How AI Chatbots Are Revolutionizing Customer Service Support
- Introduction: Why AI Chatbots and Virtual Assistants Are Reshaping Customer Service
- Introduction: Why AI Chatbots and Virtual Assistants Are Reshaping Customer Service
- Accelerating Adoption of AI-Driven Chatbots and Virtual Assistants
- The Significance of 24/7 Availability and Scalability
- The Dual Role of AI: Augmenting Humans and Automating Routine Tasks
- Cutting Through the Hype: What to Expect
- Foundations of AI in Customer Service: Architecture, Models, and Capabilities
- Foundations of AI in Customer Service: Architecture, Models, and Capabilities
- Core AI Technologies Powering Conversational Agents
- Architectural Components: From Knowledge Bases to Machine Learning Pipelines
- Demystifying Transformer-Based Large Language Models: Strengths and Limitations
- Connecting the Dots: Why These Foundations Matter for Customer Service
- Performance Metrics and Technical Challenges: Accuracy, Scalability, and Error Handling
- Performance Metrics and Technical Challenges: Accuracy, Scalability, and Error Handling
- Measuring Chatbot Performance
- Technical Challenges: Ambiguity, Multilingual Support, and Error Management
- Mitigating Risks: Guardrails, Fallbacks, and Regulatory Compliance
- Summary
- Human-AI Collaboration: Balancing Automation with Empathy and Expertise
- Human-AI Collaboration: Balancing Automation with Empathy and Expertise
- Task Segmentation: Defining Roles for AI and Humans
- Workforce Impacts and Agent Upskilling
- Ethical Considerations: Transparency and Building Customer Trust
- Integrating Support Workflows and Visual Engagement Tools
- Ethical and Privacy Considerations in Deploying AI Chatbots
- Ethical and Privacy Considerations in Deploying AI Chatbots
- Transparency and Informed Consent: Building the Foundation of Trust
- Data Privacy and Security: Protecting Sensitive Customer Information
- Addressing Bias and Ensuring Ethical AI Behavior
- Architectural Strategies to Enforce Compliance and Safeguard Consumer Rights
- Comparative Analysis: AI Chatbots Versus Traditional Customer Support Models
- Comparative Analysis: AI Chatbots Versus Traditional Customer Support Models
- Cost Efficiency and ROI: AI Chatbots and Virtual Assistants Surpass Traditional Support
- Response Speed and Scalability: AI Enables Instant, Around-the-Clock Support
- Customer Satisfaction: Combining AI Efficiency with Human Empathy
- Situational Effectiveness: When AI Excels and When Human Intervention Is Essential
- Key Takeaways
- Future Directions: Emerging Trends and the Evolving Landscape of AI in Customer Service
- Future Directions: Emerging Trends and the Evolving Landscape of AI in Customer Service
- Emotional Intelligence and Continual Learning: Chatbots That Understand and Evolve
- Seamless Omnichannel Integration: AI as the Conductor of Customer Journeys
- Regulatory Landscape and Ethical Guardrails: Navigating Uncharted Waters
- Workforce Transformation: Reinventing Roles in an AI-Augmented Era
- Strategic Navigation: Balancing Opportunity with Prudence
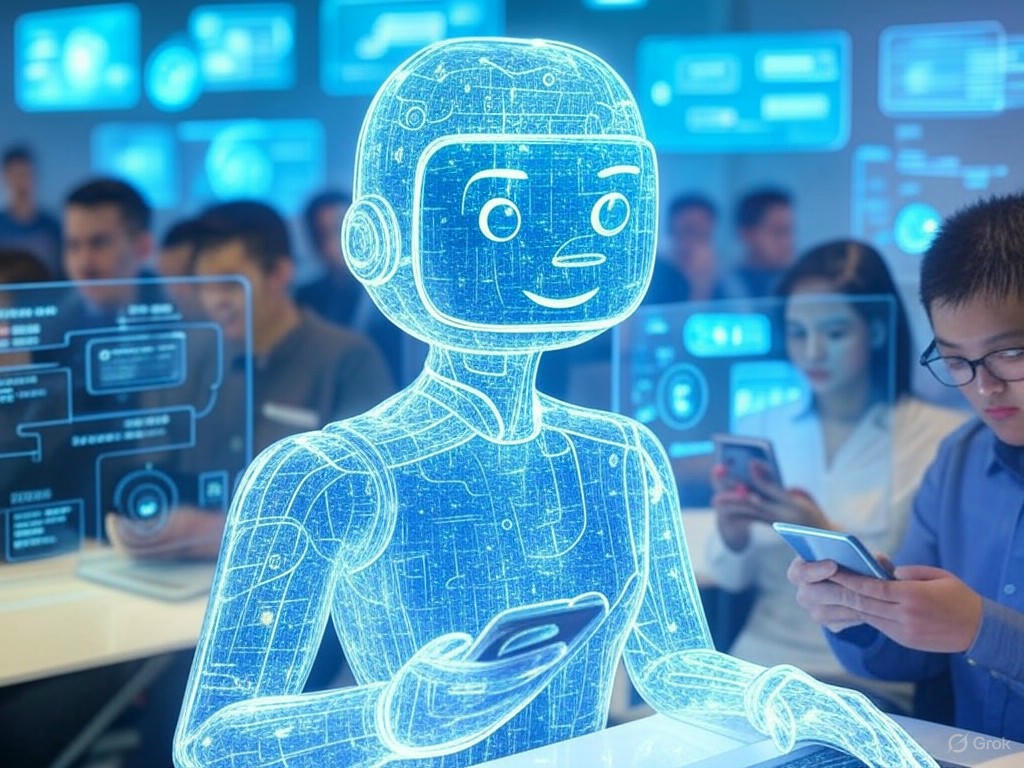
Introduction: Why AI Chatbots and Virtual Assistants Are Reshaping Customer Service
Introduction: Why AI Chatbots and Virtual Assistants Are Reshaping Customer Service
Have you noticed how your customer service interactions increasingly involve chatbots or virtual assistants? This shift is not incidental; it reflects a profound transformation driven by the rapid adoption of AI technologies designed to meet evolving consumer expectations and dynamic business demands.
Accelerating Adoption of AI-Driven Chatbots and Virtual Assistants
By 2025, AI-powered chatbots and virtual assistants have become foundational components of customer support infrastructures rather than experimental tools. Consider these key data points:
- Nearly 80% of companies are already using or planning to adopt AI chatbots for customer service, signaling a significant strategic shift (Desk365.io).
- The global chatbot market has grown from $2.47 billion in 2021 to over $15.57 billion in 2025, with projections surpassing $47 billion by 2030 (ExplodingTopics, GlobeNewswire).
- Over 987 million people engage with AI chatbots worldwide today, making these systems a primary touchpoint for customer interactions (ExplodingTopics).
- Industries such as healthcare, retail, energy, and hospitality are at the forefront of AI adoption, customizing AI tools to address their unique customer service challenges (Zendesk, GlobeNewswire).
This rapid adoption reflects more than cost-saving efforts. AI is fundamentally reshaping how companies conceptualize customer service—transitioning from reactive, human-only models to hybrid systems where AI manages routine inquiries, allowing human agents to focus on complex cases.
The Significance of 24/7 Availability and Scalability
What makes AI exceptionally attractive for customer service now is its ability to deliver around-the-clock, scalable support that aligns with rising customer expectations.
Today’s customers demand instant responses anytime and anywhere. AI chatbots and virtual assistants offer:
- 24/7 availability, which eliminates wait times and enables businesses to support global, time-zone-spanning audiences without proportional increases in staffing costs (Quora, Zendesk).
- Scalability, allowing AI to handle surges in inquiry volumes—whether during promotional campaigns or unexpected service disruptions—without sacrificing response quality (GoTriple, LinkedIn).
- Personalization at scale, with AI systems analyzing extensive customer data to provide tailored recommendations and proactive support, enhancing engagement and satisfaction (Zendesk, ROI CX Solutions).
For instance, Yum! Brands’ AI systems deployed at drive-thru lanes have increased order processing speed by 10–15% while improving accuracy, demonstrating AI’s real-world operational efficiency (Desk365.io).
However, this scalability requires careful oversight. Without it, AI may deliver inconsistent responses or fail to adequately address nuanced customer emotions—critical in sensitive or high-stakes interactions.
The Dual Role of AI: Augmenting Humans and Automating Routine Tasks
A key nuance often lost in the hype is that AI chatbots and virtual assistants do not simply replace human agents—they augment them.
- Automating routine tasks: AI excels at handling repetitive, low-complexity queries such as order status updates, FAQs, or appointment scheduling. This automation frees human agents from monotonous tasks, enabling them to focus on higher-value activities (Verloop.io, Invoca).
- Augmenting human agents: AI tools support agents by delivering real-time information, sentiment analysis, and conversation summaries. This empowers agents to respond faster, more accurately, and with greater empathy during complex interactions (IBM, Crescendo.ai).
This human-AI symbiosis is essential. Humans bring empathy and judgment to interactions, while AI provides scale, speed, and data-driven insights. Yet, the journey toward AI maturity is ongoing; McKinsey finds that only about 1% of organizations consider themselves advanced in AI deployment, underscoring the work ahead to optimize this partnership.
Ethical considerations are paramount. AI systems inherit biases from their training data, raising concerns about fairness and discrimination in customer interactions. Ensuring transparency, continuous human oversight, and strong governance frameworks is critical to augmenting service quality without compromising trust (Zendesk, Dante AI).
Cutting Through the Hype: What to Expect
Despite media hype dubbing 2025 “the year of the AI agent,” the reality is more measured. Fully autonomous AI agents capable of complex decision-making remain under development. Present AI solutions serve as powerful assistants rather than replacements, requiring careful orchestration and continuous refinement (IBM).
Organizations that approach AI deployment thoughtfully—aligning technology capabilities with human expertise and ethical guardrails—are realizing measurable benefits, including:
- Customer service cost reductions averaging up to 25% (Desk365.io, 2025).
- Significant improvements in customer satisfaction and reductions in escalation rates (Intercom, Salesforce case studies).
- Enhanced agent productivity and reduced burnout by offloading repetitive tasks to AI.
In summary, AI chatbots and virtual assistants are reshaping customer service not by supplanting humans but by redefining the division of labor—letting AI handle predictable inquiries while humans focus on exceptional, nuanced interactions.
This article will explore these dynamics in greater depth, examining the technical capabilities driving AI-powered customer service, the ethical challenges businesses must navigate, and the evolving future of AI in this critical domain.
Aspect | Details / Data Points | Sources |
---|---|---|
Company Adoption Rate | Nearly 80% of companies using or planning to adopt AI chatbots for customer service | Desk365.io |
Market Growth | Chatbot market grew from $2.47B (2021) to $15.57B (2025), projected >$47B by 2030 | ExplodingTopics, GlobeNewswire |
User Engagement | Over 987 million people engage with AI chatbots worldwide | ExplodingTopics |
Leading Industries | Healthcare, retail, energy, hospitality customizing AI tools | Zendesk, GlobeNewswire |
24/7 Availability | Eliminates wait times, supports global audiences without proportional staffing increases | Quora, Zendesk |
Scalability | Handles surges in inquiry volume without sacrificing quality | GoTriple, LinkedIn |
Personalization at Scale | AI analyzes customer data to provide tailored recommendations and proactive support | Zendesk, ROI CX Solutions |
Operational Efficiency Example | Yum! Brands’ AI increased drive-thru order speed by 10-15% with improved accuracy | Desk365.io |
Human-AI Task Division | AI automates routine tasks; humans handle complex cases and provide empathy | Verloop.io, Invoca, IBM, Crescendo.ai |
AI Deployment Maturity | Only ~1% of organizations consider themselves advanced in AI deployment | McKinsey |
Ethical Concerns | Bias in AI training data; need for transparency and human oversight | Zendesk, Dante AI |
Cost Reduction | Customer service cost reductions up to 25% | Desk365.io, 2025 |
Customer Satisfaction | Significant improvements and reduced escalation rates | Intercom, Salesforce case studies |
Agent Productivity | Increased productivity and reduced burnout by offloading repetitive tasks to AI | Various sources |
Foundations of AI in Customer Service: Architecture, Models, and Capabilities
Foundations of AI in Customer Service: Architecture, Models, and Capabilities
What drives the intelligence behind modern chatbots and virtual assistants? To fully appreciate their transformative role in customer service, it’s essential to explore the core AI technologies and architectural frameworks powering these systems. Let’s lift the veil on the key components—natural language processing (NLP), generative AI models, intent recognition, dialogue management—and understand how they integrate with backend systems to deliver seamless, human-like support.
Core AI Technologies Powering Conversational Agents
At the foundation of every smart chatbot lies Natural Language Processing (NLP). Moving far beyond simple keyword matching, NLP enables machines to understand and generate human language in a natural, personalized manner. Reflecting its critical role in digital transformation, the global NLP market is projected to reach nearly $157 billion by 2030.
Modern NLP depends heavily on deep learning, especially transformer-based large language models (LLMs) like GPT-4. These models have revolutionized how AI understands context, nuance, and intent by processing entire sentences or conversations simultaneously, unlike earlier sequential methods. Imagine transformers as expert editors scanning a manuscript in one sweep, spotting key themes and references to generate coherent, context-aware responses.
Supporting NLP are intent recognition systems that interpret the purpose behind a customer’s message. These systems accurately classify user intent even when inputs are ambiguous, typo-ridden, or lack clear keywords. Think of this as a skilled concierge who deciphers vague requests and directs customers appropriately. Since most chatbots operate within predefined intents trained on historical data, enhancing intent recognition accuracy directly improves chatbot effectiveness and customer satisfaction.
Behind the curtain, dialogue management systems orchestrate the conversation flow—deciding when to ask clarifying questions, provide answers, or escalate to human agents. This component acts as the conversation’s conductor, ensuring smooth transitions and coherent exchanges that feel natural to users.
Architectural Components: From Knowledge Bases to Machine Learning Pipelines
Developing an effective chatbot involves more than just AI models; it requires a robust ecosystem. A vital architectural element is the AI-powered knowledge base. Unlike static FAQs, these dynamic repositories leverage NLP and machine learning to quickly surface relevant information and even generate content on demand. Platforms like Guru and Korra embed AI-driven search engines that recommend precise answers, reducing average call times and boosting customer satisfaction.
This knowledge base works hand in hand with machine learning pipelines—automated workflows that handle data ingestion, model training, evaluation, and deployment. These pipelines ensure consistent performance, scalability, and continuous improvement by retraining models on fresh data. Automation here is crucial: tasks that once took weeks can now be completed in hours, enabling chatbots to evolve with changing customer behavior.
Equally important is seamless integration with backend enterprise systems such as CRM, ERP, and supply chain management platforms. This connectivity allows chatbots to access personalized customer data, check order statuses, process returns, or trigger workflows in real time. For example, H&M’s virtual assistant Erica leverages such integrations to deliver tailored shopping support, enhancing the customer experience.
Demystifying Transformer-Based Large Language Models: Strengths and Limitations
The term “transformer” might sound technical, but it can be grasped through analogy. Imagine a large language model as a vast library filled with countless books (training data). When given a prompt, the model functions like a hyper-efficient librarian who not only retrieves relevant references but also synthesizes new content by predicting what text should come next.
This predictive power is enabled by self-attention mechanisms, which weigh the importance of each word relative to others within the input. For example, in the sentence, “When Mary and John went to the store, John gave a drink to…,” attention heads help the model recall “Mary” as the likely recipient, ensuring coherent predictions.
However, transformers are not without limitations. Their dependence on massive datasets means they can inadvertently reproduce biases or inaccuracies embedded in training data. Additionally, they lack genuine understanding—producing plausible outputs based on statistical patterns rather than true comprehension. This can lead to occasional errors, especially in nuanced or highly specialized customer queries.
Furthermore, while LLMs excel at generating fluent and contextually relevant text, they may struggle with maintaining long-term conversational memory or managing tasks that require real-time data updates unless augmented with retrieval systems connected to live databases.
Connecting the Dots: Why These Foundations Matter for Customer Service
The synergy of NLP, intent recognition, dialogue management, and backend integration forms the backbone of AI-driven customer support. These technologies empower chatbots to autonomously resolve up to 69% of customer issues, reducing operational costs by as much as 30% while improving response times and satisfaction.
Yet, the most effective solutions employ hybrid approaches—where AI handles routine inquiries and human agents focus on complex, sensitive issues. For this to succeed, architectures must support smooth escalation paths and continuous learning, adapting to evolving customer needs.
Experience shows that organizations investing in strong AI pipelines, maintaining rich, up-to-date knowledge bases, and clearly defining chatbot intents position themselves to achieve substantial ROI. At the same time, vigilance around ethical considerations, transparency, and responsible AI use remains paramount to building lasting customer trust.
The foundation of AI in customer service is a sophisticated blend of advanced models and thoughtful engineering. A deep understanding of these building blocks equips businesses to deploy chatbots and virtual assistants that are not only efficient but also genuinely helpful and trustworthy.
Component | Description | Examples / Details | Impact on Customer Service |
---|---|---|---|
Natural Language Processing (NLP) | Enables machines to understand and generate human language naturally | Deep learning, transformer-based LLMs like GPT-4 | Improves personalized, context-aware interactions; supports digital transformation |
Intent Recognition | Interprets the purpose behind customer messages even if ambiguous or typo-ridden | Classifies user intent using historical data | Enhances chatbot effectiveness and customer satisfaction |
Dialogue Management | Manages conversation flow, deciding questions, answers, escalation | Orchestrates smooth, coherent exchanges | Ensures natural-feeling conversations and smooth escalations |
AI-Powered Knowledge Base | Dynamic repositories leveraging NLP and ML to surface relevant info | Platforms like Guru, Korra with AI-driven search engines | Reduces call times, boosts satisfaction by precise answers |
Machine Learning Pipelines | Automated workflows for data ingestion, model training, deployment | Enables fast retraining and continuous improvement | Improves scalability and adaptability of chatbots |
Backend Integration | Connects chatbots to CRM, ERP, supply chain systems | Example: H&M’s Erica virtual assistant | Provides personalized support, real-time workflows |
Transformer-Based LLMs | Models using self-attention to predict coherent, context-aware text | Large training datasets, self-attention mechanisms | Generates fluent responses but may reproduce biases or errors |
Hybrid AI-Human Approach | Combines AI for routine tasks with human agents for complex issues | Supports smooth escalation and continuous learning | Improves resolution rates and customer trust |
Performance Metrics | Effectiveness indicators of AI in customer service | Resolves up to 69% of issues; reduces costs by 30% | Reduces operational costs; improves response times and satisfaction |
Performance Metrics and Technical Challenges: Accuracy, Scalability, and Error Handling
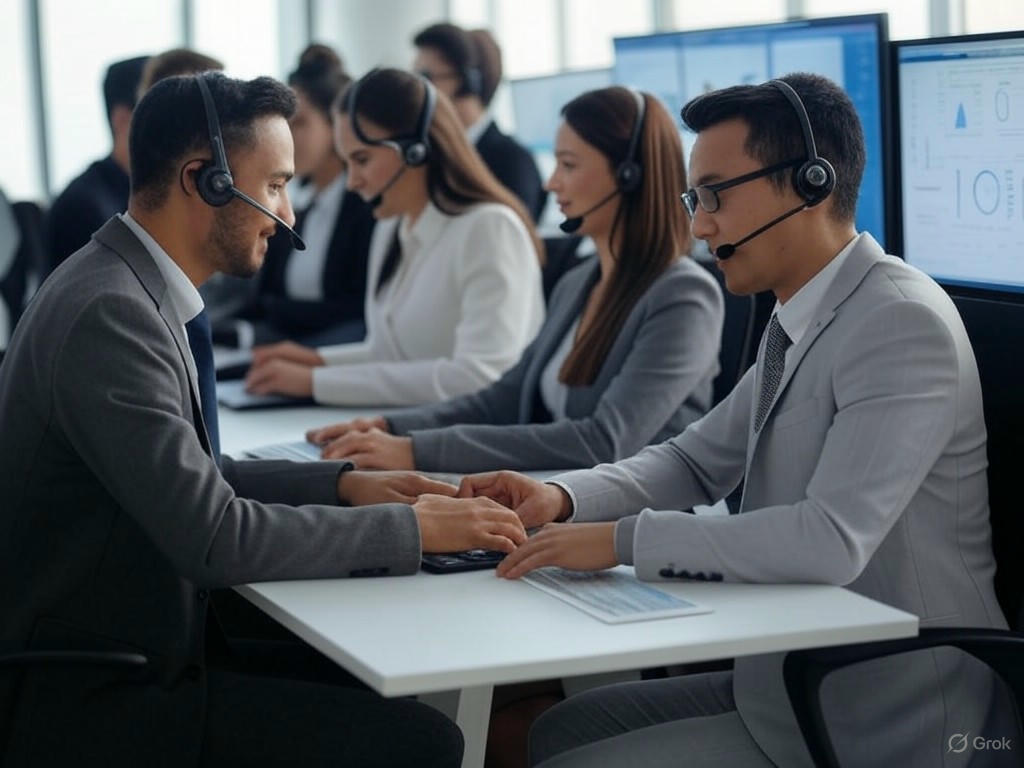
Performance Metrics and Technical Challenges: Accuracy, Scalability, and Error Handling
How can we accurately assess the effectiveness of AI chatbots and virtual assistants in customer service? Beyond the initial excitement around automation and instant response, true success depends on measurable performance and the system’s ability to manage complex, real-world interactions reliably.
Measuring Chatbot Performance
Deploying a chatbot is just the beginning. Evaluating its impact requires a comprehensive look at several critical metrics that reflect both efficiency and user experience:
-
Response Accuracy: This measures how often chatbot replies are relevant, coherent, and factually correct. Confident AI defines “conversation relevancy” as the proportion of relevant responses within a dialogue, while “knowledge retention” gauges how well the chatbot maintains context over multiple conversational turns. Both are essential for natural, multi-step interactions.
-
Resolution Time and Average Handle Time (AHT): The speed at which a chatbot resolves customer inquiries directly influences satisfaction and operational costs. Calabrio’s Bot Analytics highlights AHT as a key performance indicator, helping organizations optimize call center workflows and resource allocation.
-
User Satisfaction Scores (CSAT) and Net Promoter Scores (NPS): These subjective metrics capture the customer’s overall experience, indicating how well the chatbot meets expectations. Industry analysts like Nova Quinn emphasize that continuous measurement and improvement of user satisfaction are crucial for long-term chatbot success.
-
Containment Rate and First Contact Resolution (FCR): These metrics assess the chatbot’s ability to resolve issues without escalating to human agents. Gartner projects that by 2025, AI chatbots will handle up to 70% of customer interactions, making high containment and FCR rates vital for achieving scalable support.
-
Scalability and Cost per Automated Interaction: As chatbots manage growing volumes, their performance must scale without degradation. Cost efficiency is equally important—according to Upskillist, AI-powered chatbots can reduce the cost per interaction from approximately $19.50 (human agents) to $0.50–$0.70, delivering significant savings.
Real-world implementations demonstrate these benefits. Salesforce reported a 56% reduction in escalation rates after deploying generative AI chatbots, while Databricks achieved a 20% boost in customer satisfaction, underscoring AI’s potential to enhance both efficiency and experience.
Technical Challenges: Ambiguity, Multilingual Support, and Error Management
Despite rapid progress, AI chatbots continue to face technical hurdles that can impact reliability and user trust.
-
Handling Ambiguous or Complex Queries: Natural language is rich with nuance, idioms, and slang, which can confuse even advanced NLP systems. Novasarc’s global survey found that 50% of consumers frequently experience frustration with chatbots, often due to misinterpretation or inability to handle unpredictable inputs.
-
Multilingual Support: In today’s globalized market, providing real-time assistance across multiple languages is essential. Companies like Powerling leverage AI-driven translation and localization to break down language barriers, while virtual assistants such as those from Wishup enable cost-effective global customer engagement.
-
Error Propagation in Generative AI Responses: Generative models are powerful but prone to “hallucinations”—plausible-sounding but incorrect or misleading answers. This risk amplifies during complex, multi-turn dialogues where reasoning across context is required. TenUpSoft highlights the importance of architectural safeguards to detect and contain such errors before they affect customers.
-
Contextual Memory and Dialogue Management: Maintaining conversation coherence over extended interactions remains challenging. Effective dialogue management balances remembering past exchanges with adapting to new inputs, demanding sophisticated system design and continuous model training.
Mitigating Risks: Guardrails, Fallbacks, and Regulatory Compliance
As chatbots assume greater responsibility, risks related to misinformation, bias, and legal liability escalate. Thoughtful system design and compliance frameworks are essential to manage these challenges.
-
Ethical Guardrails: Guardrails constrain chatbot behavior to prevent biased, harmful, or legally problematic outputs. Experts like Renu Khandelwal advocate for continuous monitoring and adaptive controls to align AI behavior with ethical standards and corporate policies.
-
Fallback Mechanisms: When a chatbot encounters uncertainty or cannot confidently address a query, seamless escalation to human agents or default safe responses is critical. Callin.io stresses that fallback mechanisms preserve accuracy and maintain customer trust by ensuring complex or sensitive issues receive human attention.
-
Regulatory Compliance: The evolving legal landscape demands transparency, data protection, and accountability. For example, Utah’s AI Policy Act (Utah HB0452) mandates these principles for AI systems, particularly in sensitive domains like mental health services. Legal experts at Davis Polk emphasize that such regulations require auditable decision trails and proactive error mitigation strategies to reduce risks.
-
Balancing Automation with Human Oversight: A hybrid approach remains the safest and most effective strategy. Enkrypt AI recommends combining AI efficiency with human judgment to mitigate risks from AI hallucinations and ensure adherence to ethical and regulatory frameworks.
Summary
Evaluating chatbot performance requires a multi-faceted approach—including accuracy, speed, user satisfaction, containment, and scalability. While AI chatbots excel at handling routine inquiries efficiently, challenges persist in managing ambiguous language, supporting multiple languages, and controlling error propagation in generative models.
Implementing robust guardrails, fallback strategies, and complying with emerging regulations such as Utah’s AI Policy Act are vital steps to reduce misinformation risks and build user trust. As AI technologies mature, the future of customer service will depend on harmonizing technological capabilities with ethical considerations, human oversight, and regulatory compliance. The potential is transformative—but so too is the responsibility.
Metric | Description | Importance / Impact | Example / Source |
---|---|---|---|
Response Accuracy | Measures relevance, coherence, and factual correctness of chatbot replies; includes conversation relevancy and knowledge retention. | Essential for natural, multi-step interactions. | Confident AI defines conversation relevancy and knowledge retention. |
Resolution Time and Average Handle Time (AHT) | Speed at which chatbot resolves inquiries. | Influences customer satisfaction and operational costs. | Calabrio’s Bot Analytics highlights AHT as key KPI. |
User Satisfaction Scores (CSAT) and Net Promoter Scores (NPS) | Subjective metrics capturing overall customer experience. | Indicates how well chatbot meets expectations; crucial for long-term success. | Nova Quinn emphasizes continuous measurement and improvement. |
Containment Rate and First Contact Resolution (FCR) | Ability to resolve issues without escalation to human agents. | Vital for scalable support; Gartner projects AI chatbots handling 70% of interactions by 2025. | Gartner projections. |
Scalability and Cost per Automated Interaction | Performance must scale with volume; cost efficiency per interaction. | Reduces operational expenses significantly. | Upskillist reports cost reduction from $19.50 to $0.50–$0.70 per interaction. |
Escalation Rate Reduction | Reduction in issues escalated to human agents. | Indicates improved chatbot effectiveness. | Salesforce reported 56% reduction post AI chatbot deployment. |
Customer Satisfaction Improvement | Increase in customer satisfaction levels. | Reflects enhanced experience. | Databricks achieved 20% boost in satisfaction. |
Technical Challenge | Description | Impact | Example / Source |
---|---|---|---|
Handling Ambiguous or Complex Queries | Difficulty interpreting nuance, idioms, slang in natural language. | Leads to user frustration and misinterpretations. | Novasarc survey: 50% consumers frequently frustrated. |
Multilingual Support | Providing real-time assistance in multiple languages. | Essential for global customer engagement. | Powerling and Wishup use AI-driven translation/localization. |
Error Propagation in Generative AI Responses | Risk of hallucinations—plausible but incorrect answers. | Reduces reliability, risks misinformation. | TenUpSoft stresses need for architectural safeguards. |
Contextual Memory and Dialogue Management | Maintaining conversation coherence over extended interactions. | Necessary for natural dialogue flow. | Requires sophisticated design and continuous training. |
Mitigation Strategy | Description | Purpose | Example / Source |
---|---|---|---|
Ethical Guardrails | Constraints to prevent biased, harmful, or illegal outputs. | Aligns AI behavior with ethical standards and policies. | Renu Khandelwal advocates continuous monitoring and adaptive controls. |
Fallback Mechanisms | Escalation to human agents or safe default responses when uncertain. | Preserves accuracy and customer trust. | Callin.io stresses importance of seamless fallback. |
Regulatory Compliance | Adhering to transparency, data protection, and accountability laws. | Reduces legal risks and ensures auditable decisions. | Utah HB0452 AI Policy Act; Davis Polk legal experts. |
Balancing Automation with Human Oversight | Combining AI efficiency with human judgment. | Mitigates AI hallucination risks; enforces ethical and regulatory adherence. | Enkrypt AI recommends hybrid approach. |
Human-AI Collaboration: Balancing Automation with Empathy and Expertise
Human-AI Collaboration: Balancing Automation with Empathy and Expertise
How can organizations strike the right balance between AI efficiency and human empathy in customer service? The key lies in task segmentation—assigning routine, repetitive queries to AI chatbots while reserving complex, sensitive interactions for human agents who bring empathy and expertise.
Task Segmentation: Defining Roles for AI and Humans
AI-powered chatbots excel at managing high-volume, straightforward tasks such as order tracking, frequently asked questions, and appointment scheduling. These functions leverage advanced conversational AI capabilities that operate 24/7 without fatigue or downtime. For instance, platforms like Zendesk’s AI agents and Zoho SalesIQ’s Zobot efficiently handle common inquiries, significantly deflecting routine questions and freeing human agents to focus on higher-value interactions.
However, complexity arises quickly beyond these basic queries. When customers encounter sensitive issues—such as billing disputes, emotional support needs, or multi-step problem-solving—human agents are essential. Their nuanced judgment and empathetic communication preserve the quality of the customer experience where AI alone falls short.
A useful analogy is an airport security checkpoint: automated scanners rapidly process standard baggage checks, but human officers intervene when irregularities or suspicious situations arise. Similarly, AI systems triage and resolve routine requests, escalating exceptions to human agents. This hybrid approach allows businesses to scale support efficiently without sacrificing personalized care.
Workforce Impacts and Agent Upskilling
Adopting AI in customer service does not replace human agents; instead, it augments their capabilities. Research from McKinsey (2025) shows that most employees are ready to embrace AI tools, but successful adoption depends on leadership commitment to training and cultural change.
Customer service agents evolve into “knowledge curators,” leveraging AI-powered insights to deliver faster, more accurate responses. Training programs increasingly emphasize blending traditional soft skills—such as empathy and communication—with AI literacy. For example:
- AI tools can provide real-time suggestions and next-best-actions, enabling agents to focus on rapport-building instead of searching through knowledge bases.
- Virtual collaboration platforms, including virtual whiteboards and multi-agent AI systems, facilitate seamless coordination between human agents and specialized AI assistants handling analytics, sentiment detection, or workflow automation.
This integrated ecosystem fosters resilience and operational flexibility, as highlighted in Deloitte’s research on AI-human teamwork.
Ethical Considerations: Transparency and Building Customer Trust
Ethical AI deployment is foundational to maintaining sustainable customer trust. Customers increasingly expect transparency about when they are interacting with AI versus human agents. Clear disclosure respects customer autonomy and sets realistic expectations regarding AI’s abilities and limitations.
Explainability is also critical. AI systems should provide clear rationales for decisions—such as refund denials based on policy—rather than generic or opaque responses. This helps reduce frustration and builds confidence in automated support.
Data privacy and bias mitigation remain top concerns. With AI models trained on extensive customer data, companies must ensure compliance with regulations like the EU’s GDPR and emerging frameworks such as the EU AI Act. PwC’s findings reveal that 85% of consumers trust companies that use AI ethically, underscoring the competitive advantage of responsible AI governance.
Integrating Support Workflows and Visual Engagement Tools
Human-AI collaboration extends beyond chat windows. Emerging visual engagement tools—including co-browsing, augmented reality overlays, and interactive virtual assistants—enhance the quality and effectiveness of customer support.
Imagine a customer struggling with software installation: an AI assistant monitors the conversation, suggesting troubleshooting steps, while a human agent joins via co-browsing to guide more complex configurations. This smooth handoff between AI and human expertise creates a richer, more satisfying support experience.
Platforms like Startek’s hybrid support system exemplify this synergy, combining AI-driven efficiency with a personalized human touch. Recognized with industry awards, such integrated workflows empower agents with AI-assisted knowledge while preserving the empathy and judgment that only humans can provide.
In summary, the optimal customer service model in 2025 and beyond is not AI versus humans, but AI plus humans. By thoughtfully segmenting tasks, investing in agent upskilling, maintaining transparency, and adopting interactive tools, organizations can deliver support that is both efficient and deeply human. This balanced approach cuts through the hype, recognizing AI’s transformative capabilities while honoring the irreplaceable value of human empathy and expertise.
Aspect | Details |
---|---|
Task Segmentation | AI handles routine queries (order tracking, FAQs, scheduling); Humans handle complex, sensitive issues (billing disputes, emotional support, multi-step problems) |
Examples of AI Platforms | Zendesk’s AI agents, Zoho SalesIQ’s Zobot |
Workforce Impact | AI augments human agents; agents become knowledge curators; training blends empathy and AI literacy |
Agent Upskilling Tools | Real-time suggestions, next-best-actions, virtual whiteboards, multi-agent AI systems |
Ethical Considerations | Transparency about AI use, explainability of AI decisions, data privacy compliance (GDPR, EU AI Act), bias mitigation |
Customer Trust | 85% trust companies using AI ethically (PwC) |
Visual Engagement Tools | Co-browsing, augmented reality overlays, interactive virtual assistants |
Example of Integrated Support | Startek’s hybrid support system combining AI efficiency with human empathy |
Overall Model | AI plus humans, balancing efficiency with empathy and expertise |
Ethical and Privacy Considerations in Deploying AI Chatbots
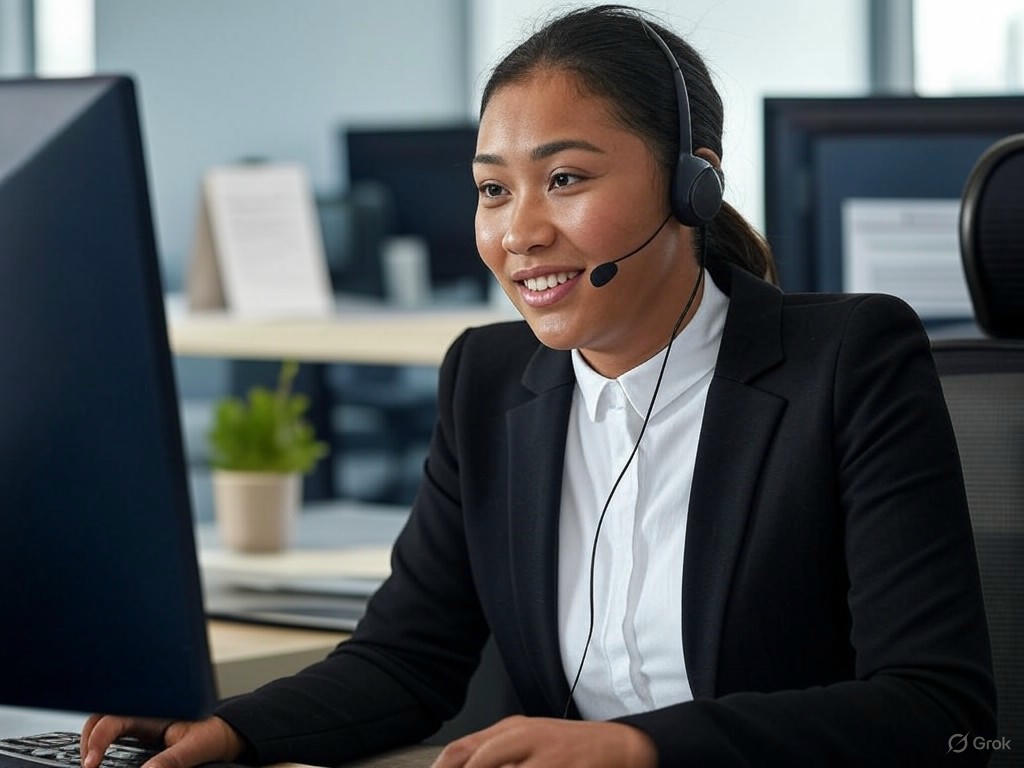
Ethical and Privacy Considerations in Deploying AI Chatbots
AI chatbots have transformed customer service by delivering instant, scalable support around the clock. Yet, beneath this seamless experience lie critical ethical and privacy challenges that businesses must proactively address to maintain trust and compliance.
Transparency and Informed Consent: Building the Foundation of Trust
Customers increasingly expect to know when they are interacting with AI, not a human agent. Undisclosed AI interactions raise ethical concerns and are becoming legal liabilities. For instance, California’s recent lawsuits and North Carolina’s Senate Bill 624 mandate clear disclosure of AI chatbot involvement and require explicit informed consent before engagement.
Transparency involves more than just announcing “you are talking to a bot.” It includes:
- Clear disclosure at the conversation’s start that the agent is AI-powered.
- Plain-language explanations of the chatbot’s capabilities and limitations.
- Offering easy options for escalation to human agents, particularly for complex or sensitive issues.
- Regular updates to disclosures as AI functionality and data policies evolve.
This ongoing transparency aligns with consumer expectations. According to Zendesk’s 2024 CX Trends report, 59% of consumers anticipate AI reshaping their interactions within two years, but 63% express concern about bias and fairness. Transparent communication helps prevent “chatbot fatigue,” where customers feel trapped in automated loops without recourse to human support.
Data Privacy and Security: Protecting Sensitive Customer Information
Chatbots routinely process sensitive personal data—from account details to health information—making them prime targets for cyberattacks. The consequences of data breaches are severe: eroding customer trust and attracting regulatory penalties.
Security forecasts are sobering: by 2025, 93% of organizations expect daily AI-driven cyberattacks (Gartner, 2024 AI Security Survey). To mitigate these risks, companies must integrate robust security practices into chatbot design, including:
- Data minimization: Collect only the information essential for the interaction.
- Encryption: Secure data both in transit and at rest to prevent unauthorized access.
- Strong authentication and authorization: Ensure only legitimate users and processes interact with the system.
- Anonymization and de-identification: Comply with laws like North Carolina Senate Bill 624 to protect user identity.
- Regular security audits and vulnerability assessments: Identify and address emerging threats proactively.
- Incident response plans: Enable rapid containment and mitigation of any breaches.
Compliance with a complex regulatory landscape is also essential. The California Invasion of Privacy Act requires explicit written consent before analyzing voice recordings, while the FCC treats AI-generated calls as robocalls under its rules. Moreover, biometric data use in AI raises additional privacy concerns, prompting specific state laws such as Washington’s biometric consent statute.
Implementing these safeguards is not merely about legal adherence. It’s a strategic imperative to preserve user confidence in an era where 73% of consumers worry about their data privacy when interacting with chatbots (SmythOS). Proactive privacy measures build long-term brand loyalty.
Addressing Bias and Ensuring Ethical AI Behavior
Fairness remains a core ethical challenge in AI chatbot deployment. AI models inherit biases from their training data, developer assumptions, and algorithmic design. A chatbot trained primarily on one demographic’s language may misunderstand or misrepresent others, leading to unfair or even offensive interactions.
Unchecked bias can degrade service quality for certain groups, expose companies to legal risks, and damage reputations. Mitigating bias requires a comprehensive approach:
- Using diverse, representative training datasets to encompass broad user demographics.
- Incorporating human-in-the-loop evaluations by diverse teams to detect and correct biased outputs.
- Designing chatbots to grasp nuanced user contexts rather than defaulting to generic responses.
- Establishing clear ethical guidelines and accountability frameworks.
- Empowering users with feedback channels to report problematic behaviors.
Regulators like the Federal Trade Commission (FTC) are increasing scrutiny over AI’s potential for bias and misinformation. Firms that invest in fairness and transparency not only reduce legal exposure but also gain competitive advantage by delivering inclusive, trustworthy customer experiences.
Architectural Strategies to Enforce Compliance and Safeguard Consumer Rights
Technology itself can embed compliance and ethical guardrails. Modern chatbot architectures incorporate controls such as:
- Limiting chatbot scope to reduce hallucinations and false information.
- Automatically detecting and escalating ambiguous or high-risk queries to human agents.
- Enforcing strict data retention policies aligned with GDPR, CCPA, and the EU AI Act.
- Applying cryptographic techniques to secure data flows and prevent tampering.
- Maintaining detailed, privacy-respecting logs for auditing and accountability.
For example, dialog management systems monitor conversation flow in real time to ensure compliance with ethical standards. This design philosophy treats AI chatbots as assistants—not replacements—preserving essential human oversight where it matters most.
In summary, deploying AI chatbots in customer service involves balancing innovation with responsibility. Transparent disclosure and informed consent foster trust. Rigorous data privacy and security safeguards protect customers and comply with evolving regulations. Active bias mitigation ensures fairness and ethical integrity. Companies embedding these principles into their AI strategies are poised to build trustworthy, human-centric support experiences that meet both regulatory demands and rising customer expectations.
Consideration | Key Points | Examples / Regulations | Best Practices |
---|---|---|---|
Transparency and Informed Consent |
|
|
|
Data Privacy and Security |
|
|
|
Bias and Ethical AI Behavior |
|
|
|
Architectural Strategies for Compliance |
|
|
|
Comparative Analysis: AI Chatbots Versus Traditional Customer Support Models
Comparative Analysis: AI Chatbots Versus Traditional Customer Support Models
How do AI chatbots and virtual assistants compare to traditional human-only customer support? This question is central to many businesses’ strategic plans in 2025, as efficiency, scalability, and rising customer expectations intersect. Let’s examine the evidence to cut through the hype and understand where AI excels and where human expertise remains essential.
Cost Efficiency and ROI: AI Chatbots and Virtual Assistants Surpass Traditional Support
For organizations prioritizing operational cost reduction, AI-powered chatbots and virtual assistants offer compelling financial benefits. Companies adopting AI-driven customer service report an average 25% reduction in service costs (Desk365, 2025), a significant saving when scaled globally.
Key highlights include:
- Strong ROI: For every $1 invested in AI, companies achieve an average return of $3.50, with some reporting returns up to $8 (Desk365, 2025).
- 24/7 operation: Chatbots provide round-the-clock service, eliminating the need for costly night shifts or overtime while efficiently handling routine inquiries at scale.
- Lower overhead: AI reduces expenses related to salaries, benefits, office space, and equipment compared to human agents (OutsourcingAngel, 2025).
- Flexible virtual assistants: Outsourced virtual assistants combine human skills with scalability and cost savings of up to $30,500 annually per full-time employee equivalent, without long-term hiring commitments (OutsourcingAngel, 2025).
A notable example is Yum! Brands’ AI deployment at drive-thrus, where AI systems increased order processing speed by 10–15% and improved accuracy, delivering both cost savings and revenue growth (Desk365, 2025). This illustrates AI’s capacity to streamline operations while enhancing service quality.
In contrast, traditional human-only support models face high labor costs and limited scalability. Outsourcing can ease some expenses but still requires ongoing human resource management and offers less predictable scalability.
Response Speed and Scalability: AI Enables Instant, Around-the-Clock Support
One of AI chatbots’ most visible advantages is speed. By 2025, AI systems are projected to handle up to 95% of all customer interactions instantly, eliminating wait times typical of human agents constrained by shifts and call volumes (Desk365, 2025).
Key factors include:
- 24/7 availability across multiple channels—web, apps, social media, and voice—ensuring seamless access anytime (Zendesk, 2025).
- Inherent scalability: AI can simultaneously engage thousands of customers without additional cost or performance loss (Kodif, 2025).
- Proactive personalization: AI-driven predictive analytics and sentiment analysis enable scalable, tailored customer experiences (DevRev, 2025; NICE, 2025).
However, speed alone doesn’t guarantee effectiveness. Complex or emotionally sensitive issues often require human empathy and nuanced judgment. AI chatbots excel at routine tasks such as FAQs, order tracking, and appointment scheduling but may struggle with ambiguous or delicate situations.
Customer Satisfaction: Combining AI Efficiency with Human Empathy
Customer satisfaction blends speed, accuracy, personalization, and emotional connection. AI’s impact here is promising but nuanced.
Insights include:
- Widespread AI adoption: 80% of companies use AI to enhance customer experience, with many customers appreciating faster responses and 24/7 support (Desk365, 2025).
- Preference for humans in complex cases: 52% of customers still prefer human interaction for complex issues, and 59% of support professionals recommend a human-led support approach (HiverHQ, 2025).
- Transparency expectations: 58% of support agents believe customers should be informed when AI is involved (HiverHQ, 2025).
- High AI accuracy: Well-trained chatbots deliver context-aware responses that raise satisfaction. Vodafone UK’s TOBi chatbot, handling over 1 million monthly interactions, receives positive feedback (Japeto AI, 2025).
- Risks of poor AI experiences: 32% of customers abandon a brand after a single bad AI interaction (AmplifAI, 2025).
The consensus favors a hybrid model: AI efficiently manages routine inquiries, while humans handle complex, emotional, or escalated issues (Nextiva, 2025; McKinsey, 2025). This synergy improves satisfaction and optimizes resource allocation.
Situational Effectiveness: When AI Excels and When Human Intervention Is Essential
AI chatbots and virtual assistants transform specific customer service contexts but do not fully replace humans.
Where AI excels:
- Routine, repetitive tasks such as FAQs, order updates, appointment scheduling, and basic troubleshooting (Kodif, 2025; Japeto AI, 2025).
- High-volume, low-complexity interactions, managing demand surges without wait times or extra staffing (Desk365, 2025).
- Data-driven personalization by integrating with CRM and knowledge bases for tailored recommendations and reminders (BAI.org, 2025).
- Providing extended hours and global reach, supporting customers across time zones without fatigue (KineticStaff, 2025).
Where human intervention is critical:
- Complex problem-solving that requires judgment, creativity, or coordination across departments (McKinsey, 2025).
- Emotional intelligence and empathy in complaint handling, sensitive issues, and trust-building (HiverHQ, 2025).
- Ethical decision-making to prevent bias and ensure fairness in AI-driven responses (Zendesk, 2025).
- Quality assurance and training, where humans curate AI training data to maintain accuracy and brand voice (Infomineo, 2025).
While generative AI is advancing toward simulating personality and conversational nuance more akin to humans (CMSWire, 2025), the unpredictable nature of human communication and ethical challenges mean fully autonomous AI support is not yet feasible.
Key Takeaways
-
AI chatbots and virtual assistants deliver substantial cost savings and scalability benefits over traditional human-only support, backed by strong ROI across industries.
-
Speed and 24/7 availability are AI’s core strengths, enabling instantaneous handling of high volumes of routine queries.
-
Customer satisfaction improves when AI and humans collaborate—AI manages simple tasks, while humans address complex, sensitive interactions.
-
Situational awareness is crucial: AI excels in structured, predictable scenarios, but human judgment remains vital for nuanced or ethical cases.
-
The future of customer service lies in hybrid models that leverage AI efficiency without sacrificing the empathy and critical thinking unique to humans.
For organizations shaping their 2025 customer service strategies, adopting AI means amplifying human impact, not replacing it. Success requires thoughtful integration, ongoing training, and transparent communication to thrive in the evolving AI-powered customer experience landscape.
Aspect | AI Chatbots and Virtual Assistants | Traditional Human-Only Support |
---|---|---|
Cost Efficiency and ROI | 25% reduction in service costs; ROI of $3.50 per $1 invested; lower overhead costs; cost savings up to $30,500 per FTE annually | High labor costs; ongoing HR management; less predictable scalability |
Response Speed and Scalability | Handles up to 95% of interactions instantly; 24/7 availability; scalable to thousands of customers simultaneously; proactive personalization | Limited by shifts and call volumes; no 24/7 coverage without extra costs; scaling requires more staff |
Customer Satisfaction | 80% AI adoption; faster responses; positive feedback on accuracy; risk of customer abandonment after poor AI interactions; transparency expectations | Preferred by 52% of customers for complex issues; valued for empathy and nuanced judgment; recommended by 59% of support professionals |
Situational Effectiveness | Excels at routine, repetitive tasks, high-volume low-complexity interactions, data-driven personalization, extended hours | Essential for complex problem-solving, emotional intelligence, ethical decision-making, quality assurance and training |
Operational Model | Hybrid approach favored: AI manages routine tasks, humans handle complex/emotional issues | Human-led support recommended for complex and sensitive cases |
Future Directions: Emerging Trends and the Evolving Landscape of AI in Customer Service
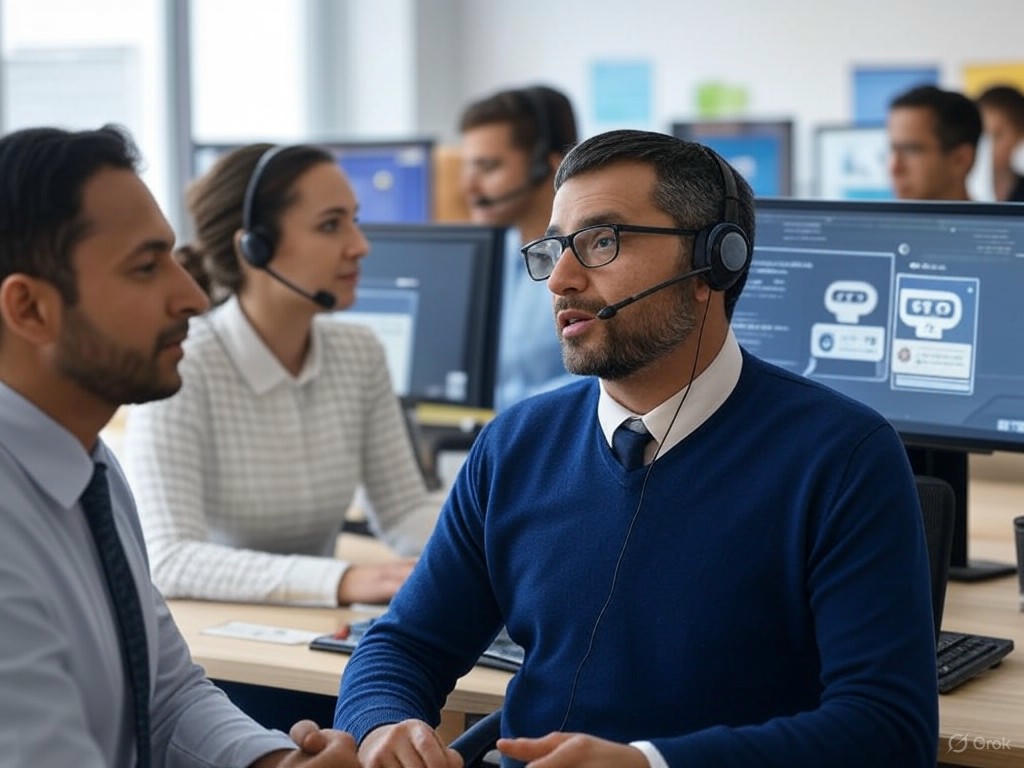
Future Directions: Emerging Trends and the Evolving Landscape of AI in Customer Service
Imagine a future where the chatbot you interact with not only answers your questions but also senses your frustration, anticipates your needs, and effortlessly switches between chat, voice, email, and social media without missing a beat. This vision is rapidly becoming reality as AI in customer service evolves toward more emotionally intelligent, omnichannel, and continuously learning systems by the mid-2020s.
Emotional Intelligence and Continual Learning: Chatbots That Understand and Evolve
Traditional chatbots, often limited to scripted responses, are being replaced by AI-powered agents with genuine emotional intelligence. By 2027, these chatbots will harness advanced sentiment analysis and emotion AI to interpret customer moods such as frustration, excitement, or distress. This goes far beyond keyword detection—it involves contextual understanding of tone, pace, and subtle linguistic nuances.
A compelling example is DevRev’s Turing chatbot, which integrates predictive analytics with AI-driven sentiment gauges to create personalized, human-like conversations. This approach not only elevates customer satisfaction but also reduces employee burnout by empathetically automating routine queries.
At the heart of these advancements lies continual learning. Unlike static, pre-trained models, modern chatbots adapt in real time, refining their responses based on incoming data, customer feedback, and shifting language patterns. This ongoing learning cycle is critical for managing complex queries and unexpected conversational turns, boosting accuracy and relevance over time.
However, these innovations bring challenges. The quality, diversity, and ethical curation of training data are paramount. Biased or outdated datasets risk perpetuating harmful stereotypes or missing emerging trends. Maintaining rigorous evaluation and bias mitigation processes is essential to uphold fairness and trust.
Seamless Omnichannel Integration: AI as the Conductor of Customer Journeys
Customer service no longer occurs in isolated channels. Today’s consumers expect seamless experiences across chat, email, voice, social media, and even in-person interactions. AI’s role is evolving from isolated tools to orchestrators of connected omnichannel journeys.
Platforms like DevRev’s AI-native system unify customer insights across all touchpoints, equipping agents with a 360-degree real-time view of interactions, preferences, and pain points. This integration helps businesses reduce response times and deliver hyper-personalized support tailored to the customer’s immediate context.
According to Forbes, companies offering omnichannel services retain 89% of their customers, underscoring the competitive advantage of integrated experiences. By 2025, AI-driven customer journey mapping powered by machine learning will not only track but proactively optimize these journeys. AI will identify friction points, anticipate customer needs before they arise, and automate certain support steps to improve agility.
This integration extends to emerging technologies like the Internet of Things (IoT), where real-time device data streams feed AI systems to preempt issues or recommend products, further blurring the lines between service and experience.
Regulatory Landscape and Ethical Guardrails: Navigating Uncharted Waters
As AI becomes deeply embedded in customer service, regulatory scrutiny is intensifying. Recent U.S. legislation such as Virginia’s HB 2124 and New Jersey’s A 3540 impose criminal penalties for malicious use of AI-generated synthetic content. Montana’s Right to Compute Act mandates risk management policies for AI systems controlling critical infrastructure, signaling heightened concerns about accountability and security.
At the federal level, the White House’s AI Action Plan emphasizes transparency, privacy, and fairness, while a patchwork of state and federal regulations requires businesses to collaborate across IT, legal, and compliance teams. This regulatory complexity demands that organizations embed privacy-by-design and ethical AI principles into their workflows.
Ethical deployment of AI is more than compliance—it’s a trust imperative. Prioritizing data privacy and transparent AI practices fosters stronger, long-term customer relationships at a time when consumers are increasingly aware and wary of how their data is used.
Workforce Transformation: Reinventing Roles in an AI-Augmented Era
Contrary to fears of wholesale job displacement, AI in customer service is transforming roles rather than eliminating them. McKinsey research highlights that while almost all companies invest in AI, only 1% consider themselves advanced in AI maturity, indicating vast untapped potential to empower employees.
AI automates routine tasks, freeing human agents to focus on complex, emotionally nuanced interactions that require empathy and judgment. This shift elevates customer service professionals toward more strategic, empathetic, and creative problem-solving roles.
Furthermore, AI’s rise is spawning entirely new career paths. Agentic AI—capable of autonomous workflow orchestration—is creating demand for specialists who design, monitor, and optimize these systems. Cybersecurity experts, AI ethics officers, and compliance professionals are also expanding in response to evolving risks and regulations.
Emerging roles include:
- AI trainers and data curators who ensure models learn fairly and effectively.
- AI-human interaction designers crafting seamless, intuitive conversational experiences.
- Compliance officers navigating complex, evolving regulatory frameworks.
- AI-assisted customer experience strategists leveraging data-driven insights to enhance engagement.
For businesses, investing in workforce reskilling and fostering cross-functional collaboration is key to preparing employees to work with AI—not in competition with it.
Strategic Navigation: Balancing Opportunity with Prudence
The evolution of AI in customer service represents a strategic inflection point. Organizations that leverage AI’s capabilities in emotional intelligence, omnichannel integration, and continual learning can unlock unprecedented efficiency and deepen customer loyalty.
However, success requires thoughtful, balanced approaches:
- Embed AI transparently within existing workflows, maintaining human oversight to handle complex or sensitive cases.
- Stay abreast of evolving regulations to ensure ongoing compliance and ethical governance.
- Prioritize data quality and diversity to mitigate bias and enhance model robustness.
- Invest in workforce development to complement AI with human skills.
- Continuously measure impact, adapting strategies based on real-world feedback and customer outcomes.
By doing so, businesses can move beyond the hype to position AI as an enabler of genuine customer connection and resilient operations. The winners will be those who view AI not merely as a technology upgrade but as a holistic transformation in how they understand, engage, and support their customers.
Theme | Key Trends | Examples/Details | Challenges & Considerations |
---|---|---|---|
Emotional Intelligence & Continual Learning |
|
|
|
Seamless Omnichannel Integration |
|
|
|
Regulatory Landscape & Ethical Guardrails |
|
|
|
Workforce Transformation |
|
|
|
Strategic Navigation |
|
|
|