AI and SEO in 2024: How Google’s AI Transforms Content Ranking
- Introduction: The AI Revolution in Search Engines and Why It Matters for SEO
- Introduction: The AI Revolution in Search Engines and Why It Matters for SEO
- Google’s Latest AI Capabilities: Transforming Search at the Core
- Decoding User Intent with AI: The New Frontier for SEO
- The Rise of Zero-Click Searches: Rethinking Visibility and Engagement
- Why This Matters Now: Navigating the AI-Driven SEO Landscape
- Foundations of Google’s AI-Driven Search Algorithms: From BERT to MUM and Beyond
- Foundations of Google’s AI-Driven Search Algorithms: From BERT to MUM and Beyond
- BERT: Unlocking Contextual Language Understanding
- MUM: A Leap Toward Multimodal and Multilingual Comprehension
- The Transformer Architecture: The Engine Under the Hood
- From Keywords to Intent: The New Paradigm in Search Ranking
- Illustrating AI’s Handling of Ambiguous or Novel Queries
- Decoding Google’s Ranking Signals in the AI Era: Expertise, Trustworthiness, and Context
- Decoding Google’s Ranking Signals in the AI Era: Expertise, Trustworthiness, and Context
- The Rising Importance of the E-E-A-T Framework
- Beyond Keywords: AI’s Sophisticated Content Evaluation
- Technical SEO: The Hidden Infrastructure Supporting AI’s Judgments
- Personalization and Context: How AI Tailors Search Results
- Balancing Innovation with Ethical SEO Practices
- Technical Deep Dive: How AI Algorithms Integrate Semantic Search, Vector Ranking, and Real-Time Processing
- Technical Deep Dive: How AI Algorithms Integrate Semantic Search, Vector Ranking, and Real-Time Processing
- Semantic Search and Vector Embeddings: From Keywords to Meaning
- Real-Time Processing Architecture: Scaling AI at Web Scale
- Hybrid Ranking: Merging Traditional IR and Deep Learning
- Challenges: Scalability, Interpretability, and Fairness
- Bringing It All Together
- SEO Strategies for Thriving in Google’s AI-Powered Search Landscape
- SEO Strategies for Thriving in Google’s AI-Powered Search Landscape
- Creating Content That Resonates with AI and Humans Alike
- Capturing Featured Snippets, Voice Search, and Zero-Click Opportunities
- Technical SEO: Foundations for AI-Friendly Crawling and User Experience
- Ethical Use of AI in Content Creation: Balancing Efficiency with Authenticity
- Real-World Adaptations: Lessons from SEO Leaders
- Comparative Analysis: Google’s AI Search Versus Competitors and Emerging Models
- Comparative Analysis: Google’s AI Search Versus Competitors and Emerging Models
- Benchmarking AI Architectures and Ranking Algorithms
- Differences in User Interaction Models
- Competitive Dynamics and SEO Implications
- Maintaining Google’s Competitive Edge: What It Means for Stakeholders
- Future Trajectories: Anticipating the Evolution of AI in Search and Its Broader Societal Impact
- Future Trajectories: Anticipating the Evolution of AI in Search and Its Broader Societal Impact
- Toward Greater Contextual Awareness and Multimodal Search Integration
- Explainability and Transparency: Demystifying AI Ranking Decisions
- Personalization Balanced with Privacy Concerns
- Societal Implications: Navigating Information Reliability and Democratization
- Strategic Recommendations for Businesses and SEO Professionals
- Conclusion
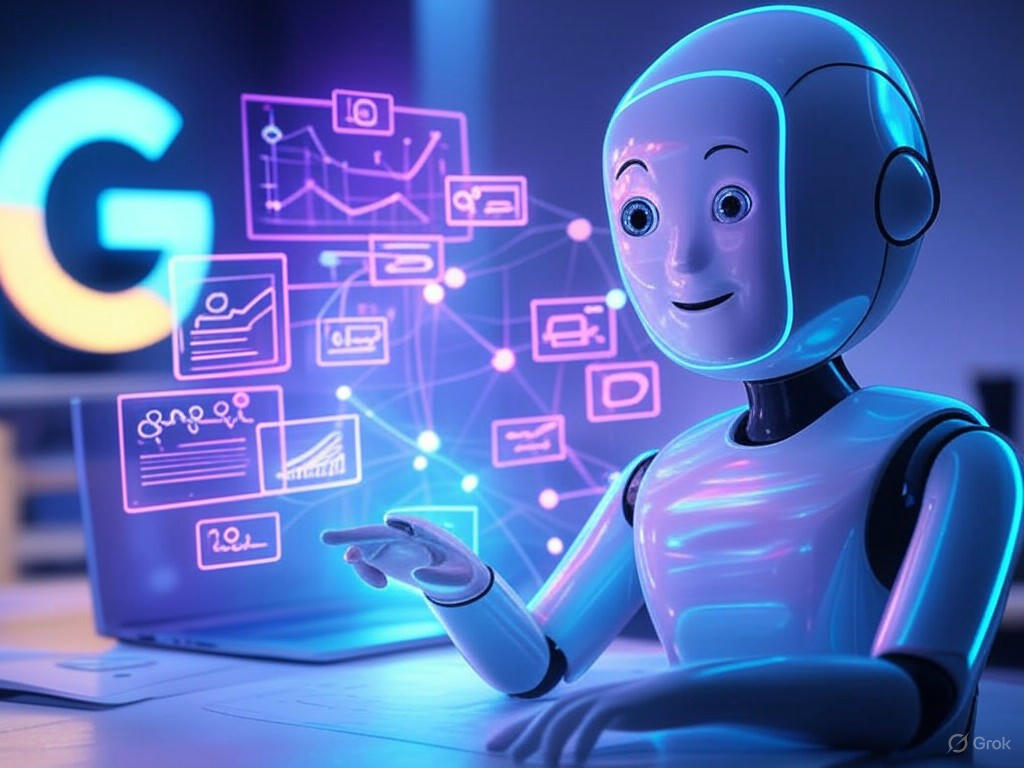
Introduction: The AI Revolution in Search Engines and Why It Matters for SEO
Introduction: The AI Revolution in Search Engines and Why It Matters for SEO
Imagine searching online shifting from typing isolated keywords to engaging in a natural conversation with an intelligent assistant. This is no longer futuristic speculation but the unfolding reality of 2025, propelled by rapid advancements in artificial intelligence—especially within Google’s search engine. Over the past year, Google has dramatically accelerated integrating AI capabilities throughout its search ecosystem, fundamentally transforming how content is ranked, discovered, and consumed.
Google’s Latest AI Capabilities: Transforming Search at the Core
In April 2025, Google announced ambitious plans to embed AI deeply across sectors, with search as a primary focus. Features like AI Mode in Google Search Labs empower users to ask complex, conversational questions and receive nuanced, context-aware answers instantly. According to Semrush data, 13.14% of desktop searches in the U.S. now trigger AI Overviews—an impressive increase from 6.49% just two months earlier.
These AI Overviews summarize the most relevant information directly on the search results page, often reducing the need for users to click through to individual websites. This transformation is driven by advanced natural language understanding models that go far beyond simple keyword matching.
Google’s AI builds on pioneering technologies such as RankBrain, BERT, and the latest Gemini series (Gemini 2.5 Pro), interpreting user intent with remarkable precision. Importantly, these systems evaluate not only content relevance but also its expertise, experience, authority, and trustworthiness—collectively known as E-E-A-T—a framework emphasized even more in Google’s 2025 core algorithm updates.
For SEO professionals, this marks a paradigm shift. Traditional practices like keyword stuffing or focusing solely on traffic volume no longer suffice. Instead, it’s essential to craft genuinely valuable, authoritative content that aligns closely with user intent. Google’s AI doesn’t just crawl and index pages—it “reads” and synthesizes content to deliver the most comprehensive, trustworthy answers possible.
Decoding User Intent with AI: The New Frontier for SEO
How precisely can a search engine understand what a user truly wants? Thanks to leaps in natural language processing (NLP), Google now excels at interpreting the subtle context behind queries. Whether a user has informational, navigational, transactional, or commercial intent, AI-driven algorithms tailor results accordingly.
Think of Google’s AI as a skilled librarian who not only hears your question but anticipates your underlying needs. For example, a search like “best noise-cancelling earbuds for travel” signals transactional intent—seeking purchase advice—not just informational content about earbuds. This nuanced understanding pushes SEO beyond simple keyword targeting toward a holistic grasp of user goals.
Moreover, conversational AI enables users to phrase queries naturally—through long-tail questions, voice commands, or follow-ups that evolve the search context. To adapt, SEO strategies must:
- Emphasize content that addresses specific questions and pain points
- Integrate conversational keywords and natural language phrases
- Prioritize experience and authority signals to meet Google’s heightened E-E-A-T standards
Industry leaders such as Bloomreach and Creaitor.ai highlight that leveraging AI to decode search intent is no longer optional—it is essential to produce content that resonates and ranks well in 2025’s AI-driven search landscape.
The Rise of Zero-Click Searches: Rethinking Visibility and Engagement
AI-powered search has also fueled the rise of zero-click searches, where users find their answers directly on the search results page without clicking through to any website. Currently, over 58% of U.S. Google searches end this way.
Google delivers these frictionless experiences via featured snippets, knowledge panels, and AI Overviews, benefiting users but challenging SEO professionals and content creators. The traditional organic traffic metric is losing relevance, shifting the focus to visibility within zero-click environments—a field sometimes called Answer Engine Optimization (AEO).
Key implications for SEO include:
- Structured data and schema markup become critical for helping Google understand and present your content in enhanced formats.
- Content must provide concise, authoritative answers that AI models prefer to showcase.
- Brand visibility shifts from clicks to owning the answer itself, necessitating strategic emphasis on reputation and trust signals.
SEO professionals must balance zero-click strategies with classic SEO fundamentals, combining technical optimization with compelling, user-centric content.
As SEO expert David William Jenkin notes, zero-click searches compel a rethink of the entire content funnel—from discovery to engagement—while Google’s AI increasingly “owns” the immediate answer space.
Why This Matters Now: Navigating the AI-Driven SEO Landscape
The convergence of Google’s AI advancements, enhanced natural language understanding, and evolving search result formats means SEO in 2025 is not merely an extension of past practices—it is a fundamental transformation.
To stay competitive, content creators and SEO professionals should:
- Stay abreast of Google’s AI rollout timelines and critical algorithm updates, such as the March 2025 core update
- Embrace AI-powered tools to analyze user intent, optimize content structure, and monitor zero-click search performance
- Prioritize quality, expertise, and genuine user value over shortcuts or superficial tactics
- Adapt to emerging engagement metrics beyond clicks, including brand visibility and SERP ownership
The stakes are high. As Google’s AI models become more sophisticated, they will increasingly curate and synthesize information autonomously. SEO’s future lies in genuinely serving users’ evolving needs within a complex, AI-driven search ecosystem.
In the sections ahead, we will explore these themes in depth—examining how Google’s AI functions behind the scenes, its impact on interpreting search intent, and practical strategies SEO professionals can adopt to thrive amid the AI revolution in search.
Topic | Details |
---|---|
Google’s AI Features | AI Mode in Google Search Labs, AI Overviews summarizing relevant info on SERPs |
Search Trigger Stats | 13.14% of desktop searches trigger AI Overviews (up from 6.49% two months prior) |
Core Technologies | RankBrain, BERT, Gemini 2.5 Pro |
SEO Focus Shift | From keyword stuffing to valuable, authoritative content aligned with user intent and E-E-A-T |
User Intent Types | Informational, Navigational, Transactional, Commercial |
SEO Strategy Adaptations | Emphasize specific questions, conversational keywords, E-E-A-T signals, and experience |
Zero-Click Search Stats | Over 58% of U.S. Google searches end without clicks |
Zero-Click SEO Implications | Use structured data, provide concise authoritative answers, focus on brand visibility and trust |
Recommended SEO Actions | Monitor Google AI updates, use AI tools for intent analysis, prioritize quality and engagement metrics |
Foundations of Google’s AI-Driven Search Algorithms: From BERT to MUM and Beyond
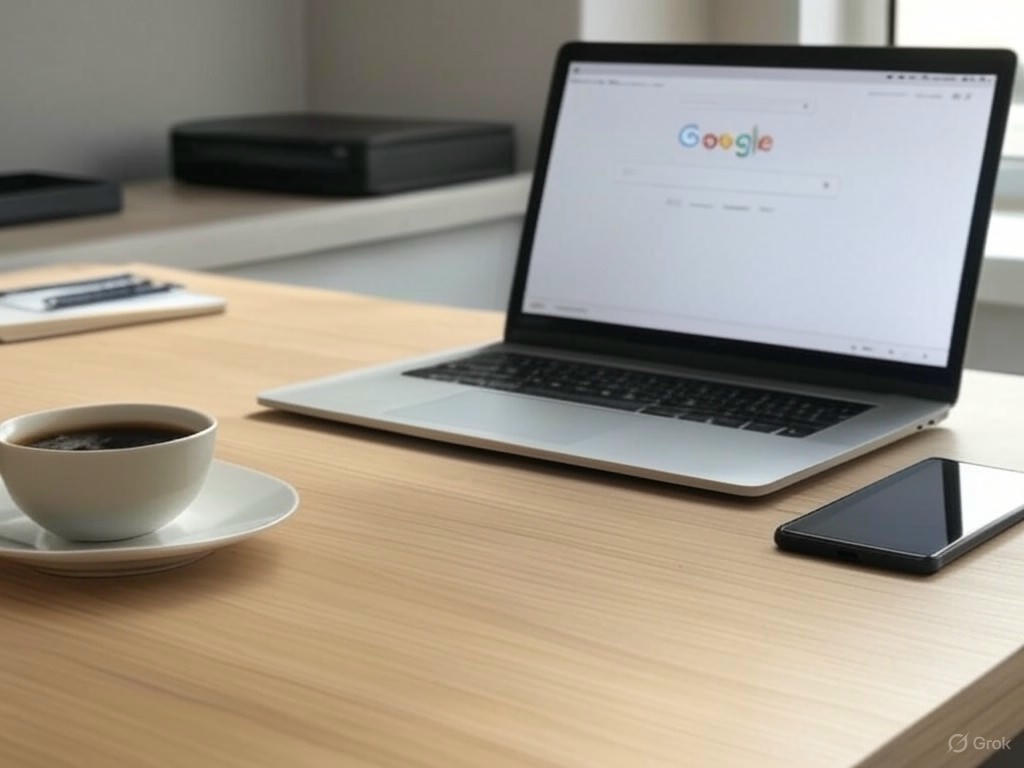
Foundations of Google’s AI-Driven Search Algorithms: From BERT to MUM and Beyond
What happens when a search engine learns to understand language as humans do? Google’s evolution from simple keyword matching to deep contextual comprehension is a transformative journey powered by advanced AI models like BERT and MUM, all built on the groundbreaking transformer architecture. These innovations have fundamentally reshaped how search interprets queries, ranks content, and delivers answers—pushing SEO strategies away from keyword stuffing toward semantic precision and genuine user intent.
BERT: Unlocking Contextual Language Understanding
Before BERT, Google’s search primarily matched keywords in queries to words on pages, often missing the subtlety of human language. Introduced in late 2019, BERT (Bidirectional Encoder Representations from Transformers) marked a breakthrough by processing words in both directions simultaneously.
Unlike earlier models that read text left-to-right or right-to-left, BERT’s bidirectional approach allows it to grasp the full context of a sentence, capturing nuances invisible to previous algorithms. For example, consider the query:
“Can you get medicine for someone pharmacy”
Traditional keyword-based search would focus on individual keywords like “medicine,” “someone,” and “pharmacy” without understanding the implied meaning of picking up medicine on behalf of someone else. BERT interprets the query holistically, improving relevance by understanding relationships between words.
Google reports that BERT improves understanding for roughly 1 in 10 English searches in the U.S., supporting over 70 languages worldwide. For SEO professionals, this signals a clear shift: content must be clear, naturally phrased, and focused on addressing real user intent rather than mechanical keyword optimization.
MUM: A Leap Toward Multimodal and Multilingual Comprehension
If BERT revolutionized contextual language understanding, MUM (Multitask Unified Model), announced in 2021, represents a quantum leap. MUM is reportedly 1,000 times more powerful than BERT and can understand and generate information across 75 languages and multiple modalities—including text, images, and potentially audio and video.
Imagine a complex, real-world query like:
“I hiked Mount Adams last year and want to hike Mount Fuji next fall. What should I know differently about weather, permits, and gear?”
MUM can synthesize information from diverse formats and languages to provide a comprehensive, nuanced answer. It can also integrate images you upload alongside textual queries, creating a search experience more akin to consulting a human expert.
This multimodal capability marks a departure from traditional SEO tactics. Keyword optimization alone is no longer sufficient. Content must be truly relevant, authoritative, and capable of satisfying complex user needs across multiple contexts and data types. MUM’s multilingual reach also amplifies the impact of localized, high-quality content, enabling Google to serve users worldwide with greater precision.
The Transformer Architecture: The Engine Under the Hood
Both BERT and MUM owe their breakthroughs to the transformer architecture introduced by Vaswani et al. in 2017. Unlike recurrent neural networks (RNNs) or convolutional models that process data sequentially or locally, transformers leverage a self-attention mechanism. This enables the model to weigh the importance of each word relative to others in a sentence or passage—regardless of position.
Transformers are computationally efficient and scale well, critical for handling billions of search queries with millisecond latency. They excel at capturing long-range dependencies in text, delivering a richer, more nuanced understanding of language than earlier models.
Google continues to innovate on this foundation with developments like “Titans,” which integrate neural memory modules to extend context retention and reasoning capabilities. This advancement supports complex, multi-turn interactions and personalized search experiences, pushing the boundaries of AI’s role in search.
From Keywords to Intent: The New Paradigm in Search Ranking
How do these AI models transform Google’s ranking of content? Traditional SEO heavily emphasized keyword placement in titles, headers, and body text to signal relevance. Today, BERT, MUM, and their transformer backbone prioritize semantic comprehension and user intent.
Google no longer “counts” keywords mechanically. Instead, it seeks content that genuinely aligns with the meaning behind a query. For instance, ambiguous or novel queries such as:
“best ways to reduce carbon footprint at home”
are now met with results that understand the underlying intent: practical, actionable advice rather than pages stuffed with related keywords.
In real-time ranking decisions, AI models analyze multiple signals, including:
- The contextual match between query and content
- Multimodal cues such as images, videos, and text
- Language nuances and syntactic structure
- User engagement patterns indicating satisfaction
This shift challenges SEO professionals to produce content that is clear, logically structured, and addresses the full scope of user questions. Expertise and trustworthiness play an increasingly important role, as Google’s AI looks for credible sources to feature in AI-generated overviews and featured snippets.
Illustrating AI’s Handling of Ambiguous or Novel Queries
Consider the query:
“Can I use an image of the Eiffel Tower to promote my travel blog?”
This is a multifaceted question involving legal, cultural, and marketing considerations. Earlier keyword-based systems might return generic travel content or image rights pages without fully grasping the intent.
Thanks to MUM and transformer-powered models, Google can interpret the complex nature of this query—understanding that the user seeks guidance on image rights related to a specific landmark—and provide results combining legal advice, image sourcing, and promotional tips.
Similarly, for a query like:
“best smartphone camera for night photography”
AI models synthesize product specifications, user reviews, expert opinions, and related images to deliver a comprehensive, nuanced answer that transcends simple keyword matching.
In summary, Google’s AI-driven search algorithms—powered by BERT, MUM, and the transformer architecture—have ushered in a paradigm shift from keyword-centric to intent-centric search. These models enable nuanced query interpretation, multimodal understanding, and sophisticated information synthesis at scale.
For SEO professionals and content creators, success now depends on embracing this shift by crafting authoritative, user-focused content that speaks the language of human intent—not just keywords. This approach aligns directly with the evolving standards of expertise, experience, authoritativeness, and trustworthiness (E-E-A-T) emphasized in Google’s 2025 algorithms, ensuring content remains relevant and competitive in the AI-driven search landscape.
Aspect | BERT | MUM | Transformer Architecture |
---|---|---|---|
Introduction Year | 2019 | 2021 | 2017 |
Core Function | Bidirectional contextual language understanding | Multimodal and multilingual comprehension | Self-attention mechanism enabling context weighting |
Language Support | 70+ languages | 75 languages | Underlying architecture, language-agnostic |
Multimodal Capabilities | No (text only) | Yes (text, images, potential audio/video) | Supports multimodal models |
Power Compared to BERT | Baseline | 1,000 times more powerful | Foundation for both BERT and MUM |
SEO Impact | Shift from keyword matching to semantic understanding | Need for relevant, authoritative, multimodal content | Enables efficient processing and richer understanding |
Example Query Handling | Understands implied meaning in queries | Synthesizes complex, multilingual, multimodal information | Enables long-range dependency capture in text |
Technological Innovation | Bidirectional reading of text | Multitask unified model integrating modalities | Self-attention replacing RNNs and CNNs |
Future Developments | Basis for further improvements | Enhanced with neural memory modules (Titans) | Supports extensions for memory and reasoning |
Decoding Google’s Ranking Signals in the AI Era: Expertise, Trustworthiness, and Context

Decoding Google’s Ranking Signals in the AI Era: Expertise, Trustworthiness, and Context
What truly distinguishes content that ranks highly in Google’s AI-powered search landscape today? The answer goes far beyond mere keyword matching. Google’s evolving algorithms increasingly prioritize the principles encapsulated in the E-E-A-T framework: Experience, Expertise, Authoritativeness, and Trustworthiness. These factors now form the cornerstone of content quality assessment in 2025, as AI enables more nuanced understanding and ranking capabilities.
The Rising Importance of the E-E-A-T Framework
Google’s focus on E-E-A-T has intensified with recent core updates, including the pivotal March 2025 rollout. Instead of simply scanning for keywords or backlinks, Google’s AI-driven systems and human quality raters scrutinize whether content demonstrates genuine expertise and real-world experience.
This is especially critical for Your Money or Your Life (YMYL) topics—such as health, finance, and safety—where the stakes are high. Websites covering these areas benefit greatly from employing domain experts and showcasing verifiable credentials like clear author bios and transparent contact information. These elements significantly bolster trustworthiness signals.
A fitting analogy is that Google no longer wants a well-dressed salesperson reciting a script; it seeks a seasoned professional who can demonstrate firsthand knowledge and confidently stand behind their claims. Consequently, SEO strategies focused solely on keyword stuffing or backlink accumulation without building authentic authority are increasingly ineffective.
Beyond Keywords: AI’s Sophisticated Content Evaluation
Google’s AI evaluates content quality well beyond keyword presence. Advanced models analyze depth, comprehensiveness, and corroboration from credible sources to identify the most valuable information.
Research shows that top-ranking pages typically average around 1,500 words, but more importantly, this length reflects meaningful detail rather than filler content. The best-performing content thoroughly answers user queries, integrates personal insights or direct experience, and cites authoritative sources, outperforming superficial or repetitive pages.
Google’s updated Search Quality Rater Guidelines explicitly target “filler” content and exaggerated claims. AI-generated text is not penalized by default; what matters is the content’s originality, accuracy, and usefulness. For example, AI-generated content that is carefully fact-checked, edited, and enriched with human expertise can rank on par with human-written material. This reflects a hybrid model where AI acts as a powerful tool but cannot replace the nuanced judgment that comes with experience and credibility.
Corroboration plays a vital role. Google’s AI seeks alignment with well-established consensus—particularly for critical topics—mirroring academic peer review processes where multiple credible sources validate findings before acceptance.
Technical SEO: The Hidden Infrastructure Supporting AI’s Judgments
While AI advances rapidly, technical SEO remains a fundamental pillar enabling effective content evaluation. Elements like site speed, structured data, and crawlability significantly influence how efficiently AI crawlers access and assess your content.
-
Site Speed: Slow-loading pages frustrate users and increase bounce rates. Google interprets these engagement metrics as signals of lower quality. Fast, responsive websites continue to enjoy clear ranking advantages, especially on mobile devices where over 60% of global users access the web.
-
Structured Data: Implementing schema markup helps AI models better understand the context and relationships within your content. Structured data enables Google to generate enhanced search features such as rich snippets and AI Overviews, which boost visibility and click-through potential.
-
Crawlability: A well-organized site architecture facilitates seamless navigation and indexing, allowing Google’s AI to discover fresh content promptly. Using content clusters and topical silos helps Google map your site’s relevance and authority more accurately.
Though technical SEO might feel like the “plumbing” behind your site, neglecting it handicaps AI’s ability to evaluate your content thoroughly. Think of it as maintaining a well-lit, organized library that enables a skilled librarian (AI) to find and recommend the best books quickly.
Personalization and Context: How AI Tailors Search Results
Google’s AI-powered search increasingly incorporates rich contextual signals—including user location, device type, search history, and even conversational context from AI modes—to personalize results dynamically. This means SEO is no longer a one-size-fits-all endeavor. Content relevance must now adapt to diverse user intents and scenarios.
For example, a search for a restaurant will prioritize local results based on the user’s geographic data. AI models also analyze prior queries to refine answers or suggest follow-up questions, creating a conversational search experience rather than a static list of links.
However, this personalization introduces complex privacy considerations. Google and regulatory bodies are focused on ensuring AI-driven personalization respects user data protections and actively mitigates algorithmic biases. Key compliance pillars include transparency in data use, explicit consent mechanisms, and ongoing bias audits.
Ethical SEO practitioners must stay informed about evolving privacy regulations such as the EU AI Act and state-level laws, and advocate for responsible AI deployment that balances personalization with user trust.
Balancing Innovation with Ethical SEO Practices
The AI era in search demands a strategic blend of technical rigor, authoritative content creation, and ethical responsibility. SEO professionals who invest in genuine expertise, maintain transparent and trustworthy signals, and optimize their technical infrastructure are best positioned to thrive amid Google’s AI advancements.
At the same time, acknowledging uncertainties—such as occasional AI misattributions or shifting regulatory requirements—prepares practitioners for continuous adaptation. Ultimately, the AI-enhanced search ecosystem rewards those who prioritize value, credibility, and user-centric design over shortcuts or gimmicks.
In summary, mastering Google’s AI-powered ranking signals requires embracing the full spectrum of E-E-A-T, delivering substantive and well-sourced content, optimizing core technical SEO fundamentals, and respecting the nuanced context of personalization and privacy. This holistic approach cuts through hype and aligns SEO strategies with the evolving realities of search technology and user expectations.
Aspect | Description | Key Points |
---|---|---|
E-E-A-T Framework | Experience, Expertise, Authoritativeness, and Trustworthiness are core to content quality. | Prioritized in 2025; critical for YMYL topics; requires domain experts and verifiable credentials. |
Content Evaluation Beyond Keywords | AI assesses depth, comprehensiveness, originality, and corroboration from credible sources. | ~1,500 words with meaningful detail; fact-checked AI content can rank well; avoids filler and exaggerated claims. |
Technical SEO | Supports AI content evaluation through infrastructure and accessibility. | Site speed, structured data (schema markup), crawlability; impacts user experience and indexing. |
Personalization and Context | AI incorporates user location, device, history, and conversational context to tailor results. | Dynamic results; privacy compliance with transparency, consent, and bias audits important. |
Ethical SEO Practices | Balancing innovation with transparency and trustworthiness. | Focus on genuine expertise, transparency, continuous adaptation to regulations, and user-centric design. |
Technical Deep Dive: How AI Algorithms Integrate Semantic Search, Vector Ranking, and Real-Time Processing
Technical Deep Dive: How AI Algorithms Integrate Semantic Search, Vector Ranking, and Real-Time Processing
How does Google’s AI-powered search transcend simple keyword matching to deliver results that feel intuitive, relevant, and personalized? The answer lies in a sophisticated blend of semantic understanding, vector space modeling, hybrid ranking techniques, and a real-time data processing architecture engineered to handle the staggering scale of the web.
Semantic Search and Vector Embeddings: From Keywords to Meaning
At the core of modern AI search engines is semantic search, which moves beyond the traditional “find exact keywords” approach. Instead, it focuses on understanding intent and context—the underlying reasons behind a query.
Google’s Knowledge Graph illustrates this evolution well, expanding from processing 570 million entities a decade ago to managing over 8 billion entities and 800 billion facts today. This vast structured knowledge base enables the search engine to identify distinct concepts (entities) and their relationships, allowing results to align with user intent rather than just matching surface-level terms.
Technically, this is powered by vector embeddings—dense, numerical representations of text and other data types that capture semantic meaning within a high-dimensional space. Imagine each word, sentence, or document as a point in a multi-dimensional universe where distance reflects meaning similarity. Queries and content are mapped into this space, so the system matches on meaning, not just exact lexical overlap.
For example, a query like “best vegan breakfast near me” isn’t matched simply by scanning for “vegan” and “breakfast.” Vector embeddings capture the semantic relationship between “vegan,” “plant-based,” “morning meal,” and location context, enabling Google to surface relevant local restaurants that traditional keyword search might miss.
Key technical elements include:
- Machine learning models that convert queries and documents into vector embeddings.
- Storage of these embeddings in vector databases optimized for high-dimensional nearest neighbor search.
- Use of similarity scoring metrics, such as cosine similarity, to rank results by semantic relevance.
This approach is critical for handling conversational and natural language queries, voice searches (which now account for over 50% of internet searches with an average of 29 words per query), and multimodal inputs like images and videos.
Real-Time Processing Architecture: Scaling AI at Web Scale
Delivering semantically rich, personalized results instantly requires a robust and scalable infrastructure. Google’s AI search operates on massive data pipelines that continuously ingest, preprocess, and index content in near real-time.
Core architectural components include:
- Data ingestion pipelines built on cloud-native, distributed technologies like Google Cloud Dataflow and Apache Kafka. These handle continuous streams of crawled web data, user interaction logs, and contextual signals.
- Indexing and embedding generation, performed through specialized AI models that extract entity relationships, generate vector embeddings, and annotate content with structured metadata.
- Hybrid storage solutions that combine traditional inverted indexes for keyword lookups with vector databases such as Pinecone or Weaviate, optimized for millisecond nearest neighbor queries across billions of records.
- Personalization layers that incorporate user-specific signals—search history, location, device type, time of day—and process these through ML models to dynamically adjust rankings.
This pipeline balances latency and throughput, ensuring users receive contextually relevant answers without perceptible delay. For instance, Google’s hybrid ranking approach employs Reciprocal Rank Fusion (RRF) to merge semantic and keyword-based results, achieving both precision and recall at scale.
Hybrid Ranking: Merging Traditional IR and Deep Learning
While semantic vector search is powerful, it’s not a silver bullet. Google’s AI search blends semantic embeddings with classical Information Retrieval (IR) methods to leverage the strengths of both.
Traditional IR models like BM25 excel at handling exact keyword matches and provide interpretable ranking signals. Deep learning models capture nuanced semantic relationships and broader context. Google’s hybrid ranking process includes:
- Running parallel queries on sparse token-based indices (for keywords) and dense vector embeddings (for semantic content).
- Combining results using algorithms like Reciprocal Rank Fusion, balancing contributions from each signal to produce a unified and highly relevant ranking.
- Applying deep learning models for re-ranking results by incorporating contextual features and user behavior signals.
This hybrid approach enhances relevance while managing computational costs—a crucial factor for real-time systems processing billions of queries daily.
Challenges: Scalability, Interpretability, and Fairness
Despite these technological advances, significant challenges remain:
-
Scalability: Managing vector embeddings and semantic indexes at web scale demands enormous computational resources and efficient algorithms such as Hierarchical Navigable Small World (HNSW) graphs to maintain millisecond query response times.
-
Interpretability: Deep learning–driven rankings often behave like “black boxes,” making it difficult for SEO professionals and users to understand why a particular result was surfaced. Explainable AI (XAI) techniques are emerging to improve transparency but currently have limited adoption.
-
Fairness: AI ranking systems risk perpetuating biases embedded in training data or user behavior patterns, leading to skewed or unfair outcomes. Efforts to detect and mitigate bias, including bias-aware agents that combine large language model reasoning with bias detection tools, are critical but still evolving.
Bringing It All Together
Google’s AI ranking ecosystem exemplifies a complex, multi-layered system where:
- Semantic search and vector embeddings provide a rich understanding of content and queries.
- Real-time data ingestion and processing pipelines power personalized, instantaneous results.
- Hybrid ranking synthesizes traditional IR techniques with deep learning to optimize relevance.
- Ongoing research addresses transparency, scalability, and ethical implications.
For SEO professionals and content creators, this means focusing less on rigid keyword stuffing and more on crafting entity-rich, contextually meaningful content aligned with user intent and Google’s E-E-A-T framework. Technical SEO remains essential to ensure AI systems can access and evaluate content effectively.
For technologists and strategists, it’s a reminder that AI search is a balancing act of advanced modeling, rigorous engineering, and principled fairness.
Looking ahead, the next frontier will likely feature tighter integration of multimodal data, continual personalization through federated learning, and improved explainability—all while maintaining a commitment to equitable and transparent AI systems that serve users fairly.
Aspect | Description | Key Components / Examples |
---|---|---|
Semantic Search and Vector Embeddings | Moves beyond keyword matching to understand intent and context using vector space modeling. | Knowledge Graph with 8B+ entities, vector embeddings, cosine similarity, machine learning models, vector databases |
Real-Time Processing Architecture | Massive data pipelines ingest and process content near real-time for personalized, instant results. | Google Cloud Dataflow, Apache Kafka, embedding generation, hybrid storage (inverted index + vector DB), personalization layers |
Hybrid Ranking | Combines classical IR methods and deep learning to optimize relevance and computational efficiency. | BM25, vector embeddings, Reciprocal Rank Fusion (RRF), deep learning re-ranking |
Challenges | Technical and ethical issues in scalability, interpretability, and fairness of AI ranking. | HNSW graphs for scalability, Explainable AI (XAI), bias-aware agents, fairness mitigation techniques |
SEO Implications | Focus on entity-rich, contextually meaningful content aligned with user intent and E-E-A-T framework. | Technical SEO, content aligned with semantic search, avoiding keyword stuffing |
Future Directions | Tighter multimodal integration, continual personalization, improved explainability, equitable AI. | Federated learning, multimodal data, explainability improvements |
SEO Strategies for Thriving in Google’s AI-Powered Search Landscape
SEO Strategies for Thriving in Google’s AI-Powered Search Landscape
How do you stay competitive when Google’s AI algorithms don’t just rank pages—they interpret, summarize, and even answer queries directly? The shift toward AI-driven search demands a recalibration of SEO tactics, emphasizing not only technical precision but also authentic, user-focused content crafted with a deep understanding of intent and trustworthiness.
Creating Content That Resonates with AI and Humans Alike
Google’s AI rewards quality over quantity and penalizes fluff or keyword stuffing. Central to content evaluation is the E-E-A-T framework: Experience, Expertise, Authoritativeness, and Trustworthiness. This framework has become the backbone of content quality assessment, especially after the Helpful Content updates and the 2025 core algorithm enhancements.
- Experience matters: Demonstrate firsthand knowledge through original research, detailed case studies, or personal insights. For instance, a healthcare blog citing a physician’s direct observations consistently outranks generic medical summaries.
- Expertise and authoritativeness: Credentials, citations from reputable sources, and transparent author bios strengthen your site’s credibility. Google’s guidelines clearly state that “using automation to manipulate rankings is a violation of spam policies,” underscoring that AI-generated content must be carefully vetted.
- Trustworthiness: Secure HTTPS sites, fact-checked information, and transparent business practices contribute to trust signals. Trust is paramount as both users and Google’s AI grow increasingly skeptical of misinformation.
Aim for comprehensive topic coverage that anticipates related questions and subtopics. Think of your content as a carefully woven tapestry, where each thread connects logically to the next. This approach aligns with Google’s emphasis on semantic understanding, topic clusters, and conversational, long-tail queries favored by voice search.
Capturing Featured Snippets, Voice Search, and Zero-Click Opportunities
Google’s AI is reshaping search by delivering instant, concise answers directly on the results page. Featured snippets, voice search responses, and AI-generated answer boxes—often leading to zero-click searches—are now dominant features.
- Featured snippets: To secure these coveted spots, content must be well-structured, clear, and directly answer common user queries. Google typically sources snippets from pages already ranking well for target keywords. Use concise paragraphs, bullet points, and numbered lists to enhance snippet eligibility.
- Voice search: Over 50% of internet searches are voice-activated, with voice queries averaging around 29 words. Optimize by incorporating natural, conversational language and long-tail keywords that mirror spoken queries. Keep answers brief—around 30 words—to align with typical voice response lengths.
- Zero-click searches: Nearly 60% of searches in the U.S. end without a click, making Answer Engine Optimization (AEO) vital. Structure your content for AI parsing using schema markup, question-based formats, and clear, factual answers. Even without clicks, your brand gains visibility and authority.
For example, a local restaurant optimizing for voice search might target queries like “best vegan options near me open now,” structuring content to answer that question succinctly and marking up menus and business hours with structured data, as illustrated in the dependent sections.
Technical SEO: Foundations for AI-Friendly Crawling and User Experience
Even the best content won’t rank if Google’s AI can’t efficiently crawl or interpret your site. Technical SEO remains critical, especially as AI models increasingly rely on structured, well-organized data.
- Site architecture: Logical internal linking facilitates navigation for both AI and users, distributing authority and signaling content relationships. Avoid deep nesting that buries important pages.
- Crawl efficiency: Use robots.txt and XML sitemaps strategically to prioritize valuable and recently updated pages. For large sites, minimize redirect chains and consolidate duplicate content with canonical tags to optimize crawl budget.
- Page speed and lazy loading: With over 60% of users on mobile devices and nearly 60% abandoning sites loading slower than three seconds, lazy loading defers non-critical content to improve perceived load times and user engagement. Implement it carefully to avoid hindering crawlability.
- Security: HTTPS is essential for trust and ranking. Secure websites are favored by both users and Google’s algorithms.
Additionally, tools like prerendering ensure AI crawlers correctly index dynamic or JavaScript-heavy content, which traditional crawlers may struggle to interpret.
Ethical Use of AI in Content Creation: Balancing Efficiency with Authenticity
AI is a powerful assistant but cannot replace human insight. Ethical considerations are crucial to maintain originality, verifiability, and user trust.
- AI as a partner: Leverage AI tools to draft outlines, brainstorm ideas, or refine tone, but always apply human review to verify facts and enhance authenticity.
- Originality matters: Google’s algorithms demote content crafted solely to manipulate rankings, including AI-generated fluff. Combining AI-generated drafts with personal experience and detailed research preserves uniqueness.
- Transparency and fairness: AI systems can inadvertently propagate bias. Content creators must ensure diverse perspectives and avoid reinforcing stereotypes or misinformation.
- Compliance with spam policies: Avoid over-automation aimed at gaming rankings. Focus on creating genuinely helpful, accurate content that serves real users.
NP Digital exemplifies responsible AI use by combining AI tools like ChatGPT-4 and Claude with human editorial review, demonstrating an effective “human-in-the-loop” approach.
Real-World Adaptations: Lessons from SEO Leaders
Brands embracing AI-powered search changes emphasize holistic strategies that integrate content quality, technical SEO, and ethical AI use.
- Companies tracking modern engagement signals—not just pageviews but time on page and interaction depth—have seen improved rankings.
- Sites optimized for voice search and featured snippets report up to 68% more engagement.
- Incorporating structured data and semantic SEO helps maintain visibility amidst the rise of zero-click searches.
- Ethical AI use paired with human expertise fosters long-term trust and reduces risks of algorithm penalties.
In summary, succeeding in Google’s AI-driven search landscape requires thoughtful integration of technology, user-centric content, and technical excellence. The future favors those who respect both the machine’s logic and the human experience behind every query.
SEO Strategy | Key Elements | Best Practices / Examples |
---|---|---|
Creating Content That Resonates with AI and Humans |
|
|
Capturing Featured Snippets, Voice Search, and Zero-Click Opportunities |
|
|
Technical SEO: Foundations for AI-Friendly Crawling and UX |
|
|
Ethical Use of AI in Content Creation |
|
|
Real-World Adaptations by SEO Leaders |
|
|
Comparative Analysis: Google’s AI Search Versus Competitors and Emerging Models
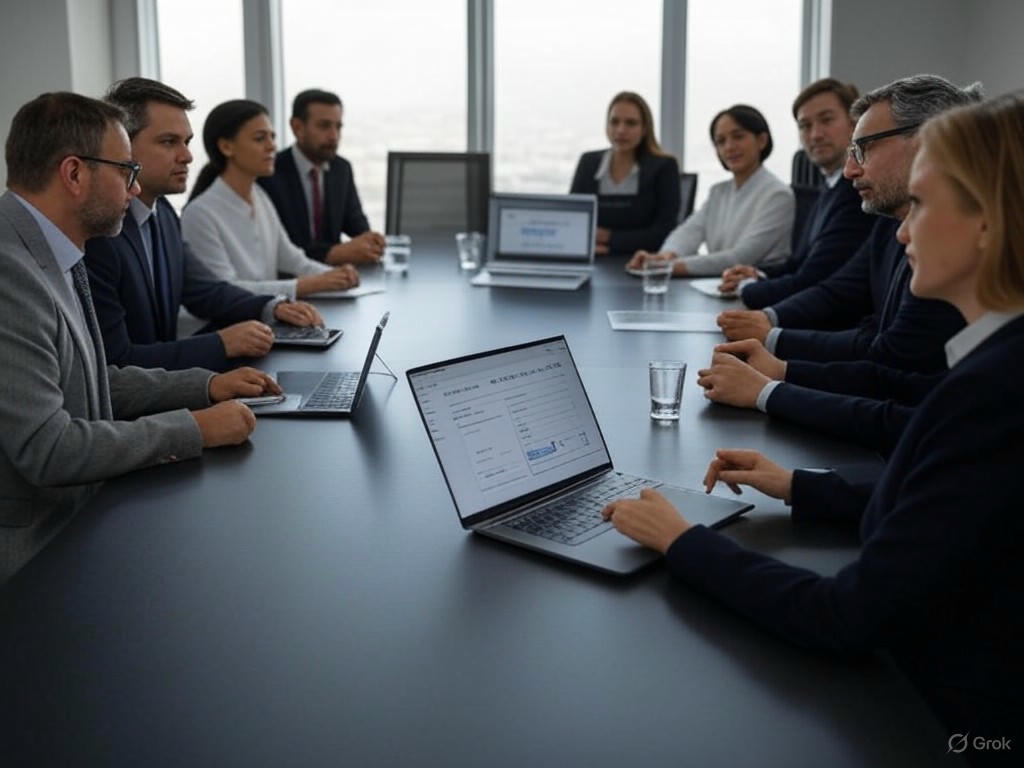
Comparative Analysis: Google’s AI Search Versus Competitors and Emerging Models
When discussing AI in search engines, Google naturally leads the conversation. Yet, understanding how Google’s AI search compares to other AI-driven platforms—such as Bing AI, ChatGPT-powered search, and emerging vector-based search technologies—is essential for marketers, SEO professionals, and content creators navigating today’s evolving landscape.
Benchmarking AI Architectures and Ranking Algorithms
Google’s AI search is powered by its advanced Gemini series, with the latest being Gemini 2.5 Pro. This model represents a sophisticated evolution emphasizing not only broad language comprehension but also enterprise-grade specificity. Google focuses on smaller, efficient models capable of running across various devices, ensuring scalability and responsiveness (TechCrunch, 2025). Gemini’s tight integration with Google’s core search infrastructure enables features like AI Overviews—concise, AI-generated summaries synthesized from multiple indexed sources with embedded clickable links—designed to enhance transparency and build user trust (Immwit, 2025).
In comparison, Bing AI leverages Microsoft’s partnership with OpenAI, integrating GPT models alongside Bing’s proprietary ranking and filtering systems. Bing continues to value traditional SEO signals such as clarity, structured data, and inbound links but also places significant weight on user satisfaction metrics. This hybrid approach balances AI-generated summaries with conventional search results, reflecting a blend of innovation and established search principles (Writesonic, 2025; Microsoft Support).
ChatGPT-powered search adopts a more purely conversational and generative approach. Unlike Google or Bing, which combine AI with ongoing web indexing, ChatGPT primarily relies on fine-tuned large language models (LLMs) trained on vast datasets. It supplements these with real-time web retrieval through partnerships and third-party providers (OpenAI, 2025). This results in an explorative, dialogue-driven search experience, well-suited for in-depth understanding but less effective at delivering real-time updates or handling fragmented, keyword-centric queries that remain common among typical users (9Rooftops, 2025).
Vector-based search platforms represent another innovative frontier. These systems store and query high-dimensional data vectors, enabling semantic similarity searches that more precisely match user intent, especially for unstructured content (LakeFS, 2023; Shakudo, 2025). Platforms like Pinecone, Weaviate, and Vespa are increasingly vital for AI applications demanding nuanced semantic understanding beyond simple keyword matching. Their architectures provide lightning-fast retrieval and seamless integration with diverse AI frameworks, though they remain more specialized and less consumer-facing compared to Google or Bing.
Differences in User Interaction Models
Google is aggressively advancing conversational AI search through its AI Mode, currently available in Labs for U.S. users. This mode supports multi-turn, complex question answering and follow-ups, blending traditional search results with AI-generated explanations and insights. The design prioritizes fluid, iterative user interactions, moving beyond static keyword queries toward dynamic conversations that better capture intent (Google Blog, 2025).
Similarly, Bing AI offers conversational search with a strong focus on natural language understanding and multi-step query refinement. Bing’s chat interface includes productivity tools like bookmarking and collections to help users organize information, aligning closely with Microsoft’s broader productivity ecosystem (PageOn.ai, 2025). However, Bing retains a stronger emphasis on structured content and concise, clear delivery to optimize user satisfaction signals.
ChatGPT search epitomizes the conversational AI experience. It excels in understanding natural language and generating human-like responses but sometimes sacrifices up-to-date factual accuracy or comprehensive coverage of live web data. Its interface encourages exploratory dialogue, making it ideal for deep dives into topics but less suited for quick fact retrieval or transactional searches compared to Google’s real-time indexing (OpenAI, 2025; 9Rooftops, 2025).
Traditional keyword-based search engines, while increasingly integrating AI features, still rely heavily on classic SEO signals such as backlinks, page structure, and keyword relevance. These engines efficiently handle straightforward, transactional queries but face growing competition from conversational AI interfaces catering to complex or multi-faceted information needs.
Competitive Dynamics and SEO Implications
The interplay among Google, Microsoft Bing, OpenAI, and emerging vector search providers is driving profound shifts in SEO strategies. Google’s continuous AI innovations—like AI Overviews that place synthesized answers atop search results and Gemini-powered AI Mode—are reshaping visibility paradigms. Content creators must now ensure their material is not only optimized for rankings but also authoritative and structured enough to be featured within AI-generated summaries (The Future of Commerce, 2025; Semrush, 2025).
Bing’s approach rewards clarity and user satisfaction, prompting marketers to optimize for both AI interpretability and foundational SEO best practices. Bing’s preference for static HTML and well-structured content means technical compliance remains critical alongside AI-friendly content creation (Writesonic, 2025).
ChatGPT and similar conversational AI tools are encouraging a shift toward content strategies focused on semantic authority, trustworthiness, and coherent narrative flow. Since these platforms rely heavily on pre-trained data and curated sources, marketers must ensure their content is factually accurate, contextually relevant, and engaging for AI consumption (Search Engine Land, 2025).
Vector-based search technologies, while currently more relevant in enterprise and AI application contexts than consumer-facing SEO, influence future SEO trends. Their ability to capture semantic relationships points toward a landscape where vector embeddings and semantic relevance become vital ranking factors in specialized scenarios (LakeFS, 2023; DataCamp, 2025).
Maintaining Google’s Competitive Edge: What It Means for Stakeholders
Google’s leadership in AI search is actively maintained through continuous enhancements in AI models, extensive multilingual support, and seamless integration with its broader ecosystem, including advertising and content discovery platforms (Coalition Technologies, 2025). Innovations like Project Astra and Android XR hint at a future where AI search transcends text, extending into multimodal and immersive experiences.
For marketers and content creators, this evolving landscape demands:
- Prioritizing high-quality, authoritative content aligned with Google’s E-E-A-T (Experience, Expertise, Authoritativeness, Trustworthiness) framework.
- Optimizing for AI Overviews by crafting fact-based, well-structured, and device-responsive content.
- Combining traditional SEO with AI-aware strategies, recognizing that ranking signals now encompass semantic relevance and user interaction metrics beyond backlinks and keywords.
- Monitoring AI search behavior across platforms, diversifying presence to include Bing, ChatGPT, and emerging AI-powered search alternatives.
- Leveraging structured data and schema markup, which remain critical for AI models to correctly interpret and feature content.
For end-users, these advancements promise more conversational, context-aware, and personalized search experiences. However, balancing AI’s generative capabilities with accuracy and transparency remains vital to mitigate risks like hallucinations or misinformation.
In summary, Google’s AI search leads through advanced model architectures and deep ecosystem integration but faces significant competition from Bing’s hybrid approach, ChatGPT’s conversational interface, and the semantic precision of vector-based platforms. To thrive in this dynamic environment, SEO and content strategies must evolve—embracing AI’s strengths while maintaining rigorous quality and trustworthiness in content creation.
Aspect | Google AI Search | Bing AI | ChatGPT-powered Search | Vector-based Search Platforms |
---|---|---|---|---|
AI Architecture | Gemini series (latest Gemini 2.5 Pro); efficient, scalable models integrated with core search | Microsoft + OpenAI GPT models; hybrid with proprietary ranking and filtering | Fine-tuned large language models; supplemented with real-time web retrieval via partners | High-dimensional data vectors enabling semantic similarity; platforms like Pinecone, Weaviate, Vespa |
Ranking Algorithms | Focus on language comprehension, enterprise specificity, AI Overviews with clickable sources | Balancing traditional SEO signals and user satisfaction metrics with AI-generated summaries | Conversational generative approach; less focused on real-time updates or fragmented queries | Semantic relevance and fast retrieval; specialized for nuanced AI applications |
User Interaction Model | AI Mode with multi-turn, complex Q&A; blends traditional results with AI insights; dynamic conversations | Conversational search with NLP and multi-step query refinement; productivity tools like bookmarking | Exploratory, dialogue-driven; excels in natural language but less real-time accuracy | Primarily backend semantic search; less consumer-facing interaction |
SEO Implications | Need authoritative, structured content optimized for AI Overviews; focus on E-E-A-T and device responsiveness | Optimize clarity, user satisfaction, static HTML, and structured content for AI and traditional SEO | Content focused on semantic authority, trustworthiness, and coherent narratives for AI consumption | Emerging trend toward semantic relevance and embedding-based ranking in specialized contexts |
Competitive Edge | Continuous AI innovation, multilingual support, ecosystem integration, future multimodal/immersive search | Hybrid innovation combining AI and traditional SEO; aligned with Microsoft productivity ecosystem | Strong conversational AI experience; suited for deep topic exploration over quick facts | Specialized semantic search capabilities with lightning-fast retrieval; more enterprise-focused |
Future Trajectories: Anticipating the Evolution of AI in Search and Its Broader Societal Impact
Future Trajectories: Anticipating the Evolution of AI in Search and Its Broader Societal Impact
What will the search experience look like as AI matures beyond its current capabilities? Google’s AI-powered search is already reshaping how we discover and consume information, but the next phase promises deeper contextual understanding, richer multimodal interactions, and a more personalized yet privacy-conscious approach. These advances bring exciting opportunities but also critical questions about information reliability, transparency, and the societal effects of increasingly curated knowledge ecosystems.
Toward Greater Contextual Awareness and Multimodal Search Integration
Imagine asking Google not only a text query but also showing it a photo, video, or describing a sound—and receiving a coherent, comprehensive answer synthesizing all these inputs. This vision is rapidly becoming reality. Google’s AI Mode, currently available in Search Labs, supports multimodal search, enabling users to “search what you see” through tools like Google Lens, which handles nearly 20 billion visual searches monthly—20% of those related to shopping alone.
This shift is more than a novelty; it fundamentally transforms SEO strategies. Content must now be optimized for image and video queries alongside text, reflecting the rise of multimodal search.
Furthermore, conversational AI is evolving to support back-and-forth dialogues that allow clarifications and follow-ups, mimicking human interaction. Google’s Multitask Unified Model (MUM) extends beyond language to incorporate cross-lingual and cross-modal reasoning across 75 languages and multiple data types. This enhanced contextual awareness means search engines can interpret the “why” behind queries, not just the “what,” delivering more relevant and personalized results.
Explainability and Transparency: Demystifying AI Ranking Decisions
As AI models grow more complex, a pressing challenge is understanding why certain content ranks higher. Traditional SEO relied on clear signals like backlinks, keywords, and freshness, but AI’s decision-making can feel like a black box.
Transparency is not just a technical nicety—it’s an ethical imperative. Google and other search engines are exploring ways to enhance explainability, potentially through AI-generated summaries that highlight the rationale behind ranking choices and cite trusted sources.
However, recent studies reveal that AI-generated content summaries sometimes misattribute citations or confidently present incorrect information, undermining trust. This “citation problem” underscores the need for rigorous scrutiny and validation of AI’s reasoning, especially in sensitive domains like health, finance, or legal information.
Personalization Balanced with Privacy Concerns
Personalized search results are now mainstream. By analyzing location, search history, preferences, and device usage, AI tailors results much like a personal librarian who knows your tastes and needs. This personalization can significantly enhance user experience and engagement—fast-growing companies report generating 40% more revenue through personalization efforts.
Yet personalization introduces risks of filter bubbles, where users may be confined to echo chambers that reinforce existing beliefs and limit exposure to diverse viewpoints. Ethical considerations must guide how personalization balances relevance with serendipity and fairness.
Privacy remains paramount. While Google and others employ privacy-enhancing technologies (PETs) to protect user data, only about 37% of customers trust companies with their personal information. The future of AI in search depends on developing models that deliver personalization without compromising privacy, potentially through decentralized data governance or on-device processing such as federated learning.
Societal Implications: Navigating Information Reliability and Democratization
As AI increasingly mediates access to knowledge, ensuring information reliability becomes critical. AI Overviews—AI-generated summaries prominently displayed above traditional search results—reshape how users consume information, especially for Your Money or Your Life (YMYL) topics like health and finance.
Reliance on these summaries demands confidence that underlying data and models are accurate and unbiased. The democratization of knowledge is one of AI’s most hopeful promises. Generative AI lowers barriers, enabling users worldwide to access complex information in over a hundred regional languages and through intuitive modalities.
This broadened access empowers individuals to learn and innovate, potentially transforming economies and societies.
However, democratization requires ongoing ethical vigilance. As AI curates presented knowledge, it risks entrenching biases, amplifying misinformation, or marginalizing less dominant voices. Transparency, human oversight, and multi-stakeholder governance structures are essential to ensure AI serves the public good rather than narrow interests.
Strategic Recommendations for Businesses and SEO Professionals
The accelerating AI-driven transformation demands agility and foresight from businesses and SEO practitioners:
-
Embrace AI-powered SEO tools that analyze user intent, predict trending topics, and optimize content for text, image, and video queries. Traditional keyword stuffing is no longer effective.
-
Focus on authoritative, high-quality content that genuinely answers user questions, as AI models increasingly reward domain expertise, trustworthiness, and relevance over sheer volume.
-
Invest in ethical AI practices by ensuring transparency, respecting user privacy, and avoiding manipulative tactics that exploit AI’s quirks.
-
Monitor evolving AI features like AI Overviews and multimodal search, adapting strategies to capture visibility in these new search result formats.
-
Cultivate a multi-channel presence integrating social media, voice, and visual platforms to meet users where they are, recognizing that search is no longer a linear, text-only journey.
-
Stay informed and flexible, as AI search algorithms evolve rapidly. Continuous learning and rapid iteration are essential to maintaining competitive advantage.
Conclusion
AI in search is progressing toward smarter, more intuitive, and deeply personalized experiences that integrate multiple data modalities. This evolution promises unprecedented access to knowledge and efficiency gains but also magnifies challenges around trust, bias, and privacy.
Navigating this landscape requires not only technical expertise but a steadfast commitment to ethical principles and transparency. For businesses and SEO professionals willing to adapt intelligently, the future of AI-powered search offers tremendous opportunity—provided we remain vigilant about its broader societal impacts.
Aspect | Details |
---|---|
Toward Greater Contextual Awareness and Multimodal Search Integration |
|
Explainability and Transparency |
|
Personalization Balanced with Privacy |
|
Societal Implications |
|
Strategic Recommendations for Businesses and SEO Professionals |
|