Top 5 AutoML Platforms in 2024: Balanced Review & Use Cases
- Introduction: Why Comparing Top AutoML Platforms Matters in 2024
- Introduction: Why Comparing Top AutoML Platforms Matters in 2024
- AutoML: The Great Democratizer of Machine Learning
- From No-Code Simplicity to Advanced MLOps Integration
- What to Look For: Selection Criteria for Top AutoML Platforms
- Why This Analysis Matters Now
- Conclusion: Navigating the AutoML Landscape with Technical Rigor and Ethical Awareness
- Conclusion: Navigating the AutoML Landscape with Technical Rigor and Ethical Awareness
- Technical Features and Scalability: Striking the Right Balance
- User Accessibility: Democratizing AI with Necessary Caution
- Ethical Considerations and AI Governance: Beyond Compliance Checklists
- The Uncertain Frontier: Performance Limits and the Evolving Role of Human Oversight
- Viewing AutoML as an Enabler, Not a Silver Bullet
- 1. Google Cloud Vertex AI: Unified MLOps with Scalable AutoML
- Google Cloud Vertex AI: Unified MLOps with Scalable AutoML
- An End-to-End Managed Architecture
- Technical Specifications and Model Support
- Performance, Monitoring, and Enterprise Use Cases
- Weighing the Pros and Cons
- Final Thoughts
- 2. DataRobot: Enterprise AI Automation for Predictive Modeling
- DataRobot: Enterprise AI Automation for Predictive Modeling
- Automated Feature Engineering, Model Selection, and Validation
- Explainability and Model Governance: Transparency at Scale
- Deployment Options and Real-Time Prediction APIs
- Use Cases: Finance, Healthcare, and Manufacturing
- 3. Microsoft Azure Machine Learning: Robust Cloud-Native AutoML with MLOps Integration
- Microsoft Azure Machine Learning: Robust Cloud-Native AutoML with MLOps Integration
- AutoML Capabilities Enhanced by MLOps Integration
- Developer Experience and Ecosystem Compatibility
- Scalability: Distributed Training and GPU Acceleration
- Responsible AI and Ethical AI Toolkits
- Use Cases: Retail and Industrial IoT Deployments
- 4. Alteryx Intelligence Suite: Democratizing AI with No-Code and Low-Code Automation
- Alteryx Intelligence Suite: Democratizing AI with No-Code and Low-Code Automation
- User-Friendly Interface Empowering Non-Technical Users
- Technical Capabilities: Data Connectors, Automated Feature Engineering, and Visual Workflow Design
- Strengths: Accelerating Time-to-Insight for Business Analysts and SMEs
- Limitations: Complex Model Customization and Scalability Challenges
- Real-World Impact: Marketing Analytics and Customer Segmentation
- 5. IBM Watson Studio AutoAI: Hybrid Cloud AI with Emphasis on Model Explainability and Compliance
- 5. IBM Watson Studio AutoAI: Hybrid Cloud AI with Emphasis on Model Explainability and Compliance
- Core Automation Features: Comprehensive Pipeline Automation and Framework Support
- Explainability, Bias Detection, and Audit Trails: Built for Ethical and Compliant AI
- Use Cases: Addressing Compliance and Transparency in High-Stakes Industries
- Advanced Pipeline Generation and Hybrid Cloud Integration
- Final Thoughts
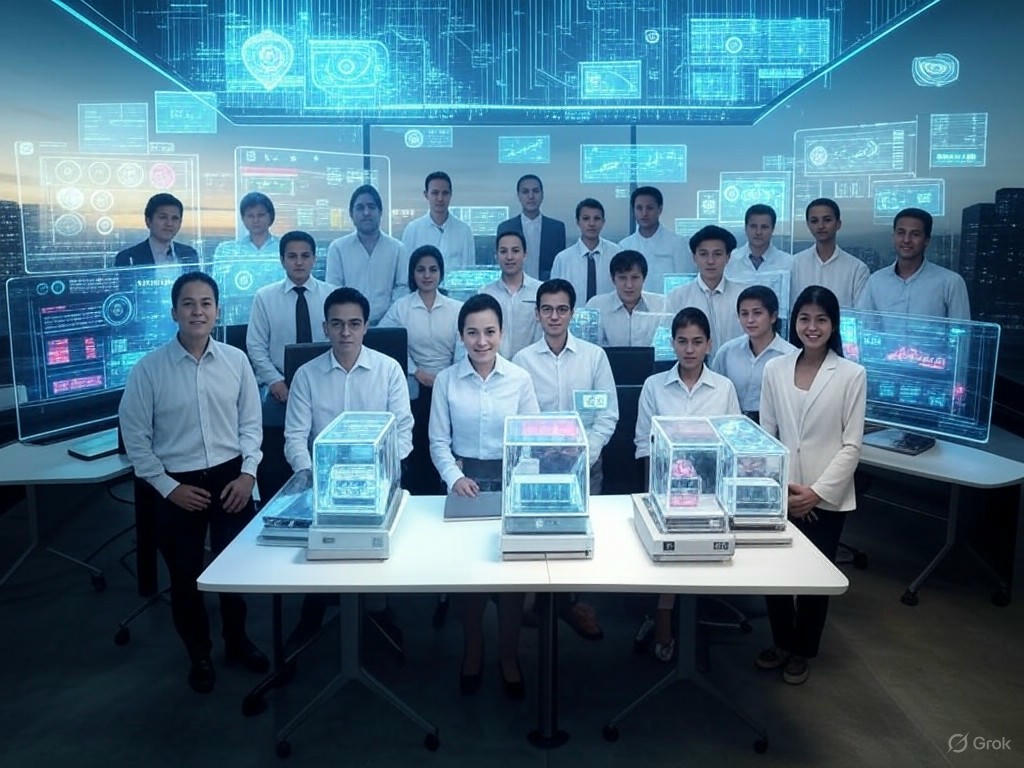
Introduction: Why Comparing Top AutoML Platforms Matters in 2024
Introduction: Why Comparing Top AutoML Platforms Matters in 2024
What if building effective machine learning models no longer required deep expertise or months of painstaking manual effort? That’s the promise—and increasingly, the reality—of Automated Machine Learning, or AutoML. In 2024, AutoML has evolved from a niche convenience into a pivotal technology that democratizes AI, accelerates adoption, and reshapes how organizations innovate with data.
AutoML: The Great Democratizer of Machine Learning
AutoML automates the entire machine learning pipeline, including data preprocessing, feature engineering, model selection, and hyperparameter tuning. This level of automation significantly lowers technical barriers, enabling domain experts, business analysts, and even non-technical professionals to leverage predictive analytics and AI-driven insights.
For example, retailers use AutoML-powered recommendation engines to personalize shopping experiences, while companies like Airbus employ it to predict aircraft sensor failures well before they occur, enhancing operational reliability.
Looking ahead, by 2025 nearly three-quarters of enterprise AutoML implementations are expected to include end-to-end lifecycle management. This reflects a significant maturation beyond simple no-code tools. Model development timelines have compressed from months to days—or even hours—supporting rapid experimentation and deployment.
However, with this power comes responsibility. Ethical considerations such as bias mitigation, data privacy, and transparency remain critical as AI becomes increasingly embedded in decision-making processes.
From No-Code Simplicity to Advanced MLOps Integration
AutoML began as a no-code solution aimed at making machine learning accessible to everyone. Today, it is rapidly evolving into sophisticated platforms that automate model building while integrating seamlessly with MLOps workflows.
This evolution is crucial because building a model is only part of the story. Deploying, monitoring, and maintaining models at scale requires robust operational support.
Leading platforms—including Google’s Vertex AI AutoML, Microsoft Azure AutoML, and Amazon SageMaker Canvas—combine user-friendly interfaces with granular control and transparency for developers. Open-source solutions like Uber’s Ludwig specialize in simplifying large language model tasks with minimal coding, catering to niche use cases.
This blend of accessibility and advanced integration reflects the growing recognition that AutoML must serve diverse user personas—from business analysts seeking quick insights to data scientists requiring customizable pipelines.
What to Look For: Selection Criteria for Top AutoML Platforms
Selecting the right AutoML platform is less about choosing the flashiest tool and more about aligning features with practical needs. Key criteria include:
- Technical Capability: Support for various data types and algorithms, along with advanced features like automated feature engineering and hyperparameter optimization.
- Ease of Use: Intuitive, no-code or low-code interfaces and comprehensive documentation to enable rapid onboarding.
- Scalability: Ability to process large datasets, support distributed training, and integrate smoothly with cloud infrastructure.
- Integration: Compatibility with existing data pipelines, MLOps ecosystems, and deployment environments.
- Ethical Considerations: Tools to detect and mitigate bias, ensure model explainability, and maintain data privacy compliance.
- Real-World Applicability: Proven success in industry scenarios such as retail personalization, predictive maintenance, or financial risk modeling.
These criteria help cut through the common hype around AutoML—often marketed as “magic in a box” but sometimes lacking transparency or adaptability.
This article adopts a persona-driven, evidence-based approach, combining rigorous technical analysis with ethical and operational insights. The goal is to provide a balanced perspective grounded in actual deployments and user experiences.
Why This Analysis Matters Now
As AutoML platforms proliferate and mature, organizations face a paradox of choice. The right platform can accelerate AI initiatives, reduce operational costs, and empower broader teams. The wrong choice can lead to wasted resources, opaque models, and missed opportunities.
This article offers a deep dive into the top 5 AutoML platforms of 2024, analyzing their strengths, limitations, and ideal use cases. It is a technical yet accessible guide tailored for data practitioners, IT decision-makers, and business leaders aiming to make informed decisions—not just follow trends.
In a rapidly shifting AI landscape, evidence-based, persona-driven analysis is essential to distinguish genuine innovation from marketing noise. AutoML is transforming how intelligence is built into systems; understanding where it excels and where caution is warranted is key to unlocking its full potential.
Selection Criteria | Description |
---|---|
Technical Capability | Support for various data types and algorithms, automated feature engineering, hyperparameter optimization |
Ease of Use | Intuitive no-code or low-code interfaces, comprehensive documentation for rapid onboarding |
Scalability | Ability to process large datasets, distributed training, cloud infrastructure integration |
Integration | Compatibility with existing data pipelines, MLOps ecosystems, deployment environments |
Ethical Considerations | Tools for bias detection and mitigation, model explainability, data privacy compliance |
Real-World Applicability | Proven success in industry scenarios like retail personalization, predictive maintenance, financial risk modeling |
Conclusion: Navigating the AutoML Landscape with Technical Rigor and Ethical Awareness
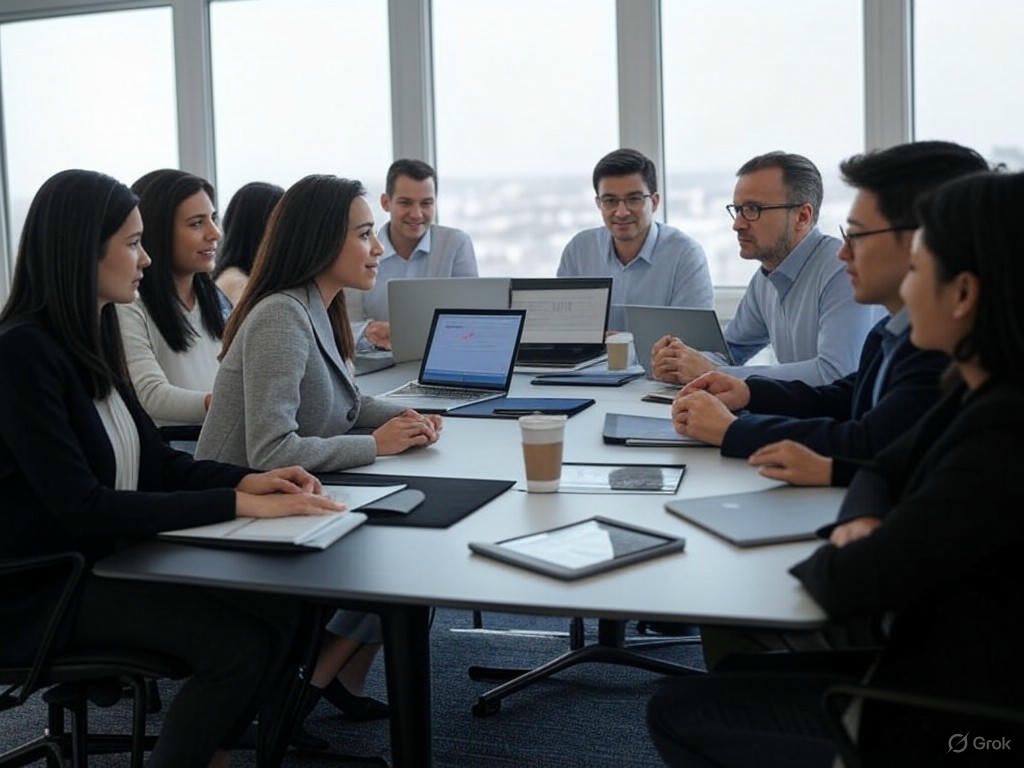
Conclusion: Navigating the AutoML Landscape with Technical Rigor and Ethical Awareness
Choosing the right AutoML platform in today’s crowded market requires a nuanced understanding of each solution’s technical strengths, scalability, user accessibility, and ethical implications. No single tool fits all organizational contexts—each platform embodies unique trade-offs that must be carefully evaluated to align with business needs and AI governance frameworks.
Technical Features and Scalability: Striking the Right Balance
AutoML platforms vary significantly in how they orchestrate the end-to-end machine learning pipeline, from data ingestion and feature engineering to model selection, training, and deployment.
-
DataRobot empowers business analysts with an intuitive interface and automated feature engineering, making predictive analytics accessible without deep coding skills. Its Feature Discovery and Autopilot modes automate complex steps like generating hundreds of new features and model validation using K-fold cross-validation, supporting regulated industries with explainability via SHAP and governance controls.
-
H2O AutoML offers a robust open-source approach favored by data scientists who value algorithmic breadth and flexibility. Written in Java, it efficiently handles supervised learning tasks but, like most platforms, has limited support for unsupervised or reinforcement learning.
-
Google Vertex AI AutoML and Microsoft Azure Machine Learning stand out as cloud-native, end-to-end platforms integrating automated model building with mature MLOps ecosystems. They provide scalable infrastructure, distributed training, and seamless integration with cloud data services, supporting diverse modalities including tabular data, vision, video, and text. Vertex AI’s incorporation of the Gemini AI model family and Azure ML’s responsible AI toolkits highlight their technical sophistication and enterprise readiness.
-
Uber Ludwig specializes in large language models (LLMs) with minimal coding, appealing to teams advancing state-of-the-art NLP applications.
Scalability extends beyond raw compute capacity to include data quality management, versioning, and workload orchestration. Cloud platforms offer auto-scaling and redundancy essential for production environments with fluctuating demands but may trade off customization or transparency. Conversely, open-source platforms provide more control but require greater expertise and operational investment.
User Accessibility: Democratizing AI with Necessary Caution
AutoML’s promise to democratize machine learning is realized by lowering technical barriers, enabling business analysts and subject matter experts to participate actively in AI initiatives. Platforms like DataRobot and Amazon SageMaker Canvas exemplify this by offering no-code or low-code interfaces that facilitate rapid prototyping and deployment.
However, simplifying model development carries risks:
- Important nuances such as data biases, data quality issues, and domain-specific contexts can be obscured.
- Interpretability challenges arise, particularly in regulated sectors like healthcare and finance, where understanding model decisions is critical.
- Default configurations may not suit complex or unique business environments, risking suboptimal or misleading outcomes.
Therefore, human expertise remains indispensable. Data scientists and domain experts must validate models, diagnose errors, and ensure that automated pipelines faithfully reflect organizational objectives and constraints. AutoML should be viewed as an augmentation tool that empowers humans rather than a replacement for expert judgment.
Ethical Considerations and AI Governance: Beyond Compliance Checklists
Ethical AI is no longer optional but foundational. While 77% of companies prioritize AI compliance, only 4% have dedicated cross-functional teams to manage it, underscoring a gap between intent and execution.
When evaluating AutoML platforms, organizations should consider:
-
Transparency: Availability of explainability tools like SHAP and LIME to clarify model decisions and comply with regulatory requirements such as the EU AI Act.
-
Bias Mitigation: Mechanisms to detect and reduce bias are essential to avoid discriminatory outcomes, as past AI failures (e.g., biased hospital algorithms or Amazon’s hiring tool) have demonstrated.
-
Data Privacy and Security: Compliance with GDPR, HIPAA, CCPA, and other frameworks is critical, especially when handling sensitive or personal data.
-
Governance Integration: Support for embedding AI governance frameworks (such as Credo AI or Holistic AI) facilitates risk management, auditability, and accountability.
Ignoring these facets risks reputational damage, legal penalties, and erosion of stakeholder trust. Aligning platform capabilities with organizational AI ethics and governance policies ensures responsible and sustainable AI adoption.
The Uncertain Frontier: Performance Limits and the Evolving Role of Human Oversight
Despite rapid advances, AutoML is not a universal solution. Automated pipelines excel at supervised learning with clean, labeled data but remain challenged by unsupervised learning, reinforcement learning, and complex domain-specific adaptations.
Real-world deployments reveal ongoing uncertainties:
- Models can degrade due to data drift or changes in operational environments.
- Automated hyperparameter tuning may optimize for certain metrics (e.g., accuracy or AUC-ROC) but overlook business-critical nuances.
- Proper selection and interpretation of evaluation metrics require domain expertise.
These realities reinforce the vital role of human oversight. Data scientists should continuously monitor model performance, validate outputs, and iteratively tune pipelines. AutoML serves best as a dynamic enabler within a human-in-the-loop framework, ensuring models remain accurate, fair, and aligned with evolving business objectives.
Viewing AutoML as an Enabler, Not a Silver Bullet
The true value of AutoML lies in accelerating machine learning adoption and broadening participation across organizational roles. Yet, success demands a clear-eyed understanding of its limitations and responsibilities.
Key takeaways include:
-
Align platform features with your specific use cases and team expertise. There is no universal solution; matching capabilities to needs prevents costly missteps.
-
Embed ethical AI principles and compliance from the outset. Incorporate transparency, bias detection, and governance into workflows to build trust and meet regulatory demands.
-
Maintain human-in-the-loop processes. Automation expedites workflows but cannot substitute for domain knowledge, critical judgment, and contextual awareness.
-
Commit to continuous evaluation and adaptation. AI systems operate in dynamic environments; ongoing monitoring and iterative improvement are essential.
When implemented with technical rigor and ethical stewardship, AutoML platforms unlock significant business value by empowering organizations to innovate faster and more inclusively. However, like any advanced technology, they require thoughtful integration, vigilant oversight, and an adaptive mindset to realize their full potential responsibly.
Aspect | DataRobot | H2O AutoML | Google Vertex AI AutoML | Microsoft Azure Machine Learning | Uber Ludwig |
---|---|---|---|---|---|
Target Users | Business analysts, minimal coding | Data scientists, algorithmic flexibility | Enterprise, cloud-native users | Enterprise, cloud-native users | NLP teams, minimal coding |
Key Technical Features | Automated feature engineering, Feature Discovery, Autopilot, SHAP explainability, governance controls | Open-source, broad algorithm support, Java-based, supervised learning focus | Cloud-native, Gemini AI integration, scalable training, multi-modal data support | Cloud-native, MLOps ecosystem, responsible AI toolkits, multi-modal data support | Large language models specialization, minimal coding |
Scalability | Supports regulated industries with explainability; automation with less coding | Efficient for supervised tasks; requires expertise for deployment | Scalable infrastructure, distributed training, auto-scaling, redundancy | Scalable infrastructure, distributed training, auto-scaling, redundancy | Focus on NLP scalability with low coding effort |
User Accessibility | Intuitive no-code/low-code interface for analysts | Requires data science expertise | Cloud-integrated, user-friendly interfaces | Cloud-integrated, user-friendly interfaces | Minimal coding for NLP model building |
Ethical & Governance Features | Explainability (SHAP), governance controls | Limited explicit governance tools | Responsible AI toolkits, compliance support | Responsible AI toolkits, compliance support | Not specifically focused on ethics/governance |
Strengths | Accessibility, explainability, regulated industries support | Algorithmic flexibility, open-source control | Enterprise readiness, multi-modal data, cloud-native MLOps | Enterprise readiness, multi-modal data, cloud-native MLOps | State-of-the-art NLP with simple workflows |
Limitations | Less suited for deep technical customization | Limited unsupervised/reinforcement learning support | Potential trade-offs in customization/transparency | Potential trade-offs in customization/transparency | Focus limited to NLP applications |
1. Google Cloud Vertex AI: Unified MLOps with Scalable AutoML
Google Cloud Vertex AI: Unified MLOps with Scalable AutoML
What does it mean to truly unify machine learning development and operations? Google Cloud’s Vertex AI exemplifies this vision by delivering an end-to-end managed service that seamlessly integrates AutoML, custom training, and MLOps pipelines into a single, cohesive platform. This architecture is designed not only for convenience but also to scale with the complexity and volume of enterprise workloads while supporting rigorous data governance and compliance.
An End-to-End Managed Architecture
Vertex AI streamlines every stage of the ML lifecycle—from data ingestion and model training to deployment and continuous monitoring. It provides intuitive workflows that reduce operational friction and accelerate time-to-insight. For example, users can start with AutoML to build models without writing code, then switch to custom training for more control or specialized architectures. This flexibility is essential for enterprises managing heterogeneous data sources and varying levels of in-house ML expertise.
A standout feature is Vertex AI Pipelines, which orchestrates complex workflows as directed acyclic graphs (DAGs) composed of containerized components. This enables reproducible, auditable ML workflows tightly integrated with Google Cloud services like BigQuery for scalable data warehousing and Dataplex for unified data governance.
In practice, companies like Lowe’s leverage Vertex AI Search to dynamically generate product recommendations that respond to complex customer queries in real time. This demonstrates the platform’s capability to handle large-scale inference with sophisticated business logic and low latency.
Technical Specifications and Model Support
Vertex AI supports a wide array of model types across multiple modalities, including tabular data, vision, video, and text. This multimodal capability is powered in part by Google’s Gemini family of AI models. The latest, Gemini 2.5 Pro, offers advanced reasoning and multimodal understanding, processing text, code, PDFs, images, video, and audio. Developers can access these models through Vertex AI Studio and Firebase SDKs to embed cutting-edge generative AI features directly into applications.
For vision and video tasks, Vertex AI supports image classification, object detection, and action recognition, addressing use cases such as surveillance, media analytics, and quality control. Tabular data workflows—common in finance, healthcare, and manufacturing—benefit from AutoML’s automated model tuning, while custom training jobs allow data scientists to run distributed training using preferred frameworks.
Google Cloud’s data ecosystem integration is seamless. Whether datasets reside in BigQuery, Cloud Storage, or AlloyDB, Vertex AI pipelines access and orchestrate data effortlessly. This unified access reduces engineering overhead and accelerates experimentation in complex cloud environments.
Performance, Monitoring, and Enterprise Use Cases
Scalability and flexibility are meaningful only if model performance remains reliable over time. Vertex AI incorporates sophisticated automated monitoring tools, including Model Monitoring v2, which proactively detects model drift and feature skew by analyzing changes in feature attribution scores. Early detection of data distribution shifts is critical in regulated industries where model decisions require trustworthiness and auditability.
The platform also provides built-in evaluation metrics for traditional predictive models and generative AI models, enabling continuous assessment and tuning across diverse data modalities and business contexts.
From an enterprise standpoint, Vertex AI excels where data complexity and governance converge. Features like the Model Context Protocol (MCP) standardize secure access of AI models to data sources, supporting compliance and ethical AI practices. Moreover, Google Cloud’s Sovereign AI offerings address data sovereignty requirements for global organizations.
Home Depot’s deployment of agentic AI solutions on Vertex AI illustrates the platform’s versatility. Their conversational agents deliver 24/7 expert guidance by integrating product recommendations and how-to instructions, showcasing capabilities beyond conventional batch inference.
Weighing the Pros and Cons
Vertex AI’s unified platform reduces the friction of deploying and managing machine learning at scale. Its extensive model support and tight integration with Google Cloud’s ecosystem make it an attractive choice for enterprises seeking sophisticated AI capabilities without building infrastructure from scratch.
However, this deep integration entails a learning curve and creates dependence on Google’s cloud infrastructure. Enterprises with strict multi-cloud strategies or on-premises requirements may find this cloud-centric model limiting.
Cost is another consideration. Vertex AI employs consumption-based pricing that covers compute, storage, and model-specific usage such as Gemini API calls. Heavy real-time inference workloads can increase expenses, though access to next-generation GPUs and specialized VM types offers options to optimize the performance-cost balance.
Final Thoughts
Google Cloud’s Vertex AI represents the maturation of AutoML platforms into fully integrated MLOps ecosystems. It is a compelling choice for organizations ready to invest in a comprehensive AI development environment supporting diverse data types and complex deployment pipelines.
Its automated monitoring and governance features embody a sophisticated understanding of enterprise needs, balancing innovation with operational rigor. For use cases involving AI scale across multiple teams, modalities, and compliance regimes, Vertex AI provides a powerful foundation.
As with any platform, success depends on thoughtful implementation—prioritizing data quality, ethical considerations, and ongoing model stewardship to unlock its full potential.
Aspect | Details |
---|---|
Platform | Google Cloud Vertex AI |
Architecture | End-to-end managed service integrating AutoML, custom training, and MLOps pipelines |
Key Features | Vertex AI Pipelines (DAG workflows), integration with BigQuery and Dataplex, Vertex AI Search, Model Monitoring v2, Model Context Protocol (MCP), Sovereign AI offerings |
Model Support | Tabular data, vision, video, text, multimodal (Gemini 2.5 Pro model family) |
Use Cases | Product recommendations (Lowe’s), conversational agents (Home Depot), surveillance, media analytics, quality control, finance, healthcare, manufacturing |
Integration | Seamless with Google Cloud data ecosystem (BigQuery, Cloud Storage, AlloyDB) |
Monitoring & Performance | Automated model monitoring detecting drift and feature skew, continuous evaluation metrics for predictive and generative AI |
Pros | Unified platform, scalable, extensive model support, tight Google Cloud integration, advanced governance and compliance features |
Cons | Learning curve, dependency on Google Cloud infrastructure, may not suit strict multi-cloud or on-premises strategies, consumption-based pricing can be costly for heavy workloads |
Pricing Model | Consumption-based (compute, storage, Gemini API usage) |
Target Users | Enterprises seeking comprehensive AI environment with diverse data types and complex deployment pipelines |
2. DataRobot: Enterprise AI Automation for Predictive Modeling
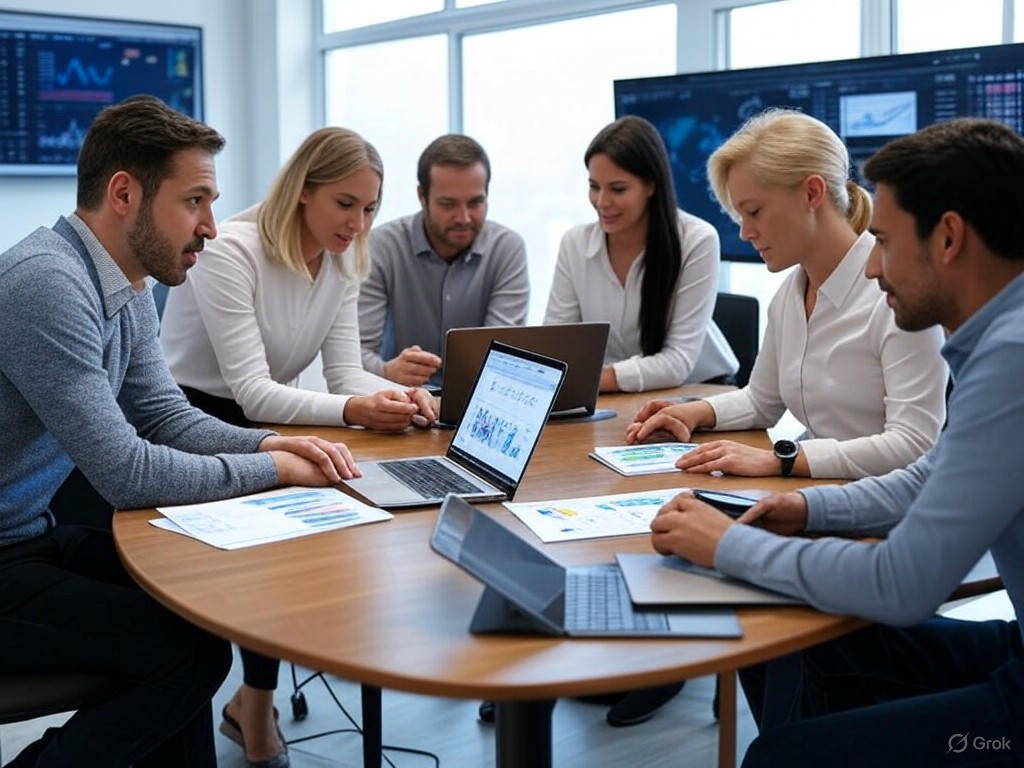
DataRobot: Enterprise AI Automation for Predictive Modeling
How can one platform effectively serve both seasoned data scientists and business users without sacrificing power or transparency? DataRobot offers a compelling solution with an end-to-end AI automation platform that balances technical sophistication and user accessibility.
Automated Feature Engineering, Model Selection, and Validation
Central to DataRobot’s platform is its automated feature engineering, transforming raw data into predictive insights with minimal manual effort. Its Feature Discovery capability intelligently generates hundreds of new features across multiple datasets—even those with varying granularities—while pruning redundant or low-impact features. This automation helps data scientists enrich their datasets automatically, uncovering subtle patterns that might otherwise remain hidden.
DataRobot supports hundreds of algorithms, spanning classical regression models, advanced ensembles, and deep learning techniques. Its Autopilot mode automates model selection by recommending the best candidate for deployment based on rigorous evaluation using K-fold cross-validation. The platform’s data partitioning strategy—dividing data into training, validation, and holdout sets—ensures models generalize well and performance estimates are reliable.
Importantly, this automation does not limit user control. DataRobot offers specialized workflows tailored for time series and frequency-severity models common in insurance and finance. It also handles mixed data types—including numeric, categorical, and image data—through featurizers that convert images into numeric representations, enabling multimodal modeling within a single project.
Explainability and Model Governance: Transparency at Scale
In regulated sectors such as finance and healthcare, AI explainability is essential. DataRobot meets these demands with robust tools centered on SHAP (Shapley Additive exPlanations). SHAP provides mathematically grounded, intuitive insights into how each feature influences individual predictions. These row-level explanations are generated efficiently, helping both technical teams and business stakeholders understand the rationale behind model decisions.
Complementing explainability, DataRobot’s governance features ensure models comply with internal policies and external regulations. This includes role-based access controls, detailed audit logs, change management, and traceability of prediction results. The platform can generate individualized compliance documentation aligned with frameworks such as SR 11-7 for banking model risk management, significantly reducing time-to-deployment in regulated environments.
By centralizing governance, DataRobot empowers organizations to scale AI safely across departments while maintaining transparency—critical when deploying AI in sensitive contexts where trust and accountability are paramount.
Deployment Options and Real-Time Prediction APIs
Enterprise flexibility demands diverse deployment modalities, and DataRobot delivers:
- Cloud: Rapid, serverless deployments that spin up in minutes, leveraging partnerships with providers like NVIDIA for scalable AI workloads.
- On-premises: For organizations with strict data residency or compliance needs, DataRobot supports self-managed installations integrated with Kubernetes infrastructures, including Nutanix’s GPT-in-a-Box solution.
- Hybrid: Combining on-premises and cloud resources, hybrid deployments enable sensitive data to remain on-premises while offloading compute-intensive tasks to the cloud.
Once deployed, DataRobot exposes real-time prediction APIs supporting JSON and CSV formats, facilitating seamless integration into production applications. These APIs offer instant predictions with features like drift detection and “humility” rules to flag uncertain outputs. Intelligent model caching optimizes response times and resource efficiency.
Use Cases: Finance, Healthcare, and Manufacturing
DataRobot’s versatility is evident across industries where compliance and transparency are critical:
-
Finance: Banks embed DataRobot in credit risk scoring and fraud detection pipelines. Explainability tools satisfy regulatory requirements for model interpretability, while governance frameworks support audit readiness.
-
Healthcare: Predictive models assist in patient risk stratification and treatment optimization. DataRobot’s capacity to handle diverse data types—including time series and images—enables richer modeling. Compliance documentation and traceability are crucial to meet HIPAA and privacy regulations.
-
Manufacturing: Predictive maintenance and quality control benefit from DataRobot’s automated feature engineering applied to sensor data, combined with real-time prediction APIs that trigger alerts to minimize downtime.
Despite these strengths, challenges remain. Automated feature engineering depends on up-to-date secondary datasets to maintain accuracy, complicating data pipeline management. Additionally, while SHAP explanations enhance transparency, their computational cost and complexity can be significant, especially for high-dimensional data.
In summary, DataRobot delivers a powerful combination of automation, explainability, and governance tailored to both enterprise AI teams and business users. Its extensive algorithm support and flexible deployment options make it a strong candidate for organizations seeking scalable, compliant AI solutions. However, users must be mindful of maintaining data freshness and navigating the complexities of interpreting detailed model explanations at scale. DataRobot exemplifies how AutoML can move beyond hype by embedding scientific rigor and ethical guardrails throughout the AI lifecycle.
Category | Details |
---|---|
Automated Feature Engineering | Feature Discovery generates hundreds of features across datasets; prunes redundant/low-impact features; supports mixed data types including numeric, categorical, and images via featurizers. |
Model Selection & Validation | Supports hundreds of algorithms (regression, ensembles, deep learning); Autopilot mode recommends best models using K-fold cross-validation; data partitioning into training, validation, holdout sets. |
Explainability & Governance | Uses SHAP for feature impact explanations; role-based access control; audit logs; compliance documentation aligned with regulations like SR 11-7; change management and traceability. |
Deployment Options | Cloud (serverless, NVIDIA partnerships); On-premises (Kubernetes, Nutanix GPT-in-a-Box); Hybrid (combines on-premises and cloud resources). |
Real-Time Prediction APIs | Supports JSON and CSV formats; features drift detection, humility rules, and intelligent model caching for efficient predictions. |
Use Cases | Finance: credit risk scoring, fraud detection with regulatory compliance. Healthcare: patient risk stratification, treatment optimization with HIPAA compliance. Manufacturing: predictive maintenance and quality control using sensor data and real-time alerts. |
Challenges | Requires up-to-date secondary datasets for feature engineering; SHAP explanations can be computationally intensive for high-dimensional data. |
3. Microsoft Azure Machine Learning: Robust Cloud-Native AutoML with MLOps Integration
Microsoft Azure Machine Learning: Robust Cloud-Native AutoML with MLOps Integration
Microsoft’s Azure Machine Learning (Azure ML) distinguishes itself as a cloud-native AutoML platform deeply integrated with a mature MLOps ecosystem. This synergy empowers organizations to accelerate model development while ensuring rigorous lifecycle management, scalability, and adherence to responsible AI principles.
AutoML Capabilities Enhanced by MLOps Integration
Azure ML’s AutoML automates repetitive and time-consuming tasks such as algorithm selection and hyperparameter tuning. It executes parallel pipelines that explore a variety of algorithms and parameter combinations across problem domains including time-series forecasting, image classification, and natural language processing (NLP). Ensemble models are enabled by default to enhance predictive accuracy. The platform uses Logistic Regression as the default meta-model for classification and ElasticNet for regression, while offering advanced users the flexibility to customize featurization and experiment parameters through its Python SDK.
What sets Azure ML apart is how seamlessly AutoML is woven into its MLOps framework:
- Pipelines automate and orchestrate end-to-end workflows encompassing data preparation, training, evaluation, and deployment. These pipelines can be defined programmatically using the Python SDK or configured via the Azure ML studio’s UI.
- Experiment Tracking captures comprehensive metrics, parameters, and artifacts across runs, supported by native MLflow autologging for streamlined metric collection and comparison.
- Model Versioning and Registry maintain a centralized repository where every trained model is registered, versioned, and tracked within the workspace, facilitating consistent deployment and governance.
This tight integration enhances reproducibility and collaboration, which are critical in enterprise-grade AI environments. For example, a retail company can use Azure ML AutoML to develop forecasting models for inventory management and then deploy and monitor these models reliably through Azure ML’s MLOps infrastructure.
Developer Experience and Ecosystem Compatibility
Azure ML prioritizes a rich developer experience centered around its Python SDK v2 and integrated Jupyter notebooks hosted within the Azure ML studio. The SDK v2 introduces consistent feature naming and command-line tooling, simplifying CI/CD pipeline creation and experimentation workflows. Interactive notebooks enable data scientists to iterate rapidly within the browser, supporting collaboration through features like inline comments and export options.
Azure ML’s ecosystem extends integration beyond model development into a comprehensive data platform:
- Azure Synapse Analytics integration enables scalable data wrangling and analytics by connecting ML workspaces to Synapse and leveraging Apache Spark pools directly from notebooks.
- Data Services Compatibility allows pipelines to consume versioned datasets registered across Azure Blob Storage, Data Lake, and other Azure storage services, ensuring traceability throughout the ML lifecycle.
- Python Environment Management is streamlined with pre-configured VM images like Data Science VMs, which come pre-installed with popular ML frameworks alongside the Azure ML SDK.
This interconnected environment supports end-to-end workflows—from raw data ingestion and feature engineering to model training and secure deployment—all within a unified, cloud-native platform.
Scalability: Distributed Training and GPU Acceleration
Azure ML supports the computational demands of large-scale model training through robust infrastructure and distributed training capabilities:
- ND-Series Virtual Machines offer cutting-edge NVIDIA A100 and H100 Tensor Core GPUs, connected via InfiniBand networking to provide high bandwidth and low latency essential for parallel deep learning workloads.
- Distributed Training Frameworks include support for PyTorch’s DistributedDataParallel, TensorFlow’s native distribution strategies, and first-class integration with DeepSpeed, enabling near-linear scalability for massive models.
- Flexible Compute Targets allow users to provision GPU-enabled VMs on demand, optimizing cost-performance ratios by scaling compute resources up or down as needed.
This powerful infrastructure enables complex workloads such as computer vision models for retail shelf monitoring and IoT sensor data analysis in manufacturing environments.
Responsible AI and Ethical AI Toolkits
Addressing the growing importance of ethical AI, Azure ML embeds responsible AI functionalities directly into its platform:
- Fairness and Explainability Toolkits provide capabilities for assessing model fairness, identifying potential biases, and interpreting model decisions to promote transparency.
- Security and Compliance are ensured through Azure’s adherence to global standards like GDPR and PCI-DSS, which is particularly vital for regulated industries deploying AI solutions.
- Responsible AI Workflows and Templates guide developers to embed ethical considerations throughout the ML lifecycle—from data preprocessing through deployment—supporting accountable, transparent AI practices.
For instance, a retailer deploying customer segmentation models can leverage these toolkits to prevent discrimination based on protected attributes, thereby maintaining regulatory compliance and public trust.
Use Cases: Retail and Industrial IoT Deployments
Azure ML’s blend of scalable AutoML, strong MLOps capabilities, and embedded responsible AI tooling suits scenarios demanding secure, compliant, and high-throughput AI solutions:
- Retail: By combining Azure IoT with AutoML, retailers enable smart experiences such as real-time inventory forecasting, personalized promotions, and automated checkout. The platform’s scalability supports extensive IoT device networks and continuous model updates managed through MLOps.
- Industrial IoT: Manufacturers utilize Azure ML to analyze sensor data for predictive maintenance, anomaly detection, and quality control. Distributed training capabilities accommodate high-dimensional sensor inputs, while security and compliance features safeguard sensitive operational data.
These examples highlight Azure ML’s suitability as an enterprise-grade AI platform balancing cutting-edge innovation with operational rigor and ethical safeguards.
In summary, Microsoft Azure Machine Learning’s cloud-native AutoML platform excels through its comprehensive MLOps integration, scalable training infrastructure, and built-in responsible AI capabilities. Organizations prioritizing operational excellence, compliance, and scalability—especially in retail and IoT sectors—will find Azure ML a compelling and well-supported choice. Its deep ecosystem compatibility and developer-friendly tools enable rapid progression from experimentation to production-grade AI with confidence.
Category | Details |
---|---|
AutoML Capabilities | Automates algorithm selection, hyperparameter tuning; supports time-series forecasting, image classification, NLP; default meta-models: Logistic Regression (classification), ElasticNet (regression); ensemble models enabled; customizable via Python SDK |
MLOps Integration | Pipelines for workflow automation; experiment tracking with MLflow autologging; model versioning and registry for governance and reproducibility |
Developer Experience | Python SDK v2 with consistent feature naming; integrated Jupyter notebooks; supports CI/CD pipeline creation; collaboration features like inline comments and export |
Ecosystem Compatibility | Integration with Azure Synapse Analytics and Apache Spark; supports Azure Blob Storage, Data Lake datasets; pre-configured Data Science VMs with ML frameworks |
Scalability | ND-Series VMs with NVIDIA A100 & H100 GPUs; InfiniBand networking; supports DistributedDataParallel, TensorFlow distribution, DeepSpeed; flexible GPU compute provisioning |
Responsible AI Features | Fairness and explainability toolkits; compliance with GDPR, PCI-DSS; responsible AI workflows and templates |
Use Cases | Retail: real-time inventory forecasting, personalized promotions, automated checkout; Industrial IoT: predictive maintenance, anomaly detection, quality control |
4. Alteryx Intelligence Suite: Democratizing AI with No-Code and Low-Code Automation
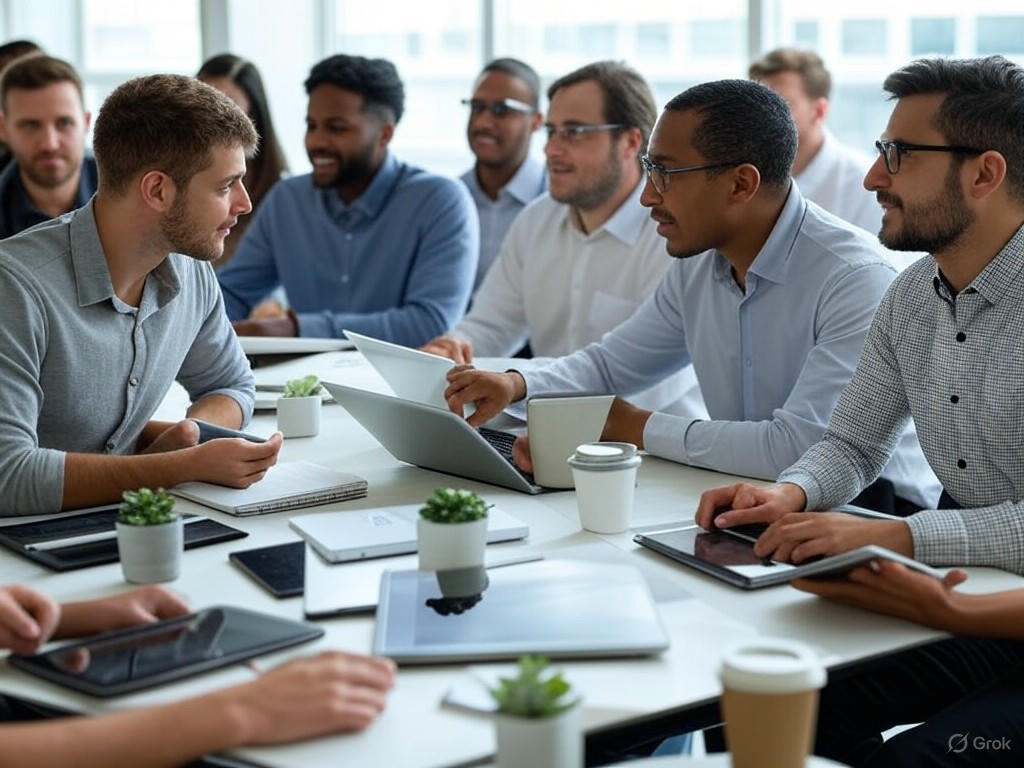
Alteryx Intelligence Suite: Democratizing AI with No-Code and Low-Code Automation
How can organizations empower business analysts and SMEs to leverage machine learning without turning every project into a developer-intensive effort? Alteryx Intelligence Suite addresses this challenge by encapsulating complex analytics within an intuitive no-code and low-code environment. This approach accelerates time-to-insight for users beyond traditional data science roles.
User-Friendly Interface Empowering Non-Technical Users
Alteryx Intelligence Suite is fundamentally designed to lower barriers to advanced analytics. Its drag-and-drop, visual interface enables users to prepare, blend, and analyze data without writing Python or R code—though scripting options remain available for those who want greater control. The platform’s guided workflows walk users through statistical analyses and machine learning model building step-by-step, making it accessible to business analysts and subject matter experts.
Beyond structured data, Alteryx extends its capabilities with integrated text mining and computer vision features. Using OCR and natural language processing, it unlocks insights from unstructured sources such as PDFs, images, and customer feedback. For instance, marketing teams can extract sentiment trends from customer reviews rapidly, enabling fast ideation and experimentation without relying on data scientists.
Technical Capabilities: Data Connectors, Automated Feature Engineering, and Visual Workflow Design
Under the hood, Alteryx supports a wide array of data connectors, seamlessly integrating with sources like Google Analytics 4, Microsoft Power BI, SharePoint, and OneDrive. This connectivity simplifies data ingestion, allowing users to blend diverse datasets within a single, coherent workflow.
Automated feature engineering is a standout technical strength. By generating features based on predefined data relationships and types, tools like New Feature Types and Build Features reduce the need for manual trial-and-error. This automation expedites model iteration cycles by enriching predictive models with meaningful variables without requiring deep statistical expertise.
Visual workflow design is a hallmark of the platform. Users build analytical pipelines by linking tools on a canvas, instantly visualizing data flows and transformations. This transparency clarifies complex processes and facilitates collaboration, reproducibility, and maintenance across organizational teams.
Strengths: Accelerating Time-to-Insight for Business Analysts and SMEs
Alteryx excels at democratizing AI by enabling rapid experimentation and AI adoption driven by business needs. Its no-code and low-code environment empowers analysts to transcend spreadsheet limitations and manual reporting. This agility allows prototyping machine learning models in hours instead of weeks.
Use cases such as customer segmentation and marketing analytics showcase this strength. Organizations can swiftly generate customer segments based on purchasing behavior or sentiment analysis and apply insights to targeted campaigns without bottlenecks. For example, a retail client might identify underperforming SKUs to optimize inventory, achieving millions in cost savings.
Additionally, Alteryx’s AI-guided workflows and Education Mode support user skill development, promoting data literacy alongside immediate business impact. According to a 2025 survey by Alteryx, 7 in 10 analysts report increased effectiveness when using AI and automation tools like this, highlighting a shift toward strategic, value-added roles rather than concerns about displacement.
Limitations: Complex Model Customization and Scalability Challenges
Despite its accessibility, Alteryx Intelligence Suite is not a universal solution for all data science challenges. Its emphasis on no-code and assisted modeling limits flexibility for complex model customization and cutting-edge algorithm experimentation. Power users requiring full control over hyperparameters or custom architectures may find the platform restrictive.
Scalability is another consideration. While Alteryx supports deployment and sharing at scale, its capabilities may fall short for enterprise-grade demands involving massive parallel processing or real-time streaming analytics. Competitors offering deeper big data integrations and distributed computing frameworks might better serve such needs.
Frequent product updates reflect active development but can introduce a learning curve for teams striving to keep pace with evolving features and versions.
Real-World Impact: Marketing Analytics and Customer Segmentation
Alteryx Intelligence Suite’s core value lies in business-driven AI adoption where speed and usability outweigh maximal algorithmic complexity. Marketing analytics teams benefit from rapid experimentation with blended customer data, combining demographic, behavioral, and sentiment signals to personalize outreach effectively.
In customer segmentation projects, automated feature engineering and visual workflows enable rapid iteration through different segment definitions and predictive models. This fosters a test-and-learn culture closely aligned with business objectives.
Prominent companies such as Amway, Chick-fil-A, and The Coca-Cola Company leverage Alteryx to automate data workflows, reduce manual effort, and accelerate insight delivery, underlining the platform’s practical impact across industries.
In summary, Alteryx Intelligence Suite stands out by democratizing AI through its no-code and low-code automation capabilities. It streamlines data preparation, feature engineering, and model building workflows to deliver faster business insights, particularly for business analysts and SMEs. However, organizations should carefully weigh its ease of use against limitations in advanced customization and scalability when selecting an AutoML platform aligned with their operational and technical requirements.
Aspect | Details |
---|---|
Platform Focus | Democratizing AI with no-code and low-code automation for business analysts and SMEs |
User Interface | Drag-and-drop visual interface with guided workflows; scripting options available |
Data Capabilities | Supports structured and unstructured data with text mining, OCR, NLP, and computer vision |
Data Connectors | Google Analytics 4, Microsoft Power BI, SharePoint, OneDrive, and more |
Automated Feature Engineering | Tools like New Feature Types and Build Features to generate meaningful variables automatically |
Workflow Design | Visual canvas for building analytical pipelines with transparent data flows and transformations |
Strengths | Accelerates time-to-insight, enables rapid ML prototyping, supports marketing analytics and customer segmentation, promotes data literacy |
Limitations | Limited complex model customization, scalability challenges for enterprise-grade big data and real-time analytics, frequent updates can introduce learning curve |
Use Cases | Customer segmentation, marketing analytics, inventory optimization, sentiment analysis from unstructured data |
Notable Clients | Amway, Chick-fil-A, The Coca-Cola Company |
Impact | Enables business-driven AI adoption with rapid experimentation and insight delivery |
5. IBM Watson Studio AutoAI: Hybrid Cloud AI with Emphasis on Model Explainability and Compliance
5. IBM Watson Studio AutoAI: Hybrid Cloud AI with Emphasis on Model Explainability and Compliance
What happens when automated machine learning meets the rigorous demands of highly regulated industries? IBM Watson Studio’s AutoAI provides a compelling solution by combining powerful end-to-end automation with a strong focus on explainability, bias detection, and compliance—essential pillars for sectors such as healthcare and financial services.
Core Automation Features: Comprehensive Pipeline Automation and Framework Support
AutoAI goes far beyond simple model building. It automates the entire machine learning pipeline—from raw data preprocessing and feature engineering to algorithm selection and hyperparameter optimization. Users simply input their datasets, and AutoAI generates multiple candidate models optimized on user-selected metrics like accuracy, F1 score, or error rates.
Notably, AutoAI supports multiple popular AI frameworks, including TensorFlow and PyTorch. This flexibility allows data science teams to work within familiar ecosystems and extend generated pipelines with custom code when needed.
Integration with IBM Cloud Pak for Data creates a unified hybrid cloud environment where data ingestion, transformation, and model deployment operate seamlessly together. Organizations can securely connect to on-premises, private cloud, or public cloud data sources, preserving data sovereignty while leveraging cloud scalability.
Another significant advantage is AutoAI’s ability to export the entire generated pipeline as Python code formatted within Jupyter notebooks. This feature accelerates prototyping and enables data scientists to customize or extend workflows, effectively avoiding the “black-box” automation trap that can hinder transparency and control.
Explainability, Bias Detection, and Audit Trails: Built for Ethical and Compliant AI
Watson Studio AutoAI stands out for its deep commitment to transparency, fairness, and governance—critical requirements as AI increasingly impacts sensitive decisions.
The platform includes robust built-in tools for model explainability, offering detailed insights into feature importance and decision-making logic. For example, in healthcare diagnostics, clinicians rely on explanations to understand why a model flagged a patient as high risk, fostering trust and supporting patient safety.
Simultaneously, AutoAI incorporates bias detection mechanisms that identify potential unfairness or data imbalances that could skew outcomes. In financial services, this helps prevent discriminatory practices in credit scoring or fraud detection, ensuring models meet ethical and regulatory standards.
Crucially, Watson Studio maintains comprehensive audit trails documenting every step of the model lifecycle—from data ingestion through training to deployment. This traceability supports compliance with regulations like GDPR and HIPAA, providing defensible records for audits and governance.
Use Cases: Addressing Compliance and Transparency in High-Stakes Industries
Watson Studio AutoAI’s strengths are particularly evident in industries where regulatory compliance and model transparency are non-negotiable.
-
Healthcare Diagnostics: AutoAI enables rapid development of predictive models for patient risk stratification and anomaly detection. Its explainability features ensure clinicians and stakeholders can interpret and trust AI-driven recommendations, balancing innovation with patient safety.
-
Financial Services: The platform automates credit risk scoring and fraud detection workflows while embedding bias detection and auditability. This helps financial institutions accelerate model deployment cycles without compromising regulatory oversight.
Advanced Pipeline Generation and Hybrid Cloud Integration
Under the hood, AutoAI’s pipeline automation is sophisticated and versatile. Instead of producing a single model, it generates multiple pipelines combining different algorithms and preprocessing steps. Each pipeline is optimized through extensive hyperparameter tuning and validated using techniques like K-fold cross-validation. Users can compare pipelines side-by-side and select the best performer or create ensemble models by blending top candidates.
Integration with IBM Cloud Pak for Data amplifies these capabilities by providing a unified data fabric and governance layer across hybrid environments. Teams can securely access data wherever it resides, orchestrate AI workflows, and collaborate effectively across distributed or cross-functional groups.
Final Thoughts
IBM Watson Studio AutoAI exemplifies how AutoML has evolved beyond convenience tools into robust platforms designed for complex, high-stakes environments. By combining comprehensive automation with explainability, bias mitigation, and compliance within a hybrid cloud framework, it addresses the critical challenges many enterprises face today.
That said, no platform is a silver bullet. Watson Studio’s real strength lies in accelerating model development while embedding controls that help ensure ethical standards and regulatory requirements are met. For organizations in regulated sectors, this balance between agility and governance can be a decisive factor in their AI strategy.
Aspect | Details |
---|---|
Core Automation Features | End-to-end ML pipeline automation including data preprocessing, feature engineering, algorithm selection, hyperparameter optimization; supports TensorFlow and PyTorch; exports pipelines as Python code in Jupyter notebooks; integrates with IBM Cloud Pak for Data hybrid cloud environment. |
Explainability & Bias Detection | Built-in tools for model explainability (feature importance, decision logic); bias detection mechanisms to identify unfairness or data imbalances; comprehensive audit trails for model lifecycle documentation supporting GDPR and HIPAA compliance. |
Use Cases | Healthcare Diagnostics: Predictive models with explainability for trust and safety. Financial Services: Automated credit risk scoring and fraud detection with bias detection and auditability. |
Advanced Pipeline Generation | Generates multiple optimized pipelines with hyperparameter tuning and K-fold cross-validation; supports pipeline comparison and ensemble modeling. |
Hybrid Cloud Integration | Unified data fabric and governance across hybrid environments via IBM Cloud Pak for Data; secure data access across on-premises, private, and public clouds; supports collaboration across distributed teams. |
Key Strengths | Combines automation with explainability, bias mitigation, and compliance; designed for regulated industries requiring ethical AI and regulatory adherence; accelerates model development while embedding governance controls. |