5 Small Businesses Thriving with AI: Real Lessons & Strategies
- Introduction: Why AI Matters for Small Businesses Now
- Introduction: Why AI Matters for Small Businesses Now
- The Economic Stakes: AI’s Growing Role in Small Business Success
- Barriers: The Real-World Hurdles Small Businesses Face
- Cutting Through the Hype: A Practical, Balanced Look Ahead
- Why This Matters Now
- Understanding AI Technologies Empowering Small Businesses
- Understanding AI Technologies Empowering Small Businesses
- Generative AI: The Creative Engine
- Machine Vision: Teaching Computers to See
- Predictive Analytics: Forecasting the Future
- Robotic Process Automation (RPA): Automating the Routine
- Performance Metrics and Practical Constraints
- Navigating Limitations with Strategic Adoption
- Five Small Businesses Winning with AI: In-Depth Case Studies
- Five Small Businesses Winning with AI: In-Depth Case Studies
- 1. Content Creation Reinvented: Jennifer’s Marketing Agency
- 2. Virtual Auditions at Scale: Allcasting’s AI-Powered Casting Platform
- 3. Marketing Optimization: A Local Retailer’s AI-Enhanced Campaigns
- 4. Inventory Management: A Specialty Food Store’s AI Leap
- 5. Investment Analytics: A Financial Advisory Startup’s AI Edge
- Common Themes and Lessons Learned
- Comparative Analysis: Benchmarking AI Adoption Across Small Business Models
- Comparative Analysis: Benchmarking AI Adoption Across Small Business Models
- Common Threads and Divergent Paths in AI Adoption
- Factors Driving AI Success in Small Businesses
- Measuring Impact: Efficiency, Customer Experience, and Competitive Advantage
- Synthesizing Insights: Key Takeaways for Small Businesses
- Ethical, Regulatory, and Data Governance Considerations for SMEs Using AI
- Ethical, Regulatory, and Data Governance Considerations for SMEs Using AI
- Navigating Ethical Dilemmas: Bias, Transparency, Privacy, and Consent
- Understanding the Regulatory Landscape Impacting SMEs
- Best Practices for Responsible AI Governance in SMEs
- Building Trust: The Competitive Edge for SMEs
- Future Trends and Emerging Opportunities: What Small Businesses Should Watch
- Future Trends and Emerging Opportunities: What Small Businesses Should Watch
- Revolutionary Advances in Generative AI: Lowering Barriers, Raising Stakes
- AI-as-a-Service and IoT: Synergizing for Operational Excellence
- The Evolving AI Ecosystem: Competition, Democratization, and Organizational Shifts
- Proactive Steps for Small Businesses: Preparing to Innovate and Lead
- Closing Thoughts
- Conclusion: Synthesizing Lessons and Strategic Recommendations for Small Businesses
- Conclusion: Synthesizing Lessons and Strategic Recommendations for Small Businesses
- Pragmatic Benefits and Real-World Challenges
- Strategic Recommendations for AI Adoption
- Balancing Enthusiasm with Critical Assessment
- Looking Ahead: AI’s Evolving Role in Small Business Futures
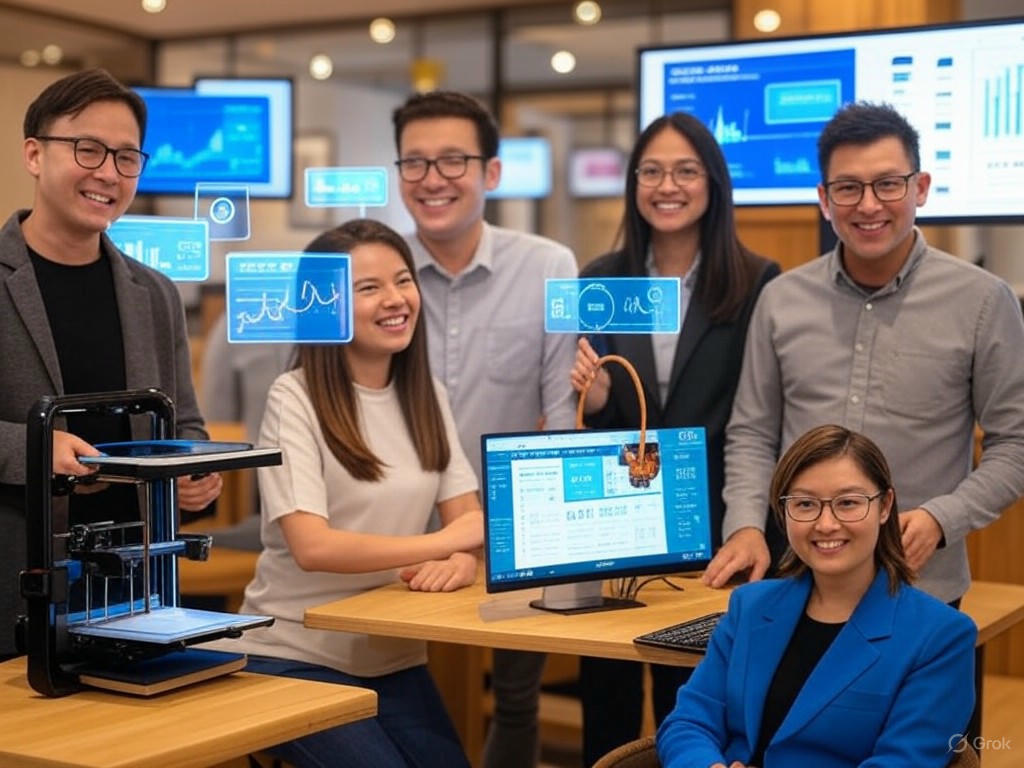
Introduction: Why AI Matters for Small Businesses Now
Introduction: Why AI Matters for Small Businesses Now
What if you knew that nearly eight out of ten small businesses have already started using AI? According to the 2025 Small Business AI Report by Service Direct, 77% of small businesses have adopted AI in some form. This widespread adoption signals that AI is not just a future trend but a present-day reality transforming how even modest operations drive growth, boost efficiency, and engage customers.
The Economic Stakes: AI’s Growing Role in Small Business Success
The economic impact of AI is immense. McKinsey estimates the global AI opportunity at $4.4 trillion, highlighting potential far beyond large corporations. For small and medium-sized businesses (SMBs) in the U.S., this could mean an annual productivity increase of up to 3.4 percentage points, according to ITIF research.
Further supporting this growth, Salesforce’s 2025 survey reports that 90% of SMBs using AI have seen revenue gains, and 78% plan to increase AI investments in the coming year. AI has evolved from a luxury to a competitive necessity for small businesses aiming to thrive in today’s market.
This momentum is reflected in spending patterns: small businesses typically invest over $10,000 annually on AI tools, focusing on sales, marketing, and customer support. These areas deliver quick, measurable wins. Startups and sole proprietors often begin with entry-level AI applications like AI-powered chatbots or email marketing automation, while more established firms scale into advanced AI solutions.
Barriers: The Real-World Hurdles Small Businesses Face
Despite the excitement, AI adoption comes with challenges. The biggest barrier is a lack of understanding — nearly 78% of non-AI adopters have no plans to experiment with AI, often due to budget constraints and limited technical expertise.
Implementing AI requires not only investment but also knowledge to choose, integrate, and maintain the right tools effectively. Security and trust are also major concerns. With AI systems handling sensitive data, risks like breaches and algorithmic bias are real and must be addressed.
Additionally, privacy and compliance requirements grow more complex as AI tools advance, which can intimidate small businesses that lack dedicated IT or data science staff.
There’s also a common misconception that generative AI or large language models will automatically solve every problem. The reality is more nuanced: success depends on aligning AI capabilities with clear business goals and maintaining human oversight to ensure responsible use.
Cutting Through the Hype: A Practical, Balanced Look Ahead
This article takes a grounded approach, focusing on five small businesses currently winning with AI. Through concrete case studies, we reveal practical lessons on what works, common pitfalls, and how ethical considerations such as data privacy and algorithmic fairness are essential to building trust with customers and employees.
We’ll highlight how these businesses have overcome budget and expertise limitations by starting with “high-impact, low-effort” AI applications—like automating routine customer inquiries with AI-powered chatbots or analyzing user behavior to inform product decisions. Each example demonstrates that AI should augment human decision-making, not replace it.
Alongside success stories, we emphasize ethical imperatives including transparency, fairness, and compliance. Small businesses must prioritize these to create sustainable and responsible AI adoption.
Why This Matters Now
AI is no longer exclusive to tech giants or companies with massive IT budgets. Cloud computing, AI-as-a-Service (AIaaS), and subscription-based models have democratized AI access, with some tools available for as little as $20 per month.
However, increased accessibility comes with responsibility—to understand AI’s limitations, invest thoughtfully, and embed ethical principles in design and deployment.
For small businesses, AI’s promise is transformational but requires pragmatic adoption strategies and a clear-eyed view of risks. This article aims to cut through the hype with evidence-based insights, making AI’s potential accessible to both technical and non-technical readers eager to leverage AI effectively without overlooking real-world constraints.
By learning from peers who have successfully harnessed AI, you’ll gain a practical roadmap to integrate this powerful technology into your small business thoughtfully, efficiently, and ethically.
Aspect | Details |
---|---|
AI Adoption Rate | 77% of small businesses (2025 Small Business AI Report by Service Direct) |
Global AI Opportunity | $4.4 trillion (McKinsey estimate) |
Productivity Increase for SMBs | Up to 3.4 percentage points annually (ITIF research) |
Revenue Gains from AI | 90% of SMBs using AI have seen revenue gains (Salesforce 2025 survey) |
Future AI Investment Plans | 78% of SMBs plan to increase AI investments |
Annual AI Spending | Typically over $10,000 on AI tools |
Common AI Applications | Sales, marketing, customer support, AI-powered chatbots, email marketing automation |
Biggest Barrier to AI Adoption | Lack of understanding; 78% of non-adopters have no plans to experiment |
Other Barriers | Budget constraints, limited technical expertise, security and trust concerns, privacy and compliance complexity |
Ethical Considerations | Data privacy, algorithmic fairness, transparency, compliance |
AI Accessibility | Cloud computing, AI-as-a-Service, subscription models (starting around $20/month) |
Understanding AI Technologies Empowering Small Businesses
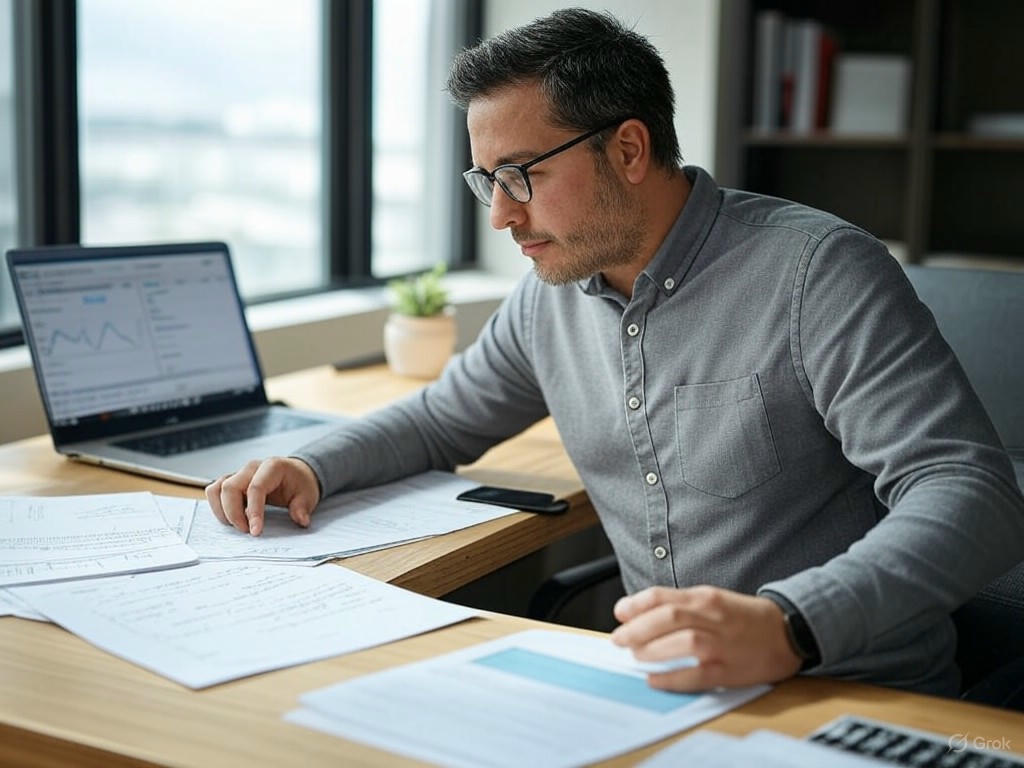
Understanding AI Technologies Empowering Small Businesses
What powers the AI tools that 77% of small businesses are using in 2025? To demystify this technology, let’s explore four key AI capabilities transforming small business operations today: generative AI, machine vision, predictive analytics, and robotic process automation (RPA). Understanding their technical foundations, performance metrics, and practical constraints will help business leaders cut through the hype and make informed decisions about AI adoption.
Generative AI: The Creative Engine
Generative AI, exemplified by models like OpenAI’s GPT series, has become a flagship technology in AI’s recent rise. These models are neural networks trained on massive text datasets, enabling them to generate human-like language, code, images, or music. Imagine a well-read librarian who has absorbed thousands of books and uses that knowledge to compose essays, answer questions, or brainstorm ideas on demand—that’s how GPT models operate at scale.
At their core, generative AI systems use a transformer architecture with self-attention mechanisms. This means the model dynamically weighs the importance of each word relative to others in a sentence, allowing it to consider long-range context and produce coherent, relevant outputs. This capability powers diverse applications such as writing marketing copy, summarizing reports, or running AI-powered chatbots.
However, generative AI is not without challenges. It demands vast training data and substantial computational resources, which can make initial implementation costly for small businesses. Additionally, these models occasionally generate plausible but incorrect “hallucinations,” so human oversight remains essential—especially when automating customer-facing communications. As seen with Jennifer’s Marketing Agency, success comes from aligning AI outputs with brand voice and verifying quality to maintain engagement and trust.
Machine Vision: Teaching Computers to See
If generative AI focuses on language, machine vision gives AI the ability to interpret images and video. Using neural networks called convolutional neural networks (CNNs), machine vision mimics aspects of human visual processing. Techniques like stereo vision employ two cameras to perceive depth, while methods such as laser triangulation or structured light project patterns to create precise 3D object models.
Small retailers often use machine vision to automate stock level tracking or product quality monitoring, reducing manual labor and errors. However, these systems require training on representative image datasets to achieve high accuracy and need careful calibration to handle challenges like variable lighting or cluttered environments. Latency can also be a concern when real-time processing is necessary.
Predictive Analytics: Forecasting the Future
Predictive analytics leverages historical data and machine learning to forecast outcomes such as sales trends, customer churn, or inventory shortages. Think of it as having a seasoned analyst who identifies patterns in past data to guide future decisions.
Unlike generative AI or machine vision, predictive analytics can start with simpler models like regression or decision trees, scaling up to complex algorithms as data and needs grow. Small businesses can now access user-friendly tools that integrate predictive insights into finance, marketing, or operations without requiring deep data science expertise.
Accuracy is critical: models trained on incomplete or biased data risk misleading decision-making. Latency is generally less critical since predictions often support strategic planning rather than real-time actions. A common hurdle for small businesses is collecting sufficient quality data to feed these models effectively.
Robotic Process Automation (RPA): Automating the Routine
RPA automates repetitive, rule-based tasks such as data entry, invoicing, or order processing. Unlike adaptive AI models, traditional RPA bots follow scripted workflows to mimic human interaction with software.
Think of RPA as a virtual assistant tirelessly handling mundane tasks, freeing employees for higher-value work. Modern RPA tools increasingly integrate AI to manage exceptions and improve over time.
For small businesses, RPA offers quick wins by reducing errors and speeding up processes without major IT overhauls. However, as workflows grow complex, scalability and maintenance become challenges. Over 90% of automation projects across industries fail, often due to poor alignment with business processes or inadequate planning, underscoring the importance of careful tool selection and implementation.
Performance Metrics and Practical Constraints
When evaluating AI technologies, small businesses should consider key performance metrics:
-
Accuracy: The frequency with which AI produces correct results. For example, a chatbot’s ability to accurately answer customer queries directly affects satisfaction.
-
Latency: The speed of AI responses. Real-time applications like chatbots or inventory monitoring require low latency, while batch predictions tolerate delays.
-
Data Requirements: AI thrives on data volume and quality. Limited datasets common in small businesses can constrain model performance or necessitate partnering with vendors offering pre-trained models.
These technical factors intersect with real-world constraints such as budget limits, staff AI literacy, data privacy regulations, and ethical considerations. For instance, generative AI outputs must be monitored to avoid copyright infringement or misaligned messaging, ensuring alignment with company values and customer trust.
Navigating Limitations with Strategic Adoption
Most small businesses start AI adoption with targeted, manageable projects—for example, using AI-powered chatbots to automate customer service queries or applying predictive analytics to optimize marketing campaigns. This incremental approach helps mitigate risks, control costs, and build internal AI fluency.
It’s crucial to recognize AI is not a “set it and forget it” solution. Continuous monitoring, model retraining, and workflow adjustments are necessary to sustain benefits and prevent pitfalls such as bias or data drift.
In summary, while AI technologies offer remarkable capabilities, their successful deployment depends on understanding both the technical workings and practical constraints. Small businesses that combine enthusiasm for AI with clear-eyed evaluation and ethical responsibility—as demonstrated by the case studies featured in this article—are best positioned to gain a competitive edge in today’s transformative business landscape.
AI Technology | Description | Technical Foundations | Performance Metrics | Practical Constraints | Use Cases |
---|---|---|---|---|---|
Generative AI | Generates human-like language, code, images, or music. | Transformer architecture with self-attention mechanisms, trained on massive text datasets. | Accuracy (quality of output), Latency (response speed) | High training data & computational resources; occasional hallucinations; requires human oversight. | Marketing copywriting, report summarization, AI chatbots. |
Machine Vision | Enables AI to interpret images and video. | Convolutional Neural Networks (CNNs), stereo vision, laser triangulation, structured light. | Accuracy (image recognition), Latency (real-time processing) | Needs representative image datasets, calibration challenges, lighting & environment sensitivity. | Stock tracking, product quality monitoring. |
Predictive Analytics | Forecasts outcomes using historical data and machine learning. | Regression, decision trees, complex ML algorithms. | Accuracy (forecast reliability), Latency (usually less critical) | Requires sufficient quality data; risk of bias; data collection challenges. | Sales trend forecasting, customer churn prediction, inventory management. |
Robotic Process Automation (RPA) | Automates repetitive, rule-based tasks. | Scripted workflows mimicking human interaction; integrates AI for exception handling. | Accuracy (error reduction), Latency (process speed) | Scalability and maintenance challenges; requires alignment with business processes. | Data entry, invoicing, order processing. |
Five Small Businesses Winning with AI: In-Depth Case Studies
Five Small Businesses Winning with AI: In-Depth Case Studies
What does AI success look like for a small business operating on a tight budget? The outdated notion that AI is only for tech giants with massive data lakes no longer holds true. Increasingly, small businesses across diverse sectors are leveraging AI tools to enhance efficiency, creativity, and revenue—while responsibly managing ethical considerations and data governance.
Below, we explore five compelling real-world cases demonstrating how AI can be a practical, scalable asset for small enterprises. Each example highlights the AI tools used, implementation strategies, challenges faced, measurable results, and how ethical and governance concerns were addressed—all framed within budget-conscious approaches that enable growth.
1. Content Creation Reinvented: Jennifer’s Marketing Agency
Jennifer’s small marketing agency faced a common challenge: consistently producing high-quality content without escalating costs. They integrated an AI-powered content creation tool, AIContentPad, which enabled a 30% increase in content output while cutting production costs by 62%. Engagement rates doubled, proving AI’s ability to streamline workflows and amplify creative impact.
Implementation was deliberate. Jennifer’s team trained the AI on brand voice templates and industry-specific themes to ensure outputs required minimal editing. This human-AI collaboration shifted creative staff focus from repetitive drafting to ideation and strategy. By choosing a customizable tool over costly bespoke platforms, they built scalability without overspending.
Ethical guardrails were integral from the start. The agency transparently disclosed AI’s role in content creation and employed plagiarism detection to maintain integrity. Sensitive client data used in AI training was carefully managed in line with emerging data governance best practices, reinforcing trust.
Key takeaway: Small teams can significantly boost content production quality and volume by thoughtfully customizing AI tools and maintaining transparency about AI involvement.
2. Virtual Auditions at Scale: Allcasting’s AI-Powered Casting Platform
In the entertainment industry, Allcasting revolutionized virtual auditions by deploying AI to analyze video submissions for performance quality, appearance, and casting criteria. This automation dramatically reduced manual review time, enabling the small team to efficiently process a surge in applicants with limited staff.
Their AI workflows leveraged cloud-based tools like Microsoft 365 Copilot to automate administrative tasks, alongside agentic AI for optimizing scheduling and communication. Freed from routine logistics, human resources focused on creative decision-making and client engagement.
Budget constraints were managed by selecting pay-as-you-go AI services, avoiding heavy upfront costs. Scalability was built-in—AI algorithms dynamically adjusted to handle increasing volumes without proportional staff increases.
Ethical considerations were prioritized. Allcasting implemented strong data privacy protocols, encrypting audition submissions and restricting access. To mitigate bias, they regularly audited AI outputs and maintained human oversight to identify anomalies, ensuring fair and responsible assessments.
Key takeaway: AI can democratize access and expand operational capacity in niche markets like virtual casting, provided robust governance protects privacy and mitigates bias.
3. Marketing Optimization: A Local Retailer’s AI-Enhanced Campaigns
Facing tough competition, a small retailer adopted AI marketing tools including Sprout Social, Semrush, and ChatGPT to optimize campaigns. Utilizing AI for audience segmentation, personalized content, and scheduling, they reduced marketing time by 25% and increased lead conversions by 30%.
The retailer chose affordable SaaS platforms with intuitive interfaces, minimizing the team’s learning curve. AI-generated social media posts and emails preserved brand voice through templates and iterative human editing, balancing automation with authenticity.
Data privacy and compliance posed challenges amid evolving regulations. The business proactively implemented data governance frameworks—encrypting customer data and limiting AI training to anonymized datasets. Transparent communication about AI’s marketing role helped sustain customer trust.
Key takeaway: Small businesses can enhance marketing effectiveness and efficiency by integrating accessible AI tools while upholding ethical data stewardship and human creativity.
4. Inventory Management: A Specialty Food Store’s AI Leap
Inventory mismanagement drains resources for many small retailers. A specialty food store implemented AI-driven demand forecasting tools that analyze sales trends, seasonality, and external factors like weather to optimize stock levels. This reduced overstock by 20% and stockouts by 15%, improving cash flow and customer satisfaction.
The store selected cloud-based AI solutions integrated with their existing point-of-sale system, minimizing operational disruption and enabling smooth scaling as business expanded. Staff training was focused and concise, empowering the team to interpret AI insights without needing deep technical expertise.
Ethical practices included transparency with suppliers regarding AI use and strict adherence to data privacy standards for customer purchase data. The store committed to periodic AI system audits to detect drift or bias, ensuring ongoing reliability.
Key takeaway: Even modest AI investments in operations such as inventory forecasting can yield significant cost savings and service improvements when integration and governance are thoughtfully managed.
5. Investment Analytics: A Financial Advisory Startup’s AI Edge
A lean financial advisory startup adopted AI-powered analytics platforms to enhance portfolio management and client reporting. AI tools sifted through market data to generate risk assessments, accelerating decision-making and enabling personalized investment advice.
Given the sensitive nature of financial data, the startup selected AI services aligned with regulatory compliance requirements. They layered human judgment over AI-generated insights, acknowledging that fiduciary responsibility demands ethical oversight beyond automation.
Cost control was achieved by leveraging AI-as-a-service platforms rather than developing proprietary models. Scalability was inherent—AI handled increasing data complexity as client numbers grew without a proportional increase in human workload.
Transparency was central. The firm openly disclosed AI’s role in analytics to clients and maintained rigorous data security protocols, anticipating stricter AI regulations in finance.
Key takeaway: AI can augment specialized advisory services by delivering actionable insights at scale, but ethical and regulatory vigilance remains essential.
Common Themes and Lessons Learned
-
Budget-Conscious Scalability: All businesses prioritized accessible AI platforms with flexible pricing and cloud integration, avoiding costly custom developments.
-
Human-AI Collaboration: AI augmented rather than replaced human expertise, preserving creativity, judgment, and ethical oversight.
-
Ethical and Data Governance: Proactive transparency, data privacy, bias mitigation, and compliance frameworks were foundational, not afterthoughts.
-
Measurable Outcomes: AI adoption drove productivity gains of 20–60%, cost reductions of 25–60%, and engagement or conversion improvements of 30–100%.
-
Implementation Challenges: Common hurdles included learning curves and data privacy concerns, underscoring the importance of training and clear governance policies.
These case studies dispel the myth that AI is out of reach for small businesses. When deployed thoughtfully—with attention to ethics and data governance—AI becomes a powerful equalizer, unlocking new levels of efficiency and innovation across diverse industries.
For small businesses ready to experiment, these examples provide a grounded roadmap to integrate AI effectively, responsibly, and sustainably.
Business | AI Tools Used | Implementation Strategies | Challenges Faced | Measurable Results | Ethical & Governance Actions |
---|---|---|---|---|---|
Jennifer’s Marketing Agency | AIContentPad | Customized AI on brand voice templates; human-AI collaboration; chose customizable tool over bespoke platforms | Maintaining content quality; managing sensitive client data | 30% increase in content output; 62% cost reduction; doubled engagement rates | Transparent AI disclosure; plagiarism detection; careful data governance |
Allcasting’s AI-Powered Casting Platform | Microsoft 365 Copilot; agentic AI | Cloud-based pay-as-you-go services; automated administrative tasks and scheduling | Data privacy; mitigating bias in AI assessments | Reduced manual review time; scalable applicant processing without staff increase | Data encryption; access restrictions; AI output audits; human oversight |
Local Retailer’s AI-Enhanced Campaigns | Sprout Social; Semrush; ChatGPT | Affordable SaaS tools with intuitive interfaces; AI-generated content with human editing | Data privacy compliance; evolving regulations | 25% reduced marketing time; 30% increased lead conversions | Data encryption; anonymized datasets; transparent AI use communication |
Specialty Food Store’s Inventory Management | AI-driven demand forecasting tools | Cloud integration with POS system; focused staff training | Ensuring AI reliability; data privacy with customer purchase data | 20% reduced overstock; 15% reduced stockouts | Transparency with suppliers; data privacy adherence; periodic AI audits |
Financial Advisory Startup’s Investment Analytics | AI-powered analytics platforms | AI-as-a-service; layered human judgment over AI insights | Regulatory compliance; fiduciary responsibility | Faster risk assessments; scalable client data handling | Open AI role disclosure; rigorous data security; compliance alignment |
Comparative Analysis: Benchmarking AI Adoption Across Small Business Models
Comparative Analysis: Benchmarking AI Adoption Across Small Business Models
What differentiates small businesses that thrive with AI from those that struggle? The answer lies in a nuanced combination of industry context, organizational readiness, resource allocation, and a realistic assessment of AI maturity. By analyzing five successful small business case studies alongside global SME trends, we can uncover patterns that clarify AI adoption and reveal common pitfalls to avoid.
Common Threads and Divergent Paths in AI Adoption
Globally, about 75% of small and medium-sized businesses (SMBs) are experimenting with AI, with 90% of those reporting revenue gains directly tied to AI initiatives (Salesforce). Yet, this widespread enthusiasm conceals significant variation beneath the surface.
Key commonalities among successful AI adopters include:
-
Strategic Alignment: Small businesses that integrate AI into their core operations—not as isolated pilot projects—tend to outperform. For instance, manufacturing SMEs adopting predictive maintenance AI have achieved measurable efficiency improvements by embedding these tools within their production workflows (Gorillias).
-
Incremental Scaling: Rather than undertaking a full-scale AI transformation, effective adopters begin with targeted use cases, often in marketing automation or customer service, and expand based on clear, measurable outcomes (Moveworks).
-
Employee Engagement: Contrary to some leadership fears, employees frequently embrace AI tools when they recognize tangible benefits, such as automating repetitive tasks and augmenting their roles (McKinsey).
However, differences become evident when considering industry type and organizational maturity:
-
Industry Influence: Tech-focused sectors and service operations lead AI adoption, reflecting both the availability of AI solutions and easier integration. Retail SMEs typically emphasize digital marketing AI, while manufacturing SMEs prioritize operational AI like predictive analytics (OECD, Hostinger).
-
AI Maturity Levels: Though most businesses invest in AI, only about 1% consider themselves mature adopters (McKinsey). Many small businesses face fragmented AI strategies and underdeveloped governance, which limit their ability to realize full value.
-
Resource Availability: Larger small businesses—those with more employees and higher revenues—allocate more substantial budgets to AI tools and training. This broader investment supports adoption across departments and yields higher returns on investment (Service Direct).
Factors Driving AI Success in Small Businesses
What truly propels AI initiatives from experimentation to transformative assets? Four critical factors stand out:
-
Organizational Readiness and Culture
Successful AI adoption hinges less on technology and more on fostering a culture ready to embrace change. Leadership that clearly articulates an AI vision aligned with strategic goals facilitates smoother adoption. Embedding AI as a natural extension of business processes avoids siloed projects that fail to gain traction (Lantern, Dallas Innovates).
-
Focused Use Case Selection
Small businesses that identify three to six high-impact AI use cases—such as chatbots automating customer inquiries or AI-driven inventory management—achieve faster wins and build internal confidence (Moveworks, Stellar). This pragmatic approach contrasts with overambitious efforts that overwhelm limited resources.
-
Data Strategy and Governance
AI’s power depends on quality data. Many small businesses struggle with fragmented data sources and weak governance, resulting in inconsistent AI outcomes. Establishing unified data access and governance frameworks—even at a modest scale—improves reliability and compliance (LinkedIn Hari Prasad Govindarajan).
-
Resource Allocation and External Partnerships
Budget constraints and talent shortages remain major barriers. However, leveraging cloud-based AI platforms and partnering with specialized vendors can offset these challenges. For example, a textile SME’s targeted investment of €58,000 in a predictive AI system significantly enhanced loom performance, illustrating how focused spending can yield outsized returns (Gorillias).
Measuring Impact: Efficiency, Customer Experience, and Competitive Advantage
When AI delivers, benefits arise across several dimensions:
-
Efficiency Gains: AI-powered automation tools such as Microsoft 365 Copilot help small businesses reduce workflow bottlenecks and lower operational costs. Service operations report cost savings up to 49% attributed to AI-driven efficiencies (Microsoft Blog, Hostinger).
-
Enhanced Customer Experience: AI chatbots and predictive analytics enable personalized interactions and faster problem resolution. Small businesses leveraging AI-powered customer service report quicker response times and higher satisfaction scores, translating into repeat business and increased loyalty (Net2Phone).
-
Competitive Edge: Early AI adopters position themselves as innovators in crowded markets. Automating routine tasks frees human capital to focus on creativity and strategic initiatives—a key differentiator when competing against larger firms (McKinsey, Orion Policy Institute).
Yet, not all AI journeys succeed. Instances where adoption fell short offer instructive lessons:
-
Overestimating AI as a Cure-All: Some small businesses mistakenly expect generative AI or large language models to automatically solve complex challenges without proper process redesign or data readiness, leading to wasted investment and disappointment (Orion Policy Institute).
-
Shadow AI Risks: Informal, ungoverned AI use by employees—known as “Shadow AI”—introduces security vulnerabilities and compliance risks. Approximately 68% of AI users in SMEs do not inform management, while 14% of managers forbid AI use, highlighting a disconnect that undermines coordinated strategy (Gorillias).
-
Lack of Trust and Understanding: Persistent concerns about data privacy, ethics, and cost hinder adoption. Nearly 78% of small businesses that have not adopted AI cite lack of understanding or implementation barriers as primary reasons (Service Direct).
Synthesizing Insights: Key Takeaways for Small Businesses
AI adoption is a marathon, not a sprint. Small businesses aiming to succeed with AI should consider the following:
-
Invest in organizational readiness and leadership that champions AI as a strategic enabler, not just a technology novelty.
-
Prioritize AI use cases offering clear ROI and manageable complexity, especially in customer-facing and operational areas.
-
Establish foundational data governance and integrate AI seamlessly into existing workflows to maximize impact.
-
Leverage cloud and platform-based AI solutions to overcome resource constraints, while maintaining vigilance on security and ethical considerations.
Ultimately, AI is a tool demanding deliberate, context-aware application. Success stories show that when small businesses combine technology with thoughtful strategy and cultural buy-in, AI transforms from buzzword to tangible business advantage. Ignoring these fundamentals risks missed opportunities and unnecessary risks—reminding us that AI’s promise depends as much on people and processes as on algorithms.
Aspect | Successful AI Adopters | Challenges/Barriers |
---|---|---|
Strategic Alignment | AI integrated into core operations; targeted use cases; incremental scaling | Fragmented AI strategies; siloed pilot projects |
Industry Influence | Tech and service sectors lead adoption; Retail focuses on marketing AI; Manufacturing on operational AI | Lower AI maturity in certain sectors; limited solution availability |
Organizational Readiness | Leadership champions AI; culture embraces change; employee engagement positive | Lack of leadership vision; employee resistance; shadow AI risks |
Resource Allocation | Larger SMBs invest more in AI tools and training; use of cloud platforms and partnerships | Budget constraints; talent shortages; uncoordinated investments |
AI Maturity | Clear data governance; unified data access; measurable outcomes | Only ~1% mature adopters; weak governance; inconsistent data |
Measurable Impact | Efficiency gains (up to 49% cost savings); enhanced customer experience; competitive advantage | Overestimating AI capabilities; lack of trust and understanding |
Common Pitfalls | Focused use cases; pragmatic scaling; strong data strategy | Overambitious efforts; shadow AI; privacy and ethical concerns |
Ethical, Regulatory, and Data Governance Considerations for SMEs Using AI
Ethical, Regulatory, and Data Governance Considerations for SMEs Using AI
How can small businesses harness AI’s benefits while avoiding ethical pitfalls and regulatory challenges? This question is central to AI adoption in 2025, especially for SMEs balancing limited resources with growing demands around fairness, privacy, and compliance.
Navigating Ethical Dilemmas: Bias, Transparency, Privacy, and Consent
Ethical challenges in AI are often underestimated by small businesses. Bias in AI models is not a distant theoretical concern; it can directly impact critical decisions like hiring, customer segmentation, or credit evaluations—areas where fairness is essential.
Emerging tools such as fairness-aware algorithms and thorough data audits offer SMEs practical ways to mitigate bias. However, these solutions require upfront investment in expertise and disciplined processes, which can be challenging for resource-constrained teams.
Transparency poses another significant challenge. Without explainable AI (XAI), SMEs risk deploying “black box” models that neither customers nor employees can understand. This opacity harms trust and can lead to non-compliance with regulations demanding clarity on automated decisions. For instance, a retail SME using AI-powered credit scoring should be able to explain why a customer was declined, not just provide a yes/no outcome.
Data privacy and informed user consent remain the most urgent ethical concerns. AI’s appetite for data often conflicts with consumers’ expectations for control over their personal information. According to Secure Privacy, consumers in 2025 demand meaningful control, pushing SMEs to rethink their data collection, retention, and usage strategies.
Privacy-enhancing technologies (PETs), like data anonymization and differential privacy, help safeguard sensitive information while still enabling AI-driven insights. Equally important is obtaining clear, informed consent. The era of vague privacy policies is over. SMEs must transparently disclose what data they collect, how it will be used, and provide granular consent options.
Failure in this area risks regulatory penalties and customer alienation. The recent controversy around Meta’s AI training practices—using adult users’ public content but excluding minors and private messages—highlights the delicate balance SMEs must strike between innovation and ethical responsibility.
Understanding the Regulatory Landscape Impacting SMEs
The regulatory landscape for AI is rapidly evolving and increasingly complex, especially for SMEs. The EU AI Act, effective since August 2024, imposes risk-based requirements that small businesses must navigate carefully. Non-compliance can result in fines of up to 6% of global turnover—an especially heavy burden for smaller firms.
In the U.S., AI-specific laws are emerging at the state level. For example:
-
Virginia’s HB 2124 criminalizes synthetic digital content used in fraud.
-
Montana’s Right to Compute Act requires AI risk management policies for systems controlling critical infrastructure.
This patchwork of regulations complicates compliance for SMEs operating across multiple states.
Beyond AI-specific rules, foundational data protection laws like GDPR, CCPA, and newer state privacy laws remain critical. SMEs must implement strong data governance practices to ensure data accuracy, security, and lawful processing.
Cloud-native architectures and hybrid work setups add complexity to data flows but can also offer scalable pathways to compliance.
Fortunately, tailored guidance is becoming available. Standards like CAN/DGSI 101:2025 offer ethical AI design principles specifically for organizations with fewer than 500 employees, providing a practical framework to reduce risk and build trust.
Moreover, regulatory sandboxes under the EU AI Act allow SMEs to test AI solutions in controlled environments, easing the path toward compliance and innovation.
Best Practices for Responsible AI Governance in SMEs
AI governance need not be overwhelming or costly for small businesses. Scaled governance frameworks can unlock operational efficiencies and strengthen reputations.
Key best practices include:
-
Establish Clear AI Ethics Principles: Define core values such as fairness, transparency, accountability, and privacy early. Embedding these into your business strategy aligns AI use with customer expectations and societal norms.
-
Leverage SME-Friendly Governance Tools: Affordable platforms now simplify oversight, compliance monitoring, and real-time risk detection without requiring in-house AI ethics expertise.
-
Conduct Regular Data Audits and Bias Assessments: Periodic reviews of training data and model outputs help uncover unintended biases and data quality issues before they affect decisions.
-
Prioritize Privacy by Design: Incorporate data minimization, encryption, and granular consent management from the outset to reduce vulnerabilities and regulatory risks.
-
Train and Engage Employees: Building AI literacy and ethical awareness among staff fosters a culture of responsible AI use. Monthly security workshops and recognition programs for compliance adherence can make a tangible difference.
-
Communicate Transparently with Customers: Clearly explain AI’s role in your products or services, how decisions are made, and how data is protected. Inviting customers into iterative feedback loops enhances loyalty and trust.
-
Start Small and Iterate: Pilot AI projects with ethical guardrails in place, scaling only after demonstrating effectiveness and trustworthiness. This approach helps avoid costly mistakes and reputational damage.
-
Stay Informed on Regulations: Regularly consult legal experts and participate in AI governance summits or utilize updated standards to keep pace with evolving rules.
Building Trust: The Competitive Edge for SMEs
Ethical AI governance is more than just compliance—it’s a strategic advantage. In 2025, customers increasingly prefer brands they trust with their data and values.
SMEs that proactively address ethical concerns can strengthen brand loyalty, avoid costly penalties, and responsibly unlock AI’s transformative potential.
The balance is delicate but achievable. Use AI to augment human creativity and efficiency without sacrificing authenticity or fairness. By embedding transparency, privacy, and accountability into AI initiatives, small businesses can not only survive but thrive in this new era of intelligent automation.
In summary, managing ethical dilemmas, regulatory complexity, and governance challenges is essential for SMEs deploying AI. With tailored standards, emerging governance tools, and a commitment to transparency and fairness, small businesses can successfully navigate these challenges—building trust and sustainable growth in an AI-powered future.
Consideration | Details | Examples / Tools |
---|---|---|
Ethical Dilemmas | Bias, Transparency, Privacy, and Informed Consent are key challenges; fairness-aware algorithms and data audits help mitigate bias; explainable AI needed for transparency; privacy-enhancing technologies protect data. | Fairness-aware algorithms, Data audits, Explainable AI (XAI), Data anonymization, Differential privacy |
Regulatory Landscape | Complex and evolving regulations including EU AI Act, state laws in the U.S., foundational data protection laws; non-compliance risks heavy fines; cloud and hybrid setups impact data flows. | EU AI Act (since Aug 2024), Virginia HB 2124, Montana Right to Compute Act, GDPR, CCPA, CAN/DGSI 101:2025 standard, Regulatory sandboxes |
Best Practices for AI Governance | Establish ethics principles, use SME-friendly governance tools, perform data audits, prioritize privacy by design, train employees, communicate transparently, start small and iterate, stay informed on regulations. | AI ethics principles, Compliance monitoring platforms, Data audits, Encryption, Consent management tools, Employee training programs, Legal consultation |
Building Trust | Ethical AI governance as competitive edge; enhances brand loyalty, avoids penalties, enables responsible AI use; balance between automation and fairness. | Transparent communication, AI ethical initiatives, Customer feedback loops |
Future Trends and Emerging Opportunities: What Small Businesses Should Watch
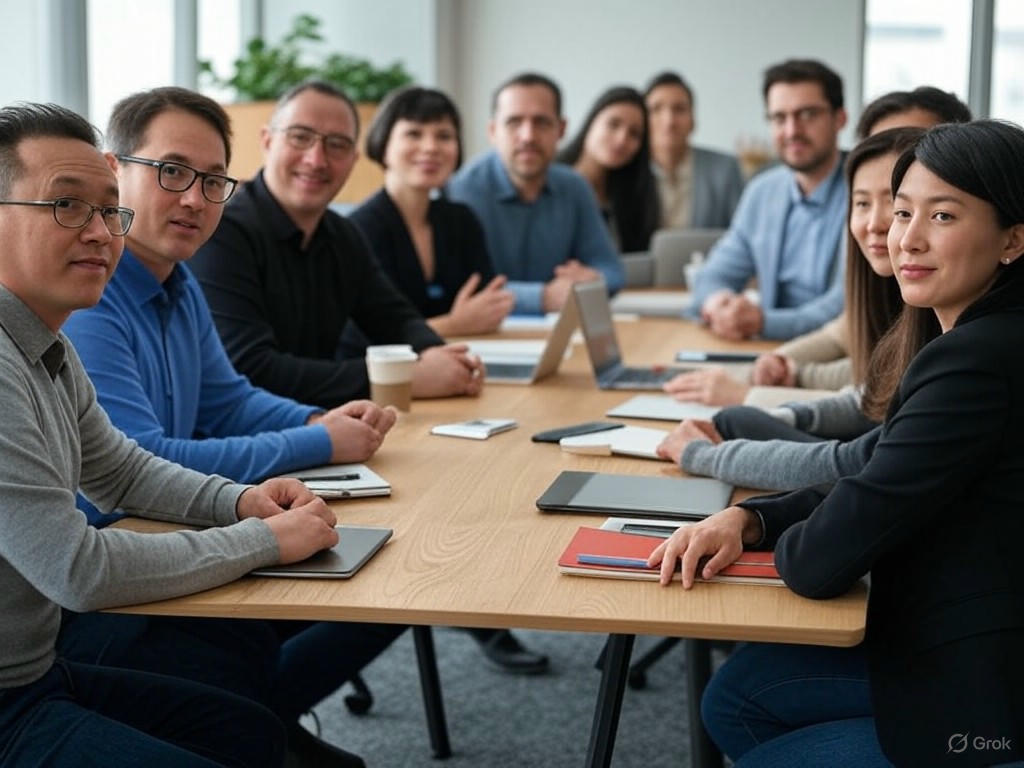
Future Trends and Emerging Opportunities: What Small Businesses Should Watch
What does the next wave of AI innovation mean for small businesses striving to compete and thrive? The landscape is evolving rapidly—not only in terms of AI’s capabilities but also in accessibility, organizational impact, and competitive dynamics. Let’s explore the key trends shaping the small business AI frontier in 2025 and beyond.
Revolutionary Advances in Generative AI: Lowering Barriers, Raising Stakes
Generative AI models are no longer exclusive to tech giants or research labs. By 2025, small and medium-sized businesses (SMBs) are increasingly accelerating their AI investments—35% slightly accelerating and 27% significantly accelerating, according to the SMB Group. This surge is driven by a new generation of generative AI tools that balance powerful capabilities with user-friendly design.
For example, tools like Jasper provide intuitive interfaces for content creation, while DALL·E 3 is freely accessible through ChatGPT and Microsoft 365 Copilot, democratizing AI-powered image generation. Knowledge management platforms such as Notion AI and Mem ease cognitive load by automatically tagging and connecting notes—helpful for busy entrepreneurs managing multiple priorities.
This accessibility doesn’t just improve convenience—it levels the playing field. Small businesses can now quickly prototype marketing campaigns, generate multimedia assets, and automate customer interactions without deep technical expertise. However, this democratization also introduces new challenges to navigate:
- Signal vs. noise: The abundance of AI tools makes selecting the right solutions critical.
- Custom vs. off-the-shelf: While custom GPTs tailored to specific business needs are emerging, many small businesses rely on third-party applications, which may create dependencies or integration challenges.
- Ethical considerations: As generative AI outputs grow more pervasive, small businesses must address content authenticity, algorithmic bias, and copyright compliance.
The future of generative AI is not simply “plug and play.” Success requires strategic adoption, ongoing AI literacy, and an ethical framework aligned with business goals.
AI-as-a-Service and IoT: Synergizing for Operational Excellence
AI and the Internet of Things (IoT) are becoming complementary forces that amplify operational efficiency for small businesses. AI-as-a-Service (AIaaS) platforms simplify access by eliminating the need to build complex AI infrastructure from scratch.
With over 32 billion connected IoT devices projected by 2030 (Statista), small businesses have unprecedented data streams to optimize everything from inventory to customer experience. Edge computing—processing data close to its source—reduces latency, enabling faster, real-time decision-making.
Practical examples include:
- AerisWeather feeding daily environmental data into Google Sheets, supporting agricultural or outdoor service operations.
- Workforce management apps that update timesheets and incident reports automatically based on IoT sensor inputs.
- Predictive maintenance in manufacturing SMEs, where AI analyzes sensor data to forecast equipment failures, minimizing costly downtime.
No-code and low-code platforms make these AIoT integrations accessible even without dedicated IT teams. Yet, security remains paramount. Small businesses must prioritize device authentication, data encryption, and regular firmware updates to protect their AIoT ecosystems from vulnerabilities.
The Evolving AI Ecosystem: Competition, Democratization, and Organizational Shifts
The AI ecosystem today is a dynamic and sometimes turbulent space. In the U.S. alone, over 2,000 AI companies operate, with hundreds of new entrants each year. Open-source foundational models and cloud platforms like AWS, Microsoft Azure, and Google Cloud dramatically expand access to AI technologies.
For small businesses, this means:
- Access to powerful AI tools once limited to large enterprises.
- Customization opportunities through low-code/no-code platforms and open-source frameworks like TensorFlow and PyTorch.
- Increased competition as AI lowers barriers to entry and enables nimble startups to challenge established players.
However, democratization brings challenges. Regulatory scrutiny is intensifying with over 900 AI-related bills introduced across U.S. states. Concerns about dominant platforms creating “bottlenecks” highlight the need for vigilance. Small businesses must balance innovation with compliance, ethical AI use, and transparency.
Organizationally, AI is reshaping work. Microsoft’s Work Trend Index reports that 82% of leaders expect to expand their workforce with AI in the next 12–18 months. For small businesses, this translates to:
- Empowering employees to focus on higher-value, strategic tasks by offloading routine work to AI.
- Investing in skilling teams to unlock AI’s full potential.
- Treating AI as a durable, affordable resource—a core business tool rather than a temporary experiment.
Proactive Steps for Small Businesses: Preparing to Innovate and Lead
How can small businesses harness these emerging AI trends rather than be overwhelmed by them? Consider these practical strategies:
- Focus on specific problems, not broad AI hype. Identify operational pain points where AI can deliver measurable improvements—such as automating customer support or optimizing supply chains.
- Pilot AI projects using scalable, accessible tools. Leverage AIaaS platforms and no-code integrations to experiment cost-effectively before making larger investments.
- Invest in AI literacy and team skilling. Build knowledge to understand AI’s capabilities and limitations, fostering responsible use and continuous innovation.
- Stay informed on regulatory developments. Proactively address data privacy, ethical considerations, and security to build trust with customers and partners.
- Leverage ecosystem partnerships. Collaborate with AI vendors, open-source communities, and local tech hubs to gain expertise and share best practices.
- Utilize AI-powered analytics. Democratized data tools can uncover new growth opportunities and support data-driven decision-making.
Closing Thoughts
The AI revolution for small businesses is no longer a distant promise—it’s unfolding now. Generative AI, AIaaS combined with IoT integration, and a rapidly evolving ecosystem are converging to create unprecedented opportunities alongside new challenges.
Success won’t come from chasing every new tool but from thoughtful, evidence-based adoption that respects ethical principles and organizational realities. Small businesses that approach AI strategically—embracing both its power and complexities—will not only survive the AI wave but thrive and redefine their industries.
Trend | Description | Examples / Strategies | Challenges / Considerations |
---|---|---|---|
Revolutionary Advances in Generative AI | Increased accessibility of generative AI tools, accelerating AI investment by SMBs. | Jasper (content creation), DALL·E 3 (image generation), Notion AI and Mem (knowledge management) | Selecting the right tools, custom vs off-the-shelf tradeoffs, ethical concerns (authenticity, bias, copyright) |
AI-as-a-Service and IoT Integration | Synergizing AI with IoT to enhance operational efficiency and real-time decision-making. | AerisWeather data in Google Sheets, workforce management apps, predictive maintenance in manufacturing | Security risks including device authentication, data encryption, firmware updates |
Evolving AI Ecosystem | Expanding number of AI companies and open-source tools democratizing AI access and customization. | Use of AWS, Azure, Google Cloud, TensorFlow, PyTorch; low-code/no-code platforms | Regulatory scrutiny, competition increase, risk of platform bottlenecks, need for compliance and transparency |
Organizational AI Impact | AI reshaping workforce roles and business operations, focusing on strategic tasks and AI as core resource. | Empowering employees, investing in AI skill development, treating AI as durable business tool | Managing change, continuous team training, balancing AI adoption with organizational goals |
Proactive Small Business Strategies | Practical steps to adopt AI effectively and responsibly. | Focus on specific problems, pilot projects with AIaaS, invest in literacy, monitor regulations, leverage partnerships, use AI analytics | Avoiding hype, ensuring ethical use, maintaining security, fostering innovation |
Conclusion: Synthesizing Lessons and Strategic Recommendations for Small Businesses
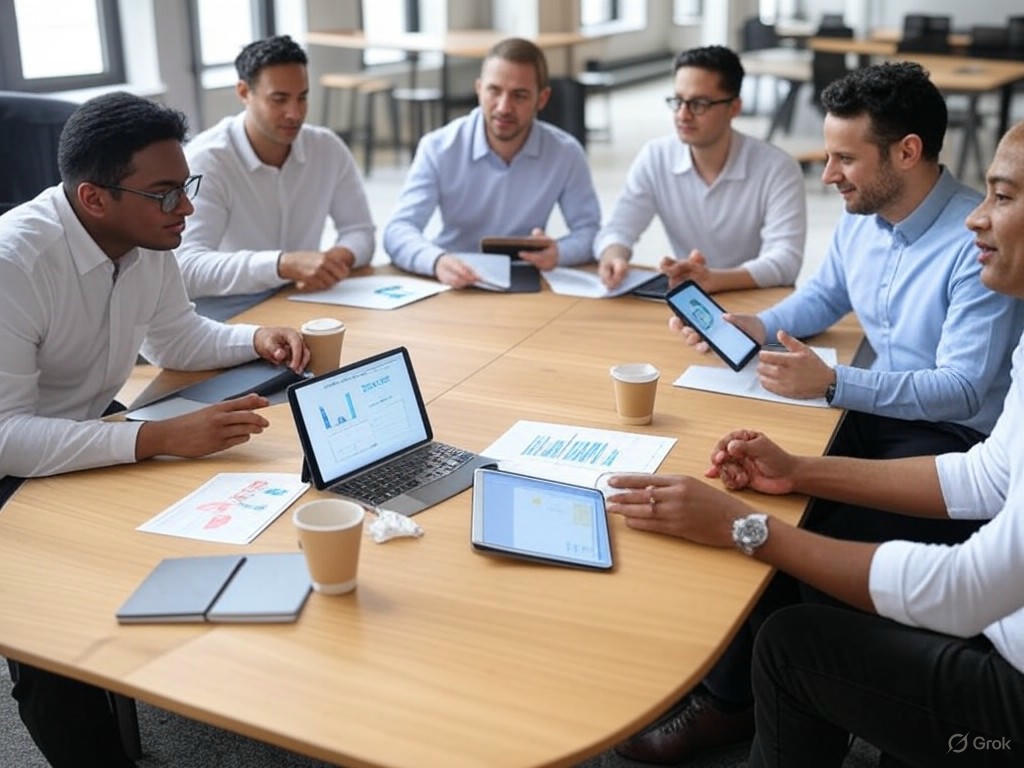
Conclusion: Synthesizing Lessons and Strategic Recommendations for Small Businesses
What can five diverse small businesses teach us about AI adoption? The clear takeaway is that AI offers tangible, pragmatic advantages, yet the journey is far from straightforward. From enhancing marketing efficiency to streamlining customer service and optimizing back-office operations, AI is no longer a futuristic concept—it is a real, accessible enabler of growth and competitiveness for small enterprises.
Pragmatic Benefits and Real-World Challenges
Recent data underscores a striking reality: 77% of small businesses have embraced AI in some form, primarily focusing on sales, marketing, and customer support. For instance, AI-powered chatbots can now handle up to 80% of routine customer inquiries, freeing staff to focus on higher-value tasks. These accessible tools often deliver quick wins, especially for smaller outfits with limited budgets, as seen in case studies like Jennifer’s Marketing Agency and the Local Retailer.
However, the AI adoption path is filled with complexities. A McKinsey study reveals that only about 1% of companies consider themselves mature in AI deployment. This mirrors what many small business leaders experience: enthusiasm tempered by uncertainty about scaling AI effectively. Common hurdles include:
- Limited AI literacy and understanding within teams
- Budget constraints restricting access to advanced tools
- Challenges integrating AI with legacy systems
- Increasing demands for privacy and data protection as AI usage expands
- Resistance to organizational change and cultural barriers
These challenges are not hypothetical; they arise in everyday operations where balancing simplicity and capability requires careful management. Notably, businesses serving other businesses tend to have a more advanced AI footprint, likely due to stronger revenues and resource availability.
Strategic Recommendations for AI Adoption
If the benefits and challenges are clear, what practical steps should small business leaders take to harness AI effectively?
1. Choose AI Solutions That Complement Your Business
Opt for AI tools designed to enhance existing workflows rather than disrupt them radically at first. Solutions that are user-friendly and integrate smoothly increase adoption rates among staff. For example, marketing teams benefit from AI tools like Fireflies.ai for conversation analytics and Grammarly for content quality assurance, which fit neatly into current processes.
2. Invest in Continuous Learning and Staff Upskilling
AI is not a “set-and-forget” technology. Cultivating a culture of ongoing education helps teams stay current with evolving tools and reduces resistance. Partnering with AI vendors who offer training and support can be transformative, as illustrated by the Specialty Food Store’s approach to team training on AI-driven demand forecasting tools.
3. Prioritize Ethical AI Practices
Ethical considerations extend beyond mere compliance. Small businesses should:
- Develop transparent AI use policies
- Monitor AI outputs to detect and mitigate bias or inaccuracies
- Ensure robust data privacy and security management
- Disclose AI use to customers when appropriate to build trust
Ethical AI adoption safeguards brand reputation and fosters long-term customer loyalty, as seen in Allcasting’s rigorous data privacy protocols and human oversight.
4. Measure Impact with Both Quantitative and Qualitative Metrics
ROI encompasses more than cost savings or sales increases. Assess how AI transforms workflows and team dynamics. Metrics such as time saved, customer satisfaction, and employee engagement provide a fuller picture of AI’s value, reflecting the comprehensive benefits reported across our case studies.
Balancing Enthusiasm with Critical Assessment
AI’s promise is compelling, but uncritical enthusiasm risks costly missteps. Data shows employees are often more ready to embrace AI than leaders expect, yet thoughtful governance remains essential to avoid pitfalls like data mishandling, algorithmic bias, or operational failures.
Small business leaders should adopt a mindset that balances innovation with risk management by:
- Conducting regular AI risk assessments
- Consulting legal counsel to navigate evolving regulations
- Maintaining human oversight in AI decision-making
This balanced approach is vital as AI continues to reshape business models and competitive landscapes.
Looking Ahead: AI’s Evolving Role in Small Business Futures
The AI revolution is not a simple on/off switch but a continuum. PwC projects AI could add $15.7 trillion to the global economy by 2030, with small businesses playing a crucial role in this transformation.
Emerging trends include:
- Increasing automation of routine tasks, freeing entrepreneurs to focus on strategy and creativity
- More sophisticated AI tools tailored for smaller budgets and specific industries
- Heightened importance of data integrity and security as AI becomes deeply embedded
- Expanded AI applications in areas like HR, supply chain, and financial forecasting
For small businesses willing to invest strategically, AI is more than a tool—it is a catalyst for resilience and scalable growth. Success lies in navigating complexity with clarity, ethics, and a continuous willingness to learn.
Key Takeaways for Small Business Leaders:
- Start small with AI applications that deliver quick, measurable wins.
- Choose adaptable, user-friendly tools that fit your existing workflows.
- Foster an ethical AI culture rooted in transparency and accountability.
- Measure impact beyond financial metrics to include team and customer benefits.
- Balance enthusiasm for AI with ongoing risk assessment and governance.
AI is reshaping the future of small business, but it demands more than technology adoption—it requires strategic vision and thoughtful leadership. The small businesses winning with AI today are those that understand this nuance and act accordingly.
Category | Details |
---|---|
Pragmatic Benefits | AI enhances marketing efficiency, customer service, and back-office operations; 77% of small businesses use AI mainly in sales, marketing, and support; AI chatbots handle up to 80% of routine inquiries. |
Challenges | Limited AI literacy, budget constraints, legacy system integration issues, privacy/data protection demands, resistance to change; only ~1% companies mature in AI deployment. |
Strategic Recommendations | 1. Choose AI tools complementing workflows (e.g., Fireflies.ai, Grammarly). 2. Invest in continuous learning and upskilling. 3. Prioritize ethical AI practices (transparency, bias monitoring, data privacy). 4. Measure impact with quantitative and qualitative metrics. |
Balanced Approach | Regular AI risk assessments, legal consultation, human oversight to avoid data mishandling, bias, operational failures. |
Future Trends | Increased automation, more tailored AI tools, importance of data integrity/security, expanded AI in HR, supply chain, financial forecasting. |
Key Takeaways | Start small, choose adaptable tools, foster ethical AI culture, measure beyond finances, balance enthusiasm with governance. |