farm data systems, and variable field conditions. Building farmer trust is crucial, as is ensuring that these robots integrate smoothly into existing workflows without disrupting operations.
AI-Driven Disease Prediction Models and Smart Irrigation Systems: Data-Driven Crop Health
While robots handle physical tasks, AI-based disease detection and smart irrigation systems serve as the cognitive core of precision agriculture.
-
Advanced deep learning architectures such as DenseNet-121 and Swin Transformer have achieved disease identification accuracy exceeding 90% for crops including banana, potato, fig, and custard apple. A hybrid model combining VGG19 with k-Nearest Neighbors (k-NN) notably reached 99.1% accuracy detecting custard apple leaf disease.
-
These models leverage high-resolution imagery and hyperspectral data that extend beyond visible light, enabling early detection of subtle disease symptoms invisible to the naked eye. Initiatives like Kaggle’s “Beyond Visible Spectrum” challenge are accelerating innovation in this space, promoting AI solutions that enhance sustainable disease management.
-
On the irrigation side, AI-integrated smart systems such as Full Nature Farms’ Rocket 2.0 combine sensor networks with AI algorithms to analyze soil moisture, weather forecasts, and crop water requirements. This platform delivers up to a 30% reduction in water consumption alongside energy savings, with real-time monitoring accessible via mobile apps for remote oversight.
-
A critical technical hurdle is harmonizing heterogeneous data streams—from soil sensors, satellite and drone imagery, to climatic databases—into precise, actionable irrigation schedules. Despite this complexity, real-world case studies confirm that these AI systems not only conserve water but also improve crop quality and yields, effectively balancing economic and environmental objectives.
Emerging Mixed Reality Interfaces for Crop Monitoring: Augmenting Farmer Insight
Mixed reality (MR) technologies are beginning to augment farmer decision-making by fusing AI and IoT data into immersive, real-time visualizations.
-
Imagine walking through a field or greenhouse while wearing smart glasses or using a tablet that overlays plant-specific data, from growth metrics to stress indicators. Research at Penn State has developed automated systems integrating IoT sensors and AI to support controlled environment agriculture, reporting enhanced crop quality and reduced inefficiencies in early pilot projects.
-
Collaborative efforts such as the University of Arizona and Purdue University’s VR-Bio-Talk project are pioneering virtual reality platforms to visualize complex biological datasets. This cross-disciplinary approach bridges biology, agriculture, and computer science, enabling intuitive interaction with multidimensional data for both researchers and farmers.
-
Complementing MR, drones equipped with advanced sensors like Lidar and AESA radar collect spatial and spectral data at scale. When combined with ground-based sensors, they provide layered insights—from soil nutrient profiles to pest presence—informing precision interventions.
Despite its promise, wide adoption of MR in agriculture faces barriers including high initial costs, technical complexity, and user acceptance challenges. Research suggests that for MR to scale effectively, interfaces must become more intuitive, affordable, and demonstrably valuable in everyday farming operations.
Assessing Effectiveness, Scalability, and Farmer Acceptance
Across these technologies, effectiveness is demonstrated through multiple pilot projects and early deployments:
-
FarmDroid FD20 has delivered measurable reductions in manual labor, pesticide usage, and CO₂ emissions, with ROI timelines aligning with practical investment horizons.
-
AI disease detection models consistently achieve high accuracy across diverse crops, enabling earlier interventions that reduce crop losses and input waste.
-
Smart irrigation systems report substantial water and energy savings, validated by real-world implementations, contributing directly to sustainability goals.
-
Mixed reality interfaces, though nascent, enhance data visualization and decision-making processes, with ongoing improvements in usability necessary for broader uptake.
Scalability varies by technology. Solar-powered robots can serve small to medium farms effectively due to their energy independence, but integrating them with existing farm management systems remains complex. Disease prediction and irrigation AI models demand substantial, high-quality data and require calibration to local environmental conditions, necessitating ongoing support and refinement.
Farmer acceptance depends largely on trust and clear value propositions. Technologies that demonstrably reduce risk, deliver economic benefits, and integrate seamlessly into existing workflows are more readily adopted. Conversely, high upfront costs, technical complexity, and uncertain reliability slow broader acceptance.
As these AI-driven solutions mature, the synergy between automation, intelligent analytics, and augmented human decision-making will drive the next agricultural revolution. The imperative is not merely to build smarter machines but to ensure they empower farmers sustainably, scalably, and accessibly—meeting the urgent challenge of feeding a growing global population within environmental limits.
Technology | Examples | Key Features | Benefits | Challenges | Farmer Acceptance Factors |
---|---|---|---|---|---|
AI-Powered Agricultural Robots | FarmDroid FD20, LaserWeeder™, Harvesting robots | Solar-powered, RTK GPS (8mm accuracy), autonomous seeding, mechanical & chemical weeding, laser weed control, mobile platforms for harvesting | Reduced manual labor, decreased pesticide use, energy independence, labor cost reduction, precision operations | Integration with farm machinery, terrain navigation, weather adaptability, upfront costs, interoperability | Trust building, ROI within 2-3 years, smooth workflow integration |
AI-Driven Disease Prediction Models & Smart Irrigation | DenseNet-121, Swin Transformer, VGG19+k-NN hybrid, Full Nature Farms’ Rocket 2.0 | High accuracy (>90%), hyperspectral & high-res imagery, sensor networks, AI algorithms for irrigation scheduling | Early disease detection, reduced crop losses, 30% water & energy saving, improved crop quality and yield | Data harmonization from multiple sources, calibration to local conditions, technical complexity | Clear economic & environmental benefits, data quality dependency, ongoing support needs |
Mixed Reality Interfaces for Crop Monitoring | Penn State IoT-AI systems, VR-Bio-Talk (University of Arizona & Purdue), Drones with Lidar & AESA radar | Immersive visualization via smart glasses/tablets, integration of AI & IoT data, multi-sensor data layering | Enhanced decision-making, improved crop quality, reduced inefficiencies | High initial costs, technical complexity, user acceptance, interface intuitiveness | Affordability, usability improvements, demonstrated operational value |
Future Trends and Innovations in AI-Driven Agriculture
Future Trends and Innovations in AI-Driven Agriculture
Imagine simulating an entire growing season before planting a single seed. Generative AI is rapidly making this possible by enabling predictive crop modeling that surpasses traditional forecasting methods. These “virtual farms” let farmers and agribusinesses test various inputs—such as water levels, fertilizer types, and planting dates—and forecast outcomes with remarkable precision.
Platforms powered by generative AI already optimize resource allocation, anticipate pest outbreaks, and model climate stress impacts. Running thousands of simulations accelerates decision-making and minimizes costly trial-and-error in the field, transforming agricultural planning into a data-driven science.
Enhanced IoT Connectivity: Building Responsive Farm Ecosystems
Complementing AI’s predictive capabilities, advancements in Internet of Things (IoT) connectivity are weaving farms into highly responsive, data-rich ecosystems. Networks of sensors continuously transmit real-time data on soil moisture, temperature, and nutrient levels, providing farmers with granular insight into crop health and environmental conditions around the clock.
Technologies like LoRaWAN overcome rural connectivity challenges, enabling seamless communication between devices. The global agriculture IoT market is projected to exceed $33 billion by 2032, underscoring the critical role of smart farming tools in enhancing efficiency and reducing waste.
Hyperspectral Imaging: Seeing Beyond the Visible Spectrum
Hyperspectral imaging represents a cutting-edge frontier in agricultural monitoring. Unlike standard RGB cameras, hyperspectral sensors capture data across dozens or hundreds of narrow spectral bands. This reveals subtle biochemical and physiological changes in crops that are invisible to the naked eye.
Such detailed spectral data enables early detection of diseases, nutrient deficiencies, and water stress long before visual symptoms appear. Snapshot hyperspectral cameras, like those developed by Cubert, now support real-time data capture, empowering farmers to intervene precisely and sustainably. The hyperspectral imaging market is expected to grow at a compound annual rate of 9.4% through 2029, reflecting its rising importance in agriculture.
AI-Powered Sustainable Farming: Climate Resilience and Resource Conservation
Technology alone does not define the future—AI-powered sustainable farming initiatives are at the forefront of addressing climate resilience and resource conservation. Precision agriculture, driven by AI and big data, fine-tunes water and fertilizer application to reduce environmental footprints while maintaining or even increasing yields.
For example, AgroAI’s intelligent irrigation platform has demonstrated water savings of up to 25% through dynamic, data-driven scheduling. Similarly, SenseGrass combines AI, machine learning, and satellite monitoring to provide real-time soil health assessments, helping farmers adapt to climate variability and preserve vital soil carbon stocks.
Regenerative agriculture, supported by AI, offers a promising holistic approach that actively restores soil health, enhances biodiversity, and fosters ecosystem services. Digital twins—virtual representations of entire farms—allow simulation of regenerative practices and measurement of their impacts before implementation. As Ron Baruchi of Agmatix emphasizes, emerging data tools will quantify farming’s effects on local ecosystems, aligning innovation with nature-positive goals.
Navigating Regulatory, Ethical, and Security Challenges
While AI’s potential is vast, it brings complex regulatory, ethical, and security considerations that will shape its future adoption in agriculture. Farmers frequently express concerns about data ownership, privacy, and transparency. Biased training data can lead to unfair AI decisions, disproportionately impacting smallholders and marginalized communities.
Without robust governance frameworks and inclusive development, AI risks deepening existing inequalities rather than alleviating them. Trusted collaborations among policymakers, technology providers, and farmers are essential to foster responsible AI ecosystems that respect local knowledge and ensure equitable access.
Cybersecurity risks—including ransomware, supply chain sabotage, and insider threats—also demand attention as farms become increasingly connected and automated. Safeguarding AI systems and data infrastructure will be critical to maintaining trust and operational continuity.
The Evolving Role of the Farmer in a Data-Driven Ecosystem
As farms become more automated and data-centric, the role of the farmer is set to evolve—not disappear. Rather than being replaced by machines, farmers will increasingly act as expert interpreters of AI-generated insights and stewards of sustainable practices.
Their deep contextual knowledge, combined with AI’s analytical power, will drive smarter and more adaptive farming. Future farmers might trade some traditional tools for digital dashboards, adopting a data scientist’s mindset while balancing hands-on experience with technological fluency.
Looking Ahead: An Agricultural Revolution in the Making
In summary, the convergence of generative AI, enhanced IoT connectivity, hyperspectral imaging, and sustainable farming practices is ushering in a new agricultural revolution. This revolution promises higher yields, optimized resource use, and greater resilience to climate shocks—if ethical challenges are addressed thoughtfully and farmers remain central to innovation.
Over the next decade, farms could transform into living laboratories where technology and nature coalesce. By blending AI-driven insights with ecological stewardship, agriculture can sustainably feed a growing global population while healing the planet.
Trend / Innovation | Description | Key Benefits | Market/Impact Data | Example Technologies / Companies |
---|---|---|---|---|
Generative AI for Predictive Crop Modeling | Simulates entire growing seasons to test inputs and forecast outcomes with high precision | Optimizes resource use, anticipates pest outbreaks, models climate stress, accelerates decision-making | Transforms agricultural planning into data-driven science | Virtual farms, AI-driven simulation platforms |
Enhanced IoT Connectivity | Networks of sensors transmit real-time data on soil moisture, temperature, nutrients | Granular crop and environmental insights, responsive farm ecosystems | Global agriculture IoT market projected to exceed $33 billion by 2032 | LoRaWAN, Smart Farming Tools |
Hyperspectral Imaging | Captures data across many spectral bands to detect biochemical changes invisible to naked eye | Early detection of diseases, nutrient deficiencies, water stress; real-time interventions | Market growing at 9.4% CAGR through 2029 | Cubert snapshot hyperspectral cameras |
AI-Powered Sustainable Farming | Precision agriculture using AI and big data to reduce environmental footprint and increase yields | Water savings, soil health assessments, climate resilience, ecosystem restoration | Water savings up to 25% demonstrated; increasing adoption of regenerative practices | AgroAI irrigation, SenseGrass, Agmatix digital twins |
Regulatory, Ethical, and Security Challenges | Issues around data ownership, privacy, bias, equitable access, and cybersecurity risks | Need for governance frameworks, trusted collaborations, and robust security measures | Critical for responsible AI adoption and maintaining trust | Policy makers, technology providers, farmers collaborations |
Evolving Role of the Farmer | Farmers become expert interpreters of AI insights and stewards of sustainable practices | Combines contextual knowledge with AI analytics; adoption of digital tools and data science mindset | Supports smarter, adaptive farming and balances technology with experience | Digital dashboards, AI decision support systems |
Conclusion: Synthesizing AI’s Potential and Challenges for Sustainable Food Production
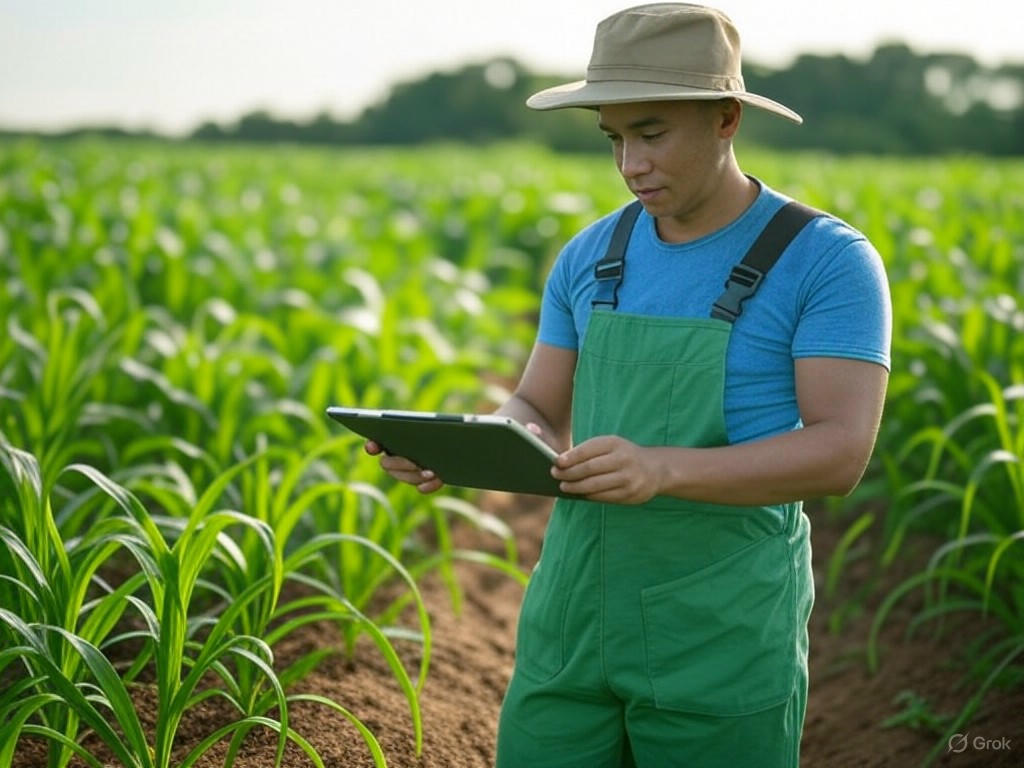
Conclusion: Synthesizing AI’s Potential and Challenges for Sustainable Food Production
What if the future of farming was defined not merely by bigger yields but by smarter, more sustainable ones? The integration of AI into agriculture offers a powerful lever to meet the critical challenge of feeding a global population expected to grow by nearly 2 billion by 2050, without expanding farmland or exhausting natural resources.
The Transformative Power of AI in Agriculture
AI technologies are already reshaping farming from the ground up. For example, Missouri S&T’s AI-powered pest detection traps combine sensors, cameras, and edge computing to provide real-time alerts, enabling farmers to protect crops more precisely while reducing pesticide use. Similarly, AI-driven smart irrigation and disease detection systems allow farmers to optimize water use and identify crop health issues early, reducing waste and improving resilience against climate variability.
The economic incentives for adopting AI in agriculture are compelling. Farmers can expect an average return of $3.70 for every dollar invested within three years. Meanwhile, the global AgTech market is projected to reach $74 billion by 2034, growing annually by over 12%. This rapid expansion reflects AI’s ability to transform disparate data streams—ranging from soil sensors and satellite imagery to weather forecasts—into actionable insights that were previously unattainable.
Balancing Technology with Human Expertise
Despite these advances, AI is not a panacea. The most effective implementations amplify rather than replace human expertise. Farmers possess indispensable contextual knowledge about local soil conditions, seasonal cycles, and market demands that no AI model can fully capture.
This human-AI partnership is essential. While AI automates data collection and provides predictive analytics, skilled farmers remain the strategic decision-makers interpreting these insights within the complexities of their operations. Colin Hurd, CEO of Mach, anticipates that the next five years will embed advanced AI technologies deeply into farm machinery and workflows, enabling farmers to work smarter and more sustainably.
Equally important is education and training. Programs like Ivy Tech Community College’s Applied Science in Agriculture and precision agriculture solutions help bridge the skills gap, empowering farmers to leverage AI tools effectively. Without this balance, AI risks becoming an inaccessible luxury or a source of frustration among those it aims to support.
Persistent Challenges and the Need for Collaborative Solutions
Despite promising results, AI adoption in agriculture faces significant barriers. High upfront costs and technical complexity limit accessibility, particularly for small and medium-sized farms. Data quality and availability remain uneven, hindering the development of robust, localized AI models. Concerns around data privacy, ownership, and ethical use also persist.
Policymakers and industry stakeholders must develop adaptive governance frameworks that encourage innovation while protecting farmers’ rights and environmental integrity. The World Economic Forum emphasizes that sustainable AI in agriculture depends on close collaboration between governments, businesses, and farming communities to overcome financial and technological obstacles.
Moreover, AI must align with regenerative agriculture principles—prioritizing not only productivity but also soil health, biodiversity, and climate mitigation. Without deliberate policy guidance, AI could inadvertently deepen environmental and social inequalities.
Charting a Responsible Path Forward
AI’s promise in agriculture is immense but conditional. Realizing this potential requires:
- Investment in affordable, user-friendly AI solutions that respect diverse farming contexts and resource levels.
- Educational initiatives to equip farmers with the skills needed to interpret and apply AI insights effectively.
- Robust policy frameworks addressing ethical, environmental, and socio-economic dimensions of AI deployment.
- Inclusive collaboration among technologists, policymakers, agronomists, and farmers to ensure equitable access and responsible innovation.
AI is not a replacement for farmers but a transformative tool—a force multiplier that, when thoughtfully integrated, can help cultivate a food system that is more productive, resilient, and sustainable. The question is no longer if AI will reshape farming, but how deliberately and responsibly we design that future.
Aspect | Details |
---|---|
AI Applications in Agriculture | Pest detection traps, smart irrigation, disease detection, predictive analytics |
Economic Impact | Average return of $3.70 per $1 invested within 3 years; AgTech market projected to reach $74 billion by 2034 with 12% annual growth |
Human-AI Partnership | AI amplifies human expertise; farmers remain decision-makers; education and training essential |
Challenges | High upfront costs, technical complexity, uneven data quality, data privacy and ethical concerns |
Policy and Governance | Need for adaptive frameworks, collaboration among governments, businesses, and farmers; alignment with regenerative agriculture principles |
Recommendations for Future | Investment in affordable AI, education initiatives, robust policy frameworks, inclusive collaboration |
Overall Conclusion | AI is a transformative tool that can enhance sustainable farming when integrated responsibly |